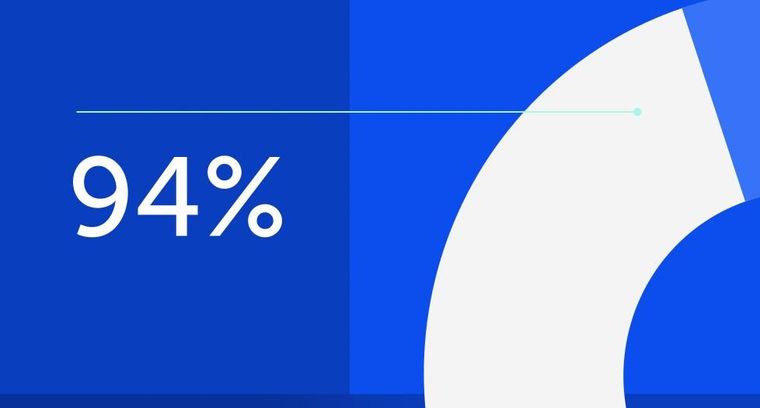
94% of researchers rate our articles as excellent or good
Learn more about the work of our research integrity team to safeguard the quality of each article we publish.
Find out more
ORIGINAL RESEARCH article
Front. Oncol.
Sec. Head and Neck Cancer
Volume 15 - 2025 | doi: 10.3389/fonc.2025.1564459
This article is part of the Research TopicMultidisciplinary Management of Oral Cancer: Diagnosis, Treatment, and RehabilitationView all 7 articles
The final, formatted version of the article will be published soon.
Select one of your emails
You have multiple emails registered with Frontiers:
Notify me on publication
Please enter your email address:
If you already have an account, please login
You don't have a Frontiers account ? You can register here
Background: Postoperative malnutrition, which significantly affects recovery and overall quality of life, is a critical concern for patients with oral cancer. Timely identification of patients at nutritional risk is essential for implementing appropriate interventions, thereby improving postoperative outcomes.Methods: This prospective study, which was conducted at a tertiary hospital in China between August 2023 and May 2024, included 487 postoperative oral cancer patients. The dataset was divided into a training set (70%) and a validation set (30%). Predictive models were developed via four supervised machine learning algorithms: logistic regression (LR), support vector machine (SVM), light gradient boosting machine (LGBM), and extreme gradient boosting (XGBoost). Nutritional risk was assessed via the Nutritional Risk Screening 2002 (NRS-2002) tool and diagnosed via the Global Leadership Initiative on Malnutrition (GLIM) criteria. Model performance was evaluated on the basis of discrimination, calibration, and clinical applicability, with SHAP analysis used for interpretability. Statistical analysis was conducted via R software, with appropriate tests for continuous and categorical variables.Results: Of the 487 oral cancer patients, 251 (51.54%) experienced postoperative malnutrition. The study cohort was split into a training set comprising 340 patients and a validation set comprising 147 patients. Seven key predictors were identified, including sex, T stage, repair and reconstruction, diabetes status, age, lymphocyte count, and total cholesterol (TC) level. The XGBoost model demonstrated an area under the curve (AUC) of 0.872 (95% CI: 0.836–0.909) in the training set and 0.840 (95% CI: 0.777–0.904) in the validation set. Calibration curves confirmed the model’s robust fit, and decision curve analysis (DCA) indicated substantial clinical benefit.Conclusion: This study represents the first development of an XGBoost-based model for predicting postoperative malnutrition in patients with oral cancer. The integration of SHAP for model interpretability, along with the creation of an intuitive web tool, enhances the model’s clinical applicability. This approach can significantly reduce malnutrition-related complications and improve recovery outcomes for oral cancer patients.
Keywords: Malnutrition, Oral neoplasms, machine learning, Shapley Additive Explanations (SHAPs), Postoperative Complications
Received: 21 Jan 2025; Accepted: 14 Apr 2025.
Copyright: © 2025 Kuang, Yu, Zhou, Zhang, Wang, Zhang, Lubamba and Bi. This is an open-access article distributed under the terms of the Creative Commons Attribution License (CC BY). The use, distribution or reproduction in other forums is permitted, provided the original author(s) or licensor are credited and that the original publication in this journal is cited, in accordance with accepted academic practice. No use, distribution or reproduction is permitted which does not comply with these terms.
* Correspondence: Xiaoqin Bi, Sichuan University, Chengdu, China
Disclaimer: All claims expressed in this article are solely those of the authors and do not necessarily represent those of their affiliated organizations, or those of the publisher, the editors and the reviewers. Any product that may be evaluated in this article or claim that may be made by its manufacturer is not guaranteed or endorsed by the publisher.
Supplementary Material
Research integrity at Frontiers
Learn more about the work of our research integrity team to safeguard the quality of each article we publish.