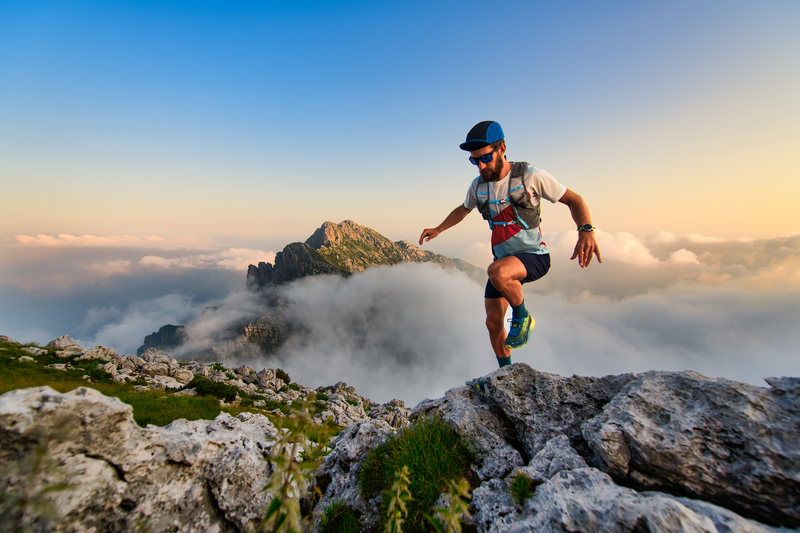
94% of researchers rate our articles as excellent or good
Learn more about the work of our research integrity team to safeguard the quality of each article we publish.
Find out more
ORIGINAL RESEARCH article
Front. Oncol.
Sec. Pharmacology of Anti-Cancer Drugs
Volume 15 - 2025 | doi: 10.3389/fonc.2025.1549851
This article is part of the Research Topic Decoding Tumor Drug Resistance: Machine Learning’s Role from Molecules to Treatment View all 4 articles
The final, formatted version of the article will be published soon.
You have multiple emails registered with Frontiers:
Please enter your email address:
If you already have an account, please login
You don't have a Frontiers account ? You can register here
Bone metastasis is a serious complication following radical prostatectomy in prostate cancer patients, significantly affecting their long-term survival. This study aims to develop a clinical predictive model utilizing Magnetic Resonance Imaging (MRI) and advanced machine learning algorithms to identify key factors that increase the risk of bone metastasis (BM).The study analyzed a cohort of 1161 prostate cancer patients, including 38 who developed bone metastasis. Preoperative T2-weighted images (T2WI) were obtained, and tumor lesions were manually delineated to extract relevant features from the imaging data. Spearman correlation analysis, the least absolute shrinkage and selection operator (LASSO) algorithm, and logistic regression were used to select and construct the model. Four machine learning algorithms-extreme gradient boosting (XGBoost), random forest (RF), support vector machine (SVM), and k-nearest neighbor (KNN) -were employed to predict BM occurrence, integrating these with clinical information.Among the four prognostic models evaluated, the XGBoost algorithm performed the best. In the training dataset, the XGBoost model achieved an AUC of 0.926 (0.870-0.982), an accuracy of 0.847 (0.773-0.921), a sensitivity of 0.880 (0.835-0.926), and a specificity of 0.829 (0.755-0.904). In the validation dataset, the XGBoost model attained an AUC of 0.706 (0.586-0.826), an accuracy of 0.687 (0.661-0.713), a sensitivity of 0.693 (0.557-0.829), and a specificity of 0.664 (0.505-0.822). The external validation dataset yielded an AUC of 0.91, demonstrating the robust predictive capabilities of the XGBoost model.The predictive model for bone metastasis in prostate cancer, developed using the XGBoost machine learning algorithm, shows high accuracy and significant clinical relevance. This model provides a valuable tool for identifying high-risk patients, potentially informing better management and treatment strategies.
Keywords: prostate cancer, bone metastasis, prognosis, risk factor, machine learning
Received: 22 Dec 2024; Accepted: 28 Jan 2025.
Copyright: © 2025 Yang, Wei, Zhou and Mao. This is an open-access article distributed under the terms of the Creative Commons Attribution License (CC BY). The use, distribution or reproduction in other forums is permitted, provided the original author(s) or licensor are credited and that the original publication in this journal is cited, in accordance with accepted academic practice. No use, distribution or reproduction is permitted which does not comply with these terms.
* Correspondence:
Fei Mao, The Affiliated Huaian No. 1 People’s Hospital of Nanjing Medical University, Huai'an, China
Disclaimer: All claims expressed in this article are solely those of the authors and do not necessarily represent those of their affiliated organizations, or those of the publisher, the editors and the reviewers. Any product that may be evaluated in this article or claim that may be made by its manufacturer is not guaranteed or endorsed by the publisher.
Research integrity at Frontiers
Learn more about the work of our research integrity team to safeguard the quality of each article we publish.