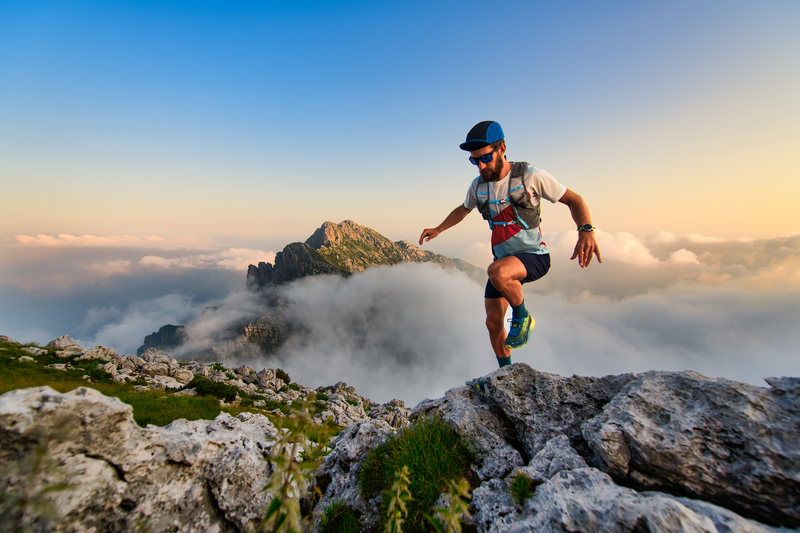
94% of researchers rate our articles as excellent or good
Learn more about the work of our research integrity team to safeguard the quality of each article we publish.
Find out more
ORIGINAL RESEARCH article
Front. Oncol.
Sec. Cancer Immunity and Immunotherapy
Volume 15 - 2025 | doi: 10.3389/fonc.2025.1567238
This article is part of the Research Topic Harnessing Big Data for Precision Medicine: Revolutionizing Diagnosis and Treatment Strategies View all 27 articles
The final, formatted version of the article will be published soon.
You have multiple emails registered with Frontiers:
Please enter your email address:
If you already have an account, please login
You don't have a Frontiers account ? You can register here
Background: Prognostic models for esophageal cancer based on contrast-enhanced chest CT can assist thoracic surgeons in devising personalized treatment plans to optimize patient outcomes. However, the extensive lymphatic drainage and early lymph node metastasis of the esophagus pose significant challenges in extracting and analyzing effective lymph node characteristics. Previous studies have focused on tumor and lymph node features separately, neglecting spatial correlations such as position, direction, and volumetric ratio. Methods: A total of 285 patients undergone radical resection surgery were retrospectively collected at Fujian Provincial Hospital from 2018 to 2022. This study proposed a tumor-lymph node projection plane constructed by projecting lymph node ROI to the tumor ROI plane. A ResNet-CBAM model, combining a residual convolutional neural network with a CBAM attention module, was employed for feature extraction and survival prediction. The PJ-group utilized tumor-lymph node projection planes as training data, while TM-group and ZC-group respectively utilized tumor ROI and concatenated images of tumor and lymph ROI as control. Additional comparisons were conducted with traditional machine learning models (Support Vector Machines, Logistic Regression, and K-Nearest Neighbors). Survival outcomes (median, 批注 [嘉徐1]: Samples were marked in 'abstract' paragraph now.
Keywords: Cross-plane projection, Prognostic prediction, esophageal cancer, ResNet (Residual Network, ResNet), CBAM (Convolutional Block Attention Module, CBAM), Breadth-first search algorithm, deep learning
Received: 26 Jan 2025; Accepted: 26 Feb 2025.
Copyright: © 2025 Xu, Huang, Chen, Wang, Lin, Tang, Shen and Xu. This is an open-access article distributed under the terms of the Creative Commons Attribution License (CC BY). The use, distribution or reproduction in other forums is permitted, provided the original author(s) or licensor are credited and that the original publication in this journal is cited, in accordance with accepted academic practice. No use, distribution or reproduction is permitted which does not comply with these terms.
* Correspondence:
Xunyu Xu, Shengli Clinical Medical College, Fujian Medical University, Fuzhou, China
Disclaimer: All claims expressed in this article are solely those of the authors and do not necessarily represent those of their affiliated organizations, or those of the publisher, the editors and the reviewers. Any product that may be evaluated in this article or claim that may be made by its manufacturer is not guaranteed or endorsed by the publisher.
Research integrity at Frontiers
Learn more about the work of our research integrity team to safeguard the quality of each article we publish.