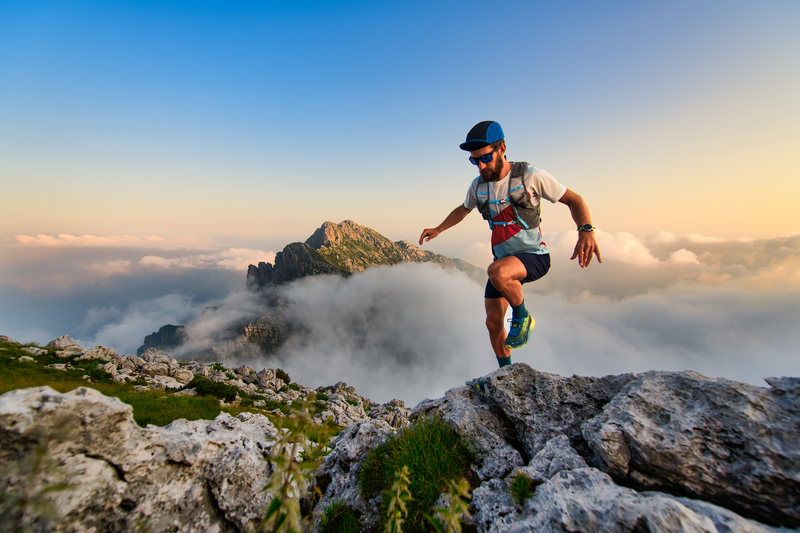
94% of researchers rate our articles as excellent or good
Learn more about the work of our research integrity team to safeguard the quality of each article we publish.
Find out more
ORIGINAL RESEARCH article
Front. Oncol. , 29 January 2025
Sec. Cancer Immunity and Immunotherapy
Volume 15 - 2025 | https://doi.org/10.3389/fonc.2025.1525414
This article is part of the Research Topic Harnessing Big Data for Precision Medicine: Revolutionizing Diagnosis and Treatment Strategies View all 22 articles
Background: Cervical lymph node metastasis (LNM) is a significant factor that leads to a poor prognosis in laryngeal cancer. Early-stage supraglottic laryngeal cancer (SGLC) is prone to LNM. However, research on risk factors for predicting cervical LNM in early-stage SGLC is limited. This study seeks to create and validate a predictive model through the application of machine learning (ML) algorithms.
Methods: The training set and internal validation set data were extracted from the Surveillance, Epidemiology, and End Results (SEER) database. Data from 78 early-stage SGLC patients were collected from Fujian Provincial Hospital for independent external validation. We identified four variables associated with cervical LNM and developed six ML models based on these variables to predict LNM in early-stage SGLC patients.
Results: In the two cohorts, 167 (47.44%) and 26 (33.33%) patients experienced LNM, respectively. Age, T stage, grade, and tumor size were identified as independent predictors of LNM. All six ML models performed well, and in both internal and independent external validations, the eXtreme Gradient Boosting (XGB) model outperformed the other models, with AUC values of 0.87 and 0.80, respectively. The decision curve analysis demonstrated that the ML models have excellent clinical applicability.
Conclusions: Our study indicates that combining ML algorithms with clinical data can effectively predict LNM in patients diagnosed with early-stage SGLC. This is the first study to apply ML models in predicting LNM in early-stage SGLC patients.
Laryngeal cancer (LC) is a malignant tumor with a relatively high incidence rate in the head and neck area, with annually increasing incidence and mortality rates (1). LC is classified into three types based on location. Among them, supraglottic laryngeal cancer (SGLC) is progresses rapidly and presents with subtle early symptoms. Early-stage LC is defined as T1 and T2 stages without distant metastasis, accounting for 66.8%-67.9% of all diagnosed cases (2). Early-stage SGLC is particularly prone to local spread, cervical lymph node metastasis (LNM), and resistance to chemotherapy, all of which contribute to a poor prognosis (3). Previous studies have shown that despite the common use of multiple treatment approaches, the overall prognosis for SGLC patients remains poor, with a 5-year survival rate of only 50% to 60% (4).
LNM is a key factor affecting treatment outcomes and prognosis in LC patients (5). Clinically, lymph nodes are evaluated through neck palpation, ultrasound, CT, or MRI (6). Despite the availability of various diagnostic methods, their sensitivity and specificity are subject to limitations (7). In addition, the clinical diagnosis of LNM may lead to false positives or false negatives, making it even more challenging to predict future developments (8). In recent years, various factors influencing the risk of LNM in LC have been reported, and corresponding prediction models have been developed (9, 10). However, the predictive performance of the models varies significantly. Therefore, there is an urgent need for a reliable and accurate predictive method to determine the preoperative status of cervical lymph nodes in SGLC patients, to guide personalized treatment selection and planning.
Machine learning (ML) is a critical branch of AI. In recent years, ML has advanced rapidly due to progress in computing, digital information, and electronic technologies (11). ML primarily focuses on identifying patterns within datasets to perform classification and prediction, thereby enabling more accurate predictions across various unrelated datasets. Consequently, ML algorithms have been extensively utilized in creating models for disease prediction (12, 13). However, there is currently no relevant research on using ML algorithm to predict LNM in patients with early-stage SGLC. In this study, we aim to find the risk factors associated with LNM in patients with SGLC and develop several ML-based models using the Surveillance, Epidemiology, and End Results (SEER) public data to screen high-risk patients for LNM.
The SEER database gathers cancer patient data representing approximately 34% of the U.S. population and spans multiple large healthcare institutions, offering high representativeness and diversity. After obtaining approval and authorization from SEER, this study collected data on patients diagnosed with early-stage SGLC from the “Incidence-SEER 12 Regs Research Data, Nov 2023 Sub (2000-2021).” First, we perform denoising on the raw data, removing any missing or outlier values. The inclusion criteria were patients diagnosed with SGLC between 2010 and 2015 as recorded in the SEER database. The exclusion criteria included: (1) tumor size unknown, (2) time from diagnosis to treatment unknown, (3) grade unknown, (4) patients with a history of other malignant tumors or those with LNM caused by other tumors. In the end, a total of 352 eligible patients were included for further analysis. Additionally, data from 78 SGLC patients who received treatment at Fujian Provincial Hospital between 2012 and 2023 were used as an independent external validation set. Furthermore, in this study, the confirmation of LNM in all patients was made through pathological examination. The process of data screening and analysis is shown in Figure 1.
In this study, clinicians used SEER Stat software (version 8.4.3) to identify eight demographic and clinicopathological variables that could impact LNM in patients with SGLC. The variables selected include sex, age at diagnosis, race, tumor count, T-stage, grade, tumor size, and time from diagnosis to treatment. And categorized based on the impact on patient prognosis and treatment options (14–16). Patients were divided into male and female groups based on sex; into two age categories at diagnosis: <65 years and ≥65 years; into racial groups: White, Black, and Other; into T1 and T2 stages according to T-stage; into tumor grades I, II, III, and IV; into single tumor and multiple tumors groups based on tumor count; into groups of ≤1 cm and >1 cm based on tumor size; and into ≤1 month and >1 month groups based on the time from diagnosis to treatment.
In this study, we developed six ML models using Python (version 3.10) to predict LNM in early-stage SGLC patients. The six models used in this study are logistic regression (LR), random forest (RF), support vector machine (SVM), k-nearest neighbor (KNN), extreme gradient boosting (XGB), and decision tree (DT). To improve the models’ generalization ability and stability, we randomly split the SEER dataset in an 8:2 ratio, using 80% of the data for training the ML algorithms and the remaining 20% for testing.Before building the ML models, we preprocess the data using One-Hot encoding (17). During training, cross-validation was performed for each model to maintain stability, A grid search method was used to automatically find the optimal hyperparameter configuration. We built the model and selected key hyperparameters to tune based on prior experience with the model and literature review. Initially, a coarse grid search was performed over a wide range to simultaneously test multiple hyperparameter combinations, and the best hyperparameter range was determined based on the model’s feedback. Then, a fine grid search was conducted to exhaustively test all possible hyperparameter combinations within the identified range, ultimately determining the model’s hyperparameter settings in preparation for subsequent model training and testing. Finally, data from patients at Fujian Provincial Hospital were used as an independent external validation.
In this study, true positive, true negative, false positive, and false negative values were utilized to derive key metrics, including the area under the receiver operating characteristic (ROC) curve (AUC), accuracy, precision, F1-score, recall, and specificity, to comprehensively assess the predictive performance of each ML model. Additionally, we examined the clinical applicability of the models using Calibration curves.
In this study, all statistical analyses were conducted using SPSS software (version 24.0, IBM) and Python (version 3.10). Descriptive statistics for categorical variables were compared using the Chi-square test or Fisher’s exact test. Univariate and multivariate logistic regression analyses were performed to identify independent risk factors for LNM in SGLC patients. Pearson correlation analysis was used to assess the relationships between variables potentially influencing LNM, and the results were visualized as a heatmap. The findings were presented as odds ratios (ORs).
This study included a total of 430 early-stage SGLC patients and evaluated eight variables. Among them, 219 patients (50.93%) did not experience LNM, while 211 patients (49.06%) did. Due to geographic and racial differences, as well as sample size limitations, significant differences were found in the variables between SGLC patients from the SEER database and those at Fujian Provincial Hospital, with the exception of the T stage (Table 1). In SGLC patients from the SEER database, no significant differences were observed between metastatic and non-metastatic patients in terms of race, gender, or the time from diagnosis to treatment.; however, other variables showed significant differences. In the independent external validation SGLC patients from Fujian Provincial Hospital, significant differences in T stage and tumor size were observed between patients with LNM and those without, while the distributions of other variables showed no significant differences (Table 2). Pearson correlation analysis of all variables indicated weak correlations and strong independence between the variables (Figure 2).
Figure 2. The results of the Pearson correlation analysis between all the variables. These variables were independent of each other with no significant correlation and no collinearity.
Univariate logistic regression analysis identified five risk factors related to LNM: age, T-stage, grade, tumor count, and tumor size. Later, multivariate logistic regression analysis showed statistically significant differences in age, T-stage, grade, and tumor size. Specifically, age (≥65 years) acted as protective factors for LNM, whereas T-stage (T2), tumor grade (III, IV), and tumor size (>1 cm) were risk factors for LNM (Table 3).
Table 3. Univariable and multivariable logistic regression analyses of risk factors for LNM in patients.
LNM status was considered as the outcome indicator. Four factors with P < 0.05 in the multivariate logistic regression analysis were used as variables for training the model. Six ML models, including DT, KNN, RF, SVM, LR, and XGB, were applied to the training set to develop predictive models. Cross-validation was performed for internal validation to assess the performance of each model. Figure 3 shows that among the six ML algorithms used in both internal and external validation, the XGB model performed strongly in ROC curve analysis. Table 4 also shows that the XGB model performs well across all evaluation metrics. Therefore, we selected the XGB model as the final model to predict LNM in SGLC patients. Figure 4 compares the predicted probabilities of the models with the actual frequencies of occurrence, highlighting the reliability of the model predictions. The predicted probabilities of our six ML models align well with the actual outcomes, indicating that the models are well-calibrated.
Figure 3. Receiver operating characteristic curves of six ML algorithms predicting early-stage SGLC patients with LNM in the validation set (A) internal validation. (B) External validation.
Figure 4. Calibration Curve of six ML algorithms predicting early-stage SGLC patients with LNM in the validation set. (A) internal validation. (B) External validation.
Figure 5 illustrates the importance of each variable in predicting early-stage SGLC LNM across the six ML algorithms. Although the importance of variables varies slightly among these ML algorithms, it is evident that T stage is the most important predictor in multiple models. Tumor grade and age also play significant roles in all models. In the XGB model, the variables are ranked in descending order of importance as follows: T stage, Grade, tumor size, age.
Figure 5. The ranking of feature importance in the six ML algorithms used to predict lymph node metastasis. (A) DT. (B) SVM. (C) XGB. (D) RF. (E) LR. (F) KNN.
LNM is a crucial indicator of distant metastasis in SGLC (18). Due to the extensive submucosal lymphatic network in the neck, SCLC is prone to cervical LNM (19). Research has shown that early-stage (pT1/2) SCLC has an LNM rate of up to 55% (18). Nearly 40% of cN0 SCLC patients develop occult cervical LNM (20). It is generally believed that when the risk of occult cervical LNM exceeds 15%, elective neck dissection should be considered (21). While prophylactic elective neck dissection can effectively reduce the risk of LNM, it also introduces additional surgical risks for patients with SCLC, such as postoperative bleeding, nerve injury, and lymphatic leakage, which can adversely affect recovery, quality of life, and even pose life-threatening risks (22–24). At present, LNM diagnosis mainly depends on cervical palpation and preoperative imaging, both of which are greatly influenced by the clinician’s expertise (25, 26). However, cervical palpation has low sensitivity and specificity, and for patients with malignant tumors, imaging tests are often necessary, despite their high cost, and are generally considered acceptable in clinical practice. However, imaging tests are limited in predicting the future risk of LNM (27). Therefore, an efficient and accurate diagnostic method is crucial. A model was developed using advanced ML algorithms to identify early-stage SGLC patients at high risk of LNM.
In this study, we applied six ML models to predict LNM in early-stage SGLC patients and identified several key findings. First, since multivariate logistic regression can simultaneously account for multiple variables, it allows for controlling confounding factors and assessing the independent effects of each variable (28, 29). By selecting variables with p-values less than 0.05 in the multivariate logistic regression analysis, we identified four independent risk factors associated with LNM: grade, age, T stage, and tumor size. Second, all six ML models were capable of predicting LNM. Finally, the XGB model demonstrated the best predictive performance in both the internal validation set and the independent external validation set from Fujian Provincial Hospital.
In recent years, many researchers have developed multiple predictive models to predict LNM in laryngeal cancer (9, 10, 19, 30). However, due to factors such as data quality, feature selection, and data diversity, the performance of these predictive models varies. Pan, Y et al. developed a nomogram to predict preoperative LNM, with an AUC value of 0.721 (10). Song, L et al. used a nomogram to predict the risk of LNM in supraglottic laryngeal squamous cell carcinoma, with an AUC value of 0.707 (19). To more accurately predict LNM in SGLC patients, we established prediction models based on six different ML algorithms for the first time. The performance of the ML models was evaluated and compared using accuracy, precision, recall, F1 score, AUC value, specificity, and calibration curves. The comprehensive evaluation of these metrics helps to provide a full understanding of the model’s performance, ensuring balanced performance across different aspects. AUC is a highly comprehensive metric, especially suitable for imbalanced datasets, as it assesses the overall performance of the model across various classification thresholds (31, 32). Therefore, we selected AUC as the primary evaluation criterion. Our results showed that XGB outperformed the other models in terms of AUC value and F1 score, both in the training set and the test set. Additionally, the AUC value of XGB was also higher than that of the models developed in previous studies.
In recent years, many clinical and pathological factors associated with LNM in early-stage SCLC have been studied (18, 33). Our study confirmed that age is an important variable in the model. Tachibana, T et al. suggested that relatively young patients with SGLC are more likely to show neck metastasis (33). Consistent with previous studies, this study found that patients with supraglottic laryngeal cancer (SCLC) under the age of 65 have a higher risk of LNM. This may be associated with the more active metabolic processes in patients under the age of 65, which can facilitate the metastasis of tumor cells to lymph nodes (34). Additionally, younger patients may adopt less healthy lifestyle habits, poor dietary choices, and harmful environmental exposures, thereby increasing the risk of cancer development and metastasis (35). Finally, compared to older patients, younger individuals may not adequately prioritize early symptoms, resulting in a more advanced stage of the tumor at diagnosis, which heightens the likelihood of LNM (36).
Grade is another key indicator. A large number of studies have shown that poorly differentiated tumors are associated with a higher frequency of cervical metastasis, and tumor differentiation is a potential predictive factor for occult cervical LNM (37, 38). The pathological grade of SGLC reflects the degree of differentiation and malignancy of tumor cells. In undifferentiated laryngeal cancer, tumor cells exhibit an immature morphology, with low differentiation, and their structure and function resemble those of primitive, immature cells (39). This leads to rapid proliferation and a higher likelihood of breaching the basement membrane, entering blood vessels and lymphatic vessels (40–42). In this way, cancer cells can spread through the lymphatic system, increasing the risk of LNM. In contrast, well differentiated tumor cells typically grow more slowly, are better differentiated and more stable, resulting in a relatively lower likelihood of LNM (43). Additionally, undifferentiated laryngeal cancer exhibits significant cellular heterogeneity, meaning that cells in different regions of the tumor may show varied growth characteristics, with some cells being more invasive and having a higher potential for metastasis (44). For these reasons, undifferentiated laryngeal cancer is more difficult to control locally, has a higher postoperative recurrence rate, and thus requires more aggressive treatment and close follow-up to prevent LNM.
Tumor size was also an important predictor. Song, L et al. constructed a nomogram based on tumor size, tumor differentiation, and LMR (lymphocyte-to-monocyte ratio), which demonstrated good predictive ability (19). Another study similarly indicated that tumor size is associated with the rate of cervical lymph node (45, 46). As tumors increase in size, their likelihood of spreading to surrounding tissues increases. Larger tumors are more prone to invading adjacent structures, including lymphatic vessels, which subsequently heightens the probability of cancer cells disseminating through the lymphatic system (47, 48). This relationship is supported by our research findings. Moreover, larger tumor size generally corresponds to a higher number of cancer cells, thereby increasing the chances of these cells infiltrating the lymphatic system and reaching the lymph nodes (49, 50). Tumor growth requires a substantial supply of blood and nutrients, which in turn stimulates angiogenesis and lymph angiogenesis. As tumors increase in size, they tend to form more new blood vessels and lymphatic vessels, providing additional pathways for cancer cells to enter the lymphatic system and consequently elevating the risk of LNM (51, 52).
T-stage is also one of the metrics in ML models. As the T-stage of a tumor increases, the likelihood of cervical LNM also increases (53). Tumors with a higher T-stage are more prone to invade surrounding tissues, potentially disrupting the normal lymphatic structure, thereby allowing tumor cells easier access to the lymphatic system and subsequent LNM (54). Additionally, higher T-stage tumors are often associated with more extensive local spread, further increasing the risk of lymph node involvement. In SGLC, lymphatic drainage primarily involves the cervical lymph nodes, with the lymphatic flow decreasing from the superior to the inferior regions (18, 55). The lymphatic network density is higher in the epiglottis and aryepiglottic folds compared to the laryngeal ventricle and false vocal cords. Tumors with a higher T-stage are more likely to metastasize to these lymph node groups via lymphatic dissemination. When the tumor invades the laryngeal ventricle and Para glottic space, laryngoscopic examination may still show a normal false vocal cord and vocal cord mucosa, with only slight surface elevation, and patients may present with minimal clinical symptoms (56). Most patients present at an advanced stage, with a low survival rate. Thus, these patients may require a combination of surgical resection, radiation therapy, and chemotherapy to address local invasiveness and LNM, to ensure a personalized treatment strategy.
As far as we know, this is the first study to apply ML models in predicting LNM in early-stage SGLC patients, and it offers a valuable tool for assessing individual LNM risk. This approach could help tailor treatment strategies based on the specific risk of LNM, potentially improving treatment outcomes while minimizing unnecessary side effects. However, there are several limitations in our study. First, this study is the small sample size from Fujian Provincial Hospital, which may affect the broader applicability and statistical power of the results. Additionally, the small sample size may limit the analytical precision of certain variables. Future research should involve a larger sample size to further validate the findings’ reliability. Second, the SEER database lacks comprehensive patient information, such as lifestyle factors, genetic data, and detailed socioeconomic status. In addition, the differences in data sources may lead to variations in sample characteristics, which could affect the performance of machine learning models on external datasets. Although we have made efforts to ensure the model’s transferability through cross-validation and multiple evaluation metrics, such differences remain a potential limitation. Finally, the study does not include biochemical markers for patients. Although this avoids the variability in testing levels across institutions, incorporating such data would enhance the predictive power of the model.
In our study, we introduced six ML-based predictive models and discovered that the XGB algorithm could be the most effective model for predicting LNM in early-stage SGLC patients. Four independent risk factors for LNM were identified through multifactorial logistic regression, including grade, T-stage, tumor size, and age. To investigate the reliability of the ML models, we also collected patient information from Fujian Provincial Hospital for independent external validation, in addition to patients from the SEER database. The calibration curve indicated that our tool performs well in clinical applications.
The raw data supporting the conclusions of this article will be made available by the authors, without undue reservation.
The studies involving humans were approved by Fujian Provincial Hospital ethics committee. The studies were conducted in accordance with the local legislation and institutional requirements. The participants provided their written informed consent to participate in this study.
HW: Writing – original draft. ZH: Data curation, Writing – original draft. JX: Visualization, Writing – original draft. TC: Methodology, Resources, Supervision, Writing – review & editing. JH: Investigation, Writing – original draft. LC: Investigation, Writing – original draft. XY: Investigation, Writing – original draft.
The author(s) declare financial support was received for the research, authorship, and/or publication of this article. This work was supported by Major scientific research projects for young and middle-aged people in Fujian Province (Grant no. 2022ZQNZD001). This study was also supported by the National Natural Science Foundation of China (Grant No. 81970899).
The authors declare that the research was conducted in the absence of any commercial or financial relationships that could be construed as a potential conflict of interest.
The author(s) declare that no Generative AI was used in the creation of this manuscript.
All claims expressed in this article are solely those of the authors and do not necessarily represent those of their affiliated organizations, or those of the publisher, the editors and the reviewers. Any product that may be evaluated in this article, or claim that may be made by its manufacturer, is not guaranteed or endorsed by the publisher.
1. Huang J, Chan SC, Ko S, Lok V, Zhang L, Lin X, et al. Updated disease distributions, risk factors, and trends of laryngeal cancer: A global analysis of cancer registries. Int J Surg. (2024) 110:810–9. doi: 10.1097/js9.0000000000000902
2. Baird BJ, Sung CK, Beadle BM, Divi V. Treatment of early-stage laryngeal cancer: A comparison of treatment options. Oral Oncol. (2018) 87:8–16. doi: 10.1016/j.oraloncology.2018.09.012
3. Molteni G, Nocini R, Mattioli F, Nakayama M, Dedivitis RA, Mannelli G, et al. Impact of lymph node ratio and number of lymph node metastases on survival and recurrence in laryngeal squamous cell carcinoma. Head Neck. (2023) 45:2274–93. doi: 10.1002/hed.27471
4. Fang R, Peng L, Chen L, Liao J, Wei F, Long Y, et al. The survival benefit of lymph node dissection in resected T1-2, cn0 supraglottic cancer: A population-based propensity score matching analysis. Head Neck. (2021) 43:1300–10. doi: 10.1002/hed.26596
5. Wang W, Liang H, Zhang Z, Xu C, Wei D, Li W, et al. Comparing three-dimensional and two-dimensional deep-learning, radiomics, and fusion models for predicting occult lymph node metastasis in laryngeal squamous cell carcinoma based on ct imaging: A multicentre, retrospective, diagnostic study. EClinicalMedicine. (2024) 67:102385. doi: 10.1016/j.eclinm.2023.102385
6. Zhao X, Li W, Zhang J, Tian S, Zhou Y, Xu X, et al. Radiomics analysis of ct imaging improves preoperative prediction of cervical lymph node metastasis in laryngeal squamous cell carcinoma. Eur Radiol. (2023) 33:1121–31. doi: 10.1007/s00330-022-09051-4
7. Aktaş A, Gürleyik MG, Aydın Aksu S, Aker F, Güngör S. Diagnostic value of axillary ultrasound, mri, and (18)F-fdg-pet/ct in determining axillary lymph node status in breast cancer patients. Eur J Breast Health. (2022) 18:37–47. doi: 10.4274/ejbh.galenos.2021.2021-3-10
8. Allegra E, Franco T, Domanico R, La Boria A, Trapasso S, Garozzo A. Effectiveness of therapeutic selective neck dissection in laryngeal cancer. ORL; J Oto-rhino-laryngology Its Related Specialties. (2014) 76:89–97. doi: 10.1159/000360995
9. Chen LY, Weng WB, Wang W, Chen JF. Analyses of high-risk factors for cervical lymph node metastasis in laryngeal squamous cell carcinoma and establishment of nomogram prediction model. Ear Nose Throat J. (2021) 100:657s–62s. doi: 10.1177/0145561320901613
10. Pan Y, Zhao X, Zhao D, Liu J. Lymph nodes dissection in elderly patients with T3-T4 laryngeal cancer. Clin Interventions Aging. (2020) 15:2321–30. doi: 10.2147/cia.S283600
11. Chafai N, Bonizzi L, Botti S, Badaoui B. Emerging applications of machine learning in genomic medicine and healthcare. Crit Rev Clin Lab Sci. (2024) 61:140–63. doi: 10.1080/10408363.2023.2259466
12. Kolasa K, Admassu B, Hołownia-Voloskova M, Kędzior KJ, Poirrier JE, Perni S. Systematic reviews of machine learning in healthcare: A literature review. Expert Rev Pharmacoeconomics Outcomes Res. (2024) 24:63–115. doi: 10.1080/14737167.2023.2279107
13. Zhang B, Shi H, Wang H. Machine learning and ai in cancer prognosis, prediction, and treatment selection: A critical approach. J Multidiscip Healthcare. (2023) 16:1779–91. doi: 10.2147/jmdh.S410301
14. Ahmad A, Nawaz MI. Molecular mechanism of vegf and its role in pathological angiogenesis. J Cell Biochem. (2022) 123:1938–65. doi: 10.1002/jcb.30344
15. Chiesa Estomba CM, Betances Reinoso FA, Lorenzo Lorenzo AI, Fariña Conde JL, Araujo Nores J, Santidrian Hidalgo C. Functional outcomes of supraglottic squamous cell carcinoma treated by transoral laser microsurgery compared with horizontal supraglottic laryngectomy in patients younger and older than 65 years. Acta Otorhinolaryngologica Italica: Organo Ufficiale Della Societa Italiana Di Otorinolaringologia E Chirurgia Cervico-facciale. (2016) 36:450–8. doi: 10.14639/0392-100x-864
16. Zhou J, Zhu X, Yang Y, Zhou L, Gong H, Xu C, et al. Predictive value of pathological carcinoma size in patients with T2 glottic laryngeal squamous cell carcinoma. Acta Oto-laryngologica. (2023) 143:317–21. doi: 10.1080/00016489.2023.2188083
17. Al-Shehari T, Alsowail RA. An insider data leakage detection using one-hot encoding, synthetic minority oversampling and machine learning techniques. Entropy (Basel Switzerland). (2021) 23:1258. doi: 10.3390/e23101258
18. Kürten CHL, Zioga E, Gauler T, Stuschke M, Guberina M, Ludwig JM, et al. Patterns of cervical lymph node metastasis in supraglottic laryngeal cancer and therapeutic implications of surgical staging of the neck. Eur Arch Oto-rhino-laryngology: Off J Eur Fed Oto-Rhino-Laryngological Societies (EUFOS): Affiliated German Soc Oto-Rhino-Laryngol Head Neck Surg. (2021) 278:5021–7. doi: 10.1007/s00405-021-06753-1
19. Song L, Heng Y, Hsueh CY, Huang H, Tao L, Zhou L, et al. A predictive nomogram for lymph node metastasis in supraglottic laryngeal squamous cell carcinoma. Front Oncol. (2022) 12:786207. doi: 10.3389/fonc.2022.786207
20. Hu C, Zhang M, Xue J, Gong H, Tao L, Zhou L. Analysis and management of occult cervical lymph node metastasis of cn0 supraglottic laryngeal carcinoma. Lin Chuang Er Bi Yan Hou Tou Jing Wai Ke Za Zhi J Clin Otorhinolaryngol Head Neck Surg. (2020) 34:615–7. doi: 10.13201/j.issn.2096-7993.2020.07.009
21. Bar Ad V, Chalian A. Management of clinically negative neck for the patients with head and neck squamous cell carcinomas in the modern era. Oral Oncol. (2008) 44:817–22. doi: 10.1016/j.oraloncology.2007.12.003
22. Deganello A, Gitti G, Meccariello G, Parrinello G, Mannelli G, Gallo O. Effectiveness and pitfalls of elective neck dissection in N0 laryngeal cancer. Acta Otorhinolaryngologica Italica: Organo Ufficiale Della Societa Italiana Di Otorinolaringologia E Chirurgia Cervico-facciale. (2011) 31:216–21.
23. Ambrosch P, Fazel A, Dietz A, Fietkau R, Tostmann R, Borzikowsky C. Multicenter clinical trial on functional evaluation of transoral laser microsurgery for supraglottic laryngeal carcinomas. Laryngo- Rhino- Otologie. (2024). doi: 10.1055/a-2321-5968
24. Riviere D, Mancini J, Santini L, Loth Bouketala A, Giovanni A, Dessi P, et al. Nodal metastases distribution in laryngeal cancer requiring total laryngectomy: therapeutic implications for the N0 neck. Eur Ann Otorhinolaryngol Head Neck Dis. (2019) 136:S35–s8. doi: 10.1016/j.anorl.2018.08.011
25. Wei B, Yao J, Peng C, Zhao S, Wang H, Wang L, et al. Clinical features and imaging examination assessment of cervical lymph nodes for thyroid carcinoma. BMC Cancer. (2023) 23:1225. doi: 10.1186/s12885-023-11721-5
26. Shao N, Wei X, Zhang Y, Luo H, Su Y, Liang L, et al. Effect of different surgical modalities on swallowing-related quality of life in patients with glottic laryngeal squamous cell carcinoma: how should we choose? Arch Med Sci: AMS. (2023) 19:550–4. doi: 10.5114/aoms/161230
27. Okeke UA, Igashi JB, Hamza MA, Ajike SO, Saheeb BD. Sonographic diagnosis of metastatic cervical lymph nodes in primary orofacial Malignancies: role of the radiologist’s experience. West Afr J Med. (2021) 38:24–7.
28. Guo Y, Strauss VY, Català M, Jödicke AM, Khalid S, Prieto-Alhambra D. Machine learning methods for propensity and disease risk score estimation in high-dimensional data: A plasmode simulation and real-world data cohort analysis. Front Pharmacol. (2024) 15:1395707. doi: 10.3389/fphar.2024.1395707
29. Gao J, Bai D, Chen H, Chen X, Luo H, Ji W, et al. Risk factors analysis of cognitive frailty among geriatric adults in nursing homes based on logistic regression and decision tree modeling. Front Aging Neurosci. (2024) 16:1485153. doi: 10.3389/fnagi.2024.1485153
30. Cui J, Wang L, Zhong W, Chen Z, Tan X, Yang H, et al. Development and validation of nomogram to predict risk of survival in patients with laryngeal squamous cell carcinoma. Biosci Rep. (2020) 40:BSR20200228. doi: 10.1042/bsr20200228
31. Li J. Area under the roc curve has the most consistent evaluation for binary classification. PloS One. (2024) 19:e0316019. doi: 10.1371/journal.pone.0316019
32. Thölke P, Mantilla-Ramos YJ, Abdelhedi H, Maschke C, Dehgan A, Harel Y, et al. Class imbalance should not throw you off balance: choosing the right classifiers and performance metrics for brain decoding with imbalanced data. NeuroImage. (2023) 277:120253. doi: 10.1016/j.neuroimage.2023.120253
33. Tachibana T, Orita Y, Marunaka H, Makihara SI, Hirai M, Gion Y, et al. Neck metastasis in patients with T1-2 supraglottic cancer. Auris Nasus Larynx. (2018) 45:540–5. doi: 10.1016/j.anl.2017.06.002
34. He X, Deng T, Li J, Guo R, Wang Y, Li T, et al. A Core-Satellite Micellar System against Primary Tumors and Their Lymphatic Metastasis through Modulation of Fatty Acid Metabolism Blockade and Tumor-Associated Macrophages. Nanoscale. (2023) 15:8320–36. doi: 10.1039/d2nr04693h
35. Paul R, Schabath MB, Gillies R, Hall LO, Goldgof DB. Hybrid models for lung nodule Malignancy prediction utilizing convolutional neural network ensembles and clinical data. J Med Imaging (Bellingham Wash). (2020) 7:24502. doi: 10.1117/1.Jmi.7.2.024502
36. Monthatip K, Boonnag C, Muangmool T, Charoenkwan K. A machine learning-based prediction model of pelvic lymph node metastasis in women with early-stage cervical cancer. J Gynecol Oncol. (2024) 35:e17. doi: 10.3802/jgo.2024.35.e17
37. Wang SX, Ning WJ, Zhang XW, Tang PZ, Li ZJ, Liu WS. Predictors of occult lymph node metastasis and prognosis in patients with cn0 T1-T2 supraglottic laryngeal carcinoma: A retrospective study. ORL; J Oto-rhino-laryngology Its Related Specialties. (2019) 81:317–26. doi: 10.1159/000503007
38. Ozdek A, Sarac S, Akyol MU, Unal OF, Sungur A. Histopathological predictors of occult lymph node metastases in supraglottic squamous cell carcinomas. Eur Arch Oto-rhino-laryngology: Off J Eur Fed Oto-Rhino-Laryngological Societies (EUFOS): Affiliated German Soc Oto-Rhino-Laryngol Head Neck Surg. (2000) 257:389–92. doi: 10.1007/s004050000231
39. Jögi A, Vaapil M, Johansson M, Påhlman S. Cancer cell differentiation heterogeneity and aggressive behavior in solid tumors. Upsala J Med Sci. (2012) 117:217–24. doi: 10.3109/03009734.2012.659294
40. Myung D-S, Oh HH, Kim JS, Lim JW, Lim CJ, Gim SE, et al. Cytochrome P450 family 46 subfamily a member 1 promotes the progression of colorectal cancer by inducing tumor cell proliferation and angiogenesis. J Anticancer Res. (2023) 43:4915–22. doi: 10.21873/anticanres.16689
41. Feng L, Yang J, Zhang W, Wang X, Li L, Peng M, et al. Prognostic significance and identification of basement membrane-associated lncrna in bladder cancer. Front Oncol. (2022) 12:994703. doi: 10.3389/fonc.2022.994703
42. Fan SJ, Cui Y, Li YH, Xu JC, Shen YY, Huang H, et al. Lncrna casc9 activated by stat3 promotes the invasion of breast cancer and the formation of lymphatic vessels by enhancing H3k27ac-activated sox4. Kaohsiung J Med Sci. (2022) 38:848–57. doi: 10.1002/kjm2.12573
43. Madishetty V, Starr AJ, Chu QD, Starr PAB. Evaluating the presence of a stage iv low-grade well-differentiated neuroendocrine tumor of the ileocecum: A case report with evaluation of staging protocol of neuroendocrine tumors and treatment options based on current available evidence. Case Rep Surg. (2023) 2023:2919223. doi: 10.1155/2023/2919223
44. Jiang H, Yu D, Yang P, Guo R, Kong M, Gao Y, et al. Revealing the transcriptional heterogeneity of organ-specific metastasis in human gastric cancer using single-cell rna sequencing. Clin Trans Med. (2022) 12:e730. doi: 10.1002/ctm2.730
45. Mutlu V, Ucuncu H, Altas E, Aktan B. The relationship between the localization, size, stage and histopathology of the primary laryngeal tumor with neck metastasis. Eurasian J Med. (2014) 46:1–7. doi: 10.5152/eajm.2014.01
46. Yoruk O, Dane S, Ucuncu H, Aktan B, Can I. Stereological evaluation of laryngeal cancers using computed tomography via the cavalieri method: correlation between tumor volume and number of neck lymph node metastases. J Craniofacial Surg. (2009) 20:1504–7. doi: 10.1097/SCS.0b013e3181b09bc3
47. Hu Q, Chen Y, Zhou Q, Deng S, Mu B, Tang J. Asb6 as an independent prognostic biomarker for colorectal cancer progression involves lymphatic invasion and immune infiltration. J Cancer. (2024) 15:2712–30. doi: 10.7150/jca.93066
48. Jangir NK, Singh A, Jain P, Khemka S. The predictive value of depth of invasion and tumor size on risk of neck node metastasis in squamous cell carcinoma of the oral cavity: A prospective study. J Cancer Res Ther. (2022) 18:977–83. doi: 10.4103/jcrt.JCRT_783_20
49. Yang HJ, Lee H, Kim TJ, Jung DH, Choi KD, Ahn JY, et al. A modified ecura system to stratify the risk of lymph node metastasis in undifferentiated-type early gastric cancer after endoscopic resection. J Gastric Cancer. (2024) 24:172–84. doi: 10.5230/jgc.2024.24.e13
50. Jia Y, Zhao H, Hao Y, Zhu J, Li Y, Wang Y. Analysis of the related risk factors of inguinal lymph node metastasis in patients with penile cancer: A cross-sectional study. Int Braz J Urol: Off J Braz Soc Urol. (2022) 48:303–13. doi: 10.1590/s1677-5538.Ibju.2021.0613
51. Wu R, Oshi M, Asaoka M, Yamada A, Takabe Y, Yan L, et al. Abstract P5-06-03: intratumoral lymphatic endothelial cell infiltration reflects lymphangiogenesis and lymph node metastasis, but is counterbalanced by immune response and better cancer biology in breast cancer tumor microenvironment. Cancer Res. (2022) 82:P5-06-3-P5-3. doi: 10.1158/1538-7445.SABCS21-P5-06-03
52. Kawasaki K, Kai K, Minesaki A, Maeda S, Yamauchi M, Kuratomi Y. Chemoradiotherapy and lymph node metastasis affect dendritic cell infiltration and maturation in regional lymph nodes of laryngeal cancer. Int J Mol Sci. (2024) 25(4). doi: 10.3390/ijms25042093
53. Li X, Wang J, Sun H, Hu Y, Wang D, Zhao G. Analysis of correlated factors of cervical lymphatic metastasis of T3 and T4 glottic carcinoma. Lin Chuang Er Bi Yan Hou Tou Jing Wai Ke Za Zhi J Clin Otorhinolaryngol Head Neck Surg. (2015) 29:1517–8.
54. Shao Y, Tu X, Liu Y, Bao Y, Ren S, Yang Z, et al. Predict lymph node metastasis in penile cancer using clinicopathological factors and nomograms. Cancer Manage Res. (2021) 13:7429–37. doi: 10.2147/cmar.S329925
55. Kowalski LP, Franco EL, de Andrade Sobrinho J. Factors influencing regional lymph node metastasis from laryngeal carcinoma. Ann Otology Rhinol Laryngol. (1995) 104:442–7. doi: 10.1177/000348949510400605
Keywords: big data, precision medicine, early-stage supraglottic laryngeal cancer, lymph node metastasis, machine learning
Citation: Wang H, He Z, Xu J, Chen T, Huang J, Chen L and Yue X (2025) Development and validation of a machine learning model to predict the risk of lymph node metastasis in early-stage supraglottic laryngeal cancer. Front. Oncol. 15:1525414. doi: 10.3389/fonc.2025.1525414
Received: 09 November 2024; Accepted: 10 January 2025;
Published: 29 January 2025.
Edited by:
Lushan Xiao, Southern Medical University, ChinaReviewed by:
Yangbing Jin, Shanghai Jiao Tong University, ChinaCopyright © 2025 Wang, He, Xu, Chen, Huang, Chen and Yue. This is an open-access article distributed under the terms of the Creative Commons Attribution License (CC BY). The use, distribution or reproduction in other forums is permitted, provided the original author(s) and the copyright owner(s) are credited and that the original publication in this journal is cited, in accordance with accepted academic practice. No use, distribution or reproduction is permitted which does not comply with these terms.
*Correspondence: Ting Chen, OTIwMTU1MTE3M0Bmam11LmVkdS5jbg==
†These authors have contributed equally to this work
Disclaimer: All claims expressed in this article are solely those of the authors and do not necessarily represent those of their affiliated organizations, or those of the publisher, the editors and the reviewers. Any product that may be evaluated in this article or claim that may be made by its manufacturer is not guaranteed or endorsed by the publisher.
Research integrity at Frontiers
Learn more about the work of our research integrity team to safeguard the quality of each article we publish.