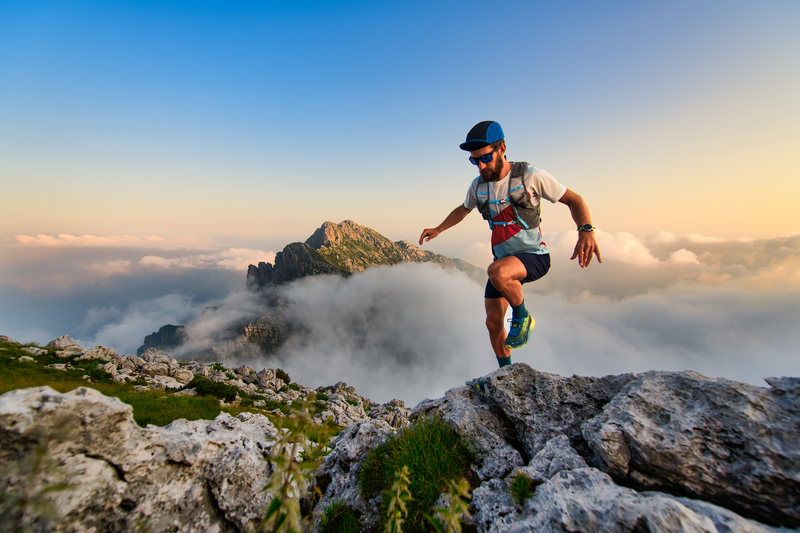
94% of researchers rate our articles as excellent or good
Learn more about the work of our research integrity team to safeguard the quality of each article we publish.
Find out more
ORIGINAL RESEARCH article
Front. Oncol.
Sec. Gastrointestinal Cancers: Colorectal Cancer
Volume 15 - 2025 | doi: 10.3389/fonc.2025.1512960
This article is part of the Research Topic Impact of the Inflammatory Microenvironment on Immune Infiltration in Colorectal and Liver Cancers: Insights for New Immunotherapeutic Strategies View all 4 articles
The final, formatted version of the article will be published soon.
You have multiple emails registered with Frontiers:
Please enter your email address:
If you already have an account, please login
You don't have a Frontiers account ? You can register here
Introduction: Lymph node metastasis is a crucial determinant of prognosis in colorectal cancer (CRC), significantly impacting survival outcomes and treatment decision-making. This study aims to evaluate the prognostic value of tumor infiltration proportion within lymph nodes (TIPLN) in N1 CRC patients and to develop a TIPLN-based nomogram to predict prognosis. Methods: A total of 416 N1 CRC patients who underwent radical resection were enrolled and divided into training and validation cohorts. Whole-slide images of lymph nodes were annotated to assess the TIPLN. Univariable and multivariable Cox regression analyses were conducted to identify independent prognostic factors and to develop a nomogram for predicting patient outcomes. The precision and discrimination of the nomogram were evaluated using the area under the receiver operating characteristic curve (AUC), concordance index (C-index), and calibration curve. Decision curve analysis (DCA) was performed to compare the net benefit of the nomogram at different threshold probabilities. Additionally, net reclassification index (NRI) and integrated discrimination improvement (IDI) were used to evaluate the nomogram's clinical utility. Results: High TIPLN levels were significantly associated with poorer overall survival (OS). Five variables, including TIPLN, were selected to construct the nomogram. The C-index in OS prediction was 0.739 and 0.753 for the training and validation cohorts, respectively. Additionally, strong precision and discrimination were demonstrated through AUC and calibration curves. The NRI (training cohort: 0.191 for 3-year and 0.436 for 5-year OS prediction; validation cohort: 0.180 for 3-year and 0.439 for 5-year OS prediction) and IDI (training cohort: 0.079 for 3-year and 0.094 for 5-year OS prediction; validation cohort: 0.078 for 3-year and 0.098 for 5-year OS prediction) suggest that the TIPLN-based nomogram significantly outperformed the clinicopathological nomogram. Furthermore, DCA demonstrated the high clinical applicability of the TIPLN-based nomogram for predicting OS. Conclusions: TIPLN could serve as a prognostic predictor for N1 CRC patients. The TIPLN-based nomogram enhances survival prediction accuracy and facilitates more informed, individualized clinical decision-making.
Keywords: colorectal cancer, N1 stage, tumor infiltration proportion, Lymph Nodes, Prognostic model
Received: 17 Oct 2024; Accepted: 03 Mar 2025.
Copyright: © 2025 Li, Chen, Xu, Zhu, Fan, Qiao, Jiang, Hao, Du, 陈 and Yuan. This is an open-access article distributed under the terms of the Creative Commons Attribution License (CC BY). The use, distribution or reproduction in other forums is permitted, provided the original author(s) or licensor are credited and that the original publication in this journal is cited, in accordance with accepted academic practice. No use, distribution or reproduction is permitted which does not comply with these terms.
* Correspondence:
Jipeng Li, Xijing Hospital,Air force Medical University, Xi’an, China
Disclaimer: All claims expressed in this article are solely those of the authors and do not necessarily represent those of their affiliated organizations, or those of the publisher, the editors and the reviewers. Any product that may be evaluated in this article or claim that may be made by its manufacturer is not guaranteed or endorsed by the publisher.
Research integrity at Frontiers
Learn more about the work of our research integrity team to safeguard the quality of each article we publish.