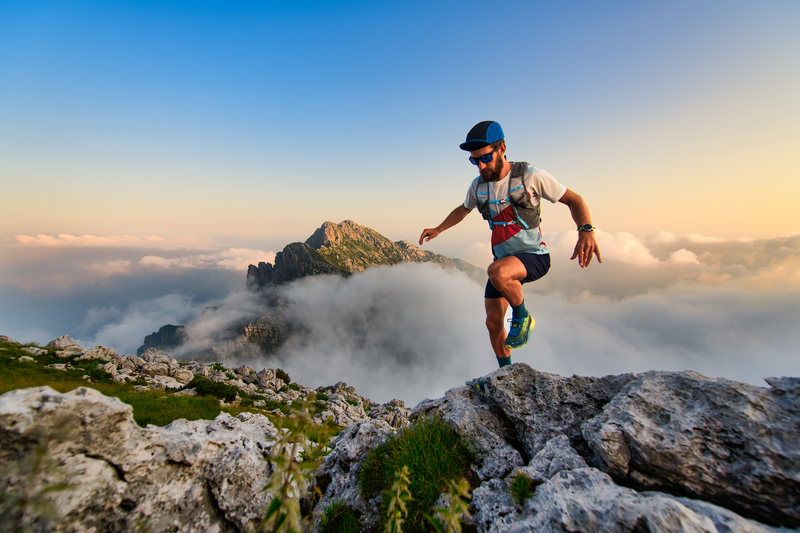
94% of researchers rate our articles as excellent or good
Learn more about the work of our research integrity team to safeguard the quality of each article we publish.
Find out more
ORIGINAL RESEARCH article
Front. Oncol.
Sec. Neuro-Oncology and Neurosurgical Oncology
Volume 15 - 2025 | doi: 10.3389/fonc.2025.1507632
The final, formatted version of the article will be published soon.
You have multiple emails registered with Frontiers:
Please enter your email address:
If you already have an account, please login
You don't have a Frontiers account ? You can register here
Glioblastoma (GBM) is the most common primary brain malignancy. Few neoantigens have been tested in trials as the cancer vaccine against GBM. To better understand the neoantigen landscape and its associated tumor microenvironment (TME) for the optimized vaccine design of our initiated GBM trial, we apply the integrative multi-omics approach to comprehensively profile the mutation, HLA typing, TCR/BCR repertoire, immune cell components on the tumor tissue and peripheral blood mononuclear cell (PMBC) specimen of 24 GBM patients. On average, 148 mutated genes and 200 mutated sites per patient were identified, with no predominant mutated sites and genes in this cohort.Diversified HLA genotypes and expression rate across A, B, and C alleles, with A30:01&A11:01, B13:02, and C06:02, as the most frequent genotypes at respective alleles. Clustered CDR3 of TCR/BCR existed in tumor tissue with decreased richness compared with PMBC. NK and Th1 cells were revealed as the predominant immune cells within the tumor microenvironment (TME).Neoantigens were feasible predicted and designed for each patient, with an average number of 107.Very few neoantigens were shared by more than two patients and no dominant neoantigen could be identified. A minimum of 11-peptide bulk was required to cover this 24-patient cohort, guaranteeing each patient could have at least one neoantigen. In summary, our data reveals a heterogeneous landscape of the neoantigen and its associated immune TME of GBM, based on which a peptide bulk is feasibly developed to cover these patients as a cohort.
Keywords: gliblastoma, neoantigen, Sequencing, heterogeneity, immunotherapy GBM, glioblastoma TME, tumor microenvironment HLA, human leukocyte antigen TCR
Received: 08 Oct 2024; Accepted: 13 Feb 2025.
Copyright: © 2025 Lin, Wei, Xu, Wang, Ling, CHENG and Zhou. This is an open-access article distributed under the terms of the Creative Commons Attribution License (CC BY). The use, distribution or reproduction in other forums is permitted, provided the original author(s) or licensor are credited and that the original publication in this journal is cited, in accordance with accepted academic practice. No use, distribution or reproduction is permitted which does not comply with these terms.
* Correspondence:
Qingtang Lin, Xuanwu Hospital, Capital Medical University, Beijing, China
Disclaimer: All claims expressed in this article are solely those of the authors and do not necessarily represent those of their affiliated organizations, or those of the publisher, the editors and the reviewers. Any product that may be evaluated in this article or claim that may be made by its manufacturer is not guaranteed or endorsed by the publisher.
Research integrity at Frontiers
Learn more about the work of our research integrity team to safeguard the quality of each article we publish.