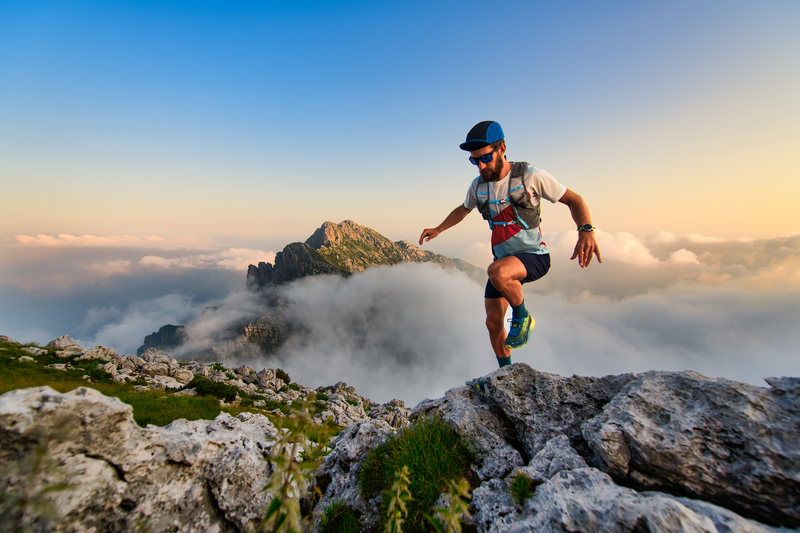
94% of researchers rate our articles as excellent or good
Learn more about the work of our research integrity team to safeguard the quality of each article we publish.
Find out more
ORIGINAL RESEARCH article
Front. Oncol.
Sec. Molecular and Cellular Oncology
Volume 15 - 2025 | doi: 10.3389/fonc.2025.1537481
The final, formatted version of the article will be published soon.
You have multiple emails registered with Frontiers:
Please enter your email address:
If you already have an account, please login
You don't have a Frontiers account ? You can register here
Background: Hepatocellular carcinoma (HCC) is a leading cause of cancer-related deaths with limited treatment options. Tumor metabolic disorder is elevated in HCC and activates the aryl hydrocarbon receptor (AHR), a transcription factor implicated in cancer progression. However, the role of AHR in regulating long non-coding RNAs (lncRNAs) and their impact on glycolipid metabolism remains underexplored. Materials and Methods: We investigated AHR's influence on several HCC cell lines treated with the AHR ligand. RNA sequencing was performed to identify the differentially expressed (DE) lncRNAs and mRNAs. We analyzed the differences and then conducted functional pathway enrichment of the identified DE lncRNAs and mRNAs. Furthermore, we constructed co-expression networks of lncRNAs and mRNAs and performed survival analysis using The Cancer Genome Atlas (TCGA) data. Results: RNA sequencing identified a substantial number of lncRNAs and mRNAs. DEG analysis identified the significant differences between them related to cancer progression, with pathways such as PI3K-Akt, VEGF, and PPAR signaling highlighted. A co-expression network was utilized to elucidate the lncRNA–mRNA interactions and their regulation of glycolipid metabolism.Survival analysis identified the AHR-regulated lncRNAs associated with poor prognosis, like ASAP1-IT1 and RMDN2-AS1. Conclusion: This study clarifies AHR's role in regulating gene expression and metabolism in HCC, revealing novel lncRNA biomarkers and potential therapeutic targets that could aid HCC . Further research is needed to explore AHR's effects on the regulation of glucose-lipid metabolism in HCC.
Keywords: Hepatocellular Carcinoma, RNA-sequencing, Aryl hydrocarbon receptors, Glucose and lipid metabolism, mRNAs
Received: 30 Nov 2024; Accepted: 21 Feb 2025.
Copyright: © 2025 Xiao, Liu, Qu, Liu, Li, Chen, Zhou and Liu. This is an open-access article distributed under the terms of the Creative Commons Attribution License (CC BY). The use, distribution or reproduction in other forums is permitted, provided the original author(s) or licensor are credited and that the original publication in this journal is cited, in accordance with accepted academic practice. No use, distribution or reproduction is permitted which does not comply with these terms.
* Correspondence:
Yanping Liu, Second Affiliated Hospital of University of South China, Hengyang, China
Disclaimer: All claims expressed in this article are solely those of the authors and do not necessarily represent those of their affiliated organizations, or those of the publisher, the editors and the reviewers. Any product that may be evaluated in this article or claim that may be made by its manufacturer is not guaranteed or endorsed by the publisher.
Research integrity at Frontiers
Learn more about the work of our research integrity team to safeguard the quality of each article we publish.