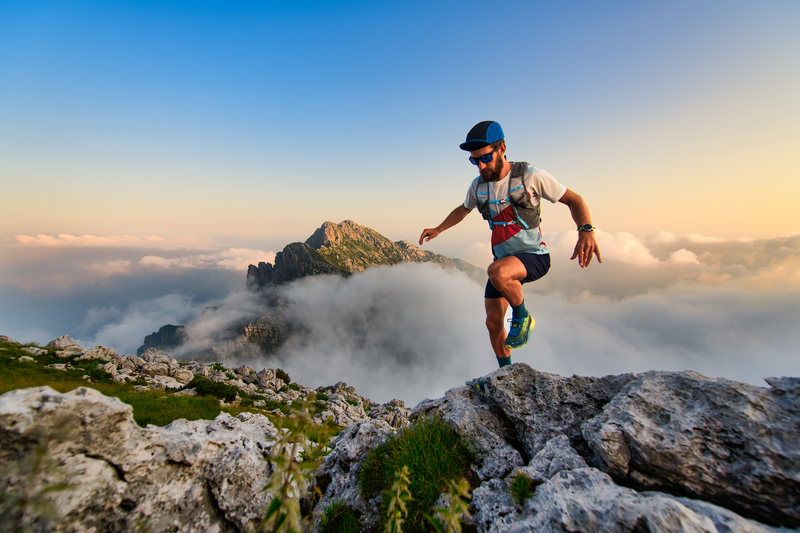
95% of researchers rate our articles as excellent or good
Learn more about the work of our research integrity team to safeguard the quality of each article we publish.
Find out more
REVIEW article
Front. Oncol.
Sec. Cancer Imaging and Image-directed Interventions
Volume 15 - 2025 | doi: 10.3389/fonc.2025.1539911
This article is part of the Research Topic Methods and Applications of Tumour Metabolic Imaging in the Preclinical and Clinical Setting View all 7 articles
The final, formatted version of the article will be published soon.
You have multiple emails registered with Frontiers:
Please enter your email address:
If you already have an account, please login
You don't have a Frontiers account ? You can register here
Dynamic imaging has obtained remarkable achievements among a variety of malignant tumors due to the development of multiple simplified scanning protocols and the emergence of whole-body PET/CT scanners, which promote wider application of dynamic PET/CT. In this paper, we mainly review the acquisition protocols of dynamic imaging, related kinetic parameters, advantages and the application of dynamic PET/CT imaging in malignant tumors, including lung cancer, hepatocellular carcinoma, breast cancer, pancreatic carcinoma, prostate neoplasm, and cancer of head and neck. Dynamic PET/CT imaging is increasingly being applied the diagnosis, staging, efficacy monitoring, and prognosis evaluation of malignant tumors. Although standardized uptake value is the most frequently employed semi-quantitative assessment index for static imaging, it is susceptible to several factors, thus cannot be used to evaluate the tracer kinetic information of the lesion. Dynamic PET/CT imaging can be used to achieve continuous assessment of the metabolic activity of a lesion over a certain time frame through quantitative measurement of kinetic parameters, such as the net uptake rate constant. Compared with conventional static imaging, dynamic scanning can be used for the early estimation of minute metabolic changes in tumors. Besides, dynamic scanning can directly and effectively reflect tracer uptake. Nevertheless, the intricacy of parameter analysis and the lengthy scanning time related to dynamic scanning limits its clinical application. Dynamic imaging has obtained remarkable achievements among a variety of malignant tumors due to the development of multiple simplified scanning protocols and the emergence of whole-body PET/CT scanners, which promote wider application of dynamic PET/CT. In this paper, we mainly review the acquisition protocols of dynamic imaging, related kinetic parameters, advantages and the application of dynamic PET/CT imaging in malignant tumors, including lung cancer, hepatocellular carcinoma, breast cancer, pancreatic carcinoma, prostate neoplasm, and cancer of head and neck.
Keywords: Dynamic PET, Dynamic acquisition, quantification, oncology, clinical application
Received: 05 Dec 2024; Accepted: 24 Mar 2025.
Copyright: © 2025 Wang, Yang, Li, Cheng, Xu and Huang. This is an open-access article distributed under the terms of the Creative Commons Attribution License (CC BY). The use, distribution or reproduction in other forums is permitted, provided the original author(s) or licensor are credited and that the original publication in this journal is cited, in accordance with accepted academic practice. No use, distribution or reproduction is permitted which does not comply with these terms.
* Correspondence:
Jin-bai Huang, The First Affiliated Hospital of Yangtze University, Jingzhou, Hubei Province, China
Disclaimer: All claims expressed in this article are solely those of the authors and do not necessarily represent those of their affiliated organizations, or those of the publisher, the editors and the reviewers. Any product that may be evaluated in this article or claim that may be made by its manufacturer is not guaranteed or endorsed by the publisher.
Research integrity at Frontiers
Learn more about the work of our research integrity team to safeguard the quality of each article we publish.