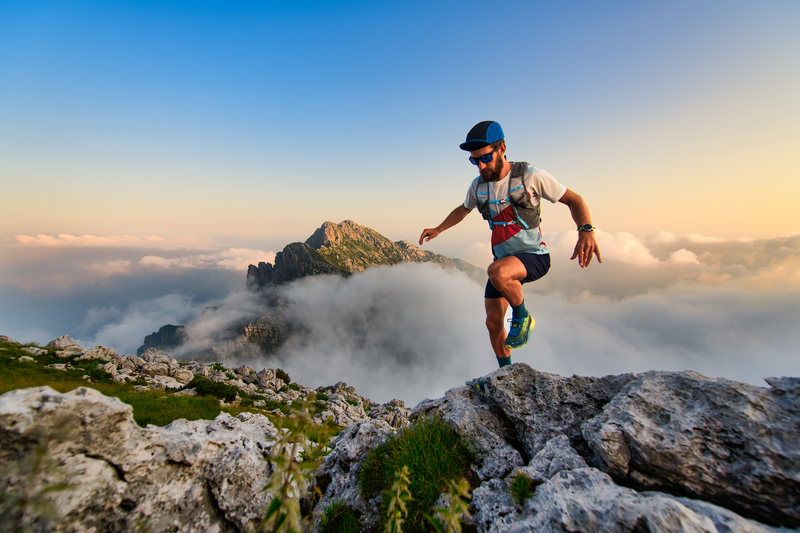
95% of researchers rate our articles as excellent or good
Learn more about the work of our research integrity team to safeguard the quality of each article we publish.
Find out more
REVIEW article
Front. Oncol.
Sec. Cancer Epidemiology and Prevention
Volume 15 - 2025 | doi: 10.3389/fonc.2025.1555247
This article is part of the Research Topic Harnessing Explainable AI for Precision Cancer Diagnosis and Prognosis View all 3 articles
The final, formatted version of the article will be published soon.
You have multiple emails registered with Frontiers:
Please enter your email address:
If you already have an account, please login
You don't have a Frontiers account ? You can register here
This study aimed to evaluate the quality and transparency of reporting in studies using machine learning (ML) for cancer diagnosis, focusing on adherence to the Consolidated Reporting Guidelines for Prognostic and Diagnostic Machine Learning Models (CREMLS), TRIPOD-AI (Transparent Reporting of a Multivariable Prediction Model for Individual Prognosis or Diagnosis), and PROBAST (Prediction Model Risk of Bias Assessment Tool). The literature search included primary studies published between February 1, 2024, and January 31, 2025, that developed or tested ML models for cancer diagnosis, treatment, or prognosis. To reflect the current state of the rapidly evolving landscape of ML applications in oncology, fifteen most recent articles in each category were selected for evaluation. Two independent reviewers screened studies and extracted data on study characteristics, reporting quality (CREMLS and TRIPOD+AI), risk of bias (PROBAST), and ML performance metrics. The most frequently studied cancer types were breast cancer (n=7/45; 15.6%), lung cancer (n=7/45; 15.6%), and liver cancer (n=5/45; 11.1%). The findings indicate several deficiencies in reporting quality, as assessed by CREMLS and TRIPOD+AI. These deficiencies primarily relate to sample size calculation, reporting on data quality, strategies for handling outliers, documentation of ML model predictors, access to training or validation data, and reporting on model performance heterogeneity. The methodological quality assessment using PROBAST revealed that 89% of the included studies exhibited a low overall risk of bias, and all studies have shown a low risk of bias in terms of applicability. Regarding the specific AI models identified as the best-performing, Random Forest (RF) and XGBoost were the most frequently reported, each used in 17.8% of the studies (n = 8). Additionally, our study outlines the specific areas where reporting is deficient, providing researchers with guidance to improve reporting quality in these sections and, consequently, reduce the risk of bias in their studies.
Keywords: Cancer, artificial intelligence, diagnosis Normal, Justified, prognosis
Received: 03 Jan 2025; Accepted: 18 Mar 2025.
Copyright: © 2025 Smiley, Villarreal-Zegarra, Reategui-Rivera, Escobar-Agreda and Finkelstein. This is an open-access article distributed under the terms of the Creative Commons Attribution License (CC BY). The use, distribution or reproduction in other forums is permitted, provided the original author(s) or licensor are credited and that the original publication in this journal is cited, in accordance with accepted academic practice. No use, distribution or reproduction is permitted which does not comply with these terms.
* Correspondence:
Aref Smiley, School of Medicine, The University of Utah, Salt Lake City, United States
Disclaimer: All claims expressed in this article are solely those of the authors and do not necessarily represent those of their affiliated organizations, or those of the publisher, the editors and the reviewers. Any product that may be evaluated in this article or claim that may be made by its manufacturer is not guaranteed or endorsed by the publisher.
Research integrity at Frontiers
Learn more about the work of our research integrity team to safeguard the quality of each article we publish.