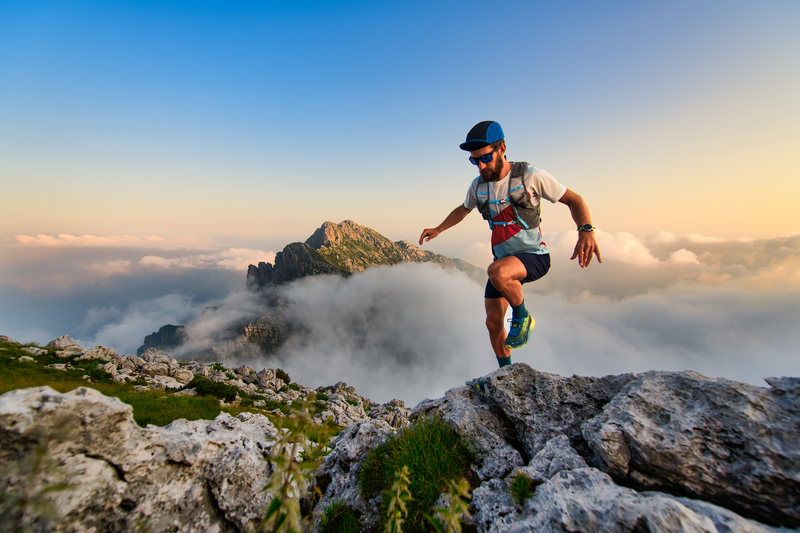
94% of researchers rate our articles as excellent or good
Learn more about the work of our research integrity team to safeguard the quality of each article we publish.
Find out more
ORIGINAL RESEARCH article
Front. Oncol.
Sec. Cancer Immunity and Immunotherapy
Volume 15 - 2025 | doi: 10.3389/fonc.2025.1565090
This article is part of the Research Topic The Role of Metabolic Reprogramming in Tumor Therapy View all 6 articles
The final, formatted version of the article will be published soon.
You have multiple emails registered with Frontiers:
Please enter your email address:
If you already have an account, please login
You don't have a Frontiers account ? You can register here
The metabolism of amino acids and derivatives (MAAD) is closely related to the occurrence and development of colorectal cancer (CRC), but the specific regulatory mechanismsare not yet clear. This study aims to explore the role of MAAD in the progression of colorectal cancer and ultimately identify key molecules that may become potential therapeutic targets for CRC.Methods: This study integrates bulk transcriptome and single-cell transcriptome to analyze and identify key MAAD-related genes from multiple levels. Subsequently, numerous machine learning methods were incorporated to construct MAAD-related prognostic models, and the infiltration of immune cells, tumor heterogeneity, tumor mutation burden, and potential pathway changes under different modes were analyzed. Finally, key molecules were identified for experimental validation.We successfully constructed prognostic models and Nomograms based on key MAADrelated molecules. There was a notable survival benefit observed for low-risk patients when contrasted with their high-risk counterparts. In addition, the high-risk group had a poorer response to immunotherapy and stronger tumor heterogeneity compared with the low-risk group. Further research found that by knocking down the DDAM-related gene SLM8, the malignant characteristics of colorectal cancer cell lines were significantly alleviated, suggesting that SLM8 may become a potential therapeutic target.The MAAD-related gene SLM8 is likely involved in the progression of CRC and could be a hopeful target for therapeutic intervention.
Keywords: Metabolism, bioinformatics, RNAseq, SLM8, colorectal cancer
Received: 22 Jan 2025; Accepted: 28 Feb 2025.
Copyright: © 2025 Yue, Fang, Yang, Feng and Ren. This is an open-access article distributed under the terms of the Creative Commons Attribution License (CC BY). The use, distribution or reproduction in other forums is permitted, provided the original author(s) or licensor are credited and that the original publication in this journal is cited, in accordance with accepted academic practice. No use, distribution or reproduction is permitted which does not comply with these terms.
* Correspondence:
Jian Yue, Chongqing Key Laboratory of Molecular Oncology and Epigenetics, The First Affiliated Hospital of Chongqing Medical University, Chongqing, China
Disclaimer: All claims expressed in this article are solely those of the authors and do not necessarily represent those of their affiliated organizations, or those of the publisher, the editors and the reviewers. Any product that may be evaluated in this article or claim that may be made by its manufacturer is not guaranteed or endorsed by the publisher.
Research integrity at Frontiers
Learn more about the work of our research integrity team to safeguard the quality of each article we publish.