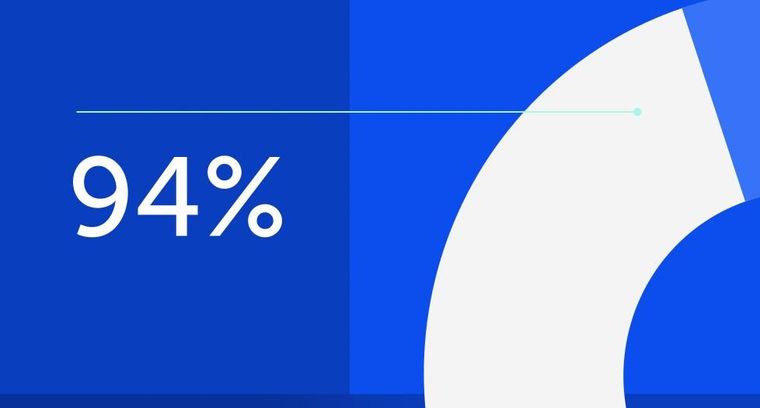
94% of researchers rate our articles as excellent or good
Learn more about the work of our research integrity team to safeguard the quality of each article we publish.
Find out more
ORIGINAL RESEARCH article
Front. Oncol.
Sec. Cancer Imaging and Image-directed Interventions
Volume 15 - 2025 | doi: 10.3389/fonc.2025.1543020
The final, formatted version of the article will be published soon.
Select one of your emails
You have multiple emails registered with Frontiers:
Notify me on publication
Please enter your email address:
If you already have an account, please login
You don't have a Frontiers account ? You can register here
Objective: To establish a combined model based on ultrasound radiomics combined with multimodal ultrasound and evaluate its value in diagnosing benign and malignant nodules classified as Chinese-Thyroid Imaging Report and Data System (C-TIRADS) 4A. Methods: Prospective collection of data from 446 patients with thyroid nodules classified as C-TIRADS 4A between December 2023 and August 2024. Based on the enrollment timeline, patients were divided into a training set (n=312) and a test set (n=134) in a 7:3 ratio. Using clinical information, multimodal ultrasound features, and radiomics features, a radiomics model was constructed using the Random Forest (RF) machine learning algorithm. Logistic regression was employed to develop the multimodal ultrasound model and the combined model. The predictive efficiency and accuracy of these models were evaluated using Receiver Operating Characteristic (ROC) curves, calibration curves, and Decision Curve Analysis (DCA). The diagnostic efficacy of junior physicians assisted by the ultrasound radiomics model was compared with that of senior physicians. DeLong's test was performed to compare the diagnostic performance of the models. Results: Multivariate analysis revealed that age (≤51 years), Sound Touch Elastography mean stiffness (STE Mean), orientation (vertical), margin (blurred), and margin (irregular) were independent risk factors for papillary thyroid carcinoma, and the multimodal ultrasound model was established. Based on 17 ultrasound radiomics features, a radiomics model was constructed using the RF machine learning algorithm. The combined model was developed by combining the two aforementioned models. In the training set, the areas under the curve (AUC) of the multimodal ultrasound model, ultrasound radiomics model, and combined model were 0.852, 0.940 and 0.956, respectively. In the test set, the AUC were 0.804, 0.832 and 0.863, respectively. DeLong's test showed that the combined model performed best in the training set, and in the test set, the combined model outperformed the multimodal ultrasound model but showed no significant difference compared to the radiomics model. DCA indicated that the combined model achieved higher net benefits within a specific threshold probability range (0.15-0.90).The combined model exhibits robust diagnostic capability in distinguishing benign from malignant thyroid nodules classified as C-TIRADS 4A.
Keywords: C-TIRADS 4A, Multimodal ultrasound, ultrasound radiomics, Papillary thyroid cancer, Benign or malignant
Received: 22 Jan 2025; Accepted: 14 Apr 2025.
Copyright: © 2025 Cui, Liu, Wang, Li, Li, Li, Bi, Mu, Guo, Yao and Zhang. This is an open-access article distributed under the terms of the Creative Commons Attribution License (CC BY). The use, distribution or reproduction in other forums is permitted, provided the original author(s) or licensor are credited and that the original publication in this journal is cited, in accordance with accepted academic practice. No use, distribution or reproduction is permitted which does not comply with these terms.
* Correspondence: Jundong Yao, The First Affiliated Hospital of Henan University of Science and Technology, Luoyang, Henan Province, China
Disclaimer: All claims expressed in this article are solely those of the authors and do not necessarily represent those of their affiliated organizations, or those of the publisher, the editors and the reviewers. Any product that may be evaluated in this article or claim that may be made by its manufacturer is not guaranteed or endorsed by the publisher.
Supplementary Material
Research integrity at Frontiers
Learn more about the work of our research integrity team to safeguard the quality of each article we publish.