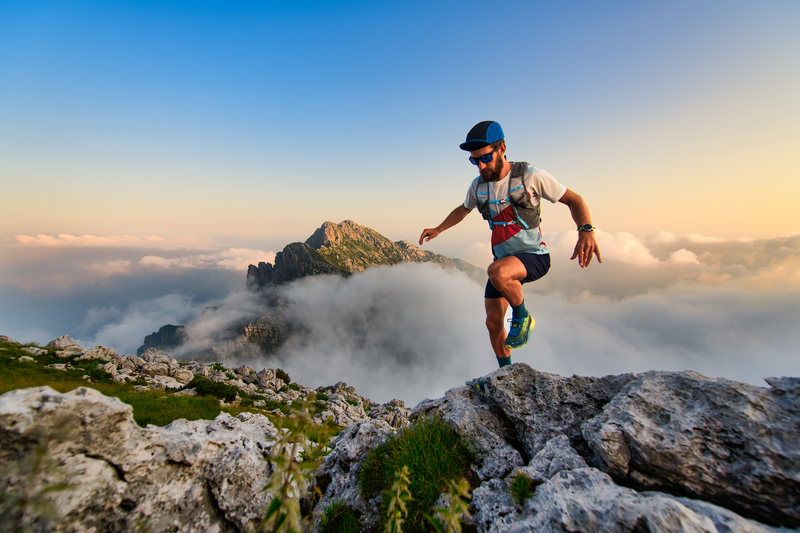
94% of researchers rate our articles as excellent or good
Learn more about the work of our research integrity team to safeguard the quality of each article we publish.
Find out more
ORIGINAL RESEARCH article
Front. Oncol.
Sec. Cancer Epidemiology and Prevention
Volume 15 - 2025 | doi: 10.3389/fonc.2025.1484896
This article is part of the Research Topic The Future of Cancer Surveillance Research View all 25 articles
The final, formatted version of the article will be published soon.
You have multiple emails registered with Frontiers:
Please enter your email address:
If you already have an account, please login
You don't have a Frontiers account ? You can register here
Age-Period-Cohort (APC) model outputs have been used extensively to produce forecasts of cancer incidence, identify emerging public health concerns, and quantify the impact of potential interventions. However, these models have not been extended to forecast cancer prevalence – the number of cancer survivors per capita. Recent advancements in cancer screening and therapeutics have substantially improved survival for many malignancies, leading to an increased need to gauge the future health resource needs of cancer survivors. Concurrent shifts in cancer incidence trends require new methods to identify the separate and joint impacts of incidence and survival changes. In this paper, we formalize methods for forecasting incidence, and introduce novel forecasting methods for prevalence which are highly flexible and interpretable. Our approach has three steps. First, we model cancer incidence trends by age, period, and birth cohort using the New APC Model. Second, we model all-cause mortality by age-at-diagnosis and year-of-diagnosis using flexible regression splines. Third, we estimate cancer prevalence as the convolution of cancer incidence and all-cause mortality, accounting for the need for backward projection of incidence to estimate prevalence during early periods. We illustrate our methods using data on invasive female breast cancer, stratified by Estrogen Receptor status, based on 1992-2019 SEER data. Our analysis illustrates how to calculate the relative impact of period vs. cohort effects on future incidence trends, the contributions of incidence trends and survival trends on future prevalence trends, and total case count estimation.
Keywords: breast cancer, Forecasting, estrogen receptor, Cancer Incidence, Cancer prevalence, Age period cohort, Joinpoint
Received: 22 Aug 2024; Accepted: 21 Feb 2025.
Copyright: © 2025 Best, Filho and Rosenberg. This is an open-access article distributed under the terms of the Creative Commons Attribution License (CC BY). The use, distribution or reproduction in other forums is permitted, provided the original author(s) or licensor are credited and that the original publication in this journal is cited, in accordance with accepted academic practice. No use, distribution or reproduction is permitted which does not comply with these terms.
* Correspondence:
Ana F. Best, Division of Cancer Treatment and Diagnosis, National Cancer Institute (NIH), Bethesda, United States
Disclaimer: All claims expressed in this article are solely those of the authors and do not necessarily represent those of their affiliated organizations, or those of the publisher, the editors and the reviewers. Any product that may be evaluated in this article or claim that may be made by its manufacturer is not guaranteed or endorsed by the publisher.
Research integrity at Frontiers
Learn more about the work of our research integrity team to safeguard the quality of each article we publish.