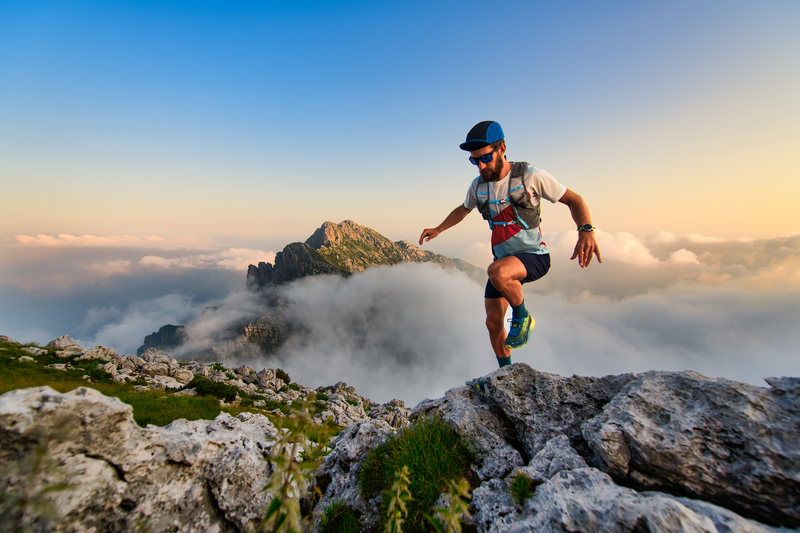
94% of researchers rate our articles as excellent or good
Learn more about the work of our research integrity team to safeguard the quality of each article we publish.
Find out more
ORIGINAL RESEARCH article
Front. Oncol.
Sec. Head and Neck Cancer
Volume 15 - 2025 | doi: 10.3389/fonc.2025.1551247
The final, formatted version of the article will be published soon.
You have multiple emails registered with Frontiers:
Please enter your email address:
If you already have an account, please login
You don't have a Frontiers account ? You can register here
Accurately differentiating benign and malignant lesions is essential for treatment. We aimed to determine differences in urine proteomics between papillary thyroid carcinomas (PTCs) and benign thyroid nodules (BTNs) and identify biomarkers for the differential diagnosis of these diseases. We collected 155 specimens. In the discovery group, 30 PTC and 31 BTN specimens were quantitatively compared using liquid chromatography-tandem mass spectrometry (MS). The diagnostic value of each significantly altered protein was calculated in the MS validation comprising 11 PTC and 10 BTN samples. Ultimately, 36 BTN and 37 PTC specimens were used for ELISA validation. Overall, 2,479 proteins were used for quantitative analysis. Compared with benign nodules, papillary carcinomas showed significant increases and decreases in the levels of 169 and 27 proteins, respectively. Neck and thyroid tumors were enriched in the disease or function category. More than 100 proteins showed good performance in the area under the receiver operating characteristic curve (>0.8) upon MS validation. Semaphorin-6D showed good performance (AUC = 0.763) in ELISA validation. Urine proteomics is an effective diagnostic tool for distinguishing benign and malignant thyroid diseases. Semaphorin-6D may serve as a disease marker for large-scale validation and use.Additionally, this study identified potential biomarkers that warrant further investigation.
Keywords: Urine proteomics, thyroid papillary carcinoma, Benign thyroid nodules, Urinary disease markers, Urine
Received: 25 Dec 2024; Accepted: 20 Mar 2025.
Copyright: © 2025 Wei, Xiao, Guo, Wang, Ke-Xin, Zhou, Sun and Yong-Tong. This is an open-access article distributed under the terms of the Creative Commons Attribution License (CC BY). The use, distribution or reproduction in other forums is permitted, provided the original author(s) or licensor are credited and that the original publication in this journal is cited, in accordance with accepted academic practice. No use, distribution or reproduction is permitted which does not comply with these terms.
* Correspondence:
Yun Zhou, China-Japan Friendship Hospital, Beijing, China
Wei Sun, Chinese Academy of Medical Sciences and Peking Union Medical College, Dongcheng, 100006, Beijing Municipality, China
Cao Yong-Tong, China-Japan Friendship Hospital, Beijing, China
Disclaimer: All claims expressed in this article are solely those of the authors and do not necessarily represent those of their affiliated organizations, or those of the publisher, the editors and the reviewers. Any product that may be evaluated in this article or claim that may be made by its manufacturer is not guaranteed or endorsed by the publisher.
Research integrity at Frontiers
Learn more about the work of our research integrity team to safeguard the quality of each article we publish.