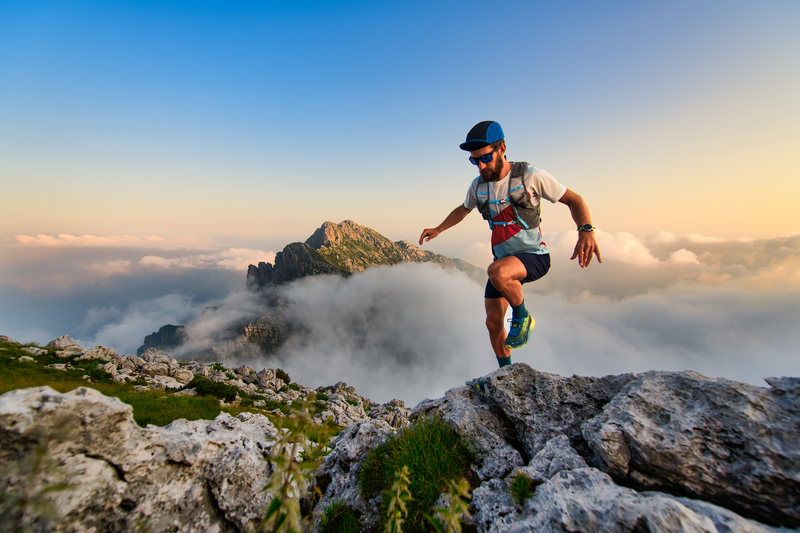
94% of researchers rate our articles as excellent or good
Learn more about the work of our research integrity team to safeguard the quality of each article we publish.
Find out more
ORIGINAL RESEARCH article
Front. Oncol.
Sec. Head and Neck Cancer
Volume 15 - 2025 | doi: 10.3389/fonc.2025.1557459
The final, formatted version of the article will be published soon.
You have multiple emails registered with Frontiers:
Please enter your email address:
If you already have an account, please login
You don't have a Frontiers account ? You can register here
Purpose: Oral squamous cell carcinoma (OSCC) often presents with unsatisfactory survival outcomes, especially in advanced stages. This study aimed to develop and validate a nomogram incorporating demographic, clinicopathologic, and treatment-related factors to improve prediction of overall survival (OS) in OSCC patients. Methods: We retrospectively utilized data from 15,204 OSCC patients in a United States database to construct a prognostic model and generate a nomogram. External validation was performed using an independent cohort of 359 patients from a specialized cancer center in China. Prognostic factors were identified using Cox regression analysis and incorporated into the nomogram. Model performance was evaluated by concordance indices (C-index), time-dependent area under the receiver operating characteristic curves (AUC), calibration plots, and decision curve analysis (DCA). A risk stratification system was developed to classify patients into high-and low-risk groups. Results: Age, sex, primary tumor site, T and N staging, and treatment modalities (including surgery, chemotherapy, and radiotherapy) were found to be independent prognostic factors. The nomogram achieved a C-index of 0.727 in the training set and 0.6845 in the validation set, outperforming the conventional TNM staging system. The nomogram's superior predictive accuracy was confirmed by higher AUC values, better calibration, and improved clinical utility as demonstrated by DCA. Risk stratification, based on the nomogram, distinguished patients into distinct prognostic groups with significant OS differences. Conclusions: This nomogram provides an effective, personalized tool for predicting OS in OSCC,. It offers clinicians a valuable aid for treatment decision-making and improves patient management.
Keywords: oral squamous cell carcinoma, oral cancer, overall survival, nomogram, prognosis
Received: 08 Jan 2025; Accepted: 21 Feb 2025.
Copyright: © 2025 Xu, Xu, Sun, Feng, Xu and Peng. This is an open-access article distributed under the terms of the Creative Commons Attribution License (CC BY). The use, distribution or reproduction in other forums is permitted, provided the original author(s) or licensor are credited and that the original publication in this journal is cited, in accordance with accepted academic practice. No use, distribution or reproduction is permitted which does not comply with these terms.
* Correspondence:
Hanwei Peng, Department of Head and Neck Surgery, Cancer Hospital, College of Medicine, Shantou University, Shantou, China
Disclaimer: All claims expressed in this article are solely those of the authors and do not necessarily represent those of their affiliated organizations, or those of the publisher, the editors and the reviewers. Any product that may be evaluated in this article or claim that may be made by its manufacturer is not guaranteed or endorsed by the publisher.
Research integrity at Frontiers
Learn more about the work of our research integrity team to safeguard the quality of each article we publish.