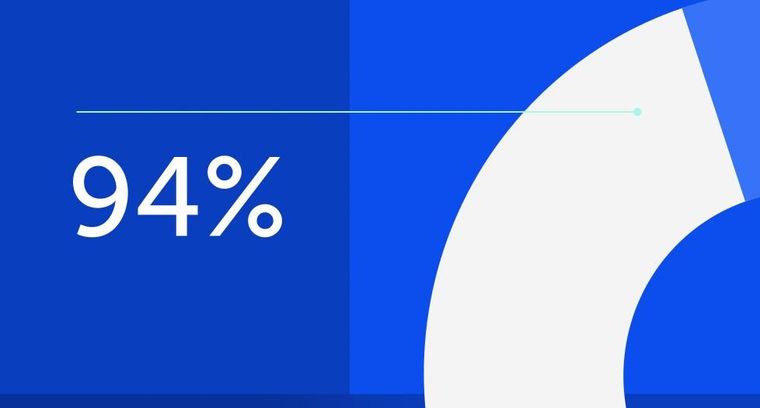
94% of researchers rate our articles as excellent or good
Learn more about the work of our research integrity team to safeguard the quality of each article we publish.
Find out more
ORIGINAL RESEARCH article
Front. Oncol.
Sec. Cancer Epidemiology and Prevention
Volume 15 - 2025 | doi: 10.3389/fonc.2025.1540195
This article is part of the Research TopicAdvancing Personalized Cancer Care: Novel Genetic and Statistical ApproachesView all 5 articles
The final, formatted version of the article will be published soon.
Select one of your emails
You have multiple emails registered with Frontiers:
Notify me on publication
Please enter your email address:
If you already have an account, please login
You don't have a Frontiers account ? You can register here
Pancreatic cancer (PC) is a lethal disease developing from either exocrine or endocrine cells. Efforts to assist early diagnosis focus on liquid biopsy methods, and especially on the detection of Extracellular Vesicles (EVs) secreted from cancer cells in their microenvironment and accumulated in systemic circulation. Multiple studies explore how EVs size, surface biomarkers or content can determine their unique role and function in the recipient cell’s gene expression, metabolism and behavior affecting cancer development.This study aimed to develop a machine learning-driven (ML) pipeline utilizing clinical variables and EV-based features to predict the presence of pancreatic tumors of different nature (exocrine/endocrine) in patients’ plasma compared to patients with benign lesions or age-matched non-oncological patients.All available plasma samples (N=126) and variables were collected prior to surgery. EVs were detected and characterized by flow cytometry-immunostaining. Data including size and a unique set of biomarkers (CD45, CD63 and EphA2) were combined with hematological/biochemical data and processed under two use cases, each formulated as a 3-class classification problem for patient risk stratification. The first use case aimed at classifying patients as with benign lesions or exocrine/endocrine neoplasms. The second use case aimed to distinguish patients with exocrine/endocrine neoplasms from non-oncological patients. Various ML methods were applied, including Logistic Regression, Random Forest, Support Vector Machines, and Extreme Gradient Boosting. Evaluation metrics, as area under the receiver operating characteristic curve (AUC-ROC), were computed, and Shapley values were utilized to determine features with the greatest impact on the discrimination of outcome groups.Analyses identified hematological and biochemical features, among significant predictors. Models demonstrated substantial accuracy and AUC-ROC values based on plasma EVs subpopulations, which scored over 0.90 in accuracy of the Random Forest and XGBoost algorithms, presenting 0.96 +/- 0.03 accuracy in the first use case and 0.93 +/- 0.04 in the second.By leveraging advanced analytical ML-driven approaches and integrating diverse data types, this study achieved significant accuracy, assisting patient’s risk estimation and supporting the feasibility for early detection of pancreatic cancer. Going beyond currently used biomarkers such as CEA, or CA19.9, EV-based features represent an added value offering increased diagnostic capacity.
Keywords: Pancreatic Cancer, Neuroendocrine neoplasms, extracellular vesicles, liquid biopsy, Flow Cytometry, machine learning
Received: 05 Dec 2024; Accepted: 31 Mar 2025.
Copyright: © 2025 Angelioudaki, Iosif, Kourou, Tzingounis, Kigka, Skreka, Costopoulos, Memos, Kataki, Konstadoulakis and Fotiadis. This is an open-access article distributed under the terms of the Creative Commons Attribution License (CC BY). The use, distribution or reproduction in other forums is permitted, provided the original author(s) or licensor are credited and that the original publication in this journal is cited, in accordance with accepted academic practice. No use, distribution or reproduction is permitted which does not comply with these terms.
* Correspondence:
Ioanna Angelioudaki, 2nd Department of Surgery, Aretaieion Hospital, Medical School, National and Kapodistrian University of Athens, Athens, Greece, Athens, Greece
Angeliki Iosif, Unit of Medical Technology and Intelligent Information Systems, Department of Materials Science and Engineering, School of Engineering, University of Ioannina, Ioannina, 45110, Greece
Disclaimer: All claims expressed in this article are solely those of the authors and do not necessarily represent those of their affiliated organizations, or those of the publisher, the editors and the reviewers. Any product that may be evaluated in this article or claim that may be made by its manufacturer is not guaranteed or endorsed by the publisher.
Supplementary Material
Research integrity at Frontiers
Learn more about the work of our research integrity team to safeguard the quality of each article we publish.