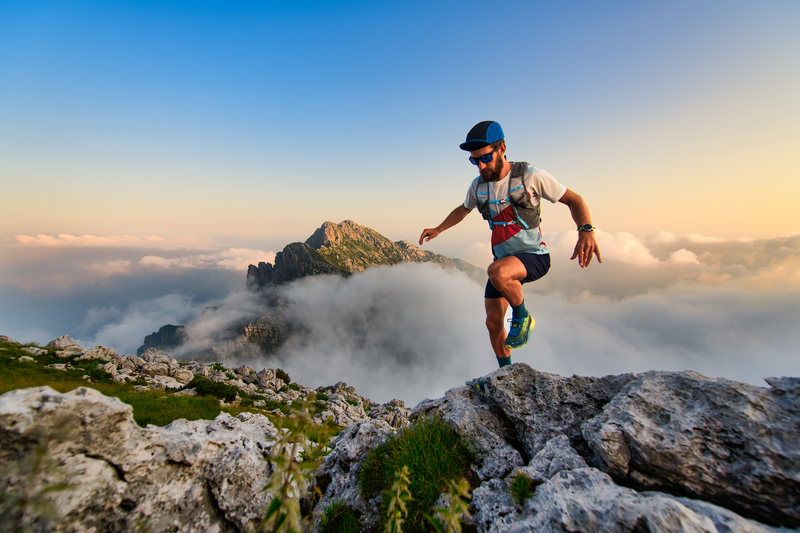
94% of researchers rate our articles as excellent or good
Learn more about the work of our research integrity team to safeguard the quality of each article we publish.
Find out more
ORIGINAL RESEARCH article
Front. Oncol.
Sec. Cancer Imaging and Image-directed Interventions
Volume 15 - 2025 | doi: 10.3389/fonc.2025.1510386
The final, formatted version of the article will be published soon.
You have multiple emails registered with Frontiers:
Please enter your email address:
If you already have an account, please login
You don't have a Frontiers account ? You can register here
Background: This study aimed to develop and validate radiomics-based nomograms for the identification of EGFR mutations in non-small cell lung cancer (NSCLC).Methods: A retrospective analysis was performed on 313 NSCLC patients, who were randomly divided into training (n = 250) and validation (n = 63) groups. Radiomic features were extracted from 18F-fluorodeoxyglucose positron emission tomography (18F-FDG PET) and thin-section computed tomography (CT) scans. After selecting optimal radiomic features, four machine learning algorithms, including logistic regression (LR), random forest (RF), support vector machine (SVM), and extreme gradient boosting (XGBoost), were used to develop and validate radiomics models. A combined model, incorporating the Rad score from the best performing radiomics model with clinical and radiological features, was then formulated. Finally, the integrated nomogram was generated. Its predictive performance and clinical utility were evaluated using receiver operating characteristic curves, calibration curves, and decision curve analysis.Results: Among the radiomics models, the RF model showed the best performance with AUCs of 0.785 (95% CI, 0.726-0.844) and 0.776 (95% CI, 0.662-0.889) in the training and validation groups, respectively. The AUCs of the clinical and radiological models in both groups were 0.711 (95% CI, 0.645-0.776) and 0.758 (95% CI, 0.627-0.890), and 0.632 (95% CI, 0.564-0.699) and 0.677 (95% CI, 0.531-0.822), respectively. The combined model achieved the highest AUCs of 0.872 (95% CI, 0.829-0.915) and 0.831 (95% CI, 0.723-0.940) in the training and validation groups, respectively. The DeLong test confirmed the superiority of the combined model over the other three models. Both the calibration curve and the DCA indicated that the radiomics nomogram was consistent and clinically useful. Conclusions: Radiomics combined with machine learning and based on 18F-FDG PET/CT images can effectively determine EGFR mutation status in NSCLC patients. Radiomics-based nomograms provide a non-invasive and visually intuitive prediction tool for screening NSCLC patients with EGFR mutations in a clinical setting.
Keywords: nomogram, Non-small cell lung cancer, PET/CT, machine learning, epidermal growth factor receptor
Received: 12 Oct 2024; Accepted: 14 Mar 2025.
Copyright: © 2025 Li, Shi, Yang, Xie, Xie, Ni and Wang. This is an open-access article distributed under the terms of the Creative Commons Attribution License (CC BY). The use, distribution or reproduction in other forums is permitted, provided the original author(s) or licensor are credited and that the original publication in this journal is cited, in accordance with accepted academic practice. No use, distribution or reproduction is permitted which does not comply with these terms.
* Correspondence:
Ming Ni, Department of Nuclear Medicine, The First Affiliated Hospital of University of Science and Technology of China Anhui Provincial Hospital, Hefei, 230001, Anhui Province, China
Xuemei Wang, The Affiliated Hospital of Inner Mongolia Medical University, Hohhot, China
Disclaimer: All claims expressed in this article are solely those of the authors and do not necessarily represent those of their affiliated organizations, or those of the publisher, the editors and the reviewers. Any product that may be evaluated in this article or claim that may be made by its manufacturer is not guaranteed or endorsed by the publisher.
Research integrity at Frontiers
Learn more about the work of our research integrity team to safeguard the quality of each article we publish.