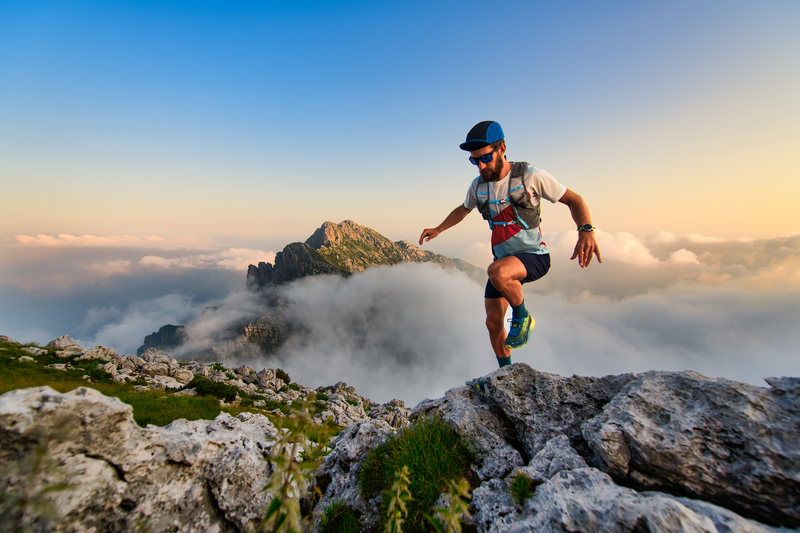
94% of researchers rate our articles as excellent or good
Learn more about the work of our research integrity team to safeguard the quality of each article we publish.
Find out more
ORIGINAL RESEARCH article
Front. Oncol.
Sec. Cancer Imaging and Image-directed Interventions
Volume 15 - 2025 | doi: 10.3389/fonc.2025.1541143
The final, formatted version of the article will be published soon.
You have multiple emails registered with Frontiers:
Please enter your email address:
If you already have an account, please login
You don't have a Frontiers account ? You can register here
Background: Most small renal masses (SRMs) grow slowly and have good prognosis, but a portion of SRMs can also demonstrate aggressive characteristics, which can be explored by the proliferation-related marker Ki67.Methods: A total of 241 patients collected from the two centers were included in the study, of which 145 patients from the First Affiliated Hospital of Guangzhou Medical University were divided into training and validation cohort, while 96 patients from Sun Yat-sen Memorial Hospital were served as test cohort. To ensure the class balance of the outcome measures, the training cohort was oversampled, resulting in an increase of 77 cases in the minority class. After variables processing and feature selecting, optimal artificial intelligence-based model was constructed to predict the Ki67 expression level, and the model performance, interpretation and application development was performed.Results: The baseline characteristics of enrolled patients were described, and no statistically significant differences were found between two centers and cohorts, both before and after oversampling. The optimal model, regularized random forest, was constructed showing AUROC values of 0.802, 0.878, and 0.668, and balanced accuracy of 0.744, 0.808, and 0.679 in the oversampling training, validation, and test cohort, respectively. Model interpretation was performed, and a web application was built.Conclusions: An artificial intelligence-based predictive model for non-invasively assessing the Ki67 expression level of SRMs was developed, thus providing valuable reference for clinical decision-making in these patients.
Keywords: Small renal mass, ki67, CT, Radiomics, artificial intelligence
Received: 07 Dec 2024; Accepted: 10 Feb 2025.
Copyright: © 2025 Lin, Ou, Luo, Jiang, Cen and Zeng. This is an open-access article distributed under the terms of the Creative Commons Attribution License (CC BY). The use, distribution or reproduction in other forums is permitted, provided the original author(s) or licensor are credited and that the original publication in this journal is cited, in accordance with accepted academic practice. No use, distribution or reproduction is permitted which does not comply with these terms.
* Correspondence:
Junyi Lin, First Affiliated Hospital of Guangzhou Medical University, Guangzhou, China
Disclaimer: All claims expressed in this article are solely those of the authors and do not necessarily represent those of their affiliated organizations, or those of the publisher, the editors and the reviewers. Any product that may be evaluated in this article or claim that may be made by its manufacturer is not guaranteed or endorsed by the publisher.
Research integrity at Frontiers
Learn more about the work of our research integrity team to safeguard the quality of each article we publish.