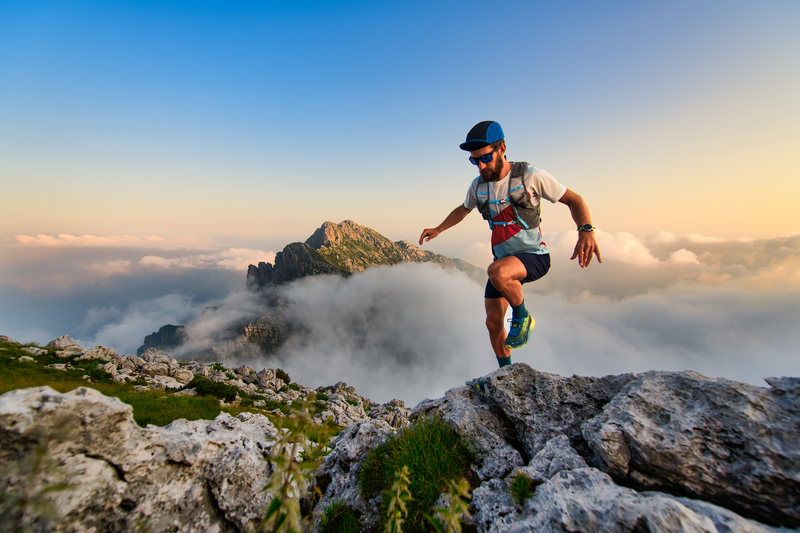
95% of researchers rate our articles as excellent or good
Learn more about the work of our research integrity team to safeguard the quality of each article we publish.
Find out more
ORIGINAL RESEARCH article
Front. Oncol.
Sec. Cancer Imaging and Image-directed Interventions
Volume 15 - 2025 | doi: 10.3389/fonc.2025.1512739
This article is part of the Research Topic Deep Learning for Medical Imaging Applications View all 14 articles
The final, formatted version of the article will be published soon.
You have multiple emails registered with Frontiers:
Please enter your email address:
If you already have an account, please login
You don't have a Frontiers account ? You can register here
Brain tumors pose significant harm to the functionality of the human nervous system. There are lots of models which can classify brain tumor type. However, the available methods did not pay special attention to long-range information, which limits model accuracy improvement. To solve this problem, in this paper, an enhanced short-range and long-range dependent system for brain tumor classification, named as EnSLDe, is proposed. The EnSLDe model consists of three main modules: the Feature Extraction Module (FExM), the Feature Enhancement Module (FEnM), and the Classification Module. Firstly, the FExM is used to extract features and the multi-scale parallel subnetwork is constructed to fuse shallow and deep features. Then, the extracted features are enhanced by the FEnM. The FEnM can capture the important dependencies across a larger sequence range and retain critical information at a local scale. Finally, the fused and enhanced features are input to the classification module for brain tumor classification. The combination of these modules enables the efficient extraction of both local and global contextual information. In order to validate the model, two public data sets including glioma, meningioma, and pituitary tumor were validated, and good experimental results were obtained, demonstrating the potential of the model EnSLDe in brain tumor classification.
Keywords: Brain tumor classification, feature extraction, Feature enhancement, long-range dependencies, Attention 1
Received: 19 Oct 2024; Accepted: 21 Mar 2025.
Copyright: © 2025 Chen, Liu, Tan, Zhang, Du, Fu and Jiang. This is an open-access article distributed under the terms of the Creative Commons Attribution License (CC BY). The use, distribution or reproduction in other forums is permitted, provided the original author(s) or licensor are credited and that the original publication in this journal is cited, in accordance with accepted academic practice. No use, distribution or reproduction is permitted which does not comply with these terms.
* Correspondence:
Wenna Chen, The First Affiliated Hospital of Henan University of Science and Technology, Luoyang, China
Disclaimer: All claims expressed in this article are solely those of the authors and do not necessarily represent those of their affiliated organizations, or those of the publisher, the editors and the reviewers. Any product that may be evaluated in this article or claim that may be made by its manufacturer is not guaranteed or endorsed by the publisher.
Research integrity at Frontiers
Learn more about the work of our research integrity team to safeguard the quality of each article we publish.