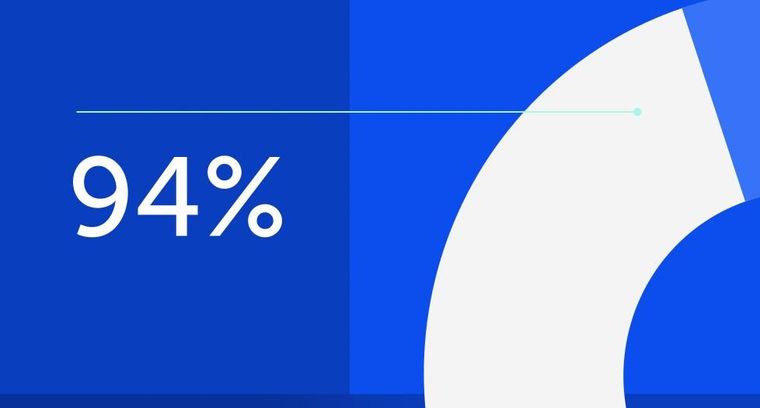
94% of researchers rate our articles as excellent or good
Learn more about the work of our research integrity team to safeguard the quality of each article we publish.
Find out more
ORIGINAL RESEARCH article
Front. Oncol.
Sec. Gynecological Oncology
Volume 15 - 2025 | doi: 10.3389/fonc.2025.1486654
This article is part of the Research TopicAdvancing Cancer Imaging Technologies: Bridging the Gap from Research to Clinical Practice Volume IIView all 6 articles
The final, formatted version of the article will be published soon.
Select one of your emails
You have multiple emails registered with Frontiers:
Notify me on publication
Please enter your email address:
If you already have an account, please login
You don't have a Frontiers account ? You can register here
Background and purpose This study sought to develop an advanced composite model to enhance the prognostic accuracy for cervical cancer patients undergoing concurrent chemoradiotherapy (CCRT). The model integrated imaging features from [18F]FDG PET/CT scans with inflammatory markers using a novel unsupervised two-way clustering approach.MethodsIn this retrospective study, 154 patients diagnosed with primary cervical cancer and treated with CCRT were evaluated using [18F]FDG PET/CT scans. A total of 1,702 radiomic features were extracted from the imaging data. These features underwent rigorous selection based on reproducibility and non-redundancy. The unsupervised two-way clustering method was then employed to simultaneously stratify patients and reduce the dimensionality of features, resulting in the generation of meta-features that were subsequently used to predict overall survival.ResultsKaplan-Meier survival analysis demonstrated that the two-way clustering method successfully stratified patients into distinct risk groups with significant survival differences (P<0.001), outperforming traditional K-means clustering. Predictive models constructed using meta-features derived from two-way clustering showed superior performance compared to those using principal component analysis (PCA), particularly when more than four features were included. The highest C-index values for the COX, COX_Lasso, and RSF models were observed with nine meta-features, yielding results of 0.691±0.026, 0.634±0.018, and 0.684±0.020, respectively. In contrast, models based solely on clinical variables exhibited lower predictive performance, with C-index values of 0.645±0.041, 0.567±0.016, and 0.561±0.033. The combination of clinical data, inflammatory markers, and radiomic features achieved the highest predictive accuracy, with a mean AUC of 0.88 ± 0.07.ConclusionIntegrating radiomic data with inflammatory markers using unsupervised two-way clustering offered a robust approach for predicting survival outcomes in cervical cancer patients. This methodology presented a promising avenue for personalized patient management, potentially leading to more informed treatment decisions and improved outcomes.
Keywords: PET/CT, cervical cancer, Unsupervised machine learning, Concurrent chemoradiotherapy (CCRT), Prognostic prediction
Received: 26 Aug 2024; Accepted: 10 Apr 2025.
Copyright: © 2025 Shi, Wang, Zhou, Ge, ZHANG, Han, Li and Shengming. This is an open-access article distributed under the terms of the Creative Commons Attribution License (CC BY). The use, distribution or reproduction in other forums is permitted, provided the original author(s) or licensor are credited and that the original publication in this journal is cited, in accordance with accepted academic practice. No use, distribution or reproduction is permitted which does not comply with these terms.
* Correspondence:
Jiangqin Han, The First Affiliated Hospital of Soochow University, Suzhou, China
Jihui Li, The First Affiliated Hospital of Soochow University, Suzhou, China
Deng Shengming, The First Affiliated Hospital of Soochow University, Suzhou, China
Disclaimer: All claims expressed in this article are solely those of the authors and do not necessarily represent those of their affiliated organizations, or those of the publisher, the editors and the reviewers. Any product that may be evaluated in this article or claim that may be made by its manufacturer is not guaranteed or endorsed by the publisher.
Supplementary Material
Research integrity at Frontiers
Learn more about the work of our research integrity team to safeguard the quality of each article we publish.