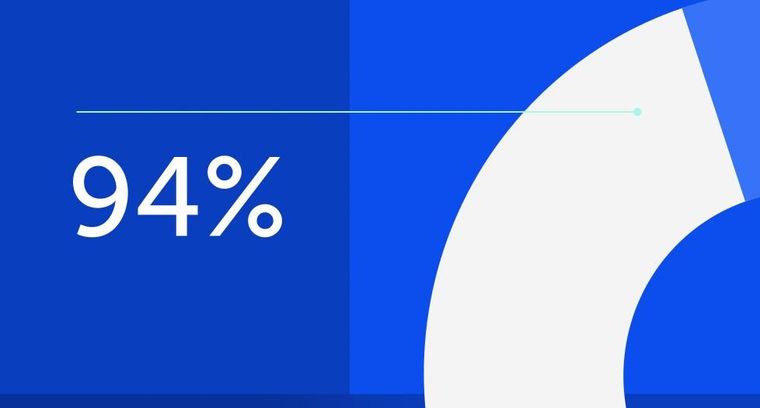
94% of researchers rate our articles as excellent or good
Learn more about the work of our research integrity team to safeguard the quality of each article we publish.
Find out more
ORIGINAL RESEARCH article
Front. Oncol.
Sec. Breast Cancer
Volume 15 - 2025 | doi: 10.3389/fonc.2025.1542643
The final, formatted version of the article will be published soon.
Select one of your emails
You have multiple emails registered with Frontiers:
Notify me on publication
Please enter your email address:
If you already have an account, please login
You don't have a Frontiers account ? You can register here
Purpose: To develop a radiomics model based on ultrasound images for predicting risk of recurrence in breast cancer patients. Methods: In this retrospective study, 420 patients with pathologically confirmed breast cancer were included, randomly divided into training (70%) and test (30%) sets, with an independent external validation cohort of 90 patients. According to St. Gallen recurrence risk criteria, patients were categorized into two groups, low-medium-risk and high-risk. Radiomics features were extracted from a radiomics analysis set using Pyradiomics. The informative radiomics features were screened using the minimum redundancy maximum relevance (mRMR) and the least absolute shrinkage and selection operator (LASSO) algorithms. Subsequently, radiomics models were constructed with eight machine learning algorithms. Three distinct nomogram models were created using the features selected through multivariate logistic regression, including the Clinic-Ultrasound (Clin-US), Clinic-Radiomics (Clin-Rad), and Clinic-Ultrasound-Radiomics (Clin-US-Rad) models. The receiver operating characteristic (ROC), calibration, and decision curve analysis (DCA) curves were used to evaluate the model’s clinical applicability and predictive performance. Results: A total of 12 ultrasound radiomics features were screened, of which wavelet.LHL first order Mean features weighed more and tended to have a high risk of recurrence. The higher the risk of recurrence, the higher the radiomics score (Rad-score) in all three sets (training, test, and external validation set, all p < 0.05). Rad-score is equally applicable in four different subtypes of breast cancer. In the test set and external validation set, the Clin-US-Rad model achieved the highest AUC values (AUC = 0.817 and 0.851, respectively). The calibration and DCA curves also demonstrated the good clinical utility of the combined model.Conclusion: The machine learning-based ultrasound radiomics model were useful for predicting the risk of recurrence in breast cancer. The nomograms show promising potential in assessing the recurrence risk of breast cancer. This non-invasive approach offers crucial guidance for the diagnosis and treatment of the condition. Keywords: Radiomics; Breast Cancer; Recurrence Risk; Ultrasound; Nomogram
Keywords: Radiomics, breast cancer, recurrence risk, ultrasound, nomogram
Received: 10 Dec 2024; Accepted: 17 Apr 2025.
Copyright: © 2025 Fan, Cui, Liu, Zhang, Fang, Wang, Qin, Yang, Tian and Zhang. This is an open-access article distributed under the terms of the Creative Commons Attribution License (CC BY). The use, distribution or reproduction in other forums is permitted, provided the original author(s) or licensor are credited and that the original publication in this journal is cited, in accordance with accepted academic practice. No use, distribution or reproduction is permitted which does not comply with these terms.
* Correspondence:
Xiuhua Yang, First Affiliated Hospital of Harbin Medical University, Harbin, Heilongjiang Province, China
Jiawei Tian, The Second Affiliated Hospital of Harbin Medical University, Harbin, China
Lei Zhang, First Affiliated Hospital of Harbin Medical University, Harbin, Heilongjiang Province, China
Disclaimer: All claims expressed in this article are solely those of the authors and do not necessarily represent those of their affiliated organizations, or those of the publisher, the editors and the reviewers. Any product that may be evaluated in this article or claim that may be made by its manufacturer is not guaranteed or endorsed by the publisher.
Supplementary Material
Research integrity at Frontiers
Learn more about the work of our research integrity team to safeguard the quality of each article we publish.