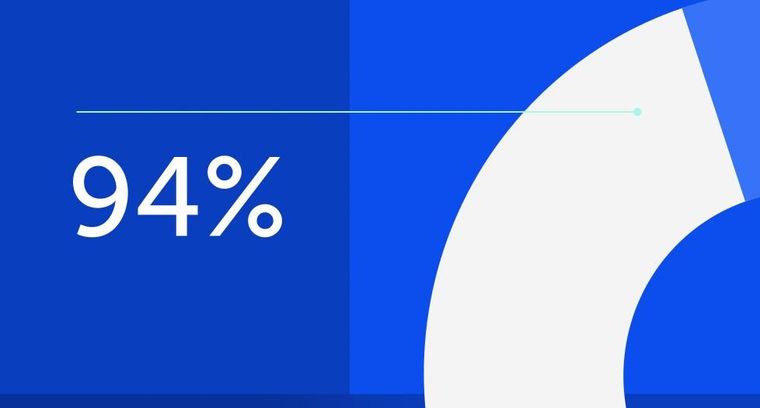
94% of researchers rate our articles as excellent or good
Learn more about the work of our research integrity team to safeguard the quality of each article we publish.
Find out more
SYSTEMATIC REVIEW article
Front. Oncol., 26 February 2025
Sec. Thoracic Oncology
Volume 15 - 2025 | https://doi.org/10.3389/fonc.2025.1424647
This article is part of the Research TopicBridging Surgical Oncology and Personalized Medicine: The Role of Artificial Intelligence and Machine Learning in Thoracic SurgeryView all articles
Introduction: This systematic review and meta-analysis aim to evaluate the efficacy of artificial intelligence (AI) models in identifying prognostic and predictive biomarkers in lung cancer. With the increasing complexity of lung cancer subtypes and the need for personalized treatment strategies, AI-driven approaches offer a promising avenue for biomarker discovery and clinical decision-making.
Methods: A comprehensive literature search was conducted in multiple electronic databases to identify relevant studies published up to date. Studies investigating AI models for the identification of prognostic and predictive biomarkers in lung cancer were included. Data extraction, quality assessment, and meta-analysis were performed according to PRISMA guidelines.
Results: A total of 34 studies met the inclusion criteria, encompassing diverse AI methodologies and biomarker targets. AI models, particularly deep learning and machine learning algorithms demonstrated high accuracy in predicting biomarker status. Most of the studies developed models for the prediction of EGFR, followed by PD-L1 and ALK biomarkers in lung cancer. Internal and external validation techniques confirmed the robustness and generalizability of AI-driven predictions across heterogeneous patient cohorts. According to our results, the pooled sensitivity and pooled specificity of AI models for the prediction of biomarkers of lung cancer were 0.77 (95% CI: 0.72 – 0.82) and 0.79 (95% CI: 0.78 – 0.84).
Conclusion: The findings of this systematic review and meta-analysis highlight the significant potential of AI models in facilitating non-invasive assessment of prognostic and predictive biomarkers in lung cancer. By enhancing diagnostic accuracy and guiding treatment selection, AI-driven approaches have the potential to revolutionize personalized oncology and improve patient outcomes in lung cancer management. Further research is warranted to validate and optimize the clinical utility of AI-driven biomarkers in large-scale prospective studies.
Lung cancer remains one of the most prevalent and lethal malignancies globally, posing significant challenges to public health despite advancements in diagnosis and treatment modalities (1, 2). Despite advances in therapeutic interventions such as targeted therapies and immunotherapy, the overall prognosis for lung cancer remains dismal, emphasizing the critical need for personalized treatment strategies (3). The intricate heterogeneity of lung cancer underscores the necessity for precise prognostic and predictive biomarkers to guide therapeutic strategies and improve patient outcomes (4). Traditional biomarker discovery approaches have been limited by their reliance on small sample sizes, low reproducibility, and insufficient consideration of the complex interactions within the tumor microenvironment (5). In recent years, the integration of artificial intelligence (AI) models has emerged as a promising approach for the identification and validation of biomarkers in various cancers, including lung cancer (6). AI-based methodologies offer a data-driven paradigm capable of analyzing large-scale genomic, transcriptomic, and clinical datasets to uncover novel biomarkers and elucidate underlying biological mechanisms (7, 8). Studies have demonstrated the efficacy of AI algorithms, including machine learning and deep learning techniques, in identifying prognostic biomarkers associated with survival outcomes and predicting treatment response in lung cancer patients (9–11). These methodologies leverage diverse data sources, including gene expression profiles, imaging features, and clinical variables, to generate predictive models with enhanced accuracy and generalizability (12). Despite these advancements, the translation of AI-derived biomarkers into clinical practice necessitates rigorous validation across heterogeneous patient cohorts and consideration of potential confounding factors.
The identification of robust prognostic and predictive biomarkers holds profound implications for optimizing therapeutic decision-making and advancing precision oncology in lung cancer (13). By stratifying patients based on their molecular profiles and risk profiles, clinicians can tailor treatment regimens to individualize care, thereby maximizing efficacy and minimizing toxicity (14). Moreover, prognostic biomarkers offer valuable insights into disease progression and patient prognosis, enabling timely interventions and facilitating patient counseling (15). While numerous studies have explored the potential of AI models for biomarker discovery in lung cancer, several key gaps persist in the existing literature. These include the limited reproducibility of findings across independent cohorts, the lack of consensus regarding optimal feature selection and model validation strategies, and the need for comprehensive meta-analyses to synthesize existing evidence and identify overarching trends. Additionally, the majority of studies have focused on single omics modalities or clinical variables, overlooking the potential synergies arising from integrating multi-omics data and incorporating spatial and temporal heterogeneity. In light of these considerations, the objective of this systematic review and meta-analysis was to comprehensively assess the landscape of AI-driven methodologies for discerning prognostic and predictive biomarkers in lung cancer, thereby elucidating their clinical utility and potential implications. The primary aim of the current review was to examine the performance of AI-driven models mainly focusing on key metrics such as specificity, sensitivity, and accuracy. A meta-analysis was performed of identified studies to assess the overall performance and reproducibility of AI-derived biomarkers and to identify existing challenges and opportunities for future research in this rapidly evolving field. By examining these key performance metrics, we assessed the reliability of these AI models and their potential to serve as non-invasive alternatives to conventional diagnostic methods in healthcare system and outline recommendations and prospects.
This systematic review and meta-analysis were conducted in accordance with the Preferred Reporting Items for Systematic Reviews and Meta-Analyses (PRISMA) guidelines to ensure comprehensive and transparent reporting of the study methodology and findings.
A comprehensive literature search was performed in electronic databases including PubMed/MEDLINE, Embase, Web of Science, Google Scholar, Science direct and Scopus from inception to date. The search strategy utilized a combination of medical subject headings (MeSH) terms and keywords related to “lung cancer,” “biomarkers,” “artificial intelligence,”, “deep learning” and “machine learning.” The search strategy was adapted to the syntax and specifications of each database to maximize sensitivity while maintaining relevance.
Studies were included in the review if they met the following criteria:
1. Investigated the use of AI models for the identification of prognostic or predictive biomarkers in lung cancer.
2. Included human participants diagnosed with lung cancer.
3. Published in English language.
4. Original research articles reporting primary data.
5. Studies with full-text availability.
Studies were excluded if they were:
1. Review articles, editorials, conference abstracts, or letters.
2. Studies focusing solely on non-human subjects.
3. Studies not relevant to the objectives of this review.
Two independent reviewers screened the titles and abstracts of retrieved articles to identify potentially eligible studies. Full-text articles were then assessed for eligibility based on the inclusion and exclusion criteria. Any disagreements between reviewers were resolved through discussion or consultation with a third reviewer.
Data extraction was performed using a standardized data extraction form, including the following information:
1. Study characteristics: authors, publication year, study design, sample size.
2. Patient demographics: age, gender, histological subtype, cancer stage.
3. AI model details: type of AI algorithm, input data types (e.g., genomic, imaging), model performance metrics (sensitivity, specificity, accuracy).
4. Biomarkers identified: prognostic or predictive biomarkers, associated outcomes.
5. Validation methods: internal or external validation, cross-validation techniques.
Outcomes of study: main findings.
The methodological quality and risk of bias of included studies were assessed independently by two reviewers using validated tools appropriate for the study design. Risk of bias was assessed using relevant tools i.e., QUADAS-2 tool. Any discrepancies were resolved through discussion or consultation with a third reviewer. Studies were evaluated based on criteria such as sample representativeness, outcome ascertainment, statistical analysis methods, and reporting transparency (Figure 1).
Figure 1. Quality assessment of included studies using QUADAS-2 tool (42).
A narrative synthesis of included studies was conducted to summarize key findings, including AI model performance, biomarkers identified, and clinical implications. The statistical analyses were carried out using R software (Version 4.3.0, Vienna, Austria). Libraries such as meta and metaphor were used to calculate the key metrics of AI models such as sensitivity, specificity, and accuracy. The random effects model was employed to evaluate the pooled sensitivity and specificity of the AI models that were involved in the prediction and prognosis biomarkers of lung cancer. Additionally, the heterogeneity was assessed among the included articles using the chi-square test and I2 index statistics.
As this study involved the analysis of existing literature, ethical approval was not required. However, ethical principles such as confidentiality and respect for intellectual property rights were upheld throughout the review process.
The PRISMA chart in Figure 2 depicts the process of selecting studies for a systematic review. In this review, the search identified 1,193 records from databases and zero records from registers. After removing duplicates and records ineligible based on automation tools or other reasons, 241 records remained. Of the 241 records, reviewers excluded 66 studies for various reasons. After this process, 175 studies were sought for retrieval, but 73 were not retrieved. This left 102 studies to be assessed for eligibility and studies excluded not being in English, investigating inappropriate interventions, lacking required data, or being review articles. Ultimately, 34 studies were included in the review. Overall, the PRISMA chart demonstrates a rigorous process for selecting studies that met the inclusion criteria for the systematic review.
The Table 1 provides a comprehensive overview of studies employing AI models for the identification of predictive and prognostic biomarkers in lung cancer. The included studies predominantly utilized retrospective designs, with sample sizes ranging from small cohorts of fewer than 100 patients to larger cohorts exceeding 3,000 individuals. The studies primarily focused on non-small cell lung cancer (NSCLC), encompassing various histological subtypes such as adenocarcinoma and squamous cell carcinoma. The biomarkers investigated included Programmed death-ligand 1 (PD-L1), Epidermal growth factor receptor (EGFR), Anaplastic lymphoma kinase (ALK), Kirsten rat sarcoma (KRAS), and others associated with tumor proliferation and therapeutic response. DL models, particularly CNNs, were frequently utilized due to their ability to process complex data such as imaging and genomic profiles. The performance of AI models was evaluated using sensitivity, specificity, and accuracy measures. Overall, the models exhibited high accuracy in predicting biomarker status, with some variability observed across different studies and validation methods. Internal and external validation techniques, including cross-validation and independent testing sets, were employed to assess the generalizability and robustness of AI models. Internal validation within the same dataset was most commonly utilized, followed by external validation using independent datasets. External validation was found to provide stronger evidence of model generalizability, particularly in studies utilizing multicenter or population-based cohorts. Future research should prioritize external validation to confirm that AI models maintain predictive accuracy across diverse patient populations and data sources, thereby increasing their reliability for clinical implementation. The findings underscore the potential of AI-driven approaches in enhancing diagnostic and therapeutic decision-making in lung cancer management. AI models demonstrated significant potential in facilitating non-invasive assessment of biomarker expression, aiding in patient stratification, treatment selection, and prognostic evaluation. By accurately predicting biomarker status using non-invasive methods such as imaging or blood tests, AI models can help clinicians tailor treatment strategies to individual patients, maximizing therapeutic efficacy while minimizing unnecessary interventions and adverse effects. Furthermore, the ability of AI models to analyze large-scale genomic and clinical data sets provides insights into the underlying molecular mechanisms driving tumor progression and treatment response, paving the way for more targeted and personalized approaches to lung cancer management. As such, the integration of AI technologies into clinical practice has the potential to revolutionize patient care by improving diagnostic accuracy, treatment outcomes, and overall survival rates in lung cancer patients.
The forest plots of the pooled sensitivity and specificity of AI-assisted diagnostic system for the identification of biomarkers in lung cancer are presented in Figures 3 and 4. According to our results, the pooled sensitivity and pooled specificity of AI models for the prediction of biomarkers of lung cancer were 0.77 (95% CI: 0.72 – 0.82) and 0.79 (95% CI: 0.78 – 0.84). These findings suggest that AI models such as machine learning and deep learning models exhibit a high level of accuracy for the early detection of prognostic and predictive biomarkers in lung cancer. While heterogeneity was observed across included studies due to variations in study design, sample sizes, and AI model specifications, a formal subgroup analysis was not conducted. Given the diversity in AI model types (e.g., CNN, SVM, ANN), data types (e.g., imaging, genomic), and biomarker targets (e.g., EGFR, PD-L1), subgrouping studies could reduce statistical power and risk overinterpretation of the results. Instead, we qualitatively discussed these factors as sources of variability, offering insights into how they may influence model performance and generalizability. This approach allowed for a more comprehensive understanding without the confounding effects of subgrouping diverse studies.
This systematic review scrutinizes the various methods of AI models for identifying the predictive and prognostic biomarkers in lung cancer. This review particularly focused on studies carried out within the past few years, mainly from 2010 – till date. The primary aim of the current review was to examine the performance of AI-driven models mainly focusing on key metrics such as specificity, sensitivity, and accuracy. By examining these key performance metrics, we assessed the reliability of these AI models and their potential to serve as non-invasive alternatives to conventional diagnostic methods in healthcare system and outline recommendations and prospects. This review reported that AI models for the identification of predictive and prognostic biomarkers in lung cancer demonstrated a high level of accuracy with pooled sensitivity and specificity values of 0.77 (95% CI: 0.72 – 0.82) and 0.79 (95% CI: 0.74 – 0.84), respectively. AI models played a substantial role in the field of cancer research in the existing literature. Most of the included studies have focused mainly on deep learning models (7, 16, 25). Notably, the Convolutional Neural Network (CNN) is the commonly used deep learning model for the identification of predictive and prognostic biomarkers in lung cancer (10, 27, 28). Several studies documented that CNN exhibited strong predictive performance in advanced stages of NSCLC (5, 10). Neural networks (NN) and Artificial Neural Networks (ANN) have also been used extensively in the literature (14, 22, 40). Morphologically, ANN plays a crucial role in differentiating benign from malignant tumor cells and in the identification of pulmonary nodules from computed tomography chest images (43, 44). Apart from Deep learning models, other techniques such as Support vector machine (SVM), Random Forest (RF), and Naïve Bayes are used extensively for the identification of cancer biomarkers (35, 40). Another systematic review reported similar results as the deep learning model was utilized to identify prognostic biomarkers in ovarian cancer (45, 46). Although the use of AI-based models in healthcare settings is promising, the generalizability still depends on the validity. Among 34 included studies, 13 studies performed cross-validation to assess the effectiveness and reliability of the AI model (11, 12, 17, 18, 20, 21, 23, 24, 26, 28, 29, 31, 33, 36, 37, 39, 40).
Most of the studies proposed the AI-based models for the identification of Epidermal growth factor receptor (EGFR) biomarker in non-small cell lung cancer (NSCLC), followed by Programmed death-ligand 1 (PD-L1) and anaplastic lymphoma kinase (ALK). Both machine learning and deep learning models can identify EGFR-mutant patients in various training and validation sets with great accuracy, especially after data optimization (19, 22). Haim et al., extracted the data from the limited number of NSCLC patients, and the DL approach was employed to categorize the patients in accordance with their EGFR mutation status. Lu and his colleagues designed a radiomic-based ML model that exhibited high accuracy in predicting the presence of EGFR T790M mutations using CT images at the time of diagnosis which can aid in targeted treatment planning for NSCLC patients (36). These results provided a sensitivity of 68.7%, a specificity of 97.7%, and a specificity of 89.8% for the identification of a positive EGFR mutation status (17). Moreover, PD-L1 is also considered as a crucial predictive biomarker of NSCLC response to immunotherapy (47). AI-assisted diagnostic models provide a non-invasive procedure to predict high PD-L1 expression of lung cancer and to infer the therapeutic outcomes in response to immunotherapy (9, 12, 48). Therefore, the accurate and efficient procedure for the evaluation of PD-L1 expression is a paramount for developing a reliable predictive marker of response (49). Cheng and his colleagues proposed AI models that exhibit notable key performance metrics such as sensitivity, specificity, and accuracy, particularly at the 1% cut-off value in evaluating the PD-L1 expression in tumor cells (12).
The current studies highlight several future aspects for future exploration in the field of biomarker discovery. One of the aspects involves the development of feature selection approaches that surpass the limitations of existing methods and could help in identifying predictive and prognostic biomarkers correctly (50). Moreover, to improve signature gene identification associated with biomarkers, non-linear methods should be developed that incorporate deep learning algorithms, such as DeepSurv (51). Another aspect involves the recommendation tackling the treatment effects on the basis of biomarker identification that not only improves the current identification methods but also emphasizes on the identification of predictive biomarkers (46). Lastly, incorporating additional independent or external cohorts plays a substantial role in conducting comprehensive evaluation into the progression, diagnosis, and treatment of lung cancer.
The current review has set limitations. Though AI models have marked their significance in the field of lung cancer prediction research, the researchers faced numerous challenges that need to be addressed. One of the common challenges for most of the included studies was inadequate data to train the model. A small sample size was included in the training as well as test dataset which did not authenticate the efficacy of the proposed AI model. Likewise, retrospective data can introduce biases that may not reflect real-world clinical settings, thereby limiting the generalizability of AI models. Additionally, we excluded the studies that were not mainly focused on biomarkers of lung cancer to maintain the quality and reliability of this systematic review. Most of these non-cancer biomarkers were associated with other applications and disorders such as metabolic and cardiovascular disorders, and the detection of these biomarkers demands further investigation. The data from recent studies were extracted so that current technologies should be discussed, and challenges should be addressed. Moreover, our search items were limited to the identification of predictive and prognostic biomarkers in lung cancer. We acknowledge the inclusion criteria in this review may affect the conclusions drawn from the studies included. However, the exclusion criteria were considered carefully by two independent experts. Therefore, this review aimed to focus on the identification of predictive and prognostic biomarkers in lung cancer.
The key takeaways from our review underscore the promising role of AI models in advancing non-invasive assessment of lung cancer biomarkers, with potential to reduce dependency on traditional biopsy methods in certain contexts. While AI models show high sensitivity and specificity in predicting biomarkers like EGFR and PD-L1, their real-world application requires rigorous validation across diverse populations. Our analysis also points to the need for prospective studies and the integration of multi-omics data to enhance model accuracy and clinical relevance. Standardized protocols in AI model development, including uniform definitions for data input and validation metrics, to facilitate comparability across studies. Ultimately, AI models could serve as valuable adjuncts in personalized lung cancer care, improving early detection, treatment planning, and patient outcomes.
This review focused on the application of AI models for identifying the predictive and prognostic biomarkers in lung cancer, mainly emphasizing the use of deep learning (DL) and machine learning (ML) models. Most of the studies developed models for the prediction of EGFR, followed by PD-L1 and ALK biomarkers in lung cancer. The pooled sensitivity and specificity values of 0.77 (95% CI: 0.72 – 0.82) and 0.79 (95% CI: 0.74 – 0.84) showed the potential of AI models for identifying true positive and true negative cases. Despite the observed heterogeneity found, our results highlight the need for the application of AI models in the prediction of biomarkers in lung cancer. Therefore, there is a need for continued research and validation in this field so that healthcare professionals will benefit from the integration of AI models in clinical practice.
The original contributions presented in the study are included in the article/supplementary material. Further inquiries can be directed to the corresponding authors.
HA: Validation, Writing – original draft. AsA: Writing – original draft, Formal analysis. LA-S: Formal analysis, Writing – review & editing. JB: Supervision, Writing – original draft. RSA: Methodology, Writing – review & editing. DA: Methodology, Writing – original draft. AlA: Conceptualization, Writing – review & editing. TA: Writing – review & editing, Methodology. SMA: Validation, Writing – original draft. SAA: Writing – original draft, Methodology. RAA: Methodology, Writing – review & editing. AbA: Methodology, Writing – review & editing, Software, Visualization. WA: Methodology, Writing – review & editing, Data curation, Formal analysis. RHA: Writing – review & editing, Conceptualization, Resources, Supervision. MA: Conceptualization, Writing – review & editing.
The author(s) declare that no financial support was received for the research, authorship, and/or publication of this article.
The authors would like to thank the Research Center at King Fahd Medical City, Riyadh Second Health Cluster, for their valuable technical support provided for the manuscript.
The authors declare that the research was conducted in the absence of any commercial or financial relationships that could be construed as a potential conflict of interest.
All claims expressed in this article are solely those of the authors and do not necessarily represent those of their affiliated organizations, or those of the publisher, the editors and the reviewers. Any product that may be evaluated in this article, or claim that may be made by its manufacturer, is not guaranteed or endorsed by the publisher.
1. Wang Z, Hu L, Li J, Wei L, Zhang J, Zhou J. Magnitude, temporal trends and inequality in global burden of tracheal, bronchus and lung cancer: findings from the Global Burden of Disease Study 2017. BMJ Global Health. (2020) 5:e002788. doi: 10.1136/bmjgh-2020-002788
2. Chaft JE, Rimner A, Weder W, Azzoli CG, Kris MG, Cascone T. Evolution of systemic therapy for stages I–III non-metastatic non-small-cell lung cancer. Nat Rev Clin Oncol. (2021) 18:547–57. doi: 10.1038/s41571-021-00501-4
3. Leiter A, Veluswamy RR, Wisnivesky JP. The global burden of lung cancer: current status and future trends. Nat Rev Clin Oncol. (2023) 20:624–39. doi: 10.1038/s41571-023-00798-3
4. Roointan A, Mir TA, Wani SI, Hussain KK, Ahmed B, Abrahim S, et al. Early detection of lung cancer biomarkers through biosensor technology: A review. J Pharm Biomed Anal. (2019) 164:93–103. doi: 10.1016/j.jpba.2018.10.017
5. Seijo LM, Peled N, Ajona D, Boeri M, Field JK, Sozzi G, et al. Biomarkers in lung cancer screening: achievements, promises, and challenges. J Thorac Oncol. (2019) 14:343–57. doi: 10.1016/j.jtho.2018.11.023
6. Hsu Y-C, Tsai Y-H, Weng H-H, Hsu L-S, Tsai Y-H, Lin Y-C, et al. Artificial neural networks improve LDCT lung cancer screening: a comparative validation study. BMC Cancer. (2020) 20:1023. doi: 10.1186/s12885-020-07465-1
7. Wu J, Liu C, Liu X, Sun W, Li L, Gao N, et al. Artificial intelligence-assisted system for precision diagnosis of PD-L1 expression in non-small cell lung cancer. Modern Pathol. (2022) 35:403–11. doi: 10.1038/s41379-021-00904-9
8. Li X, Hu B, Li H, You B. Application of artificial intelligence in the diagnosis of multiple primary lung cancer. Thorac Cancer. (2019) 10:2168–74. doi: 10.1111/1759-7714.13185
9. Tian P, He B, Mu W, Liu K, Liu L, Zeng H, et al. Assessing PD-L1 expression in non-small cell lung cancer and predicting responses to immune checkpoint inhibitors using deep learning on computed tomography images. Theranostics. (2021) 11:2098–107. doi: 10.7150/thno.48027
10. Mu W, Jiang L, Shi Y, Tunali I, Gray JE, Katsoulakis E, et al. Non-invasive measurement of PD-L1 status and prediction of immunotherapy response using deep learning of PET/CT images. J Immunother Cancer. (2021) 9. doi: 10.1136/jitc-2020-002118
11. Morgado J, Pereira T, Silva F, Freitas C, Negrão E, de Lima BF, et al. Machine learning and feature selection methods for egfr mutation status prediction in lung cancer. Appl Sci. (2021) 11:3273. doi: 10.3390/app11073273
12. Cheng G, Zhang F, Xing Y, Hu X, Zhang H, Chen S, et al. Artificial intelligence-assisted score analysis for predicting the expression of the immunotherapy biomarker PD-L1 in lung cancer. Front Immunol. (2022) 13:893198. doi: 10.3389/fimmu.2022.893198
13. Wang C, Xu X, Shao J, Zhou K, Zhao K, He Y, et al. Deep learning to predict EGFR mutation and PD-L1 expression status in non-small-cell lung cancer on computed tomography images. J Oncol. (2021) 2021. doi: 10.1155/2021/5499385
14. Adetiba E, Ibikunle F. Ensembling of EGFR mutations’ based artificial neural networks for improved diagnosis of non-small cell lung cancer. Int J Comput Appl. (2011) 20:39–47.
15. Hu D, Li X, Lin C, Wu Y, Jiang H. DDeep learning to predict the cell proliferation and prognosis of non-small cell lung cancer based on FDG-PET/CT images. Diagnostics. (2023) 13:3107. doi: 10.3390/diagnostics13193107
16. Tan X, Li Y, Wang S, Xia H, Meng R, Xu J, et al. Predicting EGFR mutation, ALK rearrangement, and uncommon EGFR mutation in NSCLC patients by driverless artificial intelligence: a cohort study. Respir Res. (2022) 23:1–13. doi: 10.1186/s12931-022-02053-2
17. Haim O, Abramov S, Shofty B, Fanizzi C, DiMeco F, Avisdris N, et al. Predicting EGFR mutation status by a deep learning approach in patients with non-small cell lung cancer brain metastases. J Neuro-Oncol. (2022) 157:63–9. doi: 10.1007/s11060-022-03946-4
18. Zhang T, Xu Z, Liu G, Jiang B, de Bock GH, Groen HJM, et al. Simultaneous identification of egfr, kras, erbb2, and tp53 mutations in patients with non-small cell lung cancer by machine learning-derived three-dimensional radiomics. Cancers. (2021) 13:1814. doi: 10.3390/cancers13081814
19. Rossi G, Barabino E, Fedeli A, Ficarra G, Coco S, Russo A, et al. Radiomic detection of EGFR mutations in NSCLC. Cancer Res. (2021) 81:724–31. doi: 10.1158/0008-5472.CAN-20-0999
20. Wang S, Shi J, Ye Z, Dong D, Yu D, Zhou M, et al. Predicting EGFR mutation status in lung adenocarcinoma on computed tomography image using deep learning. Eur Respir J. (2019) 53. doi: 10.1183/13993003.00986-2018
21. Nair JKR, Saeed UA, McDougall CC, Sabri A, Kovacina B, Raidu BVS, et al. Radiogenomic models using machine learning techniques to predict EGFR mutations in non-small cell lung cancer. Can Assoc Radiol J. (2021) 72:109–19. doi: 10.1177/0846537119899526
22. Qin X, Wang H, Hu X, Gu X, Zhou W. Predictive models for patients with lung carcinomas to identify EGFR mutation status via an artificial neural network based on multiple clinical information. J Cancer Res Clin Oncol. (2020) 146:767–75. doi: 10.1007/s00432-019-03103-x
23. Le NQK, Kha QH, Nguyen VH, Chen Y-C, Cheng S-J, Chen C-Y. Machine learning-based radiomics signatures for EGFR and KRAS mutations prediction in non-small-cell lung cancer. Int J Mol Sci. (2021) 22:9254. doi: 10.3390/ijms22179254
24. Hao P, Deng B-Y, Huang C-T, Xu J, Zhou F, Liu Z-X, et al. Predicting anaplastic lymphoma kinase rearrangement status in patients with non-small cell lung cancer using a machine learning algorithm that combines clinical features and CT images. Front Oncol. (2022) 12:994285. doi: 10.3389/fonc.2022.994285
25. Terada Y, Takahashi T, Hayakawa T, Ono A, Kawata T, Isaka M, et al. Artificial intelligence–powered prediction of ALK gene rearrangement in patients with non–small-cell lung cancer. JCO Clin Cancer Inf. (2022) 6:e2200070. doi: 10.1200/CCI.22.00070
26. Ma D-N, Gao X-Y, Dan Y-B, Zhang A-N, Wang W-J, Yang G, et al. Evaluating solid lung adenocarcinoma anaplastic lymphoma kinase gene rearrangement using noninvasive radiomics biomarkers. OncoTargets Ther. (2020), 6927–35. doi: 10.2147/OTT.S257798
27. Mahajan A, Gurukrishna B, Wadhwa S, Agarwal U, Baid U, Talbar S, et al. Deep learning based automated epidermal growth factor receptor and anaplastic lymphoma kinase status prediction of brain metastasis in non-small cell lung cancer. Explor Targeted Anti-tumor Ther. (2023) 4:657. doi: 10.37349/etat
28. Song L, Zhu Z, Mao L, Li X, Han W, Du H, et al. Clinical, conventional CT and radiomic feature-based machine learning models for predicting ALK rearrangement status in lung adenocarcinoma patients. Front Oncol. (2020) 10:369. doi: 10.3389/fonc.2020.00369
29. Chang C, Sun X, Wang G, Yu H, Zhao W, Ge Y, et al. A machine learning model based on PET/CT radiomics and clinical characteristics predicts ALK rearrangement status in lung adenocarcinoma. Front Oncol. (2021) 11:603882. doi: 10.3389/fonc.2021.603882
30. Wang S, Yu H, Gan Y, Wu Z, Li E, Li X, et al. Mining whole-lung information by artificial intelligence for predicting EGFR genotype and targeted therapy response in lung cancer: a multicohort study. Lancet Digital Health. (2022) 4:e309–19. doi: 10.1016/S2589-7500(22)00024-3
31. Shiri I, Maleki H, Hajianfar G, Abdollahi H, Ashrafinia S, Hatt M, et al. Next-generation radiogenomics sequencing for prediction of EGFR and KRAS mutation status in NSCLC patients using multimodal imaging and machine learning algorithms. Mol Imaging Biol. (2020) 22:1132–48. doi: 10.1007/s11307-020-01487-8
32. Dong Y, Hou L, Yang W, Han J, Wang J, Qiang Y, et al. Multi-channel multi-task deep learning for predicting EGFR and KRAS mutations of non-small cell lung cancer on CT images. Quantitative Imaging Med Surg. (2021) 11:2354. doi: 10.21037/qims-20-600
33. Gu Q, Feng Z, Liang Q, Li M, Deng J, Ma M, et al. Machine learning-based radiomics strategy for prediction of cell proliferation in non-small cell lung cancer. Eur J Radiol. (2019) 118:32–7. doi: 10.1016/j.ejrad.2019.06.025
34. Hong R, Liu W, Fenyö D. Predicting and visualizing STK11 mutation in lung Adenocarcinoma histopathology slides using deep learning. BioMedInformatics. (2021) 2:101–5. doi: 10.3390/biomedinformatics2010006
35. Li S, Luo T, Ding C, Huang Q, Guan Z, Zhang H. Detailed identification of epidermal growth factor receptor mutations in lung adenocarcinoma: combining radiomics with machine learning. Med Phys. (2020) 47:3458–66. doi: 10.1002/mp.14238
36. Lu J, Ji X, Liu X, Jiang Y, Li G, Fang P, et al. Machine learning-based radiomics strategy for prediction of acquired EGFR T790M mutation following treatment with EGFR-TKI in NSCLC. Sci Rep. (2024) 14:446. doi: 10.1038/s41598-023-50984-7
37. He R, Yang X, Li T, He Y, Xie X, Chen Q, et al. A machine learning-based predictive model of epidermal growth factor mutations in lung adenocarcinomas. Cancers. (2022) 14:4664. doi: 10.3390/cancers14194664
38. Jia T-Y, Xiong J-F, Li X-Y, Yu W, Xu Z-Y, Cai X-W, et al. Identifying EGFR mutations in lung adenocarcinoma by noninvasive imaging using radiomics features and random forest modeling. Eur Radiol. (2019) 29:4742–50. doi: 10.1007/s00330-019-06024-y
39. Zhao W, Yang J, Ni B, Bi D, Sun Y, Xu M, et al. Toward automatic prediction of EGFR mutation status in pulmonary adenocarcinoma with 3D deep learning. Cancer Med. (2019) 8:3532–43. doi: 10.1002/cam4.2019.8.issue-7
40. Lim CH, Koh YW, Hyun SH, Lee SJA. Machine learning approach using PET/CT-based radiomics for prediction of PD-L1 expression in non-small cell lung cancer. Anticancer Res. (2022) 42:5875–84. doi: 10.21873/anticanres.16096
41. Wang C, Ma J, Shao J, Zhang S, Liu Z, Yu Y, et al. Predicting EGFR and PD-L1 status in NSCLC patients using multitask AI system based on CT images. Front Immunol. (2022) 13:813072. doi: 10.3389/fimmu.2022.813072
42. Whiting PF, Rutjes AWS, Westwood ME, Mallett S, Deeks JJ, Reitsma JB, et al. QUADAS-2: a revised tool for the quality assessment of diagnostic accuracy studies. Ann Internal Med. (2011) 155:529–36. doi: 10.7326/0003-4819-155-8-201110180-00009
43. Flores-Fernández JM, Herrera-López EJ, Sánchez-Llamas F, Rojas-Calvillo A, Cabrera-Galeana PA, Leal-Pacheco G, et al. Development of an optimized multi-biomarker panel for the detection of lung cancer based on principal component analysis and artificial neural network modeling. Expert Syst Appl. (2012) 39:10851–6. doi: 10.1016/j.eswa.2012.03.008
44. Duan X, Yang Y, Tan S, Wang S, Feng X, Cui L, et al. Application of artificial neural network model combined with four biomarkers in auxiliary diagnosis of lung cancer. Med Biol Eng Computing. (2017) 55:1239–48. doi: 10.1007/s11517-016-1585-7
45. Kim M, Oh I, Ahn J. An improved method for prediction of cancer prognosis by network learning. Genes. (2018) 9:478. doi: 10.3390/genes9100478
46. Al-Tashi Q, Saad MB, Muneer A, Qureshi R, Mirjalili S, Sheshadri A, et al. Machine learning models for the identification of prognostic and predictive cancer biomarkers: a systematic review. Int J Mol Sci. (2023) 24:7781. doi: 10.3390/ijms24097781
47. Incorvaia L, Fanale D, Badalamenti G, Barraco N, Bono M, Corsini LR, et al. Programmed death ligand 1 (PD-L1) as a predictive biomarker for pembrolizumab therapy in patients with advanced non-small-cell lung cancer (NSCLC). Adv Ther. (2019) 36:2600–17. doi: 10.1007/s12325-019-01057-7
48. Zhao X, Bao Y, Meng B, Zheng J, Shi M. From rough to precise: PD-L1 evaluation for predicting the efficacy of PD-1/PD-L1 blockades. Front Immunol. (2022) 13:920021. doi: 10.3389/fimmu.2022.920021
49. Gravelle P, Burroni B, Péricart S, Rossi C, Bezombes C, Tosolini M, et al. Mechanisms of PD-1/PD-L1 expression and prognostic relevance in non-Hodgkin lymphoma: a summary of immunohistochemical studies. Oncotarget. (2017) 8:44960. doi: 10.18632/oncotarget.16680
50. Al-Tashi Q, Abdulkadir SJ, Rais HM, Mirjalili S, Alhussian H. Approaches to multi-objective feature selection: A systematic literature review. IEEE Access. (2020) 8:125076–96. doi: 10.1109/ACCESS.2020.3007291
Keywords: AI models, identification, prognostic and predictive biomarkers, lung cancer, systematic review, meta-analysis
Citation: AlOsaimi HM, Alshilash AM, Al-Saif LK, Bosbait JM, Albeladi RS, Almutairi DR, Alhazzaa AA, Alluqmani TA, Al Qahtani SM, Almohammadi SA, Alamri RA, Alkurdi AA, Aljohani WK, Alraddadi RH and Alshammari MK (2025) AI models for the identification of prognostic and predictive biomarkers in lung cancer: a systematic review and meta-analysis. Front. Oncol. 15:1424647. doi: 10.3389/fonc.2025.1424647
Received: 28 April 2024; Accepted: 28 January 2025;
Published: 26 February 2025.
Edited by:
Beatrice Aramini, University of Bologna, ItalyReviewed by:
Songxiao Xu, University of Chinese Academy of Sciences, ChinaCopyright © 2025 AlOsaimi, Alshilash, Al-Saif, Bosbait, Albeladi, Almutairi, Alhazzaa, Alluqmani, Al Qahtani, Almohammadi, Alamri, Alkurdi, Aljohani, Alraddadi and Alshammari. This is an open-access article distributed under the terms of the Creative Commons Attribution License (CC BY). The use, distribution or reproduction in other forums is permitted, provided the original author(s) and the copyright owner(s) are credited and that the original publication in this journal is cited, in accordance with accepted academic practice. No use, distribution or reproduction is permitted which does not comply with these terms.
*Correspondence: Hind M. AlOsaimi, SGFsb3NhaW1pQGtmbWMubWVkLnNh; Mohammed K. Alshammari, TWthYWxzaGFtbWFyaUBrZm1jLm1lZC5zYQ==
Disclaimer: All claims expressed in this article are solely those of the authors and do not necessarily represent those of their affiliated organizations, or those of the publisher, the editors and the reviewers. Any product that may be evaluated in this article or claim that may be made by its manufacturer is not guaranteed or endorsed by the publisher.
Research integrity at Frontiers
Learn more about the work of our research integrity team to safeguard the quality of each article we publish.