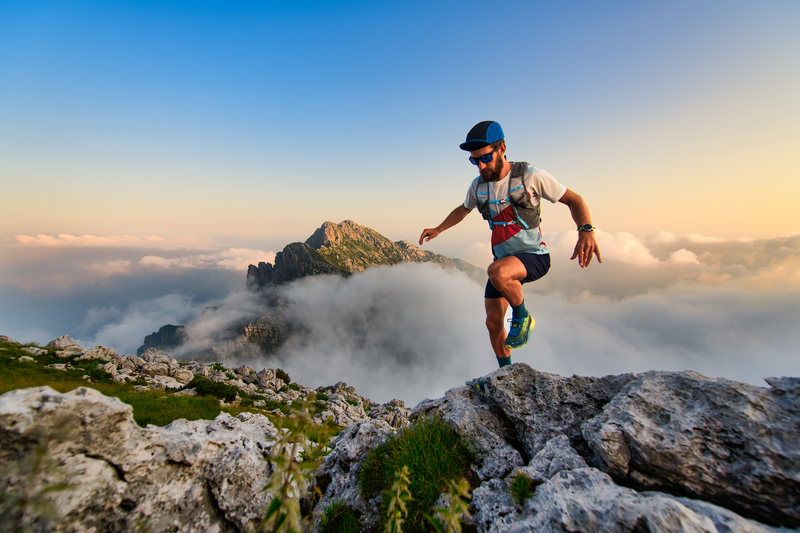
95% of researchers rate our articles as excellent or good
Learn more about the work of our research integrity team to safeguard the quality of each article we publish.
Find out more
ORIGINAL RESEARCH article
Front. Oncol.
Sec. Gastrointestinal Cancers: Colorectal Cancer
Volume 15 - 2025 | doi: 10.3389/fonc.2025.1509207
This article is part of the Research Topic Artificial Intelligence-based Multimodal Imaging and Multi-omics in Medical Research View all 3 articles
The final, formatted version of the article will be published soon.
You have multiple emails registered with Frontiers:
Please enter your email address:
If you already have an account, please login
You don't have a Frontiers account ? You can register here
Objectives: This study aims to develop and evaluate multiple machine learning models utilizing contrast-enhanced T1-weighted imaging (T1-CE) to differentiate between low-/high-infiltration of total T lymphocytes (CD3) in patients with rectal cancer.We retrospectively selected 157 patients (103 men, 54 women) with pathologically confirmed rectal cancer diagnosed between March 2015 and October 2019. The cohort were randomly divided into training dataset (n=109) and test dataset (n=48) for subsequent analysis. Seven radiomic features were selected to generate three models: LR, RF, and SVM. Diagnostic performance of the three models was compared using the Delong test. Additionally, Kaplan-Meier analysis was employed to assess DFS in patients with high and low CD3+ TIL density.The three radiomics models perform well in predicting the infiltration of CD3+ TILS, with AUC values of 0.871, 0.982, and 0.913, respectively, in the training set for the LR, RF, and SVM models. In the validation set, the corresponding AUC values were 0.869, 0.794, and 0.837, respectively. Among the radiomics models, the LR model exhibited superior diagnostic performance and robustness. The merged model, which integrated radiomics features from SVM model and clinical features from clinical model, outperformed the individual radiomics models, with AUCs of 0.8932 and 0.8829 in the training and test cohorts, respectively. Additionally, a lower expression level of CD3+ TILs cohort was independently correlated with disease-free survival (DFS) (P = 0.0041).The combined model demonstrated higher discriminatory in assessing the abundance of CD3+ TILs in rectal cancer. Furthermore, the expression of CD3+ TILs was significantly correlated with DFS, highlighting its potential prognostic value.This study is the first attempt to compare the predictive TILs performance of three machine learning models, logistic regression (LR), random forests (RF), and support vector machine (SVM) models, based on the combination of radiomics and immunohistochemistry. MRI-based combined model, composed of radiomics features from SVM model and clinical features from clinical model, exhibited higher discriminatory capability of an abundance of CD3+ TILs in rectal cancer.
Keywords: CD3, Tumor-infiltrating lymphocytes, Radiomics, rectal cancer, machine learning
Received: 10 Oct 2024; Accepted: 26 Mar 2025.
Copyright: © 2025 Ma, Hou, Yang, Wei, Mao, Guan and Zhao. This is an open-access article distributed under the terms of the Creative Commons Attribution License (CC BY). The use, distribution or reproduction in other forums is permitted, provided the original author(s) or licensor are credited and that the original publication in this journal is cited, in accordance with accepted academic practice. No use, distribution or reproduction is permitted which does not comply with these terms.
* Correspondence:
Zhenhua Zhao, Shaoxing Maternal and Child Health Hospital, shaoxing, China
Disclaimer: All claims expressed in this article are solely those of the authors and do not necessarily represent those of their affiliated organizations, or those of the publisher, the editors and the reviewers. Any product that may be evaluated in this article or claim that may be made by its manufacturer is not guaranteed or endorsed by the publisher.
Research integrity at Frontiers
Learn more about the work of our research integrity team to safeguard the quality of each article we publish.