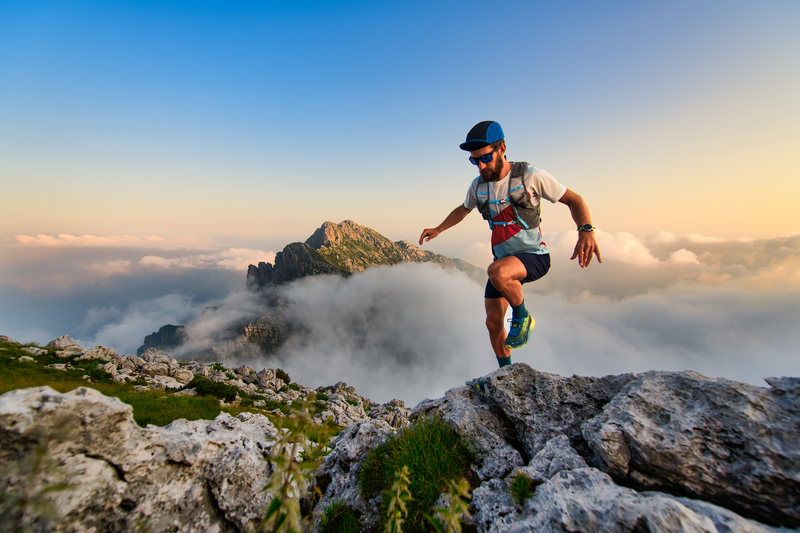
94% of researchers rate our articles as excellent or good
Learn more about the work of our research integrity team to safeguard the quality of each article we publish.
Find out more
ORIGINAL RESEARCH article
Front. Oncol.
Sec. Thoracic Oncology
Volume 15 - 2025 | doi: 10.3389/fonc.2025.1487126
The final, formatted version of the article will be published soon.
You have multiple emails registered with Frontiers:
Please enter your email address:
If you already have an account, please login
You don't have a Frontiers account ? You can register here
Objective: To explore non-small cell lung cancer (NSCLC) patients with new diagnosis of brain metastasis and construct Logistic regression model based on clinical pathology and prognosis score, and verify. Methods: A total of 158 patients newly diagnosed with brain metastasis in NSCLC were retrospectively selected from March 2020 to April 2022. The clinical data of patients were collected, and Logistic regression analysis was used to analyze the influencing factors of poor prognosis for newly diagnosed NSCLC with brain metastasis.The results of univariate analysis showed that the clinical pathological features including NLR>2.94, abnormal CEA, mediastinal lymph node metastasis, symptomatic treatment with therapeutic method, extracranial metastasis and GPS1-2 score were associated with the survival and prognosis of patients with newly diagnosed brain metastasis from NSCLC (P < 0.05). Multivariate Logistic regression analysis showed that NLR>2.94, mediastinal lymph node metastasis, CEA abnormality, extracranial metastasis, and newly diagnosed NSCLC with GPS1-2 score were independent risk factors for poor prognosis of brain metastasis (P < 0.05).Internal verification using the Bootstrap method showed that the predicted curve fitted well with the standard model curve, with the average absolute error of 0.029. The ROC curve result showed that the AUC was 0.887, and the 95%CI was 0.782-0.905, with the corresponding specificity and sensitivity of 90.50% and 80.00%, respectively. This indicates that the prediction accuracy of this Nomogram model is good.: NLR, mediastinal lymph node metastasis, CEA, extracranial metastasis and GPS are risk factors for poor prognosis of newly diagnosed brain metastasis in NSCLC. The risk factor model constructed based on these risk factors has excellent prediction value for the poor prognosis of newly diagnosed brain metastasis in NSCLC. In order to reduce the risk of newly diagnosed brain metastasis in NSCLC and improve the prognosis, targeted preventive measures are taken against the above risk factors in clinical practice.
Keywords: CLINICAL PATHOLOGY, Prognostic score, Non-small cell lung cancer, brain metastases, unfavourable prognosis
Received: 27 Aug 2024; Accepted: 17 Feb 2025.
Copyright: © 2025 Li, Wang and Ma. This is an open-access article distributed under the terms of the Creative Commons Attribution License (CC BY). The use, distribution or reproduction in other forums is permitted, provided the original author(s) or licensor are credited and that the original publication in this journal is cited, in accordance with accepted academic practice. No use, distribution or reproduction is permitted which does not comply with these terms.
* Correspondence:
Guodong Ma, Nanjing Chest Hospital, Nanjing, China
Disclaimer: All claims expressed in this article are solely those of the authors and do not necessarily represent those of their affiliated organizations, or those of the publisher, the editors and the reviewers. Any product that may be evaluated in this article or claim that may be made by its manufacturer is not guaranteed or endorsed by the publisher.
Research integrity at Frontiers
Learn more about the work of our research integrity team to safeguard the quality of each article we publish.