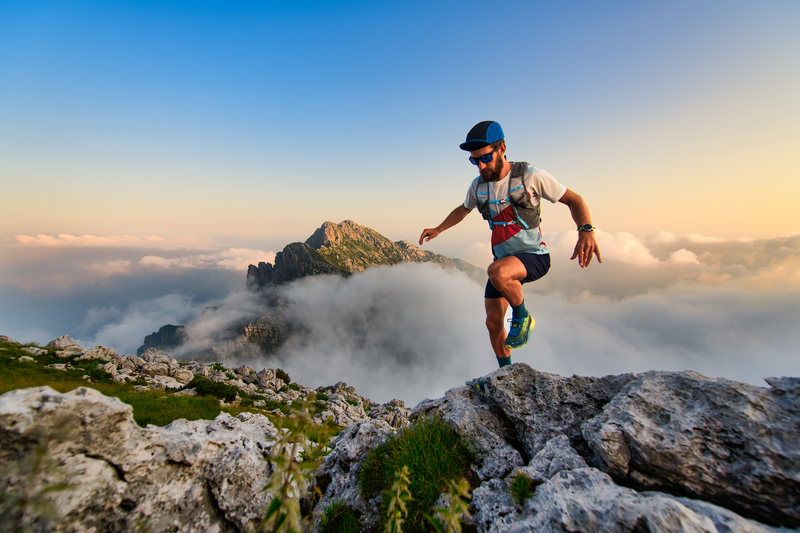
94% of researchers rate our articles as excellent or good
Learn more about the work of our research integrity team to safeguard the quality of each article we publish.
Find out more
ORIGINAL RESEARCH article
Front. Oncol.
Sec. Gynecological Oncology
Volume 15 - 2025 | doi: 10.3389/fonc.2025.1515976
This article is part of the Research Topic Interdisciplinary Approaches for Uncovering the Anti-tumor Mechanisms of Chinese Drugs and Natural Products View all articles
The final, formatted version of the article will be published soon.
You have multiple emails registered with Frontiers:
Please enter your email address:
If you already have an account, please login
You don't have a Frontiers account ? You can register here
Background: Ovarian cancer is the most prevalent malignant tumor of the female reproductive system and has the highest mortality rate among gynecological cancers. Columbianetin acetate (CE) is one of the active ingredients of Angelica sinensis, which has good antifungal and anti-inflammatory activities. However, its potential mechanism of action in ovarian cancer remains unclear. This study used network pharmacology and molecular docking technology to investigate the molecular mechanism and material basis of CE in the treatment of ovarian cancer, and further verified by in vitro experiments.Methods: Relevant targets for CE were obtained from TCMSP and SwissTargetPrediction databases. OMIM, GeneCards and DisGeNET databases were applied to screen ovarian cancer-related targets. The STRING database to obtain protein-protein interaction (PPI) network. Then key targets were obtained using Cytoscape software, followed by expression, survival and ROC diagnostic analyses of core genes using R software. GO and KEGG enrichment analyses were performed using the DAVID database. Binding ability of CE to core targets was assessed by molecular docking. KEGG sites were used to predict core gene-related pathways. Subsequently, in vitro cellular experiments were performed to further investigate the molecular mechanism of CE treatment for ovarian cancer.Results: A total of 55 CE-ovarian cancer interaction targets were identified using network pharmacology techniques. Among these, eight key targets -ESR1, GSK3B, JAK2, MAPK1, MDM2, PARP1, PIK3CA, and SRC-were screened using Cytoscape software. Core genes ESR1, GSK3B and JAK2 were obtained based on expression, prognostic and diagnostic values using R software. GO and KEGG enrichment analyses indicated that CE treatment of ovarian cancer might be related to PI3K-Akt signaling pathway, MAPK signaling pathway, ErbB signaling pathway and Ras signaling pathway. The molecular docking results showed that CE had good binding ability with core targets ESR1, GSK3B and JAK2. The results of in vitro cellular experiments indicated that CE may inhibit the proliferation and metastasis of ovarian cancer and promote apoptosis by inhibiting the PI3K/AKT/GSK3B pathway.Based on the network pharmacology approach, we predicted the potential mechanism of CE for the treatment of ovarian cancer, which provided a new idea for further research on its pharmacological mechanism.
Keywords: Network Pharmacology, Columbianetin acetate, ovarian cancer, metastasis, Apoptosis
Received: 23 Oct 2024; Accepted: 03 Feb 2025.
Copyright: © 2025 Hu, Wang, Zhang, Xie, Zhang, Liu, Li and Zhang. This is an open-access article distributed under the terms of the Creative Commons Attribution License (CC BY). The use, distribution or reproduction in other forums is permitted, provided the original author(s) or licensor are credited and that the original publication in this journal is cited, in accordance with accepted academic practice. No use, distribution or reproduction is permitted which does not comply with these terms.
* Correspondence:
Jing Zhang, Bengbu Medical College, Bengbu, 233030, Anhui Province, China
Disclaimer: All claims expressed in this article are solely those of the authors and do not necessarily represent those of their affiliated organizations, or those of the publisher, the editors and the reviewers. Any product that may be evaluated in this article or claim that may be made by its manufacturer is not guaranteed or endorsed by the publisher.
Research integrity at Frontiers
Learn more about the work of our research integrity team to safeguard the quality of each article we publish.