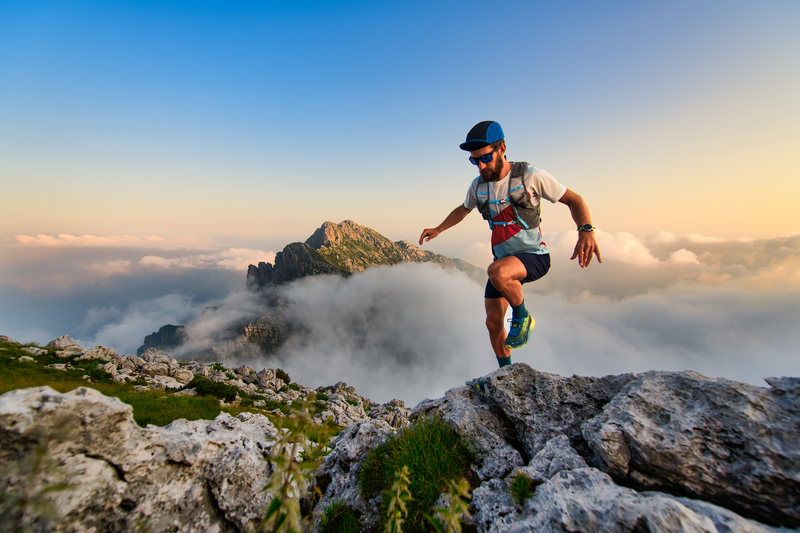
94% of researchers rate our articles as excellent or good
Learn more about the work of our research integrity team to safeguard the quality of each article we publish.
Find out more
EDITORIAL article
Front. Oncol. , 03 March 2025
Sec. Gynecological Oncology
Volume 15 - 2025 | https://doi.org/10.3389/fonc.2025.1568579
This article is part of the Research Topic Insights, Controversies, and New Developments in the Initial Treatment Decisions for Advanced Epithelial Ovarian Cancer View all 7 articles
Editorial on the Research Topic
Insights, controversies, and new developments in the initial treatment decisions for advanced epithelial ovarian cancer
Epithelial Ovarian Cancer (EOC) is one of the deadliest types of gynecological malignancies, especially in advanced cases, and the five-year survival rate remains low. Despite recent advances in surgical techniques, chemotherapy regimens, and targeted therapies, initial treatment decision making remains challenging. How to balance the effect of treatment and the quality of life of patients, how to find the best balance between individualized treatment and standardized treatment, is the core problem in current clinical practice.
This topic includes a number of high-quality research articles, covering a wide range of aspects from basic research to clinical practice. Here is a brief overview of a few representative articles:
This study developed machine learning models to predict platinum-resistant recurrence in epithelial ovarian cancer (EOC) using clinical and laboratory data from 1,392 patients. Five algorithms (DTA, KNN, SVM, RF, XGBoost) were compared, with XGBoost based on multiple logistic regression showing the best performance (AUC: 0.784, accuracy: 80.4%). Key variables influencing recurrence were identified, and models were visualized via nomograms for clinical use. The findings highlight the potential of machine learning in improving EOC recurrence prediction, though continuous model updates are needed to adapt to evolving clinical contexts.
This study compared surgical and oncological outcomes in patients with incidental borderline ovarian tumors or ovarian cancer treated with a two-stage surgical procedure versus those with suspected malignancy undergoing a single-stage procedure. Among 223 patients, those with incidental diagnoses had more surgeries and longer intervals to chemotherapy and cytoreduction completion. However, there were no significant differences in complete cytoreduction rates, complication rates, hospitalization days, recurrence risk, or survival. Despite delays, incidental diagnosis and two-stage treatment did not negatively impact oncological outcomes, supporting their feasibility in clinical practice.
This study evaluated pathological PCI (pPCI) as a prognostic marker for survival in high-grade serous ovarian cancer patients treated with neoadjuvant chemotherapy and interval cytoreductive surgery. Among 171 patients, ROC analysis identified a pPCI cut-off of 8 as optimal for predicting survival (sensitivity: 82%, specificity: 67%). Patients with pPCI ≥8 had worse overall and disease-free survival (p=0.002 and p=0.001, respectively). Despite complete cytoreduction in 88% of cases, pPCI ≥8 emerged as a poor prognostic indicator, suggesting its utility in guiding adjuvant treatment decisions, particularly in resource-limited settings.
Epithelial ovarian cancer, with a five-year survival rate of 48%, remains a significant threat, especially for advanced FIGO III-IV cases. Despite standard treatments, recurrence and chemoresistance are common. Patient-derived organoids (PDOs), a 3D cancer model, have revolutionized research by replicating disease characteristics and enabling personalized treatment strategies. PDOs predict treatment responses, facilitate drug testing, and uncover drug resistance mechanisms, offering insights into cancer progression. This review highlights PDOs as a transformative tool in ovarian cancer research, bridging foundational theories and clinical applications to advance therapeutic development and individualized care.
Homologous recombination deficiency (HRD), marked by mutations in BRCA1/2 and genomic scars, is a stable biomarker in ovarian cancer (OC). PARP inhibitors (PARPi) exploit HRD via synthetic lethality, showing significant benefits but limited by resistance and unclear long-term survival impact. Current HRD scoring lacks comprehensiveness, especially in HR-proficient cases. Beyond BRCA, understanding HR gene interactions and alternative pathways is crucial for refining PARPi efficacy and developing precise strategies for advanced, recurrent, and refractory OC patients. Further research is needed to optimize HRD’s role in treatment and resistance management.
This study evaluated the diagnostic performance of 3D ultrasonography (3DUS), 3D power Doppler (3DPD), and their combination in ovarian cancer (OC). Analyzing 18 studies (2,548 cases), 3DUS showed sensitivity of 0.89 and specificity of 0.93, while 3DPD had sensitivity of 0.90 and specificity of 0.85. Combining 3DUS and 3DPD achieved superior diagnostic efficiency, with sensitivity of 0.99, specificity of 0.95, and AUC of 0.99. The findings suggest that 3DUS and 3DPD, particularly when combined, are highly effective diagnostic tools for OC, offering improved accuracy and clinical utility.
Although the articles on this topic have provided us with a wealth of insights, there are still many disputes that need to be resolved. For example, how can we better integrate targeted therapy with immunotherapy in the initial treatment? How to further optimize individual treatment strategies through multi-omics analysis? In addition, with the accumulation of real-world data, how to translate these data into decision support tools in clinical practice is also an important direction for future research.
Overall, this topic provides new ideas and evidence for the initial treatment decision of advanced epithelial ovarian cancer through multi-angle discussion. We expect these findings to provide additional references for clinicians and ultimately improve patient outcomes and quality of life.” In the future, with more high-quality research, we believe that the treatment of advanced EOC will have a brighter future.
JG: Conceptualization, Data curation, Writing – original draft, Writing – review & editing.
The author declares that the research was conducted in the absence of any commercial or financial relationships that could be construed as a potential conflict of interest.
The author(s) declare that no Generative AI was used in the creation of this manuscript.
All claims expressed in this article are solely those of the authors and do not necessarily represent those of their affiliated organizations, or those of the publisher, the editors and the reviewers. Any product that may be evaluated in this article, or claim that may be made by its manufacturer, is not guaranteed or endorsed by the publisher.
Keywords: epithelial ovarian cancer (EOC), initial treatment decisions, machine learning in oncology, homologous recombination deficiency (HRD), patient-derived organoids (PDOs), platinum resistance, PARP inhibitors (PARPi), three-dimensional ultrasonography
Citation: Guo J (2025) Editorial: Insights, controversies, and new developments in the initial treatment decisions for advanced epithelial ovarian cancer. Front. Oncol. 15:1568579. doi: 10.3389/fonc.2025.1568579
Received: 30 January 2025; Accepted: 18 February 2025;
Published: 03 March 2025.
Edited and Reviewed by:
Robert Fruscio, University of Milano Bicocca, ItalyCopyright © 2025 Guo. This is an open-access article distributed under the terms of the Creative Commons Attribution License (CC BY). The use, distribution or reproduction in other forums is permitted, provided the original author(s) and the copyright owner(s) are credited and that the original publication in this journal is cited, in accordance with accepted academic practice. No use, distribution or reproduction is permitted which does not comply with these terms.
*Correspondence: Jianfeng Guo, Z3VvamY2MjRAZm94bWFpbC5jb20=
Disclaimer: All claims expressed in this article are solely those of the authors and do not necessarily represent those of their affiliated organizations, or those of the publisher, the editors and the reviewers. Any product that may be evaluated in this article or claim that may be made by its manufacturer is not guaranteed or endorsed by the publisher.
Research integrity at Frontiers
Learn more about the work of our research integrity team to safeguard the quality of each article we publish.