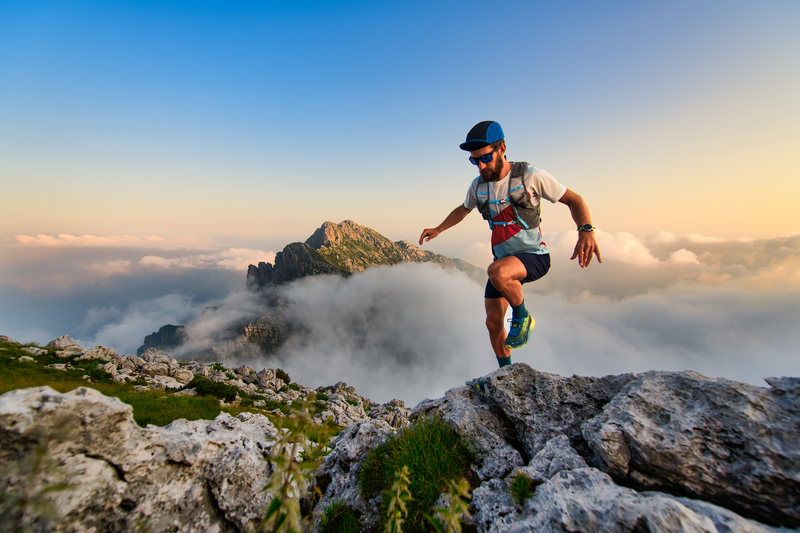
95% of researchers rate our articles as excellent or good
Learn more about the work of our research integrity team to safeguard the quality of each article we publish.
Find out more
ORIGINAL RESEARCH article
Front. Oncol.
Sec. Genitourinary Oncology
Volume 15 - 2025 | doi: 10.3389/fonc.2025.1541618
This article is part of the Research Topic Enhancing Prostate Cancer Diagnosis: Biomarkers and Imaging for Improved Patient Outcomes View all 8 articles
The final, formatted version of the article will be published soon.
You have multiple emails registered with Frontiers:
Please enter your email address:
If you already have an account, please login
You don't have a Frontiers account ? You can register here
Objective: To develop and externally validate multiparametric MRI (mpMRI) radiomics-based interpretable machine learning (ML) model for preoperative differentiating between benign and malignant prostate masses.Patients who underwent mpMRI with suspected malignant prostate masses were retrospectively recruited from two independent hospitals between May 2016 and May 2023. The prostate mass regions in T2WI and DWI MRI images were segmented by the ITK-SNAP. The 'PyRadiomics' was utilized to extracted radiomics features. The inter-and intra-observer correlation analysis, T test, Spearman correlation analysis, and the least absolute shrinkage and selection operator (LASSO) algorithm with a five-fold cross-validation were applied for feature selection. Five ML learning models were built using the chosen features. Model performance was evaluated with internal and external validation, using area under the curve (AUC), Calibration curves, and Decision curve analysis to select the optimal model. The interpretability of the most robust model was conducted via the SHAP.: A total of 567 patients were enrolled, consisting of the training (n=352), internal test (n=152), and external test (n=63) sets. 2632 radiomics features were extracted from ROIs of T2WI and DWI images, which were reduced to 18 via the LASSO. Five ML models were established, among which the RF model presented the best predictive ability, with AUCs of 0.929 (95% confidential interval [CI]: 0.885-0.963) and 0.852 (95 % CI: 0.758-0.934) in the internal and external test sets, respectively. The Calibration and Decision curve analysis confirmed the excellent clinical usefulness of the RF model. Besides, the contributing relations of the radiomics features were uncovered using the SHAP. Conclusions: Radiomics features from mpMRI combined with machine learning facilitates accurate preoperative evaluation of the malignancy in prostate masses. The SHAP can disclosed the underlying prediction process of the ML model, which may promote its' clinical applications.
Keywords: Malignant prostate mass, Multiparametric magnetic resonance imaging, Radiomics, machine learning, interpretation
Received: 08 Dec 2024; Accepted: 21 Mar 2025.
Copyright: © 2025 Zhou, Liu, Zhang, Su, Luo, Jiang, Han, Huang, Wang, Lan and Wang. This is an open-access article distributed under the terms of the Creative Commons Attribution License (CC BY). The use, distribution or reproduction in other forums is permitted, provided the original author(s) or licensor are credited and that the original publication in this journal is cited, in accordance with accepted academic practice. No use, distribution or reproduction is permitted which does not comply with these terms.
* Correspondence:
Delin Wang, First People's Hospital of Chongqing, Chongqing, China
Disclaimer: All claims expressed in this article are solely those of the authors and do not necessarily represent those of their affiliated organizations, or those of the publisher, the editors and the reviewers. Any product that may be evaluated in this article or claim that may be made by its manufacturer is not guaranteed or endorsed by the publisher.
Research integrity at Frontiers
Learn more about the work of our research integrity team to safeguard the quality of each article we publish.