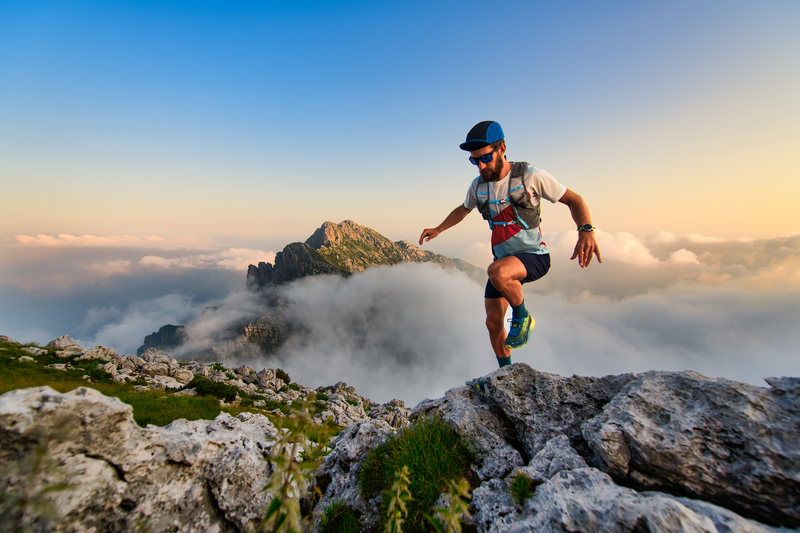
95% of researchers rate our articles as excellent or good
Learn more about the work of our research integrity team to safeguard the quality of each article we publish.
Find out more
ORIGINAL RESEARCH article
Front. Oncol. , 25 March 2025
Sec. Breast Cancer
Volume 15 - 2025 | https://doi.org/10.3389/fonc.2025.1537529
Objectives: Time-dependent diffusion MRI (TD-MRI) can measure tumor tissue microstructure, but its effectiveness in differentiating benign from malignant breast tumors is unclear. This study aims to investigate the diagnostic value of TD-MRI microstructural features for distinguishing between benign and malignant breast tumors.
Methods: This prospective study included 44 patients with malignant breast tumors and 28 with benign tumors. All subjects underwent the IMPULSED protocol on a 3.0-T MRI scanner. Imaging data were analyzed using least squares fitting in MATLAB, yielding Dex (extracellular diffusivity), Vin (intracellular volume fraction), Dmean (cell diameter), Vin/Dmean, and ADC values. The molecular subtypes of breast cancer are classified based on immunohistochemistry (IHC) results.
Results: Malignant tumors exhibited significantly lower Dmean (17.37 ± 2.74 µm vs. 22.47 ± 3.85µm, p<0.0001), higher Vin (0.41 ± 0.13% vs. 0.19 ± 0.10%, p<0.0001), and higher Vin/Dmean (2.13 ± 0.66 vs. 0.93 ± 0.61, p<0.0001) compared to benign tumors. No significant difference was found in Dex (2.15 ± 0.28 um2/ms vs. 2.25 ± 0.31 um2/ms, p>0.05). Strong correlations were observed: positive between ADC and Dmean, and negative between ADC and both Vin and Vin/Dmean. AUC values for Vin (0.92; 95% CI: 0.86-0.99), and Vin/Dmean (0.91; 95% CI: 0.83-0.98) surpassed those for ADC.
Conclusion: TD-MRI microstructure mapping effectively differentiates benign from malignant breast tumors, highlighting its potential to improve diagnostic accuracy for lesions.
By 2020, Breast Cancer has become the most prevalent malignant tumors among women, with a persistently high mortality rate (1, 2). However, there is a lack of highly specific noninvasive indicators to accurately distinguish benign and malignant breast lesions. The cell diameter and density in malignant breast tumors are typically significantly different from those in benign tumors (3). Additionally, cell size plays an important role in assessing cellular functions such as metabolism, proliferation, and tissue growth, making it relevant to the diagnosis and treatment of diseases (4–6).
Quantitative methods for noninvasively measuring cell size in vivo remains lacking. Traditional microscopic methods, such as microscopes, cell counters, and flow cytometry, rely on invasive sampling, which could involve sampling errors and observer bias. In clinical practice, the apparent diffusion coefficient (ADC) is widely used to differentiate between benign and malignant breast tumors (7, 8), but it fails to quantify cell size (9). Time-dependent diffusion-weighted magnetic resonance imaging (TD-MRI) utilizes various diffusion weightings (q-space) and diffusion times (t-space) to provide detailed tissue microstructure information, such as cell size and cell volume fraction at different diffusion length scales (9–11). Incorporating Oscillating Gradient Spin Echo (OGSE) into TD-MRI enhances sensitivity to intracellular diffusion by achieving shorter diffusion times, minimizing the influence of water exchange across cell membranes (12, 13). This method offers a clear advantage over using PGSE alone in TD-MRI (10, 14).
Several researchers have attempted to use TD-MRI to measure microstructural data of tumor tissues, including methods like DDR (15), VERDICT (3, 16), and POMACE (17). However, these methods have limitations, such as prolonged scan times due to extended diffusion times or neglecting microstructural features like cell diameter and intracellular volume fraction. TD-MRI methods based on Pulse Gradient Spin Echo (PGSE) and Oscillating Gradient Spin Echo (OGSE) have been applied in diseases such as prostate cancer and gliomas (15, 18–20) with VERDICT, POMACE and IMPULSED methods. Xu’s application of the IMPULSED (8, 21) (Imaging Microstructural Parameters Using Limited Spectrally Edited Diffusion) model introduced OGSE to measure the microstructural parameters of human breast tumors, demonstrating OGSE’s capability to directly measure cellular- level microstructural characteristics, thus providing valuable insights for tumor development and treatment response assessment. However, the study was limited to a small number of clinical samples and a lack of comparison with clinical indicators.
Therefore, our study aims to use microstructural parameters derived from TD-MRI to explore the relationship between the microstructures of benign and malignant breast tumors, compare the diagnostic efficacy of microstructural parameters and ADC, and investigate the differences in microstructural parameters of malignant breast tumors across distinct molecular subtypes (such as Luminal A, Luminal B, Basal-like, HER2-enriched).
The prospective study was approved by the Ethics Committee of our hospital (24K286-001), with informed consents obtained from all participants. 145 patients who met the inclusion criteria were enrolled between July 2023 and June 2024. The inclusion criteria were: 1) histologically confirmed primary breast cancer or benign breast tumors; 2) availability of clinical and complete pathological data; 3) conventional breast MRI and TD-MRI scans performed within one week before surgery or biopsy; 4) lesion diameter of ≥10 mm; 5) no prior surgical resection, neoadjuvant chemotherapy, or other treatments following breast tumor discovery. The exclusion criteria were: 1) unresectable tumors(n=5); 2) prior breast tumor surgery or other treatments before the breast MRI examination(n=4); 3) Incomplete clinical or medical records(n=12); 4) image quality insufficient for diagnostic needs(n=3); 5) allergies to MRI contrast agents or inability to cooperate with the examination(n=4); 6) No breast MRI examination(n=45). Finally, 44 cases of malignant tumors and 28 cases of benign tumors were included with complete histopathological data from immunohistochemical staining (IHC) conducted on pathology slides.
The TD MRI technique requires acquiring diffusion MRI signals at varying diffusion times to capture the diffusion time-dependence in different microstructural components, therefore measuring diffusion within solid tumors. All scans were performed using a 3.0-T MRI scanner (Ingenia Elition, Philips Healthcare, the Netherlands) with a maximum gradient of 45 mT/m and maximum slew rate of 220 mT/m/ms, using a 16-channel breast array coil with the participant in the prone position.
Figure 1 shows the Time-dependent diffusion MRI (TD-MRI) using a combination of the Pulsed Gradient Spin Echo (PGSE) sequence and the Oscillating Gradient Spin Echo (OGSE) sequence, with different diffusion times, to acquire MRI diffusion signals and determine the diffusion time dependence of various microstructural parameters.
Figure 1. Using the IMPULSED method to acquire pulse diagrams of Pulsed Gradient Spin–Echo and Oscillating Gradient Spin–Echo in the breast. Time–dependent diffusion MRI signals depend on the diffusion time (td). The diffusivity of water molecules within a cellular environment is influenced by this diffusion time. By using PGSE and OGSE diffusion encoding schemes at different diffusion times, measurements can be made to help reconstruct microstructural properties using biophysical models.
After routine MRI scans, which include T1-weighted imaging, T2-weighted imaging, fat-suppressed T2-weighted imaging, and diffusion-weighted imaging (DWI), an OGSE diffusion MRI sequence combining OGSE and PGSE was implemented with trapezoid-cosine gradients and echo-planar imaging acquisition. The positioning of OGSE and PGSE scans was based on fat-suppressed T2-weighted imaging to cover the area where the breast tumor has the largest diameter. OGSE data were acquired at OGSE N2 (33Hz, duration of diffusion gradient = 63.9 ms, two cycles, b = 0, 100, 200, 260 sec/mm2) and OGSE N1 (17Hz, duration of diffusion gradient = 63.9 ms, one cycle, b = 0, 250, 500, 750, 1000 sec/mm2), and PGSE at a diffusion duration and separation of 15.9 and 117.1 ms, respectively (b = 0, 300, 600, 900, 1200, 1500 sec/mm2). The following parameters were used for both sequences: three diffusion directions; repetition time ms/echo time ms, 3000/143; field of view, 160 × 160 mm; in-plane resolution, 2.75 × 2.75 mm; number of slices, 5; and section thickness, 5 mm (Table 1). Finally, enhanced T1-weighted images were obtained.
This study combines PGSE and OGSE to cover a range of diffusion times from short to long (63.9 ms to 117.1 ms). While OGSE with optimized gradient oscillations achieves a shorter effective diffusion time (Δ= 63.9 ms) by balancing total encoding duration (T) and oscillation parameters, it enhances sensitivity to restricted diffusion in smaller cellular compartments (8, 22). In contrast, PGSE extends the coverage to longer diffusion times (Δ=117.1 ms), capturing slower extracellular water mobility. Based on the IMPULSED model, low b-values (0–260 sec/mm2) primarily characterize extracellular diffusion (Dex), whereas higher b-values (up to 1500 sec/mm2) amplify sensitivity to intracellular diffusion, leveraging the pronounced signal attenuation from restricted water motion in confined spaces (23).
All images were transformed from DICOM format to NIFTI format using MRIcroGL software (https://www.nitrc.org/projects/mricrogl/). The region of interest (ROI) on the largest plane of the breast tumor based on OGSE was delineated using ITK-SNAP software (version 3.6, http://www.itksnap.org) to generate a mask image. The ROI excludes tumor necrotic areas and blood vessels, as determined by two radiologists with 5 years of experience in diagnostic imaging, who were blinded to the diagnostic outcome, with reference to contrast-enhanced MRI breast images. Using the IMPULSED model for post-processing of raw data, TD-MRI can provide microstructural information of the lesions. Fitting was performed using the least squares curve fitting in MATLAB software (version 2022b, https://www.mathworks.com/). Dex (unit:µm²/ms), Vin (unit:%), Dmean (unit:µm), and Vin/Dmean (reflecting the percentage of microstructure and cell density) were obtained. The ADC values were obtained by performing log-linear fitting of all b values at each diffusion time (td). The parameters were constrained based on physiologically relevant values, specifically: 0 < Dmean< 30 μm, 0 < Vin < 1, and 0 < Dex < 3.5 μm²/ms.
Tissue sections obtained from surgical resection or biopsy of malignant breast tumors undergo immunohistochemical (IHC) staining to assess estrogen receptor (ER) and progesterone receptor (PR) status (defined as the percentage of positively stained tumor nuclei), human epidermal growth factor receptor 2 (HER2) status, Ki-67 proliferation index, and lymph node metastasis (LN) presence. Patients are classified into molecular subtypes (Luminal A, Luminal B, Basal-like, and HER2-enriched) based on IHC results (24). For Her-2 status, tumors are classified as Her-2 negative if IHC staining is 0 or 1+, and Her-2 positive if staining is 3+ (25). Tumors with IHC staining of 2+ require further confirmation with fluorescence in situ hybridization (FISH): non-amplified FISH results indicate Her-2 negativity, whereas amplified FISH results indicate Her-2 positivity (25).
Statistical analyses were performed using GraphPad Prism software (version 9.5; www.graphpad.com/scientific-software/prism/). Normality of distribution was assessed through the Kolmogorov-Smirnov test for sample sizes >50 and the Shapiro-Wilk test for n ≤ 50. For comparisons of microstructural parameters between benign and malignant breast tumors, parametric data were analyzed with unpaired two-tailed t-tests, while non-parametric data were evaluated using Mann-Whitney-U tests. To compare the differences in time-varying diffusion MRI microstructural parameters among subtypes, a one-way analysis of variance (ANOVA) was performed. Bivariate correlations between microstructural parameters and apparent diffusion coefficient (ADC) values were examined using Pearson’s correlation coefficient for normally distributed variables and Spearman’s rank correlation coefficient for non-normally distributed data. Diagnostic performance was evaluated through receiver operating characteristic (ROC) curve analysis, with the area under the curve (AUC) reported alongside 95% confidence intervals (CI), Sensitivity and Specificity. Statistical significance was defined as p < 0.05 throughout all analyzes.
The baseline and clinical information of all patients with breast tumors are summarized in Table 2. In the benign tumor group, surgical pathology revealed as breast fibroadenoma (n=15), fibroadenomatous hyperplasia (n=8), and intraductal papilloma (n=5). In the malignant tumor group, there are ductal carcinoma in situ (n=4), invasive ductal carcinoma (n=24), invasive lobular carcinoma (n=13), and papillary carcinoma (n=3). None of the patients in the malignant tumor group received any prior treatments (including radiotherapy, chemotherapy, or surgery), and histopathological data were obtained through surgical excision or biopsy.
The Intraclass Correlation Coefficient (ICC) for the delineation of breast tumor ROI by the two radiologists was 0.96 ± 0.17, p < 0.01, demonstrating excellent consistency. Various histopathological types of benign tumors and different ER/PR, Her-2, Ki-67, and LN metastasis statuses of malignant tumors were observed in the microstructural mapping derived from TD-MRI (Figure 2). Appendix S1 presents the diffusion signal intensity under different diffusion times. A significantly decreased mean cell diameter (Dmean) was found in malignant breast tumors compared to those in benign breast tumors (17.37 ± 2.74 µm vs. 22.47 ± 3.85µm, p<0.0001) as derived from OGSE and PGSE in TD-MRI. Additionally, a significantly higher intracellular volume fraction (Vin) was observed in malignant breast tumors compared to benign ones (0.41 ± 0.13% vs. 0.19 ± 0.10%, p<0.0001), and Vin/Dmean was also higher in malignant tumors (2.13 ± 0.66 vs. 0.93 ± 0.61, p<0.0001). However, there was no significant difference in extracellular diffusivity (Dex) between the two groups (p>0.05) (Figure 3, Table 3). The ADCpgse, ADCogseN1 and ADCogseN2 of malignant breast tumors were significantly lower than those of benign breast tumors (ADCpgse, p<0.0001; ADCogseN1, p<0.0001; ADCogseN2, p<0.05).
Figure 2. Microstructural characterization of benign and malignant breast tumors in which malignant breast tumors include five different receptor status and LN status categories and benign breast tumors include three different pathohistological types. (TD–MRI technique requires acquisition of diffusion MRI signals at varying diffusion times by using a combination of oscillating gradient spin–echo (OGSE) and pulsed gradient spin–echo (PGSE) sequences to measure diffusion within solid tumors.).
Figure 3. Group comparison of microstructural properties between Benign and malignant tumors of the breast.
Table 3. Group comparison of microstructural properties between benign and malignant tumors of the breast.
Among breast cancer subtypes classified by immunohistochemical receptor status, no significant intergroup differences were observed in microstructural parameters of malignant breast tumors, including Dmean, Vin Vin/Dmean, Dex (Figure 4). However, significant differences in the ADC with multi-b-value PGSE were identified between Luminal B and Basal-like subtypes (p < 0.05).
Figure 4. Group comparisons of microstructural properties between different molecular subtypes of breast cancer.
Significant correlations were found among ADC values (including ADCpgse and ADCogseN1) with Dmean, Vin, and Vin/Dmean. Specifically, Dmean showed a strong positive correlation with ADCpgse and ADCogseN1 values (r=0.75 and r=0.73, p<0.0001), while Vin and Vin/Dmean demonstrated highly negative correlations with ADCpgse and ADCogseN1 (Vin: r=-0.87 and r=-0.79, p<0.0001; Vin/Dmean: r=-0.88 and r=-0.82, p<0.0001). However, Dex did not show significant correlations with ADCpgse and ADCogseN1 values (r=0.53 and r=0.52, p<0.0001). Furthermore, the correlation coefficients of Dmean, Vin, Vin/Dmean, and Dex with ADCogseN2 were approximately 0.13 (p>0.05), -0.21 (p>0.05), -0.27 (p<0.05), and 0.49 (p<0.0001), respectively (Figure 5). These results indicate that ADC values are significantly influenced by Dmean and Vin, while the impact of Dex is comparatively minor.
Figure 5. Correlation of microstructural properties with ADC values including ADCpgse, ADCogseN1, ADCogseN2.
Compared with ADC values, Dmean, Vin and Vin/Dmean demonstrated superior diagnostic performance in distinguishing between benign and malignant breast tumors, with the following AUC values: Dmean=0.85 (95% CI: 0.75-0.95), Vin=0.92 (95% CI: 0.86-0.997), and Vin/Dmean = 0.91 (95% CI: 0.83-0.98). In contrast, Dex showed an AUC value of 0.62 (95% CI: 0.48-0.75) (Figure 6, Table 4). The AUC values for ADCpgse, ADCogseN1 and ADCogseN2 were 0.86 (95% CI: 0.77, 0.95), 0.77 (95% CI: 0.65, 0.89), and 0.69 (95% CI: 0.56, 0.81), respectively.
Figure 6. ROC curves of microstructural properties for benign–malignant differentiation of breast tumors.
Table 4. Diagnostic performance of time–dependent diffusion mri–derived microstructural parameters between benign and malignant tumors of the breast.
This study focuses on the use of TD-MRI in differentiating between benign and malignant breast tumors. With a larger sample size, decreased Dmean and elevated Vin and Vin/Dmean were observed in malignant breast tumors. The diagnostic efficacy of Dmean, Vin, and Vin/Dmean in distinguishing benign from malignant breast tumors was higher than that of ADC. Furthermore, no significant correlation was found between microscopic structural indices and different molecular subtypes of breast cancer.
Compared to Xu’s study (8), our research validated the diagnostic accuracy of OGSE in distinguishing between benign and malignant breast tumors using the histopathology as the gold standard. Moreover, our study careful delineated regions of interest (ROIs) in breast tumors, excluding areas of necrosis and cystic changes, which enabled more precise measurement of microstructural data. Ba’s (9) study focused only on malignant breast tumors, whereas our research included both benign and malignant cases, further evaluating the application of TD-MRI in breast tumors. Additionally, the inclusion criteria specifying breast tumor greater than 10 mm was based on previous studies (21) investigating various IHC statuses and LN statuses in breast cancer. This criterion was also ensured differentiation from benign breast nodules and to facilitate accurate lesion measurement in DWI sequences.
Due to the rapid growth and high heterogeneity of malignant breast tumor cells, the Dmean of malignant breast tumors is lower than that of benign tumors. However, during actual pathological processes, the active division of malignant cells frequently leads to morphological abnormalities, including: cellular volume differentiation (smaller daughter cells generated through division or abnormally enlarged multinucleated giant cells) and irregular geometric configurations (spindle-shaped or irregular polygonal forms) (26, 27). The current IMPULSED model operates under the theoretical assumption of “spherical cells with uniform distribution,” representing an oversimplification that exhibits marked deviation from the actual microstructure of malignant tumors, while the Dmean as a mean value may merely represent a rough approximation. These unaddressed factors may potentially introduce systematic deviations in Dmean measurements. Although the Dmean of benign breast tumors in this study was higher than that of malignant tumors, the Dmean values of malignant breast tumors measured here appeared slightly elevated compared to prior studies (9). This discrepancy likely arises from our exclusion of necrotic tumor components and vascular structures during region of interest (ROI) delineation. The intracellular volume fraction (Vin) reflects cell density within solid tumor cells (28). The extracellular matrix (ECM), a fundamental component of all tissues and organs, is essential for multicellular organisms. In cancer, ECM alterations can promote tumor cell growth, and extracellular diffusivity (Dex) can quantify the ECM (29, 30). This explains why the Vin of malignant breast tumors is higher than that of benign tumors.
Currently, the clinical method for distinguishing between benign and malignant breast tumors mainly relies on ADC (10, 31). In our study, ADC values (particularly ADCpgse) were significantly lower in malignant breast tumors compared to benign ones, consistent with previous findings (10, 15). However, when comparing the diagnostic performance of ADC with microstructural data from TD–MRI, Vin (AUC = 0.92), and Vin/Dmean (AUC = 0.91) exhibited higher AUC values than ADC. This discrepancy can be attributed to the influence of tumor cell size, density, and transmembrane water mobility on ADC values, factors minimized in the microstructural data derived from TD–MRI. Among the microstructural data, Dmean showed superior diagnostic performance in distinguishing between benign and malignant breast tumors.
Different breast cancer subtypes, determined by immunohistochemical receptor status, exhibit varying cell densities, vascular distributions, and invasive capabilities (32). In our study, no significant differences in microstructural characteristics were observed between different breast cancer subtypes, which is contrary to the findings reported by Wang (33). Although differences in ADCpgse were observed between Luminal B and Basal–like subtypes in our study, these findings remain inconclusive due to the limited sample size. This is different from the results of a meta–analysis including 2990 breast tumors, where ADC could not be used for molecular subtypes of breast cancer in their study (34). In other studies investigating hormone receptor expression status in breast cancer, Kim SH et al. (35) demonstrated no statistically significant differences in ADC values based on hormone receptor expression status, HER–2 status, or lymph node metastasis. However, some studies have indicated associations between ADC values and hormone receptor status (36–38). One study found higher ADC values in HER–2 positive breast cancer (39), and another showed a correlation between ADC values and lymph node metastasis in invasive ductal carcinoma (40). Therefore, these findings require further investigation with a larger sample size.
The “Cellularity” parameter derived from TD–MRI is calculated as Vin/Dmean. In Wu’s study on prostate cancer, Cellularity demonstrated the highest diagnostic performance in distinguishing prostate cancer from clinically insignificant prostate cancer (20). In Wang’s study on predicting the effectiveness of neoadjuvant therapy in breast cancer, Cellularity had the highest AUC value among all TD–MRI parameters (34). However, this parameter has not yet been widely recognized by scientists. Although this definition differs from the traditional concept of cellularity in pathology (which refers to the number of cell cross–sections per unit area on histological slides), we believe it holds potential within the TD–MRI framework and will further explore its clinical significance in future studies. In the patients included in our study, the sample sizes for certain subtypes, such as ductal intraductal papilloma, ductal carcinoma in situ, and lobular carcinoma in situ, were small. As a result, we were unable to perform a detailed analysis of the differences in microstructural parameters between the various pathological subtypes. In future studies, we plan to increase the sample size to further investigate this aspect. It is noteworthy that in cases of fibroadenoma and fibroadenomatous hyperplasia, we observed a weak dependency of diffusion signal decay on diffusion time (Appendix S1), resulting in overestimated fitted cell sizes (highlighted in red in Figure 2). This phenomenon may be attributed to the unique histopathological features of these lesions: fibroadenomas are predominantly composed of extracellular matrix components and exhibit relatively low cellular density, which may diminish the sensitivity of diffusion time dependency to intracellular restrictions.
There are several limitations in this study. Firstly, the selection bias due to our inclusion criteria has led to a limited number of positive cases in different subtypes of malignant breast tumors. Additionally, the number of patients with malignant breast tumors was significantly higher than those with benign tumors. This phenomenon is due to many benign breast tumor patients opting only for breast ultrasound or mammography. Additionally, some patients with benign tumors did not undergo tumor resection or biopsy, resulting in a lack of pathological data. This may hinder the identification of significant intergroup differences in microstructural data when comparing breast tumors with different immunophenotypes, contrary to findings in some previous studies. Secondly, being a single–center study, our results may not fully generalize to broader populations due to variations in patient demographics and clinical practices across different centers. Thirdly, the use of a combination of biopsy and surgical pathology results in our study poses another limitation. Not all patients with breast tumors underwent surgical excision, especially those with clinically insignificant diseases (such as benign breast tumors confirmed by biopsy and showing no changes over time) or advanced breast cancer. This variation in tissue sampling methods could lead to differences in the microstructural characteristics of tumor tissues. Finally, relative slow acquisition speed lead to only 5 slices obtained in this study. This is because the multi–b–value scanning was used to ensure accurate fitting and achieve sufficient signal–to–noise ratio. Meanwhile, relatively low TD–MRI image resolution leads to the need of other imaging modalities to identify the tumor localization. In future clinical applications, the number of b–value can be reduced to shorten scanning time.
In summary, TD–MRI has been proven with significant advantage over ADC in distinguishing between benign and malignant breast tumors in this study. In future, multi–center studies could be achieved to explore microstructural differences among tumors of different pathological types to enhance our understanding and clinical applications.
The original contributions presented in the study are included in the article/Supplementary Material. Further inquiries can be directed to the corresponding author.
The studies involving humans were approved by Ethics Committee of The First Hospital of Jilin University. The studies were conducted in accordance with the local legislation and institutional requirements. The participants provided their written informed consent to participate in this study.
LB: Data curation, Methodology, Project administration, Software, Supervision, Validation, Writing – original draft, Writing – review & editing. SL: Data curation, Methodology, Project administration, Supervision, Validation, Writing – original draft, Writing – review & editing. ZW: Project administration, Supervision, Writing – review & editing. YS: Supervision, Writing – review & editing. YQ: Supervision, Writing – review & editing. ZS: Writing – review & editing. XZ (7th author): Software, Writing – review & editing. XC: Writing – review & editing. XZ (9th author): Writing – review & editing. JZ: Writing – review & editing. TJ: Data curation, Methodology, Supervision, Validation, Writing – original draft, Writing – review & editing.
The author(s) declare that financial support was received for the research and/or publication of this article. This project is supported by the Department of Finance of Jilin Province (JLSWSRCZX2023–83, JLSWSRCZX2020–052).
Authors ZS and XZ were employed by Philips Healthcare.
The authors declare that the research was conducted in the absence of any commercial or financial relationships that could be construed as a potential conflict of interest.
The author(s) declare that no Generative AI was used in the creation of this manuscript.
All claims expressed in this article are solely those of the authors and do not necessarily represent those of their affiliated organizations, or those of the publisher, the editors and the reviewers. Any product that may be evaluated in this article, or claim that may be made by its manufacturer, is not guaranteed or endorsed by the publisher.
The Supplementary Material for this article can be found online at: https://www.frontiersin.org/articles/10.3389/fonc.2025.1537529/full#supplementary-material
DDR, Diffusion Dispersion Rate; ER, Estrogen receptor; FISH, Fluorescence in situ hybridization; HER–2, Human epidermal growth factor receptor 2; IHC, Immunohistochemical; IMPULSED, Imaging microstructural parameters using limited spectrally edited diffusion; ICC, Intraclass correlation coefficient; OGSE, Oscillating gradients spin–echo; PGSE, Pulsed gradients spin–echo; POMACE, Pulsed and oscillating gradient MRI for assessment of cell size and extracellular; PR, Progesterone receptor; VERDICT, Vascular, extracellular, and restricted diffusion for cytometry in tumors.
1. Gansler T, Ganz PA, Grant M, Greene FL, Johnstone P, Mahoney M, et al. Sixty years of CA: a cancer journal for clinicians. CA Cancer J Clin. (2010) 60:345–50. doi: 10.3322/caac.20088
2. Sung H, Ferlay J, Siegel RL, Laversanne M, Soerjomataram I, Jemal A, et al. Global cancer statistics 2020: GLOBOCAN estimates of incidence and mortality worldwide for 36 cancers in 185 countries. CA Cancer J Clin. (2021) 71:209–49. doi: 10.3322/caac.21660
3. Panagiotaki E, Walker–Samuel S, Siow B, Johnson SP, Rajkumar V, Pedley RB, et al. Noninvasive quantification of solid tumor microstructure using VERDICT MRI. Cancer Res. (2014) 74:1902–12. doi: 10.1158/0008-5472.CAN-13-2511
4. Sasieni PD, Shelton J, Ormiston–Smith N, Thomson CS, Silcocks PB. What is the lifetime risk of developing cancer? The effect of adjusting for multiple primaries. Br J Cancer. (2011) 105:460–5. doi: 10.1038/bjc.2011.250
5. Hoffmann E, Gerwing M, Niland S, Niehoff R, Masthoff M, Geyer C, et al. Profiling specific cell populations within the inflammatory tumor microenvironment by oscillating–gradient diffusion–weighted MRI. J Immunother Cancer. (2023) 11:e006092. doi: 10.1136/jitc-2022-006092
6. Kozlowski J, Konarzewski M, Gawelczyk AT. Cell size as a link between noncoding DNA and metabolic rate scaling. Proc Natl Acad Sci U S A. (2003) 100:14080–5. doi: 10.1073/pnas.2334605100
7. Jiang X, Li H, Devan SP, Gore JC, Xu J. MR cell size imaging with temporal diffusion spectroscopy. Magn Reson Imaging. (2021) 77:109–23. doi: 10.1016/j.mri.2020.12.010
8. Xu J, Jiang X, Li H, Arlinghaus LR, McKinley ET, Devan SP, et al. Magnetic resonance imaging of mean cell size in human breast tumors. Magn Reson Med. (2020) 83:2002–14. doi: 10.1002/mrm.28056
9. Ba R, Wang X, Zhang Z, Li Q, Sun Y, Zhang J, et al. Diffusion–time dependent diffusion MRI: effect of diffusion–time on microstructural mapping and prediction of prognostic features in breast cancer. Eur Radiol. (2023) 33:6226–37. doi: 10.1007/s00330-023-09623-y
10. Reynaud O. Time–dependent diffusion MRI in cancer: tissue modeling and applications. Front Phys. (2017) 5:58. doi: 10.3389/fphy.2017.00058
11. Jiang X, Li H, Xie J, Zhao P, Gore JC, Xu J. Quantification of cell size using temporal diffusion spectroscopy. Magn Reson Med. (2016) 75:1076–85. doi: 10.1002/mrm.25684
12. Kamimura K, Kamimura Y, Nakano T, Hasegawa T, Nakajo M, Yamada C, et al. Differentiating brain metastasis from glioblastoma by time–dependent diffusion MRI. Cancer Imaging. (2023) 23:75. doi: 10.1186/s40644-023-00595-2
13. Baron CA, Beaulieu C. Oscillating gradient spin–echo (OGSE) diffusion tensor imaging of the human brain. Magn Reson Med. (2014) 72:726–36. doi: 10.1002/mrm.24987
15. Gore JC, Xu J, Colvin DC, Yankeelov TE, Parsons EC, Does MD. Characterization of tissue structure at varying length scales using temporal diffusion spectroscopy. NMR BioMed. (2010) 23:745–56. doi: 10.1002/nbm.v23:7
16. Panagiotaki E, Chan RW, Dikaios N, Ahmed HU, O'Callaghan J, Freeman A, et al. Microstructural characterization of normal and Malignant human prostate tissue with vascular, extracellular, and restricted diffusion for cytometry in tumours magnetic resonance imaging. Invest Radiol. (2015) 50:218–27. doi: 10.1097/RLI.0000000000000115
17. Reynaud O, Winters KV, Hoang DM, Wadghiri YZ, Novikov DS, Kim SG. Pulsed and oscillating gradient MRI for assessment of cell size and extracellular space (POMACE) in mouse gliomas. NMR Biomed. (2016) 29:1350–63. doi: 10.1002/nbm.v29.10
18. Jiang X, Dudzinski S, Beckermann KE, Young K, McKinley E, McIntyre JO, et al. MRI of tumor T cell infiltration in response to checkpoint inhibitor therapy. J Immunother Cancer. (2020) 8:e000328. doi: 10.1136/jitc-2019-000328
19. Iima M, Yamamoto A, Kataoka M, Yamada Y, Omori K, Feiweier T, et al. Time–dependent diffusion MRI to distinguish Malignant from benign head and neck tumors. J Magn Reson Imaging. (2019) 50:88–95. doi: 10.1002/jmri.26578
20. Wu D, Jiang K, Li H, Zhang Z, Ba R, Zhang Y, et al. Time–dependent diffusion MRI for quantitative microstructural mapping of prostate cancer. Radiology. (2022) 303:578–87. doi: 10.1148/radiol.211180
21. Xu J, Li K, Smith RA, Waterton JC, Zhao P, Chen H, et al. Characterizing tumor response to chemotherapy at various length scales using temporal diffusion spectroscopy. PloS One. (2012) 7:e41714. doi: 10.1371/journal.pone.0041714
22. Jiang X, Li H, Xie J, McKinley ET, Zhao P, Gore JC, et al. In vivo imaging of cancer cell size and cellularity using temporal diffusion spectroscopy. Magn Reson Med. (2017) 78:156–64. doi: 10.1002/mrm.26356
23. Novikov DS, Fieremans E, Jespersen SN, Kiselev VG. Quantifying brain microstructure with diffusion MRI: Theory and parameter estimation. NMR Biomed. (2019) 32:e3998. doi: 10.1002/nbm.v32.4
24. Cornejo KM, Kandil D, Khan A, Cosar EF. Theranostic and molecular classification of breast cancer. Arch Pathol Lab Med. (2014) 138:44–56. doi: 10.5858/arpa.2012-0442-RA
25. Fujii T, Kogawa T, Dong W, Sahin AA, Moulder S, Litton JK, et al. Revisiting the definition of estrogen receptor positivity in HER2–negative primary breast cancer. Ann Oncol. (2017) 28(10):2420–8. doi: 10.1093/annonc/mdx397
26. Ganem NJ, Storchova Z, Pellman D. Tetraploidy, aneuploidy and cancer. Curr Opin Genet Dev. (2007) 17:157–62. doi: 10.1016/j.gde.2007.02.011
27. Sahai E, Astsaturov I, Cukierman E, DeNardo DG, Egeblad M, Evans RM, et al. A framework for advancing our understanding of cancer–associated fibroblasts. Nat Rev Cancer. (2020) 20:174–86. doi: 10.1038/s41568-019-0238-1
28. Xu J, Xie J, Jourquin J, Colvin DC, Does MD, Quaranta V, et al. Influence of cell cycle phase on apparent diffusion coefficient in synchronized cells detected using temporal diffusion spectroscopy. Magn Reson Med. (2011) 65:920–6. doi: 10.1002/mrm.22704
29. Alexander DC. A general framework for experiment design in diffusion MRI and its application in measuring direct tissuemicrostructure features. Magn Reson Med. (2008) 60:439–48. doi: 10.1002/mrm.21646
30. Girigoswami K, Saini D, Girigoswami A. Extracellular matrix remodeling and development of cancer. Stem Cell Rev Rep. (2021) 17:739–47. doi: 10.1007/s12015-020-10070-1
31. Wekking D, Porcu M, De Silva P, Saba L, Scartozzi M, Solinas C. Breast MRI: clinical indications, recommendations, and future applications in breast cancer diagnosis. Curr Oncol Rep. (2023) 25:257–67. doi: 10.1007/s11912-023-01372-x
32. Fitzgerald RC, Antoniou AC, Fruk L, Rosenfeld N. The future of early cancer detection. Nat Med. (2022) 28:666–77. doi: 10.1038/s41591-022-01746-x
33. Wang X, Ba R, Huang Y, Cao Y, Chen H, Xu H, et al. Time–dependent diffusion MRI helps predict molecular subtypes and treatment response to neoadjuvant chemotherapy in breast cancer. Radiology. (2024) 313:e240288. doi: 10.1148/radiol.240288
34. Meyer HJ, Wienke A, Surov A. Diffusion–weighted imaging of different breast cancer molecular subtypes. A systematic review and meta analysis. Breast Care. (2022) 17(1):47–54. doi: 10.1159/000514407
35. Kim SH, Cha ES, Kim HS, Kang BJ, Choi JJ, Jung JH, et al. Diffusion–weighted imaging of breast cancer: Correlation of the apparent diffusion coefficient value with prognostic factors. J Magn Reson Imag. (2009) 30:615–20. doi: 10.1002/jmri.21884
36. Iima M, Honda M, Sigmund EE, Ohno Kishimoto A, Kataoka M, Togashi K. Diffusion MRI of the breast: Current status and future directions. J Magn Reson Imaging. (2020) 52:70–90. doi: 10.1002/jmri.26908
37. Kitajima K, Yamano T, Fukushima K, Miyoshi Y, Hirota S, Kawanaka Y, et al. Correlation of the SUVmax of FDG–PET and ADC values of diffusion–weighted MR imaging with pathologic prognostic factors in breast carcinoma. Eur J Radiol. (2016) 85:943–9. doi: 10.1016/j.ejrad.2016.02.015
38. Choi BB, Kim SH, Kang BJ, Lee JH, Song BJ, Jeong SH, et al. Diffusion–weighted imaging and FDG PET/CT: predicting the prognoses with apparent diffusion coefficient values and maximum standardized uptake values in patients with invasive ductal carcinoma. World J Surg Oncol. (2012) 28:126. doi: 10.1186/1477-7819-10-126
39. Baba S, Isoda T, Maruoka Y, Kitamura Y, Sasaki M, Yoshida T, et al. Diagnostic and prognostic value of pretreatment SUV in 18F FDG/PET in breast cancer: comparison with apparent diffusion coefficient from diffusion–weighted MR imaging. J Nucl Med. (2014) 55:736–42. doi: 10.2967/jnumed.113.129395
Keywords: time-dependent diffusion MRI, breast tumor, microstructural characteristics, ADC, diagnostic efficacy
Citation: Bao L, Li S, Wang Z, Sun Y, Qiu Y, Shen Z, Zhang X, Chen X, Zhang X, Zhang J and Ji T (2025) Advantages of time-dependent diffusion MRI for quantitative microstructural mapping in breast tumors. Front. Oncol. 15:1537529. doi: 10.3389/fonc.2025.1537529
Received: 01 December 2024; Accepted: 06 March 2025;
Published: 25 March 2025.
Edited by:
Eric Edward Sigmund, New York University, United StatesReviewed by:
Paola Porcari, Memorial Sloan Kettering Cancer Center, United StatesCopyright © 2025 Bao, Li, Wang, Sun, Qiu, Shen, Zhang, Chen, Zhang, Zhang and Ji. This is an open-access article distributed under the terms of the Creative Commons Attribution License (CC BY). The use, distribution or reproduction in other forums is permitted, provided the original author(s) and the copyright owner(s) are credited and that the original publication in this journal is cited, in accordance with accepted academic practice. No use, distribution or reproduction is permitted which does not comply with these terms.
*Correspondence: Tiefeng Ji, cHlnY25tQGpsdS5lZHUuY24=
†These authors have contributed equally to this work
Disclaimer: All claims expressed in this article are solely those of the authors and do not necessarily represent those of their affiliated organizations, or those of the publisher, the editors and the reviewers. Any product that may be evaluated in this article or claim that may be made by its manufacturer is not guaranteed or endorsed by the publisher.
Research integrity at Frontiers
Learn more about the work of our research integrity team to safeguard the quality of each article we publish.