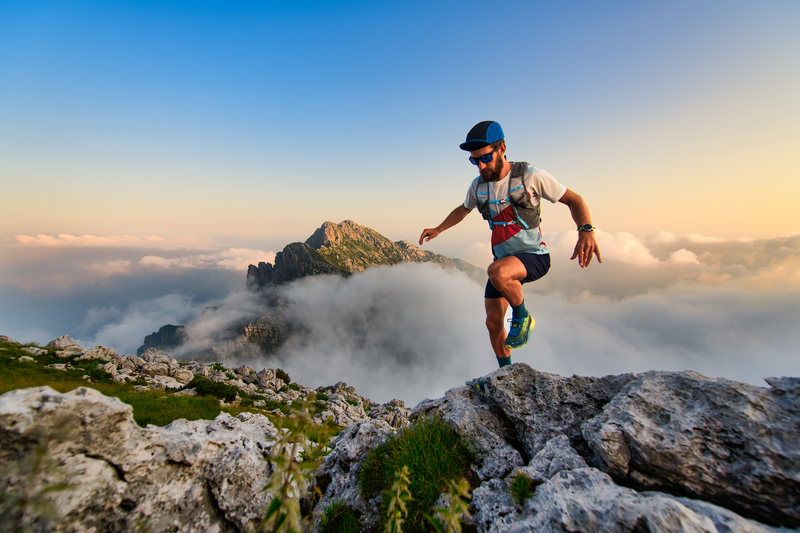
94% of researchers rate our articles as excellent or good
Learn more about the work of our research integrity team to safeguard the quality of each article we publish.
Find out more
ORIGINAL RESEARCH article
Front. Oncol.
Sec. Genitourinary Oncology
Volume 15 - 2025 | doi: 10.3389/fonc.2025.1504132
The final, formatted version of the article will be published soon.
You have multiple emails registered with Frontiers:
Please enter your email address:
If you already have an account, please login
You don't have a Frontiers account ? You can register here
Objective: The purpose of this study was to develop three predictive models utilizing clinical factors, radiomics features, and habitat features, to distinguish between nonclinically significant prostate cancer (csPCa) and clinically significant PCa (non-csPCa) on the basis of biparametricMethods: A total of 175 patients were enrolled, including 134 individuals with csPCa and 41 with non-csPCa. The clinical model was developed using optimal predictive factors obtained from univariable logistic regression and modelled through a random forest approach. Image acquisition and segmentation were performed first in the creation of both the radiomics model and the habitat model. The K-means clustering algorithm was then used exclusively for habitat generation in the development of the habitat model. Finally, feature selection and model construction were performed for both models. Model comparison and diagnostic efficacy assessment were conducted through receiver operating characteristic curve analysis, decision curve analysis (DCA), and calibration curve analysis. Results: The habitat model outperformed both the radiomics model and the clinical model in distinguishing csPCa from non-csPCa patients. The AUC values of the habitat model in the training and test sets were 0.99 and 0.93, respectively. Furthermore, DCA and the calibration curves highlighted the superior clinical utility and greater predictive accuracy of the habitat model in comparison with the other two models. Conclusion: We developed a habitat-based radiomics model with a greater ability to distinguish between csPCa and non-csPCa on the basis of bp-MRI than a traditional radiomics model and to assess the aggressiveness of prostate cancer (PCa) 删除[Mengjuan Li]: clinically significant PCa ( 删除[Mengjuan Li]:
Keywords: habitat, prostate cancer, Classification, aggressiveness, machine learning
Received: 30 Sep 2024; Accepted: 28 Feb 2025.
Copyright: © 2025 Li, Ding, Yin, Lu, Ji and Jin. This is an open-access article distributed under the terms of the Creative Commons Attribution License (CC BY). The use, distribution or reproduction in other forums is permitted, provided the original author(s) or licensor are credited and that the original publication in this journal is cited, in accordance with accepted academic practice. No use, distribution or reproduction is permitted which does not comply with these terms.
* Correspondence:
Yiding Ji, Suzhou Ninth People's Hospital, Suzhou, China
Long Jin, Suzhou Ninth People's Hospital, Suzhou, China
Disclaimer: All claims expressed in this article are solely those of the authors and do not necessarily represent those of their affiliated organizations, or those of the publisher, the editors and the reviewers. Any product that may be evaluated in this article or claim that may be made by its manufacturer is not guaranteed or endorsed by the publisher.
Research integrity at Frontiers
Learn more about the work of our research integrity team to safeguard the quality of each article we publish.