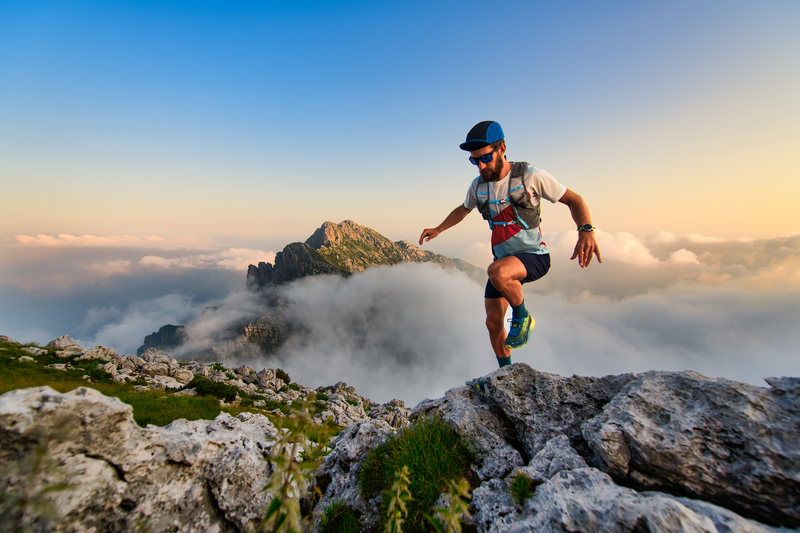
94% of researchers rate our articles as excellent or good
Learn more about the work of our research integrity team to safeguard the quality of each article we publish.
Find out more
ORIGINAL RESEARCH article
Front. Oncol. , 14 February 2025
Sec. Thoracic Oncology
Volume 15 - 2025 | https://doi.org/10.3389/fonc.2025.1502253
Objective: Young patients diagnosed with non-small cell lung cancer (NSCLC) present unique clinical, pathological, and genetic features, resulting in a highly heterogeneous patient population. The current TNM staging system is insufficient for accurately predicting their prognosis. This study aims to develop a nomogram model for survival prediction in young patients with metastatic NSCLC at initial diagnosis and further verify the effectiveness of the model.
Methods: This study enrolled 961 young patients diagnosed with metastatic NSCLC in the Surveillance, Epidemiology, and End Results (SEER) database between 2010 and 2017. The patients were allocated into a training cohort (n = 673) and an internal validation cohort (n = 288). An additional 215 patients from the Fourth Hospital of Hebei Medical University were included as a Chinese external validation cohort. Univariate and multivariate Cox regression analyses were conducted in the training cohort to identify independent risk factors influencing survival, which were used to develop a nomogram model. The model’s effectiveness was evaluated using C-index, calibration curve, receiver operating characteristic (ROC) curve, decision curve analysis (DCA) curve, and Kaplan–Meier survival curve.
Results: The multifactorial Cox regression model identified eight independent risk factors influencing overall survival (OS): race, marital status, histological type, T stage, N stage, liver metastasis, chemotherapy, and radiotherapy (all P < 0.05). These factors were incorporated into the nomogram, which achieved a C-index of 0.673 [95% confidence interval (CI) = 0.661–0.685]. The nomogram exhibited excellent prognostic value in both internal (C-index = 0.662, 95% CI = 0.643–0.681) and external (C-index = 0.724, 95% CI = 0.702–0.746) validation cohorts. In addition, calibration curves for 0.5-,1-, 2-, 3-, and 5-year OS probabilities showed close agreement between predicted and observed survival outcomes across various time points. Additionally, ROC curve analysis and Kaplan–Meier curves highlighted the robust discriminatory power of the model based on survival outcomes. Moreover, the DCA analysis revealed that the incremental net benefit of this model was significantly superior to that of the TNM staging system alone.
Conclusions: A nomogram model has been developed and validated to accurately predict the OS of young patients with metastatic NSCLC at initial diagnosis, demonstrating superior performance compared to the traditional TNM staging system. This model offers valuable guidance for precise predictions and making rational treatment decisions in clinical practice.
Lung cancer remains the leading cause of cancer mortality worldwide (1, 2). Non-small cell lung cancer (NSCLC) accounts for approximately 85% of all lung cancer cases; incidence of NSCLC per 100,000 in the United States has shown a positive trend, dropping from 46.4 in 2010 to 40.9 in 2017 overall. Nevertheless, the incidence of metastatic NSCLC at diagnosis has decreased slightly from 21.7 to 19.6 during the same period (3–5). Metastatic NSCLC demonstrates considerable heterogeneity, even within the same TNM stage, leading to varying survival outcomes, which poses a challenging issue in clinical decision-making. Due to the low incidence of lung cancer in young people, screening and early detection strategies often focus more on older populations with higher risk. Most previous studies have been single-center, small-sample, and retrospective studies, leading to a lack of consensus on the prognosis of young lung cancer. Some studies suggest that young and elderly lung cancer patients have similar prognosis, while others propose that younger patients may have better prognosis despite advanced stage due to their superior physical condition and ability to tolerate more treatment options (6–9).
Nomograms are widely utilized tools for prognostic estimation in the fields of oncology and medicine. By integrating a variety of prognostic and determinant variables, nomograms can generate an individualized numerical probability of clinical events. This capability addresses the need for models that combine biological and clinical factors, aligning with the goals of personalized medicine and enhancing the precision of patient care (10, 11). Previous studies have indicated that nomograms provide more accurate survival predictions than traditional TNM staging in early stage NSCLC (12, 13). However, there has been no prognostic model specifically developed or validated for young patients with metastatic NSCLC at initial diagnosis. There is an urgent need for research on epidemiological characteristics, treatment options, and prognostic evaluation of young patients with metastatic NSCLC.
This study utilized the Surveillance, Epidemiology, and End Results (SEER) database of the National Cancer Institute of the United States (14) to investigate the clinical features and prognostic factors of young patients with metastatic NSCLC at initial diagnosis. It also introduces and evaluates the validity of a novel prognostic nomogram model, aiming to enhance understanding, clarify prognostic factors, and optimize treatment approaches for this demographic.
Patients were selected from 17 population-based cancer registries within the SEER database (http://seer.cancer.gov/). The SEER*Stat program v8.4.3 (seer.cancer.gov/seerstat) facilitated the extraction of lung cancer patient data. Total of 961 patients diagnosed with metastatic NSCLC from 2010 to 2017, aged ≤40 years, were included based on specific criteria (1): diagnostic year between 2010 and 2017 (2), ICD-O-3/WHO 2008 site recode for lung and bronchus (3), 7th Edition Stage Group Recode: IV, and (4) age at diagnosis: ≤40 years. Exclusion criteria were (1) the pathological type was SCLC (2), incomplete clinical information (3), survival time of less than one month, and (4) missing or unknown data on variables such as age at diagnosis, race, histological type, survival status, and survival time (Figure 1).
These patients were randomly divided into training and internal validation cohorts with a bootstrapping technique in 7:3 ratio. Additionally, 215 patients with metastatic NSCLC initial diagnosed in youth from January 2013 to December 2023 at the Fourth Hospital of Hebei Medical University served as an external validation cohort. Inclusion criteria for this cohort were (1) NSCLC confirmed by histopathology (2), initial diagnosis of stage IV at the Fourth Hospital of Hebei Medical University (3), age ≤ 40 years, and (4) complete general examination and clinicopathological data. Exclusion criteria included (1) a history of other malignant tumors (2); hematological, immune system, or infectious diseases (3); prior anti-tumor treatments (4); discontinuation of treatment during hospitalization; or (5) survival time of less than 1 month.
The following variables were extracted: “Age recode with <1 year olds,” “Sex,” “Race recode (White, Black, Other),” “Marital status at diagnosis,” “Laterality,” “Primary Site–labeled,” “ Histologic Type ICD-O-3,” “Derived AJCC T, 7th ed (2010–2015).,” “Derived AJCC N, 7th ed (2010–2015).,” “Derived AJCC M, 7th ed (2010–2015).,” “Derived AJCC T, 7th ed (2016–2017).,” “Derived AJCC N, 7th ed (2016–2017).,” “Derived AJCC M, 7th ed (2016–2017).,” “SEER Combined Mets at DX-bone (2010+),” “SEER Combined Mets at DX-brain (2010+),” “SEER Combined Mets at DX-liver (2010+),” “SEER Combined Mets at DX-lung (2010+),” “Chemotherapy recode,” “Radiation recode,” “Vital status recode,” “Survival months.” In the analysis, patients were classified by age using a recode for ages ≤ 40 years. Continuous variables like age at diagnosis were converted into categorical variables, grouping patients into ≤35 years old or >35 years old.
Using univariate Cox proportional hazards regression model, potential risk factors in the training cohort were analyzed to determine the hazard ratio (HR) and 95% CI for each risk factor. Significant risk factors with P < 0.05 were subsequently included in the Cox multivariable regression analysis. Statistically significant factors (P < 0.05) in the multivariable analysis were utilized to construct a nomogram for survival probability of young metastatic NSCLC patients at 0.5-, 1-, 2-, 3-, and 5-year time points.
The discriminative ability and calibration of the chart were assessed using an internal training cohort and an external validation cohort. Model performance was evaluated based on the C-index (ranging from 0.5 for no predictive discriminative ability to 1.0 for perfect discriminative ability), with a value greater than 0.7 indicating reliable discriminative ability. The calibration curve compares predicted probability with observed results, ideally aligning along the 45° line to indicate perfect calibration. The receiver operating characteristic (ROC) curve and area under the curve (AUC) values at 0.5, 1, 2, 3, and 5 years quantify the model’s discriminative ability. The clinical utility of the chart was compared with the TNM staging system using decision curve analysis (DCA). Kaplan–Meier curves were used to analyze survival differences between high-risk and low-risk groups identified by the chart.
Statistical analyses were conducted with SPSS version 27.0 and R software version 4.3.2, with the determination of optimal thresholds for variables like body mass index (BMI), neutrophil to lymphocyte ratio (NLR), lymphocyte to monocyte ratio (LMR), and platelet to lymphocyte ratio (PLR) were conducted using X-Tile software. Categorical variables were assessed using chi-square test or Fisher’s exact test, while survival curves were compared using the log-rank test. Univariate and multivariate Cox regression analyses identified factors significantly associated with outcomes, with P values < 0.05 deemed statistically significant. Survival plots were generated using R software, and model performance was evaluated utilizing C index, calibration curves, ROC curves, DCA curves, and Kaplan–Meier curves.
In the present study, a total of 390,732 cases of lung cancer were reported in the SEER database from 2010 to 2017. Among these cases, 2,606 patients were aged 40 years or younger, accounting for approximately 0.67% of all lung cancer patients. A total of 1,222 patients out of 2,606 cases were diagnosed with stage IV lung cancer, representing 46.9% of all lung cancers in young patients. Among these, 961 patients were diagnosed with NSCLC. All analytical variables included in the SEER database are listed in Table 1. A total of 961 patients were randomly divided into the training cohort (n = 673) and the internal validation cohort (n = 288). No statistically significant differences were found between the two cohorts in terms of age, gender, race, marital status, side, primary site, histological type, T stage, N stage, M stage, presence of bone, brain, liver, lung metastasis, chemotherapy, and radiotherapy.
Table 1. Demographics and clinicopathological characteristics of the training and internal validation cohort.
Additionally, a cohort of 215 patients diagnosed with metastatic NSCLC from the Fourth Hospital of Hebei Medical University formed the external validation cohort. A comparison of the demographic and clinicopathological characteristics between the SEER cohort and the external validation cohort is presented in Table 2. Notably, all patients in the external validation cohort were Chinese, while they were categorized as “other” in the SEER database. The proportion of married patients was significantly higher in the external validation cohort than in the SEER cohort (P < 0.05), as were the proportions of bilateral lesions, adenocarcinoma, T1 stage, N3 stage, M1a stage, bone metastasis, and patients not receiving chemotherapy or radiotherapy (all P < 0.05). No significant differences were observed between the two cohorts in terms of age, gender, primary site, brain metastasis, liver metastasis, and lung metastasis (all P > 0.05). These discrepancies underscore the robustness of the external validation process.
Table 2. Demographics and clinicopathological characteristics of the external validation and SEER cohort.
The median survival time of these 961 patients was 14 months. 0.5-, 1-, 2-, 3-, and 5-year overall survival (OS) were 74.3%, 55.8%, 35.7%, 26.5%, and 14.7%, respectively. The 961 young patients with metastatic NSCLC from the SEER database in this study were randomly allocated into the training group and the internal validation group. We utilized the Cox proportional hazards regression model to conduct an analysis of the OS within the training cohort (n = 673). Univariate analysis revealed significant associations between gender, race, marital status, primary site, histological type, T stage, N stage, liver metastasis, chemotherapy, and radiotherapy with OS (P < 0.05). Conversely, age, side, M stage, bone metastasis, brain metastasis, and lung metastasis did not show significant associations with OS (P > 0.05) (Table 3). The multivariate model following stepwise regression demonstrated that race, marital status, histological type, T stage, N stage, liver metastasis, chemotherapy, and radiotherapy were significantly associated with survival (all P < 0.05).
Table 3. Univariate and multivariate Cox regression analysis of various factors for predicting OS in the training cohort.
Survival curves were generated using the Kaplan–Meier method for the 16 variables (Figure 2).
This study aimed to develop a nomogram model for young patients with metastatic NSCLC. Each risk factor was assigned a score, and the total score was summed by adding up these scores. Then, the OS rates at 0.5, 1, 2, 3, and 5 years were estimated using this total score, which could be read off the calibration curve on the total score axis. According to the survival prediction plot generated, T stage is the most influential prognostic factor, while marital status has the least impact on prognosis (Figure 3).
Figure 3. Nomogram for predicting the probability of 0.5-, 1-, 2-, 3-, and 5-year OS in young patients with metastatic NSCLC.
The nomogram underwent validation using both an internal (n = 288) and an external (n = 215) validation cohort. Calibration curves were then created for the training, internal validation, and external validation cohorts to evaluate the consistency between predicted and observed OS probabilities at intervals of 0.5, 1, 2, 3, and 5 years (Figure 4). These results demonstrate strong consistency across all cohorts, confirming robust predictive value of this nomogram.
Figure 4. Calibration curves predicting the probability of 0.5-, 1-, 2-, 3-, and 5-year OS in the training (A–E), internal validation (F–J), and external validation cohorts (K–O).
The discriminative ability of the nomogram was assessed using C-index and AUC metrics. In the training cohort, the C-index was 0.673 (95% CI = 0.661–0.685), with 0.5-, 1-, 2-, 3-, and 5-year AUCs of 0.769, 0.737, 0.730, 0.723, and 0.744, respectively. The internal validation cohort showed a C-index of 0.662 (95% CI = 0.643–0.681), and the 0.5-, 1-, 2-, 3-, and 5-year AUCs were 0.782, 0.729, 0.705, 0.695, and 0.657, respectively. The external validation cohort showed a C-index of 0.724 (95% CI = 0.702–0.746), and the 0.5-, 1-, 2-, 3-, and 5-year AUCs were 0.781, 0.852, 0.743, 0.770, and 0.752, respectively, demonstrating substantial predictive accuracy across all cohorts (Figure 5).
Figure 5. ROC curves and AUCs at 0.5-, 1-, 2-, 3-, and 5-year in the training (A), internal validation (B), and external validation cohorts (C).
The DCA revealed that the nomogram exhibited a superior net benefit in predicting survival among young patients with metastatic NSCLC compared to AJCC TNM stage (Figure 6).
Figure 6. DCA of AJCC 7th TNM stage and nomogram for OS of the training (A), internal validation (B), and external validation cohorts (C).
Patients were stratified into high-risk or low-risk group based on the risk scores obtained from the Cox proportional hazards regression model. Significant differences in survival were observed between the high and low-risk groups across the training, internal, and external cohorts, as demonstrated by Kaplan–Meier survival curves (all P < 0.0001) (Figure 7).
Figure 7. Kaplan–Meier curves of OS for risk stratification in the training (A), internal validation (B), and external validation cohorts (C).
The clinicopathologic data as well as treatment details of young metastatic NSCLC patients diagnosed at the Fourth Hospital of Hebei Medical University from 2013 to 2023 were analyzed. The external validation cohort included patients diagnosed with young NSCLC at the Fourth Hospital of Hebei Medical University between January 2013 and December 2023, with follow-up until July 31, 2024. Among them, 19 cases were lost to follow-up, and the follow-up rate was 91.2%. Univariate Cox regression analysis revealed several statistically significant predictors for survival (P < 0.05), including age, gender, marital status, smoking history, drinking history, family history, BMI, gene mutation, TNM staging, CEA, CYFBA, NSE, SCC, D-dimer, white blood cells, neutrophils, lymphocytes, monocytes, red blood cells, hemoglobin, platelets, NLR, LMR, PLR, bone metastasis, brain metastasis, liver metastasis, lung metastasis, chemotherapy, radiotherapy, immunotherapy, and targeted therapy. After Cox regression analysis, factors with P < 0.05 (family history, BMI, gene mutation, NSE, D-dimer, neutrophils, monocytes, NLR, LMR, and targeted therapy) were included in the optimized nomogram (Figure 8). The model showed a C-index of 0.796 (95% CI = 0.778–0.814). The calibration curves confirmed its accuracy (Figure 9). The AUC values for 0.5, 1, 2, 3, and 5 years were 0.863, 0.887, 0.843, 0.873, and 0.838, respectively, indicating superior discriminatory ability compared to the initial nomogram (Figure 10). The DCA curve showed that the optimized nomogram could achieve higher net gains (Figure 11).
Figure 8. Optimized nomogram for predicting the probability of 0.5-, 1-, 2-, 3-, and 5-year OS in young patients with metastatic NSCLC.
Figure 9. Calibration curves predicting the 0.5-, 1-, 2-, 3-, and 5-year OS of patients (A–E) after adding detailed clinical data and hematologic index.
NSCLC in youth is a low-incidence-rate malignancy. First, given the distinct epidemiological characteristics of young metastatic NSCLC patients compared to elderly patients and the limitations of the TNM staging system, it is imperative to utilize a nomogram in establishing a predictive model for risk assessment and survival prognosis. Second, given the relatively small population of young lung cancer patients, we utilized the SEER database to ensure objectivity and credibility in our study. Furthermore, considering variations in ethnicity and genetic background across different populations, we established a Chinese external validation cohort based on our hospital data to validate the model and identify more effective predictive factors and indicators that are more clinically accessible than those available in the SEER database.
In this study, adenocarcinoma accounted for 73.4% of all cases among the patient cohort, indicating a significant majority, with a median survival time of 17 months. The OS rates at 0.5, 1, 2, 3, and 5 years were recorded at 78.3%, 62.1%, 40.3%, 29.5%, and 16.0%, respectively. In contrast, patients diagnosed with squamous cell carcinoma exhibited a median survival time of only 7 months; their corresponding OS rates at the same intervals were found to be 54.7%, 25.3%, 13.3%, 10.7%, and 5.3% (HR = 2.052, P < 0.001). This study underscores that the prognosis for lung adenocarcinoma is significantly more favorable than that for lung squamous cell carcinoma, aligning with previous research findings (15, 16). This may be attributed to a higher prevalence of sensitive gene mutations in adenocarcinoma patients, which provides them with enhanced opportunities for targeted therapy. Previous research has generally suggested that tumor size has a greater impact on the prognosis of early stage lung cancer patients. However, the current study showed that for metastatic NSCLC patients, both tumor size and lymph node metastasis status are independent factors influencing survival. In other words, survival outcomes in young metastatic NSCLC can still vary significantly based on their individual T and N stages. This indicates that even in the presence of distant metastasis, it is important to consider T and N stages when assessing patient prognosis. Liver metastasis, commonly associated with lower OS, occurs in up to 20% of advanced NSCLC cases (17, 18), with an incidence of 21.1% in the training cohort of this study. In this study, the 0.5-, 1-, 2-, 3-, and 5-year survival rate of patients with liver metastasis were 59.2%, 45.8%, 26.1%, 16.9%, and 7.7%, which was significantly lower than that of patients without liver metastasis (76.5%, 59.5%, 37.9, 29.8%, 18.1%) (HR = 1.519, P < 0.001). Young patients are at high risk of liver metastasis and have poorer prognosis (19, 20), potentially due to a more conducive angiogenic microenvironment that promotes tumor growth and metastasis (21).
Chemotherapy is crucial for controlling tumor growth and metastasis, playing a key role in treating advanced lung cancer. This study identified chemotherapy as an independent prognostic factor for young patients with metastatic NSCLC. Patients who received chemotherapy demonstrated significantly higher survival rates (P < 0.001). Furthermore, it has been noted that younger patients with lung cancer often exhibit a more favorable response to aggressive treatments such as chemotherapy and radiotherapy. This may be attributed to their better physical condition and ability to tolerate the side effects of these treatments. Furthermore, younger patients tend to have access to more advanced treatment options, which may significantly enhance their OS prognosis (22). Moreover, the superior enduring capacity of combination therapies in young lung cancer patients may also be ascribed to their stronger immune systems and ability to recover from the toxic effects of treatment. This resilience enables them to adhere to their treatment regimens without significant interruptions, ultimately leading to a higher likelihood of successful outcomes. Therefore, this has sparked our great interest in comparing whether adding local treatment to systemic treatment can improve the survival of late-stage young patients with NSCLC (16, 23). In this study, in young patients with metastatic NSCLC, adding local treatment to systemic treatment did not provide a survival benefit. In a subgroup analysis of the group receiving systemic therapy alone and the group receiving systemic therapy combined with local therapy, we found no significant difference in survival among young patients receiving systemic therapy (HR = 1.002, P = 0.064), regardless of whether local therapy was added. This suggests that local treatment of non-highly screened metastatic NSCLC did not provide a survival benefit. There are two potential explanations for this phenomenon. The first is that radiation therapy technology was largely considered outdated a decade ago, often accompanied by significant side effects that could diminish some of the survival advantages for patients. The second, and more critical, reason is that patients in the IV stage typically have a reduced survival duration; thus, it becomes imperative to rigorously screen candidates and prioritize those with limited metastasis—such as oligometastatic patients—for radiation therapy, which may underscore the efficacy of localized treatment.
Numerous studies have consistently demonstrated a strong correlation between inflammation and the onset as well as prognosis of tumors (24, 25). Neutrophils, lymphocytes, and monocytes play pivotal roles in the inflammatory response (26), with NLR and LMR serving as hematological indicators reflecting the body’s immune status. Elevated NLR values are associated with tumor aggressiveness (27), while increased LMR values indicate enhanced lymphocyte numbers and/or reduced monocyte counts, potentially leading to heightened tumor cell cytotoxicity and inhibition of tumor progression (28, 29). Incorporating survival-related variables and hematological measures from the external validation cohort into the nomogram improved the model’s predictive accuracy, as evidenced by higher C-index and AUC values. The C-index of the nomogram was 0.673, while that of the optimized model improved significantly to 0.796, indicating a substantial enhancement in predictive performance. The AUCs for the nomogram at 0.5-, 1-, 2-, 3-, and 5-year intervals were recorded as 0.769, 0.737, 0.730, 0.723, and 0.744, respectively; conversely, those for the optimized model were notably higher at 0.863, 0.887, 0.843, 0.873, and 0.838, demonstrating a progressive strengthening of predictive efficacy over time. This highlights the importance of further identifying prognostic factors and investigating straightforward, effective hematological markers to more accurately predict young patients with metastatic NSCLC at initial diagnosis.
To the best of our knowledge, this is not only the first nomogram established to predict the survival of young patients with metastatic NSCLC at initial diagnosis based on the SEER database, but it also validates the prediction of this nomogram model through internal and external cohorts. This study also found that in addition to the TNM staging system, hematological indicators also play an important role in predicting OS. However, there are some limitations to our study. First, the SEER database provided limited treatment details, such as chemotherapy regimens and radiation doses, and no genetic mutation information, which could have influenced our results. Second, the model only combines traditional therapeutic approaches and ignores newer ones, such as targeted therapy and immunotherapy. Finally, as a retrospective study, it inherently contains selection bias.
In summary, using the SEER database, this study found that independent prognostic factors in young patients with metastatic NSCLC included race, marital status, histological type, stage T, stage N, liver metastases, chemotherapy, and radiotherapy. Based on this, a prognostic prediction model was established for young patients with metastatic NSCLC at the time of initial diagnosis, which has been verified internally and externally with high prediction accuracy and can provide clinicians with appropriate treatment strategies and prognostic evaluation means.
The raw data supporting the conclusions of this article will be made available by the authors, without undue reservation.
Written informed consent was obtained from the individual(s) for the publication of any potentially identifiable images or data included in this article.
YL: Writing – original draft, Writing – review & editing. LC: Writing – review & editing. YD: Data curation, Writing – review & editing. LL: Writing – review & editing. YZ: Data curation, Investigation, Writing – review & editing. FC: Writing – review & editing.
The author(s) declare financial support was received for the research, authorship, and/or publication of this article. This study was founded by the Medical Science Research Project of Hebei (NO. 20230923; NO. 20180487) and Hebei Medical University Graduate Education Reform Project (NO. YJGZ202405; NO. KCJSZ2025039).
The authors wish to extend their gratitude to all the patients and staff who have contributed to the SEER program. The authors also appreciate the academic support provided by the Fourth Hospital of Hebei Medical University. Thanks to the support of the Medical Science Research Project of Hebei (NO. 20230923; NO. 20180487) and Hebei Medical University Graduate Education Reform Project (NO. YJGZ202405; NO. KCJSZ2025039).
The authors declare that the research was conducted in the absence of any commercial or financial relationships that could be construed as a potential conflict of interest.
The author(s) declare that no Generative AI was used in the creation of this manuscript.
All claims expressed in this article are solely those of the authors and do not necessarily represent those of their affiliated organizations, or those of the publisher, the editors and the reviewers. Any product that may be evaluated in this article, or claim that may be made by its manufacturer, is not guaranteed or endorsed by the publisher.
1. Siegel RL, Giaquinto AN, Jemal A. Cancer statistics, 2024. CA Cancer J Clin. (2024) 74:12–49. doi: 10.3322/caac.21820
2. Adams SJ, Stone E, Baldwin DR, Vliegenthart R, Lee P, Fintelmann FJ. Lung cancer screening. Lancet. (2023) 401:390–408. doi: 10.1016/S0140-6736(22)01694-4
3. Leiter A, Veluswamy RR, Wisnivesky JP. The global burden of lung cancer: current status and future trends. Nat Rev Clin Oncol. (2023) 20:624–39. doi: 10.1038/s41571-023-00798-3
4. Tammemägi MC, Darling GE, Schmidt H, Walker MJ, Langer D, Leung YW, et al. Risk-based lung cancer screening performance in a universal healthcare setting. Nat Med. (2024) 30:1054–64. doi: 10.1038/s41591-024-02904-z
5. Ganti AK, Klein AB, Cotarla I, Seal B, Chou E. Update of incidence, prevalence, survival, and initial treatment in patients with non-small cell lung cancer in the US. JAMA Oncol. (2021) 7:1824–32. doi: 10.1001/jamaoncol.2021.4932
6. Skarin AT, Herbst RS, Leong TL, Bailey A, Sugarbaker D. Lung cancer in patients under age 40. Lung Cancer. (2001) 32:255–64. doi: 10.1016/s0169-5002(00)00233-6
7. Mauri D, Pentheroudakis G, Bafaloukos D, Pectasides D, Samantas E, Efstathiou E, et al. Non-small cell lung cancer in the young: a retrospective analysis of diagnosis, management and outcome data. Anticancer Res. (2006) 26:3175–81.
8. Lara MS, Brunson A, Wun T, Tomlinson B, Qi L, Cress R, et al. Predictors of survival for younger patients less than 50 years of age with non-small cell lung cancer (NSCLC): a California Cancer Registry analysis. Lung Cancer. (2014) 85:264–9. doi: 10.1016/j.lungcan.2014.04.007
9. Subramanian J, Morgensztern D, Goodgame B, Baggstrom MQ, Gao F, Piccirillo J, et al. Distinctive characteristics of non-small cell lung cancer (NSCLC) in the young: a surveillance, epidemiology, and end results (SEER) analysis. J Thorac Oncol. (2010) 5:23–8. doi: 10.1097/JTO.0b013e3181c41e8d
10. Balachandran VP, Gonen M, Smith JJ, DeMatteo RP. Nomograms in oncology: more than meets the eye. Lancet Oncol. (2015) 16:e173–80. doi: 10.1016/S1470-2045(14)71116-7
11. Wu Y, Lv C, Lin M, Hong Y, Du B, Yao N, et al. Novel nomogram for predicting survival in advanced non-small cell lung cancer receiving anti-PD-1 plus chemotherapy with or without antiangiogenic therapy. Front Immunol. (2023) 14:1297188. doi: 10.3389/fimmu.2023.1297188
12. Wo Y, Yang H, Zhang Y, Wo J. Development and external validation of a nomogram for predicting survival in patients with stage IA non-small cell lung cancer ≤2 cm undergoing sublobectomy. Front Oncol. (2019) 9:1385. doi: 10.3389/fonc.2019.01385
13. Wankhede D. Evaluation of eighth AJCC TNM sage for lung cancer NSCLC: A meta-analysis. Ann Surg Oncol. (2021) 28:142–7. doi: 10.1245/s10434-020-09151-9
14. Duggan MA, Anderson WF, Altekruse S, Penberthy L, Sherman ME. The surveillance, epidemiology, and end results (SEER) program and pathology: toward strengthening the critical relationship. Am J Surg Pathol. (2016) 40:e94–e102. doi: 10.1097/PAS.0000000000000749
15. Pan X, Lv T, Zhang F, Fan H, Liu H, Song Y. Frequent genomic alterations and better prognosis among young patients with non-small-cell lung cancer aged 40 years or younger. Clin Transl Oncol. (2018) 20:1168–74. doi: 10.1007/s12094-018-1838-z
16. Rich AL, Khakwani A, Free CM, Tata LJ, Stanley RA, Peake MD, et al. Non-small cell lung cancer in young adults: presentation and survival in the English National Lung Cancer Audit. QJM. (2015) 108:891–7. doi: 10.1093/qjmed/hcv052
17. Riihimäki M, Hemminki A, Fallah M, Thomsen H, Sundquist K, Sundquist J, et al. Metastatic sites and survival in lung cancer. Lung Cancer. (2014) 86:78–84. doi: 10.1016/j.lungcan.2014.07.020
18. Deng Q, Liao Z, Zhu J, Zhou J, Dong S, Jia Y. Establishment of a Nomogram for prognosis of liver metastasis in patients with non-small cell lung cancer. J Clin Med Pract. (2023) 27:18–24. doi: 10.7619/jcmp.20223574
19. Campos-Balea B, de Castro Carpeño J, Massutí B, Vicente-Baz D, Pérez Parente D, Ruiz-Gracia P, et al. Prognostic factors for survival in patients with metastatic lung adenocarcinoma: An analysis of the SEER database. Thorac Cancer. (2020) 11:3357–64. doi: 10.1111/1759-7714.13681
20. Gibson AJW, Li H, D'Silva A, Tudor RA, Elegbede AA, Otsuka SM, et al. Impact of number versus location of metastases on survival in stage IV M1b non-small cell lung cancer. Med Oncol. (2018) 35:117. doi: 10.1007/s12032-018-1182-8
21. Fang Y, Su C. Research progress on the microenvironment and immunotherapy of advanced non-small cell lung cancer with liver metastases. Front Oncol. (2022) 12:893716. doi: 10.3389/fonc.2022.893716
22. Viñal D, Martínez D, Higuera O, de Castro J. Genomic profiling in non-small-cell lung cancer in young patients. A systematic review. ESMO Open. (2021) 6:100045. doi: 10.1016/j.esmoop.2020.100045
23. Lara JD, Brunson A, Riess JW, Kelly K, Lara PN Jr, Gandara DR. Clinical predictors of survival in young patients with small cell lung cancer: Results from the California Cancer Registry. Lung Cancer. (2017) 112:165–8. doi: 10.1016/j.lungcan.2017.08.015
24. Altorki NK, Markowitz GJ, Gao D, Port JL, Saxena A, Stiles B, et al. The lung microenvironment: an important regulator of tumour growth and metastasis. Nat Rev Cancer. (2019) 19:9–31. doi: 10.1038/s41568-018-0081-9
25. Diem S, Schmid S, Krapf M, Flatz L, Born D, Jochum W, et al. M. Neutrophil-to-Lymphocyte ratio (NLR) and Platelet-to-Lymphocyte ratio (PLR) as prognostic markers in patients with non-small cell lung cancer (NSCLC) treated with nivolumab. Lung Cancer. (2017) 111:176–81. doi: 10.1016/j.lungcan.2017.07.024
26. Diakos CI, Charles KA, McMillan DC, Clarke SJ. Cancer-related inflammation and treatment effectiveness. Lancet Oncol. (2014) 15:e493–503. doi: 10.1016/S1470-2045(14)70263-3
27. Proctor MJ, Morrison DS, Talwar D, Balmer SM, Fletcher CD, O'Reilly DS, et al. A comparison of inflammation-based prognostic scores in patients with cancer. A Glasgow Inflammation Outcome Study. Eur J Cancer. (2011) 47:2633–41. doi: 10.1016/j.ejca.2011.03.028
28. Wang Y, Johnson KCC, Gatti-Mays ME, Li Z. Emerging strategies in targeting tumor-resident myeloid cells for cancer immunotherapy. J Hematol Oncol. (2022) 15:118. doi: 10.1186/s13045-022-01335-y
Keywords: young patients, metastatic NSCLC, nomogram, overall survival, prognosis
Citation: Li Y, Cao L, Ding Y, Liu L, Zhu Y and Cao F (2025) Survival prognostic nomogram for young metastatic non-small cell lung cancer: a study of the US SEER database and a Chinese cohort. Front. Oncol. 15:1502253. doi: 10.3389/fonc.2025.1502253
Received: 26 September 2024; Accepted: 20 January 2025;
Published: 14 February 2025.
Edited by:
Carlos Gil Ferreira, Instituto Oncoclínicas, BrazilReviewed by:
Pedro De Marchi, Instituto Oncoclínicas, BrazilCopyright © 2025 Li, Cao, Ding, Liu, Zhu and Cao. This is an open-access article distributed under the terms of the Creative Commons Attribution License (CC BY). The use, distribution or reproduction in other forums is permitted, provided the original author(s) and the copyright owner(s) are credited and that the original publication in this journal is cited, in accordance with accepted academic practice. No use, distribution or reproduction is permitted which does not comply with these terms.
*Correspondence: Feng Cao, NDg1MDEzNjhAaGVibXUuZWR1LmNu
Disclaimer: All claims expressed in this article are solely those of the authors and do not necessarily represent those of their affiliated organizations, or those of the publisher, the editors and the reviewers. Any product that may be evaluated in this article or claim that may be made by its manufacturer is not guaranteed or endorsed by the publisher.
Research integrity at Frontiers
Learn more about the work of our research integrity team to safeguard the quality of each article we publish.