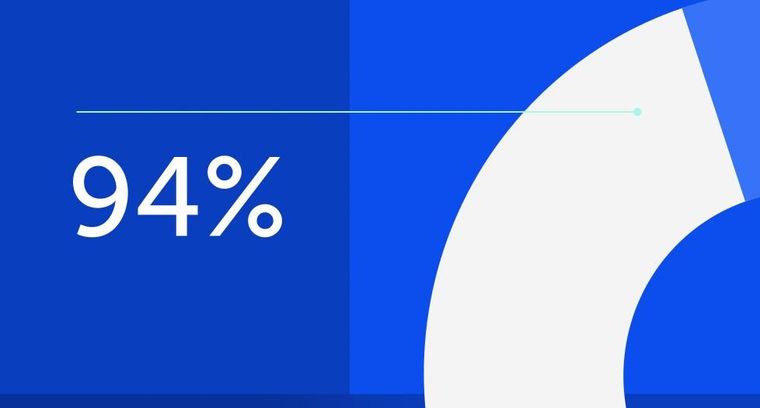
94% of researchers rate our articles as excellent or good
Learn more about the work of our research integrity team to safeguard the quality of each article we publish.
Find out more
ORIGINAL RESEARCH article
Front. Oncol.
Sec. Cancer Imaging and Image-directed Interventions
Volume 15 - 2025 | doi: 10.3389/fonc.2025.1543230
This article is part of the Research TopicRecent Trends and Advancements in Multispectral and Hyperspectral Imaging for Cancer DetectionView all articles
The final, formatted version of the article will be published soon.
Select one of your emails
You have multiple emails registered with Frontiers:
Notify me on publication
Please enter your email address:
If you already have an account, please login
You don't have a Frontiers account ? You can register here
Objective: Early detection and timely surgical intervention are crucial in reducing mortality rates associated with clinically significant prostate cancer (csPCa). Currently, clinical diagnostics primarily depend on magnetic resonance imaging (MRI) and nuclear medicine, with the potential diagnostic value of abdominal computed tomography (CT) remaining underexplored. This study aims to evaluate the effectiveness of multi-task deep learning neural networks in identifying early-stage prostate cancer using CT scans.: In this study, we enrolled 539 patients from the Department of Radiology (N=461) and Nuclear Medicine (N=78). We utilized a multi-task deep learning network model (MTDL), based on the 3DUnet architecture, to segment and analyze the collected abdominal plain CT images. The predictive performance of this model was compared with a radiomics model and a single-task deep learning model using ResNet18. A diagnostic nomogram was then developed using the multi-task deep learning approach, incorporating prediction results and PSAD, age. The diagnostic performance of the different models was evaluated using the receiver operating characteristic (ROC) curve and the area under the curve (AUC). Results: The 461 patients from the Department of Radiology were divided into training and test sets at a ratio of 6:4, while the patients from the Department of Nuclear Medicine formed the validation set. Our MTDL nomogram demonstrated AUCs of 0.941 (95% confidence interval [CI]: 0.905-0.970), 0.912 (95% CI: 0.904 -0.969), and 0.932 (95% CI: 0.883 -0.970) in the training, test, and validation cohorts, respectively. This study indicates that combining abdominal CT with a multi-task neural network model effectively diagnoses csPCa, offering superior diagnostic performance compared to clinical models. Additionally, the multi-task neural network model outperformed both the single-task neural network model and the radiomics model in diagnostic accuracy.Our study demonstrated that the MTDL nomogram can accurately predict the presence of prostate cancer using abdominal CT scans, offering significant value for the early diagnosis of prostate cancer.
Keywords: prostate cancer, Multi-task deep learning, machine learning, neural networks, computed tomography
Received: 11 Dec 2024; Accepted: 15 Apr 2025.
Copyright: © 2025 Geng, Zhang, Zhang, Li, Tian and Ma. This is an open-access article distributed under the terms of the Creative Commons Attribution License (CC BY). The use, distribution or reproduction in other forums is permitted, provided the original author(s) or licensor are credited and that the original publication in this journal is cited, in accordance with accepted academic practice. No use, distribution or reproduction is permitted which does not comply with these terms.
* Correspondence: Xiaofen Ma, the Affiliated Guangdong Second Provincial General Hospital of Jinan University, Guangzhou, China
Disclaimer: All claims expressed in this article are solely those of the authors and do not necessarily represent those of their affiliated organizations, or those of the publisher, the editors and the reviewers. Any product that may be evaluated in this article or claim that may be made by its manufacturer is not guaranteed or endorsed by the publisher.
Supplementary Material
Research integrity at Frontiers
Learn more about the work of our research integrity team to safeguard the quality of each article we publish.