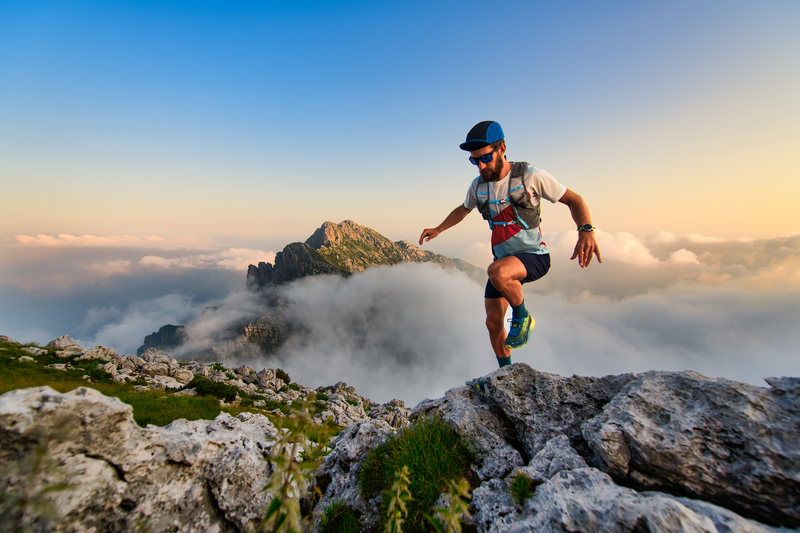
94% of researchers rate our articles as excellent or good
Learn more about the work of our research integrity team to safeguard the quality of each article we publish.
Find out more
MINI REVIEW article
Front. Oncol. , 18 February 2025
Sec. Cancer Imaging and Image-directed Interventions
Volume 15 - 2025 | https://doi.org/10.3389/fonc.2025.1531781
Purpose: This review assesses the diagnostic performance of MRI-based convolutional neural networks for identifying and grading soft tissue sarcomas, evaluating therapy responses, and assessing the risk for metastases and recurrences.
Methods: Electronic databases, specifically PubMed/MEDLINE and Google Scholar, were diligently scoured for studies that delved into the intersection of convolutional neural networks, soft tissue sarcomas, and MRI. Three topics were included: 1) differentiating and grading soft tissue sarcomas, 2) assessing therapy response, and 3) predicting metastases and recurrences.
Results: This review included 12 articles. Seven articles investigated the differentiation and grading of soft tissue sarcomas. Sensitivity for that issue ranged from 0.85 to 0.95, specificity from 0,33 to 1, and the area under the curve (AUC) from 0.74 to 0.96. Three articles investigated therapy responses, and two discussed metastasis and recurrence prediction. Only one article out of the five articles above presented accurate diagnostic values. That article examined the prediction of lung metastases and demonstrated a sensitivity of 0.47, a specificity of 0.97, and an AUC of 0.83.
Conclusion: AI applications using CNNs demonstrated robust capabilities in differentiating and grading soft tissue sarcomas using MRI. However, studies on therapy response and prediction of metastases and recurrences are still lacking.
Soft tissue sarcomas account for 1-2% of the overall incidence of adult cancer in Europe (1, 2). The annual incidence of STS varies between 1.8 and 5.0 cases per 100,000 individuals, with a peak occurrence around the age of 60. By 2025, this incidence is projected to increase, primarily attributed to insufficient progress in the prevention, diagnosis, and treatment of these malignancies (3, 4). Moreover, increasing costs of therapy for soft tissue sarcomas are expected, partly due to new drug-based treatments (5, 6). Soft tissue sarcomas are heterogeneous mesenchymal neoplasms with more than 70 histological subtypes (7, 8). Even biopsies can lead to inaccurate results due to this heterogeneity (9). Magnetic resonance imaging (MRI) is the imaging modality of choice for evaluating soft tissue sarcomas with many histological subtypes already been classified using conventional MRI (10–13). There are several classification systems for soft tissue sarcomas. The best-known system is the French Federation Nationale des Centres de Lutte Contre le Cancer (FNCLCC), based on histologic type and subtype features, tumor necrosis, and mitotic activity. It divides soft tissue sarcomas into grades I through III (14, 15). Soft tissue sarcoma staging systems are essential in guiding prognosis and treatment allocation. Precise grading and staging systems can effectively assist with monitoring and preventing local recurrences. However, existing systems do not provide sufficient accuracy for making predictions and are limited by the anatomic stage of the tumor (16). Artificial intelligence (AI) applications using convolutional neural networks (CNNs) offer promising opportunities in many fields of soft tissue sarcoma diagnostics. Capturing and collecting relevant information about pathological changes beyond human visual perception can be a promising application of CNNs in soft tissue sarcoma diagnostics (17, 18). As a consequence, AI might assist less specialized diagnostic centers in making correct diagnoses in the near future (19, 20). AI can also dive far deeper into sophisticated diagnostic methods such as gene sequencing to successfully identify soft tissue sarcomas genetic components (21, 22). Because of its objective and descriptive characteristics, AI can analyze, refine, and quantify medical images. This allows for selecting the most valuable imaging features to analyze clinical information, make differential diagnoses of tumors, and provide accurate guidance for treatment and prognosis (15, 23–27).
In this review, we investigated the potential of CNNs in soft tissue sarcoma diagnostics. For that issue, the diagnostic performance of MRI-based CNNs for differentiating and grading soft tissue sarcomas, evaluating therapy responses and risk for metastases and recurrences were evaluated.
We performed a comprehensive literature review to identify studies assessing the diagnostic performance of convolutional neural networks in magnetic resonance imaging of soft tissue sarcomas. Therefore, PubMed/MEDLINE and Google Scholar were systematically searched using selected keywords. These selected keywords and terms included “soft tissue sarcoma”, “machine learning”, “deep learning”, “artificial intelligence”, “convolutional neural network” and “MRI”. We applied multiple combinations of these keywords with appropriate Boolean operators (OR/AND) to each online database.
All observational studies on three areas of soft tissue sarcoma diagnostics were included: 1) differentiating and grading soft tissue tumors, 2) predicting metastases and recurrences, and 3) assessing therapy response. The following studies were excluded: (1) other types of studies than observational (including case reports/series, editorials, comments, correspondence, guideline, experimental, and interventional studies, as well as meta-analyses, systematic and narrative reviews); (2) grey literature or literature produced outside of the traditional academic publishing channels; (3) articles lacking available full texts in English; (4) articles using other imaging modalities than MRI; (5) animal studies; (6) studies without values on diagnostic accuracy; (7) studies not falling under the three included topics (differentiating/grading, therapy response, prediction of metastasis/recurrence).
Eighty-one publications were initially identified. After eliminating 29 duplicate studies, 52 articles were considered for title/abstract screening. After this screening, 38 studies advanced to the full-text examination phase. Following the full-text review, 26 articles were excluded due to not falling into the inclusion criteria, leaving 12 articles that addressed the research questions, met the inclusion criteria, and were therefore included in the final study (Table 1).
Dai et al. used a ResNet 50 model to differentiate between soft tissue sarcomas and atypical lipomas, achieving an AUC of 0.96, a sensitivity of 0.85, and an accuracy of 0.87 (28). Gitto et al. applied a Random Forest (RF) model to distinguish lipomas from atypical lipomatous tumors, resulting in an AUC of 0.74, high sensitivity (0.92), but low specificity (0.33). Gitto et al. found no significant difference between the AI’s performance and a radiologist’s, with the AI exhibiting a sensitivity of 0.92 compared to the radiologist’s 0.88 and a specificity of 0.33 versus the radiologist’s 0.54 (p=0.474) (29). Malinauskaite et al. utilized a Support Vector Machine (SVM) to differentiate between lipomas and liposarcomas, with an AUC of 0.926, sensitivity of 0.88, specificity of 1.0, and an accuracy of 0.927. Malinuskaite et al. found that the best-performing AI model out of their four CNNs surpassed the results of three radiologists with varying experience (10, 5, and 2 years specializing in musculoskeletal radiology). Specifically, the AI’s AUC was 0.926 compared to the radiologists’ 0.804, with a sensitivity of 0.88 versus 0.769, specificity of 1.0 against 0.84, and accuracy of 92.7% compared to 81.6% (30). Navarro et al. and Peeken et al. focused on grading soft tissue sarcomas using DenseNet 161 and LASSO-based models, achieving AUCs of 0.75 and 0.84, respectively, with similar sensitivities (0.91 and 0.90) and specificities (0.4 and 0.5) (31, 32). Xu et al. and Yang et al. differentiated soft tissue sarcomas according to malignancy grade and predicted MDM2-Gene amplification, respectively, achieving AUCs of 0.922 and 0.95, with high sensitivity and specificity values (33, 34).
Blackledge et al. achieved an accuracy of 0.981 in response assessment using an RF model (35). Gao et al. reported an accuracy of 0.833 in assessing therapy response to radiotherapy using a VGG 19 model (36). Peeken et al. used an RF-based delta model combined with other metrics to assess neoadjuvant therapy response, with an AUC of 0.79 (37).
Two studies focused on predicting metastases and recurrences. Liang et al. used a complex DLRN model based on ResNet 34 combined with multiple other algorithms (mRMR+LASSO+SVM+SMOTE) to predict lung metastases, achieving an AUC of 0.833, with sensitivity and specificity values of 0.474 and 0.972, respectively (38). Liu, S. et al. (2021) predicted recurrences using a DLRN 2-based ResNet 34 model, achieving a high AUC of 0.96 (39).
This review investigates the potential of MRI-based CNNs for identifying and grading soft tissue sarcomas, evaluating their therapy responses, and assessing the risk for metastases and recurrences.
As soft tissue sarcomas comprise a rare and heterogeneous group of malignancies, conventional diagnostic features assessing soft tissue sarcomas themselves, therapy responses, and potential risk factors for metastases and recurrences are rare. Although soft tissue sarcomas present some characteristic findings on MRI (8, 15, 23, 40–42), these characteristics are still insufficient for an overall differentiation/grading and risk stratification of soft tissue sarcomas in imaging. Integrating AI in soft tissue sarcoma diagnostics offers a promising avenue for disease management, diagnostics, and prognosis. However, as with any pioneering methodology, the implementation of AI comes with challenges. One significant issue is the small sample size observed in most studies. Peeken et al. circumvented this by orchestrating multicentric studies to bolster patient numbers (32, 37, 43). Despite such attempts, the maximum number of patients recorded in any analyzed study did not surpass 351. Meanwhile, Gao et al. adopted a different approach, producing over 15,000 synthetic images through oversampling (36). The data imbalance poses a second critical challenge. Liu X. et al. formulated a unique SRS strategy that involved two-step data splitting to enhance the balance between the training and testing datasets (44). However, this methodology led to overlaps, thereby compromising the integrity of the “true” test numbers. CNNs remain at the heart of the issue. Often perceived as black boxes, the proper CNN selection is paramount for effective results (45–48). For instance, while ResNet layers have been found to be an application, excessive layering can usher in issues like vanishing gradients, where the learning network becomes heavily dependent on initial weights, causing a regression in learning (49). Another persistent challenge is using retrospective data, which, though readily available, brings forth issues like the inability to alter past MRI settings, susceptibility to errors, and bias (50). The absence of open metadata, coupled with variable metrics and cut-offs, obstructs the comparison of study results. Guaranteeing transparency and reproducibility necessitates detailed reports on metrics, statistical hypotheses, and specific cut-offs (51).
Despite these challenges, milestones have been achieved. Yang et al., for instance, demonstrated that MDM2 gene amplification could be gleaned from image data alone (34). While certain inaccuracies like misestimating tumor grading persist, as evidenced by Xu et al.’s 26.9% upgrade rate, harnessing ample data and prudent feature selection can deliver reliable outcomes (33).
Another critical limitation is the scalability and high cost of these solutions, with implementation costs estimated to reach up to $1 million, depending on factors such as data acquisition, infrastructure, and regulatory compliance (52, 53). High computational demands and infrastructure requirements hinder adoption, particularly in low-resource settings (19–21, 52, 53). Additionally, the environmental impact of AI training processes, which contribute to increased CO2 emissions, cannot be overlooked (54, 55).
This review revealed that most studies in soft tissue sarcoma diagnostics have focused on grading and differentiating these tumors. However, research on therapy response and risk stratification for metastases and recurrences remains limited. While the overall diagnostic performance of CNN-based applications for grading and differentiating soft tissue sarcomas is relatively high, the included studies demonstrated significant variation in specificity compared to sensitivity, with some studies reporting specificities as low as 0.33. Therefore, future CNN-based applications should aim to improve specificity while maintaining high sensitivity. Yet, with continuous refinement, AI’s potential in soft tissue sarcomas and medicine, in general, is undeniable.
The future might very well behold a time when we can ascertain the grading of soft tissue sarcomas without necessitating punctures, which could lead to quicker and more effective therapy and in total lower the cost of the treatment.
The present study has several limitations. The included studies showed heterogeneous study designs, objectives, and sample sizes, making comparisons challenging. Additionally, some of the included studies had small sample sizes, which could have affected the diagnostic performance of the AI applications included. Also, most studies did not investigate confounding factors, which might have influenced outcomes.
Applications of convolutional neural networks (CNNs) demonstrate significant potential for differentiating and grading soft tissue sarcomas using MRI. However, there remains a gap in research on evaluating therapy responses and predicting metastases and recurrences, underscoring the need for further investigation in these critical areas. This study highlights the potential of AI to enable precise, non-invasive diagnostic methods for soft tissue sarcomas, reducing the reliance on invasive procedures. In the future, AI could become a valuable tool for effective treatment planning, ultimately improving patient outcomes and optimizing healthcare resources.
HV: Conceptualization, Data curation, Formal analysis, Funding acquisition, Investigation, Methodology, Project administration, Resources, Software, Supervision, Validation, Visualization, Writing – original draft, Writing – review & editing. H-UK: Supervision, Writing – review & editing. SS: Conceptualization, Data curation, Formal analysis, Funding acquisition, Investigation, Methodology, Project administration, Resources, Software, Supervision, Validation, Visualization, Writing – original draft, Writing – review & editing.
The author(s) declare that no financial support was received for the research, authorship, and/or publication of this article.
The authors declare that the research was conducted in the absence of any commercial or financial relationships that could be construed as a potential conflict of interest.
The author(s) declare that no Generative AI was used in the creation of this manuscript.
All claims expressed in this article are solely those of the authors and do not necessarily represent those of their affiliated organizations, or those of the publisher, the editors and the reviewers. Any product that may be evaluated in this article, or claim that may be made by its manufacturer, is not guaranteed or endorsed by the publisher.
1. Gatta G, van der Zwan JM, Casali PG, Siesling S, Dei Tos AP, Kunkler I, et al. Rare cancers are not so rare: the rare cancer burden in Europe. Eur J cancer. (2011) 47:2493–511. doi: 10.1016/j.ejca.2011.08.008
2. Guerrero WM, Deneve JL. Local recurrence of extremity soft tissue sarcoma. Surg Clin North Am. (2016) 96:1157–74. doi: 10.1016/j.suc.2016.05.002
3. Wibmer C, Leithner A, Zielonke N, Sperl M, Windhager R. Increasing incidence rates of soft tissue sarcomas? A population-based epidemiologic study and literature review. Ann Oncol. (2010) 21:1106–11. doi: 10.1093/annonc/mdp415
4. Pizzato M, Collatuzzo G, Santucci C, Malvezzi M, Boffetta P, Comandone A, et al. Mortality patterns of soft-tissue sarcomas worldwide up to 2018, with predictions for 2025. Eur J Cancer Prev. (2023) 32:71–80. doi: 10.1097/CEJ.0000000000000768
5. Ludwig W-D, Schildmann J. Kostenexplosion in der medikamentösen Therapie onkologischer Erkrankungen. Der Onkologe. (2015) 21:708–16. doi: 10.1007/s00761-015-2958-5
6. Qu XM, Louie AV, Ashman J, Wasif N. Cost-effectiveness analysis of preoperative versus postoperative radiation therapy in extremity soft tissue sarcoma. Int J Radiat Oncol Biol Phys. (2017) 97:339–46. doi: 10.1016/j.ijrobp.2016.10.009
7. Hui JY. Epidemiology and etiology of sarcomas. Surg Clin North Am. (2016) 96:901–14. doi: 10.1016/j.suc.2016.05.005
8. Sedaghat S, Schmitz F, Meschede J, Sedaghat M. Systematic analysis of post-treatment soft-tissue edema and seroma on MRI in 177 sarcoma patients. Surg Oncol. (2020) 35:218–23. doi: 10.1016/j.suronc.2020.08.023
9. Coindre JM. Grading of soft tissue sarcomas: review and update. Arch Pathol Lab Med. (2006) 130:1448–53. doi: 10.5858/2006-130-1448-GOSTSR
10. Schmitz F, Voigtländer H, Jang H, Schlemmer HP, Kauczor HU, Sedaghat S. Predicting the Malignancy grade of soft tissue sarcomas on MRI using conventional image reading and radiomics. Diagnostics (Basel). (2024) 14(19):2220. doi: 10.3390/diagnostics14192220
11. Sedaghat S, Sedaghat M, Krohn S, Jansen O, Freund K, Streitbürger A, et al. Long-term diagnostic value of MRI in detecting recurrent aggressive fibromatosis at two multidisciplinary sarcoma centers. Eur J radiology. (2021) 134:109406. doi: 10.1016/j.ejrad.2020.109406
12. Sedaghat S, Sedaghat M, Meschede J, Jansen O, Both M. Diagnostic value of MRI for detecting recurrent soft-tissue sarcoma in a long-term analysis at a multidisciplinary sarcoma center. BMC Cancer. (2021) 21:398. doi: 10.1186/s12885-021-08113-y
13. Sedaghat S, Schmitz F, Krieger A, Sedaghat M, Reichardt B. Appearance of recurrent adult fibrosarcoma of the soft tissue and loco-regional post-treatment changes on MRI follow-up. Eur J Plast Surgery. (2021) 44:97–102. doi: 10.1007/s00238-020-01669-1
14. Lin X, Davion S, Bertsch EC, Omar I, Nayar R, Laskin WB. Federation Nationale des Centers de Lutte Contre le Cancer grading of soft tissue sarcomas on needle core biopsies using surrogate markers. Hum Pathol. (2016) 56:147–54. doi: 10.1016/j.humpath.2016.06.008
15. Sedaghat S, Salehi Ravesh M, Sedaghat M, Both M, Jansen O. Configuration of soft-tissue sarcoma on MRI correlates with grade of Malignancy. Radiol Oncol. (2021) 55:158–63. doi: 10.2478/raon-2021-0007
16. Callegaro D, Miceli R, Mariani L, Raut CP, Gronchi A. Soft tissue sarcoma nomograms and their incorporation into practice. Cancer. (2017) 123:2802–20. doi: 10.1002/cncr.v123.15
17. Geirhos R, Rubisch P, Michaelis C, Bethge M, Wichmann FA, Brendel W. ImageNet-trained CNNs are biased towards texture; increasing shape bias improves accuracy and robustness. arXiv. (2018) arXiv:1811.12231. doi: 10.48550/arXiv.1811.12231
18. Crombé A, Roulleau-Dugage M, Italiano A. The diagnosis, classification, and treatment of sarcoma in this era of artificial intelligence and immunotherapy. Cancer Commun (Lond). (2022) 42:1288–313. doi: 10.1002/cac2.v42.12
19. Foersch S, Eckstein M, Wagner DC, Gach F, Woerl AC, Geiger J, et al. Deep learning for diagnosis and survival prediction in soft tissue sarcoma. Ann Oncol. (2021) 32:1178–87. doi: 10.1016/j.annonc.2021.06.007
20. Rosenberg A, Kochanny S, Dolezal J, Wang C, Chen H, Kather JN, et al. Prediction of histologic and molecular subsets of soft tissue sarcoma using deep learning. Am Soc Clin Oncol. (2020) 14:1–14. doi: 10.1200/JCO.2020.38.15_suppl.e23529
21. Qi L, Chen F, Wang L, Yang Z, Zhang W, Li Z. Deciphering the role of NETosis-related signatures in the prognosis and immunotherapy of soft-tissue sarcoma using machine learning. Front Pharmacol. (2023) 14:1217488. doi: 10.3389/fphar.2023.1217488
22. Qi L, Chen F, Wang L, Yang Z, Zhang W, Li Z. Integration analysis of senescence-related genes to predict prognosis and immunotherapy response in soft-tissue sarcoma: evidence based on machine learning and experiments. Front Pharmacol. (2023) 14. doi: 10.3389/fphar.2023.1229233
23. Sedaghat S, Schmitz F, Grözinger M, Sedaghat M. Malignant peripheral nerve sheath tumours in magnetic resonance imaging: primary and recurrent tumour appearance, post-treatment changes, and metastases. Pol J Radiol. (2020) 85:e196–201. doi: 10.5114/pjr.2020.94687
24. Boudabbous S, Hamard M, Saiji E, Gorican K, Poletti PA, Becker M, et al. What morphological MRI features enable differentiation of low-grade from high-grade soft tissue sarcoma? BJR Open. (2022) 4:20210081. doi: 10.1259/bjro.20210081
25. Crombé A, Marcellin PJ, Buy X, Stoeckle E, Brouste V, Italiano A, et al. Soft-tissue sarcomas: assessment of MRI features correlating with histologic grade and patient outcome. Radiology. (2019) 291:710–21. doi: 10.1148/radiol.2019181659
26. Chhabra A, Ashikyan O, Slepicka C, Dettori N, Hwang H, Callan A, et al. Conventional MR and diffusion-weighted imaging of musculoskeletal soft tissue Malignancy: correlation with histologic grading. Eur radiology. (2019) 29:4485–94. doi: 10.1007/s00330-018-5845-9
27. Chianca V, Albano D, Messina C, Vincenzo G, Rizzo S, Del Grande F, et al. An update in musculoskeletal tumors: from quantitative imaging to radiomics. Radiol Med. (2021) 126:1095–105. doi: 10.1007/s11547-021-01368-2
28. Dai M, Liu Y, Hu Y, Li G, Zhang J, Xiao Z, et al. Combining multiparametric MRI features-based transfer learning and clinical parameters: application of machine learning for the differentiation of uterine sarcomas from atypical leiomyomas. Eur radiology. (2022) 32:7988–97. doi: 10.1007/s00330-022-08783-7
29. Gitto S, Interlenghi M, Cuocolo R, Salvatore C, Giannetta V, Badalyan J, et al. MRI radiomics-based machine learning for classification of deep-seated lipoma and atypical lipomatous tumor of the extremities. Radiol Med. (2023) 128:989–98. doi: 10.1007/s11547-023-01657-y
30. Malinauskaite I, Hofmeister J, Burgermeister S, Neroladaki A, Hamard M, Montet X, et al. Radiomics and machine learning differentiate soft-tissue lipoma and liposarcoma better than musculoskeletal radiologists. Sarcoma. (2020) 2020:7163453. doi: 10.1155/2020/7163453
31. Navarro F, Dapper H, Asadpour R, Knebel C, Spraker MB, Schwarze V, et al. Development and external validation of deep-learning-based tumor grading models in soft-tissue sarcoma patients using MR imaging. Cancers. (2021) 13:2866. doi: 10.3390/cancers13122866
32. Peeken JC, Spraker MB, Knebel C, Dapper H, Pfeiffer D, Devecka M, et al. Tumor grading of soft tissue sarcomas using MRI-based radiomics. EBioMedicine. (2019) 48:332–40. doi: 10.1016/j.ebiom.2019.08.059
33. Xu W, Hao D, Hou F, Zhang D, Wang H. Soft tissue sarcoma: preoperative MRI-based radiomics and machine learning may be accurate predictors of histopathologic grade. AJR Am J roentgenology. (2020) 215:963–9. doi: 10.2214/AJR.19.22147
34. Yang Y, Zhou Y, Zhou C, Ma X. Novel computer aided diagnostic models on multimodality medical images to differentiate well differentiated liposarcomas from lipomas approached by deep learning methods. Orphanet J Rare Dis. (2022) 17:158. doi: 10.1186/s13023-022-02304-x
35. Blackledge MD, Winfield JM, Miah A, Strauss D, Thway K, Morgan VA, et al. Supervised machine-learning enables segmentation and evaluation of heterogeneous post-treatment changes in multi-parametric MRI of soft-tissue sarcoma. Front Oncol. (2019) 9:941. doi: 10.3389/fonc.2019.00941
36. Gao Y, Ghodrati V, Kalbasi A, Fu J, Ruan D, Cao M, et al. Prediction of soft tissue sarcoma response to radiotherapy using longitudinal diffusion MRI and a deep neural network with generative adversarial network-based data augmentation. Med physics. (2021) 48:3262–372. doi: 10.1002/mp.14897
37. Peeken JC, Asadpour R, Specht K, Chen EY, Klymenko O, Akinkuoroye V, et al. MRI-based delta-radiomics predicts pathologic complete response in high-grade soft-tissue sarcoma patients treated with neoadjuvant therapy. Radiother Oncol. (2021) 164:73–82. doi: 10.1016/j.radonc.2021.08.023
38. Liang H-Y, Yang S-F, Zou H-M, Hou F, Duan L-S, Huang C-C, et al. Deep learning radiomics nomogram to predict lung metastasis in soft-tissue sarcoma: a multi-center study. Front Oncol. (2022) 12:897676. doi: 10.3389/fonc.2022.897676
39. Liu S, Sun W, Yang S, Duan L, Huang C, Xu J, et al. Deep learning radiomic nomogram to predict recurrence in soft tissue sarcoma: a multi-institutional study. Eur radiology. (2022) 32:793–805. doi: 10.1007/s00330-021-08221-0
40. Sedaghat S, Salehi Ravesh M, Sedaghat M, Meschede J, Jansen O, Both M. Does the primary soft-tissue sarcoma configuration predict configuration of recurrent tumors on magnetic resonance imaging? Acta Radiol. (2022) 63:642–51. doi: 10.1177/02841851211008381
41. Sedaghat S, Schmitz F, Sedaghat M, Nicolas V. Appearance of recurrent dermatofibrosarcoma protuberans in postoperative MRI follow-up. J Plast Reconstr Aesthet Surg. (2020) 73:1960–5. doi: 10.1016/j.bjps.2020.08.089
42. Sedaghat S, Surov A, Krohn S, Sedaghat M, Reichardt B, Nicolas V. Configuration of primary and recurrent aggressive fibromatosis on contrast-enhanced MRI with an evaluation of potential risk factors for recurrences in MRI follow-up. Rofo. (2020) 192:448–57. doi: 10.1055/a-1022-4546
43. Peeken JC, Goldberg T, Knie C, Komboz B, Bernhofer M, Pasa F, et al. Treatment-related features improve machine learning prediction of prognosis in soft tissue sarcoma patients. Strahlentherapie und Onkologie. (2018) 94(9):824–34. doi: 10.1007/s00066-018-1294-2
44. Liu X, Guo L, Wang H, Guo J, Yang S, Duan L. Research on imbalance machine learning methods for MR $$ T_1 $$ T 1 WI soft tissue sarcoma data. BMC Med Imaging. (2022) 22:1–13. doi: 10.1186/s12880-022-00876-5
45. Ghassemi M, Oakden-Rayner L, Beam AL. The false hope of current approaches to explainable artificial intelligence in health care. Lancet Digital Health. (2021) 3:e745–e50. doi: 10.1016/S2589-7500(21)00208-9
46. Tölle M, Garthe P, Scherer C, Seliger JM, Leha A, Krüger N, et al. Federated foundation model for cardiac CT imaging. arXiv. (2024) arXiv:2407.07557. doi: 10.48550/arXiv.2407.07557
47. Gurnee W, Tegmark M. Language models represent space and time. arXiv. (2023) arXiv:2310.02207. doi: 10.48550/arXiv.2310.02207
48. Moor M, Banerjee O, Abad ZSH, Krumholz HM, Leskovec J, Topol EJ, et al. Foundation models for generalist medical artificial intelligence. Nature. (2023) 616:259–65. doi: 10.1038/s41586-023-05881-4
51. Pfaehler E, Zhovannik I, Wei L, Boellaard R, Dekker A, Monshouwer R, et al. A systematic review and quality of reporting checklist for repeatability and reproducibility of radiomic features. Phys Imaging Radiat Oncol. (2021) 20:69–75. doi: 10.1016/j.phro.2021.10.007
52. Walsh AP, Hamill S, Morrison I. Health information technology. In: Jonas and kovner’s health care delivery in the United States. New York: Springer. vol. 359. (2023).
53. Castonguay A, Wagner G, Motulsky A, Paré G. AI maturity in health care: An overview of 10 OECD countries. Health Policy. (2024) 140:104938. doi: 10.1016/j.healthpol.2023.104938
54. Liu V, Yin Y. Green AI: exploring carbon footprints, mitigation strategies, and trade offs in large language model training. arXiv. (2024) 4:49. doi: 10.1007/s44163-024-00149-w
Keywords: artificial intelligence, soft tissue sarcomas, MRI, CNN, metastasis, recurrence, grade, therapy
Citation: Voigtländer H, Kauczor H-U and Sedaghat S (2025) Diagnostic utility of MRI-based convolutional neural networks in soft tissue sarcomas: a mini-review. Front. Oncol. 15:1531781. doi: 10.3389/fonc.2025.1531781
Received: 20 November 2024; Accepted: 31 January 2025;
Published: 18 February 2025.
Edited by:
Ellen Ackerstaff, University of Texas MD Anderson Cancer Center, United StatesReviewed by:
Vikas Mittal, Chandigarh University, IndiaCopyright © 2025 Voigtländer, Kauczor and Sedaghat. This is an open-access article distributed under the terms of the Creative Commons Attribution License (CC BY). The use, distribution or reproduction in other forums is permitted, provided the original author(s) and the copyright owner(s) are credited and that the original publication in this journal is cited, in accordance with accepted academic practice. No use, distribution or reproduction is permitted which does not comply with these terms.
*Correspondence: Sam Sedaghat, c2Ftc2VkYWdoYXQxQGdtYWlsLmNvbQ==
Disclaimer: All claims expressed in this article are solely those of the authors and do not necessarily represent those of their affiliated organizations, or those of the publisher, the editors and the reviewers. Any product that may be evaluated in this article or claim that may be made by its manufacturer is not guaranteed or endorsed by the publisher.
Research integrity at Frontiers
Learn more about the work of our research integrity team to safeguard the quality of each article we publish.