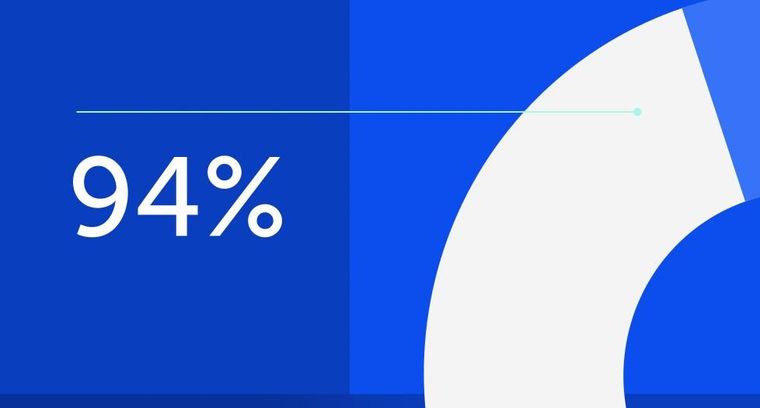
94% of researchers rate our articles as excellent or good
Learn more about the work of our research integrity team to safeguard the quality of each article we publish.
Find out more
SYSTEMATIC REVIEW article
Front. Oncol., 25 March 2025
Sec. Radiation Oncology
Volume 15 - 2025 | https://doi.org/10.3389/fonc.2025.1506778
This article is part of the Research TopicRecent developments in artificial intelligence and radiomicsView all 4 articles
Background: Nasopharyngeal carcinoma (NPC) is a malignant tumor characterized by distinct geographic and pathological features. Enhancing diagnostic accuracy and timeliness in NPC is crucial for clinical implications. Radiomics has demonstrated significant potential in the clinical management of NPC. Nonetheless, a paucity of bibliometric studies has systematically examined the existing literature in th is domain. The objective of this study was to assess the current landscape and project future trends in NPC research.
Methods: This study conducted a search on English-language literature concerning the application of radiomics within the field of nasopharyngeal carcinoma (NPC) research from January 2015 to July 1, 2024, utilizing the Web of Science Core Collection (WoSCC) database. Bibliometric and visual analyses were performed using VOSviewer and CiteSpace software on publications related to countries/regions, authors, journals, references, and keywords.
Results: A total of 311 documents were retrieved, yielding 229 eligible documents after screening, comprising 209 articles and 20 reviews. Annual publications showed an upward trend, while citations revealed a generally declining trend. Notably, China contributed the most publications (n=175). Tian Jie and Dong Di each published 13 papers, and Zhang B was the most frequently co-cited author. Frontiers in Oncology published the most articles (n=25), and the International Journal of Radiation Oncology Biology Physics had the highest citation count (n=331). Sun Yat-sen University led institutional publications (n=39). The radiomics research in NPC focuses on survival prediction, texture analysis, and distant metastasis, and may guide future research directions.
Conclusion: The application of radiomics in NRC is growing annually, as indicated by bibliometric analysis. Radiomics has enhanced the precision of preoperative diagnosis, prediction, and prognosis in NRC. Bibliometric findings offer insights into radiomics research trends. However, creating extensive NPC datasets and bridging the research-to-clinical gap pose significant challenges. Future research should focus on these areas to advance the development.
Nasopharyngeal carcinoma (NPC) is a malignant tumor originating from the epithelial cells of the nasopharynx (1). NPC exhibits significant geographic distribution differences, being predominantly prevalent in southern China, Southeast Asia, and North Africa. In these endemic areas, the pathological type of NPC is mainly the non-keratinizing subtype, which is primarily associated with Epstein-Barr Virus (EBV) infection (2). Over the past three decades, the incidence and prevalence of nasopharyngeal cancer have doubled (3). The comprehensive application of radiotherapy, chemotherapy, and emerging immunotherapy for nasopharyngeal cancer, especially with the use of intensity-modulated radiation therapy and immune checkpoint inhibitors, has significantly improved the therapeutic effect and the survival rate of patients (2, 4).
Medical imaging modalities such as magnetic resonance imaging (MRI), computed tomography (CT), and positron emission tomography (PET) are widely utilized for the early detection, diagnosis, staging, and prognostic evaluation of NPC. Radiomics studies indicate that significant high-dimensional information remains to be discovered (5). Over the past decade, rapid advancements in medical image analysis have facilitated high-throughput feature extraction and analysis, leading to the emergence of radiomics, which aims to obtain quantitative image features to support clinical decision-making (6).
The field of bibliometrics involves examining the statistical data of published materials, such as books, academic papers, and data compilations, along with their associated metadata like summaries, key terms, and reference links, to reveal connections and interactions among various publications (7).This study evaluates the NPC radiomics literature from 2015 to 2024 based on the Web of Science Core Collection (WOSCC) database, analyzing the current status of related research and predicting future directions.
The statistical analysis data of this article comes from WOSCC database.
The data for the statistical analyses in this article were obtained from the WoSCC by searching for the terms (TS=“Nasopharyngeal carcinoma” OR “Nasopharyngeal cancer” OR “NPC”) AND (TS=“Radiomics” OR “Radiogenomics” OR “Imaging genomics”). The detailed search strategy is described in the Supplementary Material. The language was restricted to “English,” and the literature type was confined to Article and Review Article for the period from 2015 to July 1,2024. To ensure the quality of the screened documents, two researchers independently reviewed the documents based on their content. Documents unrelated to the topics of “Nasopharyngeal carcinoma” and “Radiomics” were excluded. After verification, comparison, and de-duplication, 229 documents were retained. The data were exported in the format of a “tab-delimited file,” and the content of the records was selected as “complete records and cited references.” The final exported data included the title, keywords, authors, institutions, addresses, abstracts, and publication dates of each document.
We employed CiteSpace 5.8.R3 (Chaomei Chen, 2006), VOSviewer 1.6.16 (Nees Jan van Eck and Ludo Waltman, 2010), and Microsoft Excel 2019 to conduct bibliometric analysis and visualization. Annual publications were analyzed using Microsoft Excel 2019. Data were retrieved from the Web of Science database on July 1, 2024. CiteSpace, renowned for identifying collaborations, pivotal elements, internal structures, emerging trends, and dynamics within scientific research, was utilized to analyze keywords co-occurrence, timeline, and bursts (8). In CiteSpace visualization, node size corresponds to the frequency of keywords co-occurrence, while links demonstrate the relationships among them. Node and line colors vary by year, transitioning from purple to red from 2015 to July 1,2024. Nodes colored purple with rounded shapes indicate a high median centrality (≥0.10), serving as connectors among various networks (9, 10). VOSviewer specializes in creating and visualizing knowledge graphs that depict clusters, overlays, or density colors, and facilitates the analysis of co-occurrence among countries/regions,authors and co-cited authors, journals and co-cited journals, the dual-map of journals, institutions and co-cited references (11). In cluster maps, node size indicates co-occurrence frequency, uniform colors denote identical clusters, and link thickness reflects the intensity of co-occurrences, directly related to the volume of joint publications or keyword appearances. In density maps, the size and opacity of yellow-colored words and circles correlate positively with citation co-occurrence frequencies. In overlay maps, colors represent the average publication year (12).
The initial review identified 311 relevant papers. Based on the inclusion and exclusion criteria (Figure 1), a total of 229 documents related to the use of radiomics in nasopharyngeal carcinoma were included, comprising 209 articles and 20 review papers. Forty documents were excluded as they were meeting abstracts, editorial materials, and other documents irrelevant to the search content.
A total of 229 publications on the application of radiomics in NPC published between 2015 and July 2024 were retrieved from the WoSCC database.
As shown in Figure 2, the fewest published document were published in 2015 (n=1, 0.4%). Since 2015, the number of publications has steadily increased, peaking in 2022 (n=54, 23.6%). In 2023, there was a slight decrease, with 47 publications published. Publications in 2024 (n=33, 14.4%) was lower than in 2023 (n=47, 20.5%), likely because data extraction was only available up to July 1,2024.
Figure 2. Trend chart of annual publication volume of clinical applications of radiomics in NPC from 2015 to 2024. NPC, nasopharyngeal carcinoma.
The citation frequency fluctuated considerably from year to year, but the overall trend was downward. The lowest citation frequency was in 2015 with 59 times. In 2017 and 2019, citation frequencies were higher, at 935 and 927 times, with a peak in 2017. In 2023 and 2024, citation frequency significantly decreased to 109 and 29 times.
Using VOSviewer software, a cooperation network map of countries (regions) was created (Figure 3). In this map, nodes represent countries (regions), with node sizes corresponding to the number of publications from each country (region), and lines indicating cooperative relationships between countries (regions). The map reveals that China and the United States have larger nodes, each with over 25 publications. Specifically, China has the highest number of publications, totaling 175. The total link strength is notably higher among the United States, Canada, and China, with China exhibiting the highest number of links at 27. This indicates that China has the closest cooperative relationships with other countries.
Figure 3. National (regional) cooperation network map of radiomics applied to NPC research. NPC, nasopharyngeal carcinoma.
A total of 1545 authors contributed to radiomics research on NPC, with 36 authors publishing at least 5 papers (Figure 4A). Tian Jie (N=13) and Dong Di (N=13) published the most papers related to radiomics research on NPC (Figure 4A; Supplementary Material). This was followed by Zhang Lu (N=10), Zhang Shuixing (N=10), and Zhang Bin (N=10). Figure 4A shows seven colors representing seven clusters of authors. Active collaborations usually exist within the same cluster, such as between Tian Jie and Dong Di.
Figure 4. (A) Authors with documents≥5(cluster map). B Co-cited authors with citations≥20(density map).
Co-cited authors are those who are cited together in a single article (13). Out of 5028 co-cited authors, 44 had more than 20 co-citations. Figure 4B presents these authors in a density plot, clearly illustrating the high-frequency co-cited authors. The greater the number of citations, the warmer the color. The size of the words, the size of the circles, and the opacity of the yellow color are positively correlated with co-citation frequency. As shown in Figure 4B, Zhang B (N=143), Lambin P (N=101), and Zhang L (N=91) are the most frequently co-cited authors.
A total of 111 academic journals published articles on radiomics research related to NPC. The top 10 journals published 82 papers, accounting for 35.81% of all publications (Table 1).Frontiers in Oncology led with the highest number of publications (n=25), followed by European Radiology (n=11) and Cancers (n=10).
Among 1616 co-cited sources, 44 journals had >50 citations; among which, International Journal of Radiation Oncology Biology Physics (n=331), Radiotherapy and Oncology(n=329), and Frontiers in Oncology (n=323) had the greatest number of citations. Furthermore, the top 10 co-cited journals accounted for 23.75% citation of all cited sources (Table 1).
To illustrate the distribution of citing and cited journals, a dual-map overlay atlas was utilized. As depicted in Figure 5, journals that cite others are represented on the left side of the geographic map, while those that are cited appear on the right side. The colors of the lines act as indicators for various academic disciplines. Predominantly, The cited journals dealt with molecular, biology, genetics, health, nursing, and medicine, whereas the citing journals mainly dealt with medicine, medical, and clinical
Figure 5. The dual-map overlay of journals on radiomics applied to NPC research. The citing journals are on the left, the cited journals are on the right, and the colored path represents the citation relationship.
A minimum threshold of five publications per institution was established. Using VOSviewer, 20 out of 358 organizations were identified as contributors to the review of institutional co-authorships. Table 2 depicts the top 20 most productive institutions, among which GE Healthcare is the only non-Chinese institution. The institutional co-authorship network, composed of 20 institutions and organized into 5 clusters, is shown in Figure 6A.In recent years, Sun Yat-sen University, Hainan University, and GE Healthcare have emerged as prominent contributors to the field of radiomics in nasopharyngeal carcinoma. This is evident from the overlay map (Figure 6B) of the historical trend for the number of articles.
Table 2. The top 20 institutions by publication volume in the field of nasopharyngeal carcinoma radiomics.
Figure 6. (A) Institutions with documents≥5(cluster map). (B) Institutions with documents≥5(overlay map).
Table 3 spotlights the top 10 articles with the highest citation rates, each of which has been co-cited at least 41 times. The article titled’Radiomics Features of Multiparametric MRI as Novel Prognostic Factors in Advanced Nasopharyngeal Carcinoma’published in the Clin Cancer Res in 2017 was cited the most(N=72). Out of 6489 references, articles were cited more than 20 times are shown in Figure 7. Table 4 presents details of the top five highly cited articles.
CiteSpace was used to construct a keyword co-occurrence map (Figure 8A: N=285, E=599, Table 5).The node size reflects the co-occurrence frequencies, and the links indicate the co-occurrence relationships. The color of node and line represent different years; colors vary from purple to red as time goes from 2015 to 2024, and nodes with purple round mean high betweenness centrality(≥0.1).Images(centrality=0.25), chemotherapy (centrality=0.21), and carcinoma (centrality=0.17) had high centrality, which is presented with a purple circle in Figure 8.
Figure 8. (A) The co-occurrence map of keywords in nasopharyngeal carcinoma radiomics. (B) Top 20 keywords with the strongest citation bursts (sorted by the starting year). The red bars mean citation burstness. (C) The timeline view of keywords related to nasopharyngeal carcinoma radiomics.
The analysis of burst words can uncover prominent research areas within a field over a designated period. As shown in Figure 8B, the keyword visualization view over time shows that the focus of research is gradually shifting from FDG-PET to survival prediction. Further, texture analysis had the strongest bursts (strength=3.8), followed by prediction (strength=2.86) and distant metastasis (strength=2.76).
The timeline view (Figure 8C) depicted the three most frequent keywords (where applicable) in each cluster across various time points. Each cluster is represented by a horizontal line; clusters increase in size with decreasing numbers, with cluster #0 being the largest. Temporal data is displayed at the top, while keywords appear at their initial co-occurrence within the cluster. Cluster labels are derived from title and abstract information using LLR. We could see that six of the twelve clusters are still ongoing. Among them, #0 (cancer) is the biggest cluster, followed by #1 (computed tomography), #2 (adaptive radiotherapy), and #3 (radiomics).
This study analyzed the research literature related to radiomics in the field of NPC from 2015 to July 1, 2024, and found that radiomics research in this area is growing rapidly. Given that the data extraction was completed as of 1 July 2024, it is projected that the annual publication count for 2024 will be 66, and the projected citation frequency is 58. This indicates a general upward trend in the number of publications. There is a possibility that the citation frequency has declined in recent years due to the relatively lower citation rates of recently published articles. Radiomics was first proposed and applied to medical imaging in 2012 but was only gradually applied to NPC starting in 2017 (14). With the rapid development of imaging and artificial intelligence, represented by radiomics, this technology has shown great potential for clinical applications in NPC. The research focus has shifted from extracting macroscopic image features to mining deep image information and extracting large amounts of quantitative data (15, 16). Radiomics provides new auxiliary methods for diagnosis and differential diagnosis, individualized treatment, efficacy evaluation, prognosis prediction, and side effect prediction for NPC patients. From the perspective of research distribution, NPC radiomics research is predominantly conducted in China, the United States, Canada, and several other countries. Among these, China has combined a large number of cases with advanced computer technology to obtain rich clinical data and achieve practical application results. Some studies have shown that the combined application of radiomics and clinical data significantly improves the predictability of NPC compared to using only radiomics or clinical features (17). The studies on the application of radiomics in NPC included in our bibliometric analysis focused on the following topics.
Due to the intricate anatomical structure and high sensitivity to radiotherapy, NPC treatment primarily relies on radiotherapy-based comprehensive approaches. An accurate radiotherapy plan for NPC can enhance therapeutic outcomes, reduce side effects, and improve patients’ quality of life. Therefore, accurate diagnosis of NPC is crucial for the personalized administration of radiotherapy plans.
Feng, Q et al (18) constructed a diagnostic model for clinical assessment of NPC staging by extracting PET/MRI imaging histological features and semi-quantitative parameters. The results indicated that the AUC of the radiomic model in the test set was 0.83 (95% CI: 0.68-0.98), the AUC of the metabolic parameter model was 0.80 (95% CI: 0.63-0.97), and the AUC of the integrated model was 0.90 (95% CI: 0.78-1.00). This study demonstrated that the integrated model based on PET/MRI radiomic features and semi-quantitative parameters is valuable for assessing the clinical staging of NPC, and it is expected to enhance diagnostic accuracy and therapeutic efficacy.
Radiomics offers advantages not only in diagnosing diseases but also in conducting differential diagnoses. Cheng et al (19) compared three combinations of MRI features: T2WI-FS, CE-T1WI, and T2WI-FS+CE-T1WI. They found that the combination of T2WI-FS+CE-T1WI was most effective in distinguishing T1 NPC from nasopharyngeal lymphoid proliferation, with an AUC of 0.940 in the training set, 0.940 in the internal validation set, and 0.940 in the external validation set. The study suggests that MRI features based on radiomics have high accuracy in distinguishing T1 NPC from nasopharyngeal lymphoid hyperplasia, demonstrating significant potential for clinical diagnosis.
Radiotherapy in the early stages of NPC, as well as concurrent radiotherapy in advanced stages, plays a crucial role in evaluating the response and efficacy of NPC treatments, thereby aiding in optimizing clinical decision-making (15, 20).
In the study by Wang, GY et al (21), texture features were extracted from preprocessed MRI images, and histological imaging features were constructed using the LASSO logistic regression model. The study found that combining features from multiple imaging sequences, including T1WI, T2WI, T2WI FS, and CE T1WI, resulted in histological imaging features with an AUC value of 0.822 (95% CI 0.809-0.835), a sensitivity of 0.980, a specificity of 0.529, a PPV of 0.593, and an NPV of 0.949, which were significantly higher than those of CE T1WI alone. Radiomics successfully predicted early response to induction chemotherapy in NPC patients, demonstrating significant potential for clinical application.
In a study by Liu, J et al (22), texture analysis of three MRI sequences—T1W, T2W, and DWI—was compared, and k-nearest neighbor (kNN) and artificial neural network (ANN) classification algorithms were used to construct the models. The results demonstrated that DWI sequences, with an accuracy of 0.881 (ANN) and 0.929 (kNN), were the most effective in predicting the response of NPC patients to chemoradiotherapy. The external validation results were consistent with the internal validation, further supporting these findings. Radiomics shows potential in predicting treatment response, contributing to the development of personalized treatment plans.
Radiomics has also shown particular success in predicting chemotherapy response in NPC. A multi-MRI radiomics nomogram developed by Zhao et al. (23)could distinguish responders to induction chemotherapy (IC) from non-responders with high accuracy. This model, which integrated texture features from T1, T2, and contrast-enhanced MRI with clinical factors, significantly outperformed a clinical model (validation C-index 0.863 vs 0.549) in forecasting IC response and survival. Clinically, patients predicted as responders had markedly better 3-year progression-free survival (PFS) (84.81% vs 39.75%), validating that the radiomics-driven stratification corresponded to real outcome differences. Similarly, Piao et al. (24) built a logistic radiomics model from pretreatment MRI to predict neoadjuvant chemotherapy efficacy. They identified two key texture features whose combination achieved an AUC of 0.905 in differentiating chemo-sensitive versus resistant tumors. These studies underscore radiomics’ impact in guiding chemotherapeutic decision-making, sparing patients from ineffective regimens and improving personalized care.
NPC typically has a favorable prognosis, with a five-year survival rate of approximately 80% (25). Prognostic evaluation is a critical component in patient management, with accurate predictions guiding treatment intensity, thus minimizing the risk of recurrence and mortality (26).
Zhang et al. (27) developed a nomogram for predicting PFS in advanced NPC patients using LASSO regression, incorporating imaging histologic features from T2-weighted and contrast-enhanced T1-weighted MRI scans. Their model, which integrated radiomics with the TNM staging system, significantly outperformed TNM staging alone, exhibiting strong predictive performance across training and validation cohorts. Similarly, Ming et al. (28) constructed three lymph node-based prediction models using MRI-derived imaging histologic features and found that the model based on the maximal slices of the largest lymph node (LSLN) achieved superior performance in predicting overall survival. The combined model, which incorporated clinical risk factors, further improved predictive accuracy, surpassing both standalone radiomics and clinical models. These studies underscore the potential of radiomics in refining prognostic assessment and enhancing risk stratification in NPC patients.
The application of radiomics in NPC prognosis prediction extends to targeted therapy, where it has been explored as a tool to optimize patient selection and improve treatment outcomes. In a study of advanced NPC patients, a radiomics-based nomogram was developed that incorporated the use of nimotuzumab (an anti-EGFR monoclonal antibody) as a variable alongside MRI radiomic features. The combined model (radiomics + clinical factors + targeted therapy status) improved PFS prediction compared to radiomics alone, suggesting that imaging features can help identify which patients derive benefit from EGFR-targeted treatment (29). Immunotherapy is an evolving area in NPC, and radiomics is beginning to impact immune-related patient stratification. Lin et al. (30) developed an MRI-based radiomic signature in a large cohort of recurrent NPC patients that not only predicted overall survival, but was also associated with tumor immune heterogeneity. High-risk patients defined by the radiomics model had significantly worse survival, and gene expression analysis revealed that their tumors showed lower interferon activation and reduced lymphocyte infiltration.
Radiomics will be integrated into the design of radiotherapy protocols for nasopharyngeal carcinoma to achieve individualized, clinically adapted precision radiotherapy (31, 32). However, the majority of research remains at the stage of retrospective analysis or small-scale prospective trials, and the translation of radiomics models into clinical practice faces several challenges. One major issue is the lack of data standardization and quality control, as significant variations in equipment, scanning protocols, imaging parameters, and storage formats across different hospitals often lead to inconsistent model performance when applied in multi-center settings (33). Additionally, many studies lack large-scale multi-center validation or prospective cohort studies, resulting in insufficient evaluation of the reproducibility and stability of radiomics models. Furthermore, the clinical implementation of medical devices or artificial intelligence algorithms requires rigorous regulatory approval and ethical review, which is a time-consuming and complex process (34). To address these challenges, several strategies can be adopted. First, multi-center collaboration and data sharing should be prioritized by establishing unified imaging protocols or data-sharing platforms, such as joining international data alliances or specialized multi-center imaging repositories, to enhance the standardization of research. Second, large-scale, diverse population-based prospective studies should be initiated as early as possible to accumulate stronger evidence-based data, thereby gaining broader recognition in clinical practice. Finally, improving the automation level of radiomics modeling can reduce reliance on manual segmentation, image preprocessing, and other manual steps, thereby minimizing operational errors and enhancing clinical feasibility. By addressing these challenges through collaborative efforts and technological advancements, the potential of radiomics in NPC research and clinical application can be fully realized.
Developing accurate and cost-effective screening procedures remains a major challenge. Numerous studies on NPC imaging predominantly utilize MRI as a standardized dataset. However, different types of medical imaging modalities offer distinct advantages. Therefore, selecting a dataset comprising the appropriate medical images tailored to the specific task is more rational. Research on the radiogenomics of NPC remains sparse.
Indeed, another emerging direction worthy of attention is radiogenomics, a concept introduced in recent years that integrates radiology with genomics (35). Integrating imaging features with tumor genetics and molecular profiles to advance non-invasive, rapid, and cost-effective precision medicine holds significant promise for future clinical applications (36). Therefore, future studies should prioritize multidisciplinary collaboration, integrating radiomics with molecular biology, immunology, and other related fields. Fostering collaboration among radiologists, oncologists, molecular biologists, immunologists, and data scientists is crucial for advancing NPC research. In addition to technological advancements, future research should focus on building robust multidisciplinary research frameworks that facilitate data sharing and collaborative efforts across institutions and countries. Additionally, the treatment of NPC is a prolonged and multi-cycle combined process. Most current studies are based on cross-sectional images. Hence, employing multistage dynamic imaging to evaluate tumor response to chemotherapy or radiotherapy, and to predict the risk of radiotherapy to adjacent vital organs, can enhance the precision and efficacy of treatment decisions (37).
The role of artificial intelligence (AI) in NPC radiomics cannot be overlooked. Recent studies have demonstrated the immense potential of AI, particularly deep learning, in automating feature extraction, improving diagnostic accuracy, and predicting treatment outcomes. For instance, the DeepMTS model proposed by Meng et al. (38) significantly enhances the precision of NPC radiomics analysis by combining PET/CT imaging data and employing a multi-task learning approach for simultaneous tumor segmentation and survival prediction. Similarly, Horowitz DP et al.’s study (39) demonstrates how AI-enhanced CT-guided adaptive radiotherapy improves the accuracy and efficiency of treatment for abdominopelvic tumors by enabling real-time plan adjustments to account for anatomical changes. Additionally, AI technologies, particularly deep learning and CNNs, have shown significant promise in the early diagnosis of thyroid diseases by enhancing the accuracy of ultrasound image analysis and pathological detection (40). However, challenges such as data standardization, model interpretability, and the need for large-scale annotated datasets remain. Future research directions should also explore the development of AI algorithms capable of seamlessly integrating radiomics data with clinical and genomic information, paving the way for more personalized and effective NPC treatment strategies.
The literature included in our study may not comprehensively cover all relevant studies. Firstly, our study exclusively analyzed data from the Web of Science Core Collection (WoSCC) and did not include other critical databases such as PubMed, Embase, and Ovid. This limitation may have led to the omission of valuable research from other databases, especially non-English publications, such as Chinese literature, which could contain important insights on NPC radiomics. Additionally, due to the substantial volume of literature, our analysis was limited to the top-ranked institutions, authors, and journals, precluding a detailed examination of each specific article. This may have resulted in a narrower focus and missed valuable insights from lesser-cited studies or emerging research. Moreover, English predominates as the principal language in scientific research, and restricting our search to English-language publications introduces a language bias. As a result, our findings may not fully reflect all research on NPC radiomics, particularly the studies published in other languages. Furthermore, recently published high-quality articles may not have received the recognition they deserve due to low citation rates, highlighting the need for future updates in the field. Additionally, our inclusion criteria encompassed articles published from 2015 to 1 July 2024. However, ongoing updates to the database precluded the inclusion of the most recent publications. While our study focuses on NPC radiomics, we acknowledge that the field involves cross-disciplinary research with collaboration from molecular targeted therapy, immunotherapy, and radiotherapy, which we did not discuss in detail. This, along with the evolving nature of AI integration in radiomics, could further influence future directions in this research area. Finally, while we identified key trends and future directions in the application of radiomics, the transformation from laboratory research to clinical application, especially in the context of AI, was not explored in-depth, and this remains an important avenue for future research.
Scientific results and research trends regarding NPC radiomics for the period from 2015 to 1 July 2024 were analyzed by bibliometrics and visualization. Radiomics shows great potential for application in various clinical aspects of NPC such as diagnosis and differential diagnosis, efficacy assessment, and prognosis prediction. Radiomics is expected to have a practice-changing impact on the clinical field of NPC. Clinicians can revisit and adjust their diagnostic and treatment strategies based on the latest findings from radiomics studies of nodal NPC, such as incorporating radiomics features for precision diagnosis and individualized treatment plans. However, developing large-scale and comprehensive NPC datasets and overcoming the gap between research and clinical applications remain great challenges. Future research should focus on these areas to advance the development of NPC radiomics and optimize clinical decision-making.
The original contributions presented in the study are included in the article/supplementary material. Further inquiries can be directed to the corresponding authors.
MD: Formal Analysis, Writing – original draft. YL: Data curation, Writing – original draft. LY: Data curation, Validation, Writing – review & editing. CC: Supervision, Writing – review & editing. ZF: Supervision, Writing – review & editing. JD: Data curation, Supervision, Validation, Writing – review & editing.
The author(s) declare that financial support was received for the research and/or publication of this article. The Fujian Province Joint Funds for the Innovation of Science and Technology (2023Y9427, 2023Y9409), the Fujian Provincial Cancer Hospital Talent Development Program (2022YNG02), the Natural Science Foundation of Fujian Province (2021J01441), and the IIT Funds of Fujian Cancer Hospital (202412001), and The Natural Science Foundation of Fujian province (2024J011092).
The authors declare that the research was conducted in the absence of any commercial or financial relationships that could be construed as a potential conflict of interest.
The author(s) declare that no Generative AI was used in the creation of this manuscript.
All claims expressed in this article are solely those of the authors and do not necessarily represent those of their affiliated organizations, or those of the publisher, the editors and the reviewers. Any product that may be evaluated in this article, or claim that may be made by its manufacturer, is not guaranteed or endorsed by the publisher.
The Supplementary Material for this article can be found online at: https://www.frontiersin.org/articles/10.3389/fonc.2025.1506778/full#supplementary-material
NPC, Nasopharyngeal carcinoma; TS, Topic search; WOSCC, Web of Science Core Collection.
1. Tang L-L, Chen Y-P, Chen C-B, Chen M-Y, Chen N-Y, Chen X-Z, et al. The Chinese Society of Clinical Oncology (CSCO) clinical guidelines for the diagnosis and treatment of nasopharyngeal carcinoma. Cancer Commun (Lond). (2021) 41:1195–227. doi: 10.1002/cac2.12218
2. Chen Y-P, Chan ATC, Le Q-T, Blanchard P, Sun Y, Ma J. Nasopharyngeal carcinoma. Lancet. (2019) 394:64–80. doi: 10.1016/S0140-6736(19)30956-0
3. Huang G, Wang Y, Qin B, Zeng J, Tan H, Zhang D, et al. Global, regional and national burdens of Nasopharynx cancer in the adolescents and young adults from 1990 to 2019 and its predictions. BMC Cancer. (2024) 24:720. doi: 10.1186/s12885-024-12480-7
4. Chan ATC, Grégoire V, Lefebvre J-L, Licitra L, Hui EP, Leung SF, et al. Nasopharyngeal cancer: EHNS-ESMO-ESTRO Clinical Practice Guidelines for diagnosis, treatment and follow-up. Ann Oncol. (2012) 23 Suppl 7:vii83–85. doi: 10.1093/annonc/mds266
5. Duan W, Xiong B, Tian T, Zou X, He Z, Zhang L. Radiomics in nasopharyngeal carcinoma. Clin Med Insights Oncol. (2022) 16:11795549221079186. doi: 10.1177/11795549221079186
6. Gillies RJ, Kinahan PE, Hricak H. Radiomics: images are more than pictures, they are data. Radiology. (2016) 278:563–77. doi: 10.1148/radiol.2015151169
7. Broadus RN. Toward a definition of “bibliometrics. Scientometrics. (1987) 12:373–9. doi: 10.1007/BF02016680
8. Chen C. Searching for intellectual turning points: Progressive knowledge domain visualization. Proc Natl Acad Sci USA. (2004) 101:5303–10. doi: 10.1073/pnas.0307513100
9. Chen C, Hu Z, Liu S, Tseng H. Emerging trends in regenerative medicine: a scientometric analysis in CiteSpace. Expert Opin Biol Ther. (2012) 12:593–608. doi: 10.1517/14712598.2012.674507
10. Chen C. Science mapping: A systematic review of the literature. J Data Inf Sci. (2017) 2:1–40. doi: 10.1515/jdis-2017-0006
11. Van Eck NJ, Waltman L. Software survey: VOSviewer, a computer program for bibliometric mapping. Scientometrics. (2010) 84:523–38. doi: 10.1007/s11192-009-0146-3
12. van Eck NJ, Waltman L. VOSviewer manual. (2022). Available at: https://www.vosviewer.com/documentation/Manual_VOSviewer_1.6.18.pdf (Accessed July 10, 2024).
13. Li C, Wu K, Wu J. A bibliometric analysis of research on haze during 2000–2016. Environ Sci pollut Res. (2017) 24:24733–42. doi: 10.1007/s11356-017-0440-1
14. Lambin P, Rios-Velazquez E, Leijenaar R, Carvalho S, van Stiphout RGPM, Granton P, et al. Radiomics: extracting more information from medical images using advanced feature analysis. Eur J Cancer. (2012) 48:441–6. doi: 10.1016/j.ejca.2011.11.036
15. Zhang Y-M, Gong G-Z, Qiu Q-T, Han Y-W, Lu H-M, Yin Y. Radiomics for diagnosis and radiotherapy of nasopharyngeal carcinoma. Front Oncol. (2021) 11:767134. doi: 10.3389/fonc.2021.767134
16. Gillies RJ, Kinahan PE, Hricak H. Radiomics: images are more than pictures, they are data. Radiology. (2016) 278:563–77. doi: 10.1148/radiol.2015151169
17. Khongwirotphan S, Oonsiri S, Kitpanit S, Prayongrat A, Kannarunimit D, Chakkabat C, et al. Multimodality radiomics for tumor prognosis in nasopharyngeal carcinoma. PloS One. (2024) 19:e0298111. doi: 10.1371/journal.pone.0298111
18. Feng Q, Liang J, Wang L, Ge X, Ding Z, Wu H. A diagnosis model in nasopharyngeal carcinoma based on PET/MRI radiomics and semiquantitative parameters. BMC Med Imaging. (2022) 22:150. doi: 10.1186/s12880-022-00883-6
19. Cheng J, Su W, Wang Y, Zhan Y, Wang Y, Yan S, et al. Magnetic resonance imaging based on radiomics for differentiating T1-category nasopharyngeal carcinoma from nasopharyngeal lymphoid hyperplasia: a multicenter study. Jpn J Radiol. (2024) 42:709–19. doi: 10.1007/s11604-024-01544-0
20. Yang C, Jiang Z, Cheng T, Zhou R, Wang G, Jing D, et al. Radiomics for predicting response of neoadjuvant chemotherapy in nasopharyngeal carcinoma: A systematic review and meta-analysis. Front Oncol. (2022) 12:893103. doi: 10.3389/fonc.2022.893103
21. Wang G, He L, Yuan C, Huang Y, Liu Z, Liang C. Pretreatment MR imaging radiomics signatures for response prediction to induction chemotherapy in patients with nasopharyngeal carcinoma. Eur J Radiol. (2018) 98:100–6. doi: 10.1016/j.ejrad.2017.11.007
22. Liu J, Mao Y, Li Z, Zhang D, Zhang Z, Hao S, et al. Use of texture analysis based on contrast-enhanced MRI to predict treatment response to chemoradiotherapy in nasopharyngeal carcinoma. J Magn Reson Imaging. (2016) 44:445–55. doi: 10.1002/jmri.25156
23. Zhao L, Gong J, Xi Y, Xu M, Li C, Kang X, et al. MRI-based radiomics nomogram may predict the response to induction chemotherapy and survival in locally advanced nasopharyngeal carcinoma. Eur Radiol. (2020) 30:537–46. doi: 10.1007/s00330-019-06211-x
24. Yongfeng P, Chuner J, Lei W, Fengqin Y, Zhimin Y, Zhenfu F, et al. The usefulness of pretreatment MR-based radiomics on early response of neoadjuvant chemotherapy in patients with locally advanced nasopharyngeal carcinoma. Oncol Res. (2021) 28:605–13. doi: 10.3727/096504020X16022401878096
25. Lee AWM, Ng WT, Chan LLK, Hung WM, Chan CCC, Sze HCK, et al. Evolution of treatment for nasopharyngeal cancer–success and setback in the intensity-modulated radiotherapy era. Radiother Oncol. (2014) 110:377–84. doi: 10.1016/j.radonc.2014.02.003
26. Li S, Wan X, Deng Y-Q, Hua H-L, Li S-L, Chen X-X, et al. Predicting prognosis of nasopharyngeal carcinoma based on deep learning: peritumoral region should be valued. Cancer Imaging. (2023) 23:14. doi: 10.1186/s40644-023-00530-5
27. Zhang B, Tian J, Dong D, Gu D, Dong Y, Zhang L, et al. Radiomics features of multiparametric MRI as novel prognostic factors in advanced nasopharyngeal carcinoma. Clin Cancer Res. (2017) 23:4259–69. doi: 10.1158/1078-0432.CCR-16-2910
28. Xie H, Huang W, Li S, Huang M, Luo C, Li S, et al. Radiomics-based lymph nodes prognostic models from three MRI regions in nasopharyngeal carcinoma. Heliyon. (2024) 10:e31557. doi: 10.1016/j.heliyon.2024.e31557
29. Li W-Z, Wu G, Li T-S, Dai G-M, Liao Y-T, Yang Q-Y, et al. Dynamic contrast-enhanced magnetic resonance imaging-based radiomics for the prediction of progression-free survival in advanced nasopharyngeal carcinoma. Front Oncol. (2022) 12:955866. doi: 10.3389/fonc.2022.955866
30. Lin D-F, Li H-L, Liu T, Lv X-F, Xie C-M, Ou X-M, et al. Radiomic signatures associated with tumor immune heterogeneity predict survival in locally recurrent nasopharyngeal carcinoma. J Natl Cancer Inst. (2024) 116:1294–302. doi: 10.1093/jnci/djae081
31. Li C-H, Wu VW, Chiu G. A dosimetric evaluation on applying RTOG-based and CT/MRI-based delineation methods to brachial plexus in radiotherapy of nasopharyngeal carcinoma treated with helical tomotherapy. Br J Radiol. (2019) 92:20170881. doi: 10.1259/bjr.20170881
32. Manavis J, Sivridis L, Koukourakis MI. Nasopharyngeal carcinoma: the impact of CT-scan and of MRI on staging, radiotherapy treatment planning, and outcome of the disease. Clin Imaging. (2005) 29:128–33. doi: 10.1016/j.clinimag.2004.04.004
33. Denny JC, Collins FS. Precision medicine in 2030-seven ways to transform healthcare. Cell. (2021) 184:1415–9. doi: 10.1016/j.cell.2021.01.015
34. Avanzo M, Stancanello J, El Naqa I. Beyond imaging: The promise of radiomics. Phys Med. (2017) 38:122–39. doi: 10.1016/j.ejmp.2017.05.071
35. Fan M, Xia P, Clarke R, Wang Y, Li L. Radiogenomic signatures reveal multiscale intratumour heterogeneity associated with biological functions and survival in breast cancer. Nat Commun. (2020) 11:4861. doi: 10.1038/s41467-020-18703-2
36. Panayides AS, Pattichis MS, Leandrou S, Pitris C, Constantinidou A, Pattichis CS. Radiogenomics for precision medicine with a big data analytics perspective. IEEE J BioMed Health Inform. (2019) 23:2063–79. doi: 10.1109/JBHI.2018.2879381
37. Li H, Huang W, Wang S, Balasubramanian PS, Wu G, Fang M, et al. Comprehensive integrated analysis of MR and DCE-MR radiomics models for prognostic prediction in nasopharyngeal carcinoma. Vis Comput Ind BioMed Art. (2023) 6:23. doi: 10.1186/s42492-023-00149-0
38. Meng M, Gu B, Bi L, Song S, Feng DD, Kim J. DeepMTS: deep multi-task learning for survival prediction in patients with advanced nasopharyngeal carcinoma using pretreatment PET/CT. IEEE J BioMed Health Inform. (2022) 26:4497–507. doi: 10.1109/JBHI.2022.3181791
39. Horowitz DP, Wang Y-F, Lee A, Riegel AC, Pasetsky J, Elliston C, et al. Practical approach to CT-guided online adaptive radiotherapy for abdominopelvic tumors. Pract Radiat Oncol. (2025). doi: 10.1016/j.prro.2025.02.011
Keywords: nasopharyngeal carcinoma, radiomics, bibliometrics, prognosis prediction, clinical decision-making
Citation: Deng M, Lin Y, Yan L, Chen C, Fei Z and Ding J (2025) A bibliometric analysis of nasopharyngeal carcinoma radiomics: trends and insights. Front. Oncol. 15:1506778. doi: 10.3389/fonc.2025.1506778
Received: 06 October 2024; Accepted: 10 March 2025;
Published: 25 March 2025.
Edited by:
Luca Urso, University of Ferrara, ItalyReviewed by:
Xiaotao Zhang, The Affiliated Qingdao Central Hospital of Qingdao University, ChinaCopyright © 2025 Deng, Lin, Yan, Chen, Fei and Ding. This is an open-access article distributed under the terms of the Creative Commons Attribution License (CC BY). The use, distribution or reproduction in other forums is permitted, provided the original author(s) and the copyright owner(s) are credited and that the original publication in this journal is cited, in accordance with accepted academic practice. No use, distribution or reproduction is permitted which does not comply with these terms.
*Correspondence: Zhaodong Fei, ZmVpemhhb2RvbmdAZmptdS5lZHUuY24=; Jianming Ding, ZGptXzE5OTFAZmptdS5lZHUuY24=
Disclaimer: All claims expressed in this article are solely those of the authors and do not necessarily represent those of their affiliated organizations, or those of the publisher, the editors and the reviewers. Any product that may be evaluated in this article or claim that may be made by its manufacturer is not guaranteed or endorsed by the publisher.
Research integrity at Frontiers
Learn more about the work of our research integrity team to safeguard the quality of each article we publish.