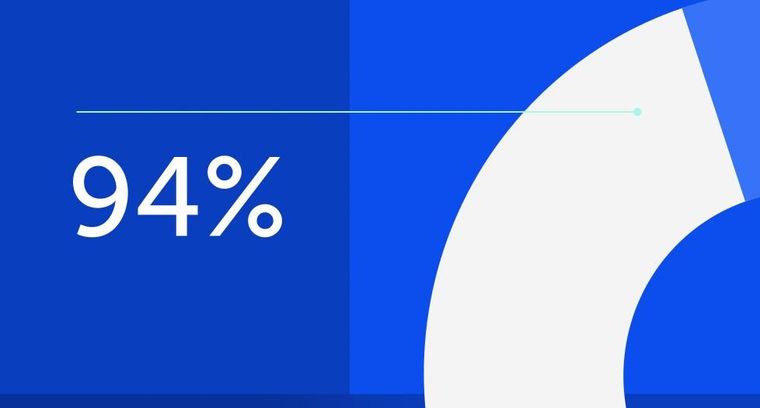
94% of researchers rate our articles as excellent or good
Learn more about the work of our research integrity team to safeguard the quality of each article we publish.
Find out more
ORIGINAL RESEARCH article
Front. Oncol.
Sec. Gastrointestinal Cancers: Colorectal Cancer
Volume 15 - 2025 | doi: 10.3389/fonc.2025.1531836
The final, formatted version of the article will be published soon.
Select one of your emails
You have multiple emails registered with Frontiers:
Notify me on publication
Please enter your email address:
If you already have an account, please login
You don't have a Frontiers account ? You can register here
Purpose: To evaluate the prognostic value of the monocyte to lymphocyte ratio (MLR) and folate receptor-positive circulating tumor cells (FR+CTCs) in patients with colorectal cancer (CRC) and to develop predictive model for post-treatment survival using machine learning (ML) algorithms. Methods: We retrospectively analyzed 67 CRC patients treated with radical surgery or chemoradiotherapy at The Central Hospital of Wuhan from January 2020 to December 2022. MLR, neutrophil to lymphocyte ratio (NLR), platelet to lymphocyte ratio (PLR) and FR+CTCs were categorized into high and low groups and clinicopathologic features were compared. Progression-Free Survival (PFS) and Overall Survival (OS) were analyzed using COX analysis and the Kaplan-Meier survival curve. Three ML algorithms, namely, random forest (RF), support vector machine (SVM), and logistic regression (LR), were utilized to construct the predictive models, and their performance metrics including accuracy, sensitivity, specificity, positive predictive value (PPV), negative predictive value (NPV), precision, recall, F1 value, AUC, and calibration curve were compared. Results: MLR, FR+ CTCs, and T stage independently predicted PFS (P<0.05), both higher MLR and FR+CTCs levels indicating a significantly shorter PFS (P=0.004). The T stage was the only factor predictive of OS (P=0.043). NLR and PLR did not show significant prognostic effects on PFS or OS (P > 0.05). The RF model demonstrated superior performance with an accuracy of 0.63, sensitivity of 0.69, PPV of 0.75, a precision of 0.43, a recall of 0.5, and an F1 value of 0.43, outperforming the other models. Conclusion: High MLR and high FR+CTCs are associated with a poorer PFS in CRC patients, suggesting their utility in prognostic assessment. NLR and PLR did not show significant prognostic value in this study. The RF algorithm-based model showed the best predictive performance for post-radical treatment outcomes in CRC.
Keywords: Colorectal cancer1, monocyte to lymphocyte ratio (MLR) 2, folate receptor-positive circulating tumor cells (FR+CTCs) 3, prognostic4, machine learning (ML)5, prediction model6
Received: 21 Nov 2024; Accepted: 08 Apr 2025.
Copyright: © 2025 Pan, Lu, Lu and Zhang. This is an open-access article distributed under the terms of the Creative Commons Attribution License (CC BY). The use, distribution or reproduction in other forums is permitted, provided the original author(s) or licensor are credited and that the original publication in this journal is cited, in accordance with accepted academic practice. No use, distribution or reproduction is permitted which does not comply with these terms.
* Correspondence:
Hongda Lu, Department of Oncology, The Central Hospital of Wuhan, Tongji Medical College, Huazhong University of Science and Technology, Wuhan, China
Hongfeng Zhang, Department of Pathology, The Central Hospital of Wuhan, Tongji Medical College, Huazhong University of Science and Technology, Wuhan, Hebei Province, China
Disclaimer: All claims expressed in this article are solely those of the authors and do not necessarily represent those of their affiliated organizations, or those of the publisher, the editors and the reviewers. Any product that may be evaluated in this article or claim that may be made by its manufacturer is not guaranteed or endorsed by the publisher.
Supplementary Material
Research integrity at Frontiers
Learn more about the work of our research integrity team to safeguard the quality of each article we publish.