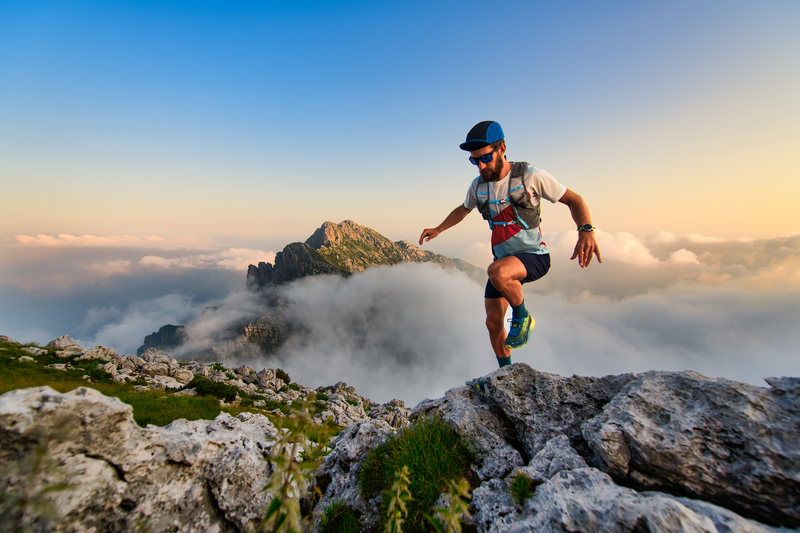
94% of researchers rate our articles as excellent or good
Learn more about the work of our research integrity team to safeguard the quality of each article we publish.
Find out more
ORIGINAL RESEARCH article
Front. Oncol.
Sec. Breast Cancer
Volume 15 - 2025 | doi: 10.3389/fonc.2025.1552802
The final, formatted version of the article will be published soon.
You have multiple emails registered with Frontiers:
Please enter your email address:
If you already have an account, please login
You don't have a Frontiers account ? You can register here
Background: Pathological complete response (pCR) is an important indicator for evaluating the efficacy of neoadjuvant chemotherapy (NAC) in breast cancer. The role of systemic inflammation markers in predicting pCR and the long-term prognosis of breast cancer patients undergoing NAC remains controversial. The purpose of this study was to explore the potential predictive and prognostic value of systemic inflammation markers (NLR, PLR, LMR, NMR) and clinicopathological characteristics in breast cancer patients receiving NAC and construct a pCR prediction model based on these indicators. Methods: A retrospective analysis was conducted on 209 breast cancer patients who
Keywords: breast cancer, systemic inflammation markers, PCR, prognosis, predictive model
Received: 29 Dec 2024; Accepted: 10 Mar 2025.
Copyright: © 2025 Sun, Guan, Yu, Zhang, Tao, Zhang and Yao. This is an open-access article distributed under the terms of the Creative Commons Attribution License (CC BY). The use, distribution or reproduction in other forums is permitted, provided the original author(s) or licensor are credited and that the original publication in this journal is cited, in accordance with accepted academic practice. No use, distribution or reproduction is permitted which does not comply with these terms.
* Correspondence:
Yongzhong Yao, Nanjing Drum Tower Hospital, Nanjing, China
Disclaimer: All claims expressed in this article are solely those of the authors and do not necessarily represent those of their affiliated organizations, or those of the publisher, the editors and the reviewers. Any product that may be evaluated in this article or claim that may be made by its manufacturer is not guaranteed or endorsed by the publisher.
Research integrity at Frontiers
Learn more about the work of our research integrity team to safeguard the quality of each article we publish.