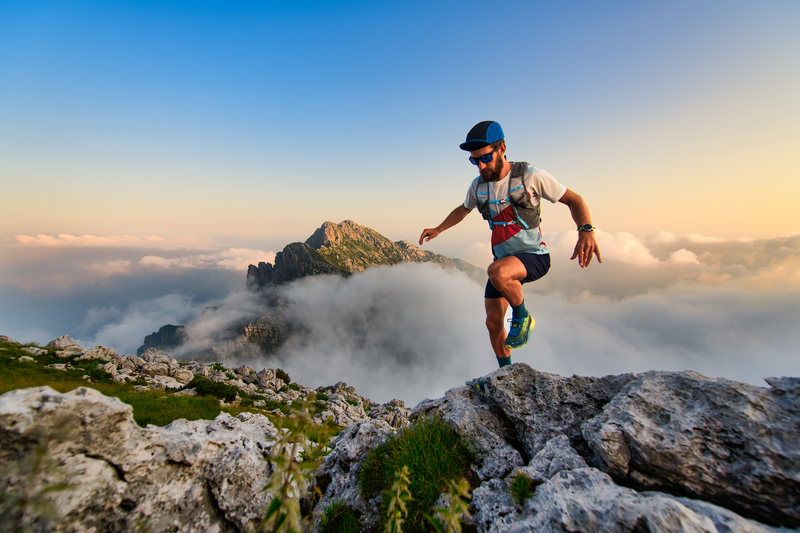
95% of researchers rate our articles as excellent or good
Learn more about the work of our research integrity team to safeguard the quality of each article we publish.
Find out more
ORIGINAL RESEARCH article
Front. Oncol.
Sec. Radiation Oncology
Volume 15 - 2025 | doi: 10.3389/fonc.2025.1534740
This article is part of the Research Topic Recent Advances in Radiation Oncology for the Management of Thoracic Malignancies View all 4 articles
The final, formatted version of the article will be published soon.
You have multiple emails registered with Frontiers:
Please enter your email address:
If you already have an account, please login
You don't have a Frontiers account ? You can register here
The integration of artificial intelligence (AI) in automatic image segmentation systems enhances clinical target volume (CTV) evaluation for small cell lung cancer (SCLC). This study analyzed data from 180 SCLC patients (2010–2021) treated with curative radiotherapy, utilizing AI-driven segmentation and recursive feature elimination to model recurrence. Tumor size (≥5cm) independently impacted local control (HR=1.635, p=0.028), with 3-year recurrence rates of 61.1% vs. 86.7% (p=0.004). Recurrence predominantly occurred in regions 10R, 10L, 4R, and 7 (67.65% of cases). A random forest model incorporating 110 clinical variables achieved 77% accuracy in predicting recurrence. AI-based CTV delineation identified initial tumor regions (GTV/GTVnd) as critical recurrence zones, offering a clinically viable tool for optimizing radiotherapy targeting and patient outcomes.
Keywords: Small Cell Lung Cancer, Clinical target volume (CTV), artificial intelligence, Local recurrence, Prediction model
Received: 26 Nov 2024; Accepted: 04 Apr 2025.
Copyright: © 2025 Shen, Shen, Wang, Guan, Di, Liu, Chen, Li, Hu and ZHANG. This is an open-access article distributed under the terms of the Creative Commons Attribution License (CC BY). The use, distribution or reproduction in other forums is permitted, provided the original author(s) or licensor are credited and that the original publication in this journal is cited, in accordance with accepted academic practice. No use, distribution or reproduction is permitted which does not comply with these terms.
* Correspondence:
Jie Shen, Chinese Academy of Medical Sciences and Peking Union Medical College, Dongcheng, China
Disclaimer: All claims expressed in this article are solely those of the authors and do not necessarily represent those of their affiliated organizations, or those of the publisher, the editors and the reviewers. Any product that may be evaluated in this article or claim that may be made by its manufacturer is not guaranteed or endorsed by the publisher.
Research integrity at Frontiers
Learn more about the work of our research integrity team to safeguard the quality of each article we publish.