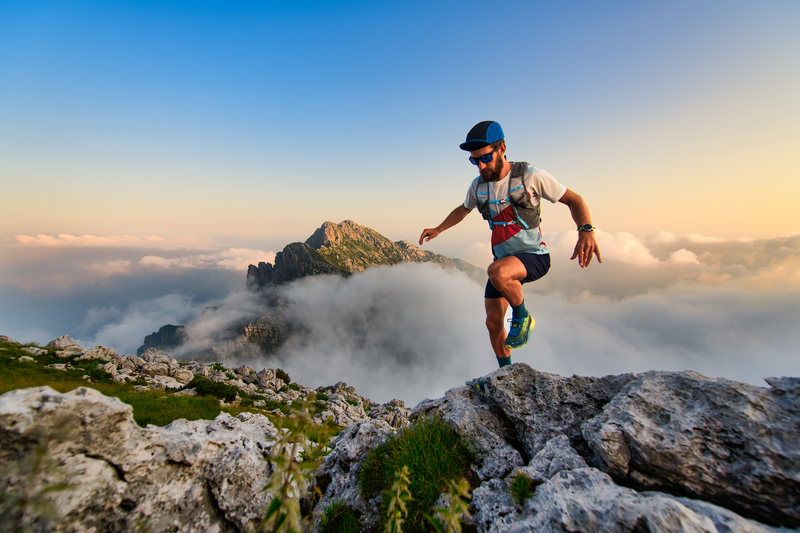
94% of researchers rate our articles as excellent or good
Learn more about the work of our research integrity team to safeguard the quality of each article we publish.
Find out more
ORIGINAL RESEARCH article
Front. Oncol.
Sec. Cancer Metabolism
Volume 15 - 2025 | doi: 10.3389/fonc.2025.1557638
The final, formatted version of the article will be published soon.
You have multiple emails registered with Frontiers:
Please enter your email address:
If you already have an account, please login
You don't have a Frontiers account ? You can register here
The Krebs cycle (KC) is an important pathway for cancer cells because it produces reduced coenzymes for ATP synthesis and precursors for cellular proliferation. Described changes in cancer KC enzyme activities suggested modifications in the reactions that control the KC flux compared to normal cells. In this work, kinetic metabolic models of KC of mitochondria from cancer (HepM), liver (RLM) and heart (RHM) to identify targets to decrease the KC flux were constructed from kinetic parameters (Vmax and Km) of enzymes here determined. The enzymes Vmax values were higher in the following order: RHM > HepM > RLM; meanwhile, Km values were similar. Kinetic modeling indicated that the NADH consumption reaction (complex I) exerted higher control on the Krebs cycle flux in HepM versus RLM and to a lesser extent in RHM. These results suggested that cancer cells may be more sensitive to complex I inhibition than heart and other non-cancer cells. Indeed, cancer cell proliferation was more sensitive to rotenone (a complex I inhibitor) than heart and non-cancer cells. In contrast, cell proliferation had similar sensitivities to malonate, an inhibitor of succinate dehydrogenase, an enzyme that does not exert control. Our results showed that kinetic modeling and metabolic control analysis allow the identification of high flux-controlling targets in cancer cells that help to design strategies to specifically inhibit their proliferation. This can minimize the toxic effects in normal cells, such as the cardiac ones that are highly sensitive to conventional chemotherapy.
Keywords: Cancer, Krebs cycle, Mitochondria, Complex I, Heart, Liver, kinetic modeling, Metabolic Control Analysis
Received: 08 Jan 2025; Accepted: 04 Mar 2025.
Copyright: © 2025 Hernández-Esquivel, Del Mazo-Monsalvo, Pacheco Velázquez, Feregrino-Mondragón, Robledo-Cadena, Sánchez-Thomas, Jasso-Chávez, Saavedra and Marín Hernández. This is an open-access article distributed under the terms of the Creative Commons Attribution License (CC BY). The use, distribution or reproduction in other forums is permitted, provided the original author(s) or licensor are credited and that the original publication in this journal is cited, in accordance with accepted academic practice. No use, distribution or reproduction is permitted which does not comply with these terms.
* Correspondence:
Alvaro Marín Hernández, Departamento de Bioquímica, Instituto Nacional de Cardiología, Ciudad de México, Mexico
Disclaimer: All claims expressed in this article are solely those of the authors and do not necessarily represent those of their affiliated organizations, or those of the publisher, the editors and the reviewers. Any product that may be evaluated in this article or claim that may be made by its manufacturer is not guaranteed or endorsed by the publisher.
Research integrity at Frontiers
Learn more about the work of our research integrity team to safeguard the quality of each article we publish.