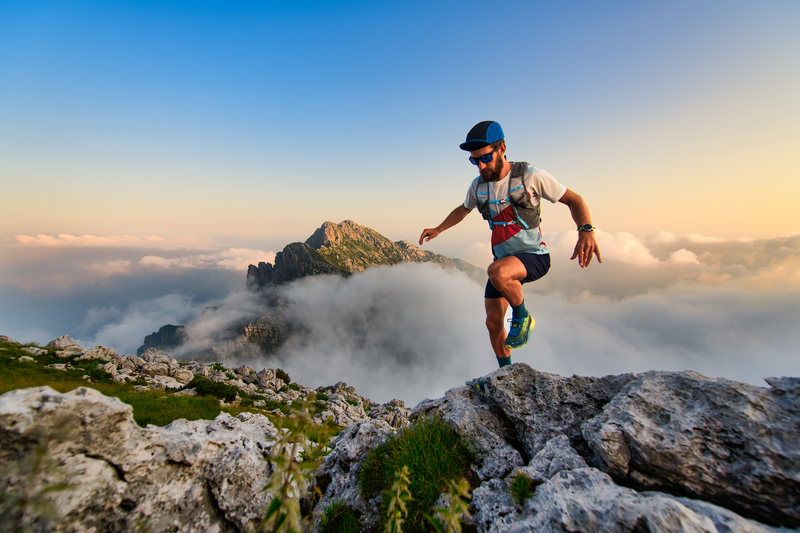
94% of researchers rate our articles as excellent or good
Learn more about the work of our research integrity team to safeguard the quality of each article we publish.
Find out more
ORIGINAL RESEARCH article
Front. Oncol.
Sec. Cancer Molecular Targets and Therapeutics
Volume 15 - 2025 | doi: 10.3389/fonc.2025.1511564
The final, formatted version of the article will be published soon.
You have multiple emails registered with Frontiers:
Please enter your email address:
If you already have an account, please login
You don't have a Frontiers account ? You can register here
Lung squamous cell carcinoma (LUSC) is a malignant disease associated with poor therapeutic responses and prognosis. Preliminary studies have shown that the dysregulation of long non-coding RNAs (LncRNAs) is linked to cancer development and prognosis. However, research on the role of LncRNAs in LUSC remains limited. In this study, we aimed to develop a LncRNA signature for improved prognostic prediction in LUSC and to elucidate the underlying mechanisms. We utilized expression data of LncRNAs and clinical information from 471 LUSC patients in The Cancer Genome Atlas (TCGA), randomly dividing them into a training set (n=236) and a testing set (n=235). A prognostic signature model comprising seven LncRNAs was constructed using multivariate Cox regression analysis based on the training set. Using a risk score cutoff value of -0.12 (log2transformed), patients were categorized into high-risk (n=101) and low-risk (n=370) groups. The high-risk group demonstrated significantly worse overall survival (OS) compared to the low-risk group (p<0.0001). The risk score showed strong prognostic predictive ability for LUSC patients, as evidenced by the area under the ROC curve (AUC: 0.66, 0.67, and 0.67) and nomogram analysis (C-index, calibration, and decision curve analysis) for 1-, 3-, and 5-year survival predictions. Independent prognostic factors for LUSC were identified, including risk group (HR=0.3, 95% CI: 0.22-0.4), stage (HR=1.78, 95% CI: 1.28-2.48), and age (HR=1.02, 95% CI: 1.00-1.04). KEGG enrichment analysis revealed that mRNAs influenced by the seven targeted LncRNAs, associated with immune evasion, were primarily linked to pathways such as chemical carcinogenesis, Th17 cell differentiation, NF-κB signaling, and proteoglycans in cancer. Expression levels of 14 target genes related to tumor immune tolerance were significantly suppressed, with eight confirmed via real-time PCR and western blot analysis. Additionally, CIBERSORT analysis of immune cellrelated gene expression between normal and LUSC tissues indicated activation of the immune system in LUSC patients. In conclusion, our findings highlight the clinical significance of the seven LncRNA signature in predicting survival outcomes for LUSC patients.
Keywords: long non-coding RNA, prognosis, Lung squamous cell carcinoma, immune escape, seven LncRNA signature
Received: 15 Oct 2024; Accepted: 05 Mar 2025.
Copyright: © 2025 ge, lin, Xuequan, jiang and Hu. This is an open-access article distributed under the terms of the Creative Commons Attribution License (CC BY). The use, distribution or reproduction in other forums is permitted, provided the original author(s) or licensor are credited and that the original publication in this journal is cited, in accordance with accepted academic practice. No use, distribution or reproduction is permitted which does not comply with these terms.
* Correspondence:
zhengli jiang, Zhejiang Taizhou Hospital, Taizhou, China
Yan Hu, Zhejiang Taizhou Hospital, Taizhou, China
Disclaimer: All claims expressed in this article are solely those of the authors and do not necessarily represent those of their affiliated organizations, or those of the publisher, the editors and the reviewers. Any product that may be evaluated in this article or claim that may be made by its manufacturer is not guaranteed or endorsed by the publisher.
Research integrity at Frontiers
Learn more about the work of our research integrity team to safeguard the quality of each article we publish.