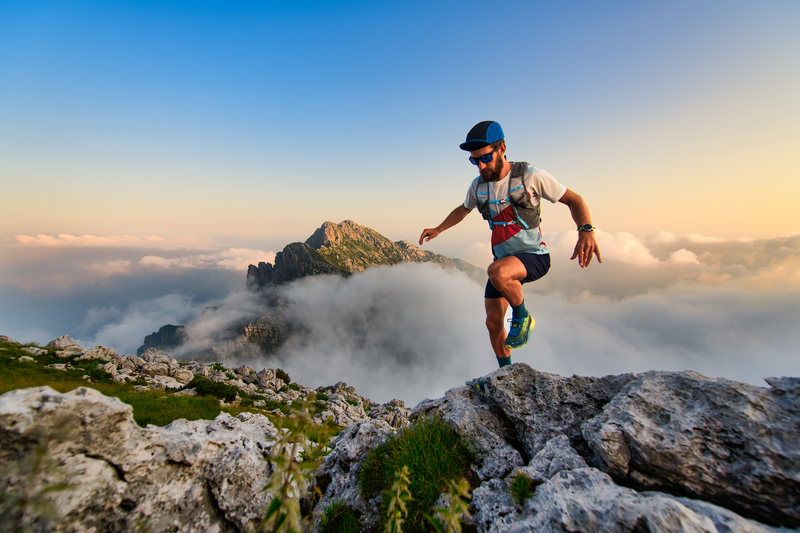
95% of researchers rate our articles as excellent or good
Learn more about the work of our research integrity team to safeguard the quality of each article we publish.
Find out more
ORIGINAL RESEARCH article
Front. Oncol.
Sec. Cancer Imaging and Image-directed Interventions
Volume 15 - 2025 | doi: 10.3389/fonc.2025.1523507
The final, formatted version of the article will be published soon.
You have multiple emails registered with Frontiers:
Please enter your email address:
If you already have an account, please login
You don't have a Frontiers account ? You can register here
The aim of this study was to explore the application value of dynamic contrast-enhanced magnetic resonance imaging (DCE-MRI) radiomics and heterogeneity analysis in the differentiation of molecular subtypes of luminal and non-luminal breast cancer.In this retrospective study, 388 female breast cancer patients (48.37 ± 9.41 years) with luminal (n = 190) and non-luminal (n = 198) molecular subtypes who received surgical treatment at Jilin Cancer Hospital were recruited from January 2019 to June 2023. All patients underwent breast MRI scan and DCE scan before surgery. The patients were then divided into a training set (n = 272) and a validation set (n = 116) in a 7:3 ratio. The three-dimensional texture feature parameters of the breast lesion areas were extracted. Four tumor heterogeneity parameters, including type I curve proportion, type II curve proportion, type III curve proportion and tumor heterogeneity values were calculated and normalized. Five machine learning (ML) models, including the logistic regression, naive Bayes algorithm (NB), k-nearest neighbor (KNN), decision tree algorithm (DT) and extreme gradient boosting (XGBoost) model were used to process the training data and were further validated. The best ML model was selected according to the performance in the validation set.In luminal subtype breast lesions, type Ⅲ curve proportion and heterogeneity index were significantly lower than the corresponding parameters of the non-luminal subtype lesions both in the training set and validation set. Eight features together with four heterogeneity-related parameters with significant differences between luminal and non-luminal groups were retained as radiomics signatures for constructing the prediction model. The logistic regression ML model achieved the best performance in the validation set with the highest area under the curve value (0.93), highest accuracy (86.94 %), sensitivity (87.55 %) and specificity (86.25 %).The radiomics and heterogeneity analysis based on the DCE-MRI exhibit good application value in discriminating luminal and non-luminal subtype breast cancer. The logistic regression model demonstrates the best predictive performance among various machine learning models.
Keywords: Radiomics, heterogeneity analysis, Molecular subtype, breast cancer, Magnetic Resonance Imaging
Received: 06 Nov 2024; Accepted: 27 Mar 2025.
Copyright: © 2025 Yao, Ye, Wang, Shen, Yan, Zou and Sun. This is an open-access article distributed under the terms of the Creative Commons Attribution License (CC BY). The use, distribution or reproduction in other forums is permitted, provided the original author(s) or licensor are credited and that the original publication in this journal is cited, in accordance with accepted academic practice. No use, distribution or reproduction is permitted which does not comply with these terms.
* Correspondence:
Ming Yao, Department of Radiology, Jilin Cancer Hospital, Changchun, China
Disclaimer: All claims expressed in this article are solely those of the authors and do not necessarily represent those of their affiliated organizations, or those of the publisher, the editors and the reviewers. Any product that may be evaluated in this article or claim that may be made by its manufacturer is not guaranteed or endorsed by the publisher.
Research integrity at Frontiers
Learn more about the work of our research integrity team to safeguard the quality of each article we publish.