- 1Department of Experimental Oncology, Istituto di Ricerche Farmacologiche Mario Negri IRCCS, Milan, Italy
- 2Department of Neuroscience, Istituto di Ricerche Farmacologiche Mario Negri IRCCS, Milan, Italy
- 3Tecniplast SpA, Buguggiate, Italy
Introduction: Lung cancer is the first cause of cancer death in the world, due to a delayed diagnosis and the absence of efficacy therapies. KRAS mutation occurs in 25% of all lung cancers and the concomitant mutations in LKB1 determine aggressive subtypes of these tumors. The improvement of therapeutical options for KRASG12C mutations has increased the possibility of treating these tumors, but resistance to these therapies has emerged. Preclinical animal models permit the study of tumors and the development of new therapies. The DVC system was used to measure circadian activity changes indicative of lung cancer progression in KRAS and KRAS-LKB1 transgenic mouse models.
Material and methods: KRAS and KRAS-LKB1 conditional transgenic animal models were bred and genotyped. The tumors were inducted using adeno-CRE-recombinase system. The mice were housed in a Digital Ventilated Cage (DVC®) rack measuring the locomotor activity continuously for 24/7. The progression of the tumors was monitored with MRI. The DVC system evaluated a reduction in animal locomotion during the tumor progression.
Results: KRAS and KRAS-LKB1 mutations were induced, and the tumor formation and progression were monitored over time. As expected, the onset of the tumors in the two different breeds occurred at different times. DVC system registered the locomotion activity of the mice during the light and dark phases, reporting a strong reduction, mainly, in the dark phase. In KRAS-LKB1 models, the locomotion reduction appeared more pronounced than in KRAS models.
Discussions: Transgenic animal models represent a fundamental tool to study the biology of cancers and the development of new therapies. The tumors induced in these models harbor the same genotypical and phenotypical characteristics as their human counterparts. DVC methods permit a home cage monitoring system useful for tracking animal behavior continuously 24 hours a day, 7 days a week. DVC system could determine disease progression by monitoring a single animal activity in a cage and also using group-housed animals. For these reasons, the DVC system could play a crucial role in identifying diseases at early stages and in testing new therapeutic approaches with a higher likelihood of efficacy.
1 Introduction
Lung cancer is the first cause of cancer death in men and the second in women (1–3). In most cases the disease manifests in an advanced stage, whereas only in 20% of patients the pathology is diagnosed at an early stage (4, 5). The clinical picture is further complicated by the lack of fully effective pharmacological therapies. The 5-year overall survival for these patients decreases depending on the stage at which the tumor is diagnosed (6, 7). Lung cancer is considered an environmental disease and tobacco is the principal risk factor for this pathology (8, 9). However, lung cancer can also occur in non-smokers due to various causes, and these tumors exhibit different biochemical characteristics compared to those found in smokers (10–12). The advancement of molecular biology techniques has improved the knowledge about the biology of tumors and the main mutations that characterize these malignancies. KRAS mutations represent 25% of all lung cancers (13–16). KRAS-LKB1 co-mutated tumors are a frequent and very aggressive subtype of these tumors, and in particular in non-small-cell-lung-cancer (NSCLC) (17–25). In particular, about 50% of KRAS-mutated lung cancer exhibit also deletions in LKB1. LKB1 is a kinase involved in metabolic control, redox homeostasis, and cell polarity (19, 22, 26, 27). LKB1 activates AMPK, a cellular nutrient sensor capable of detecting the ATP/ADP ratio to determine the cell’s energetic status (28, 29). This pathway is closely linked to the PI3-AKT-mTOR pathway, with which it has an opposing functional relationship. While the LKB1-AMPK pathway metabolically favors the activation of catabolic reactions, the latter promotes protein synthesis and anabolism in general. When the cell is in an energy deficit (low ATP/ADP ratio), the LKB1 pathway is activated, shutting down AKT-mTOR signaling to promote catabolic cellular reactions, aiming to restore proper cellular energetic status. In tumors, LKB1 is deleted, leading to the constitutive activation of the PI3K-AKT-mTOR pathway, resulting in a growth advantage for the tumor. KRAS modulates the activation of PI3K-AKT-mTOR pathway, therefore, the concomitant mutation between KRAS and LKB1 determine ad advantage for the tumor growth. The mutational spectrum of KRAS is particularly diverse, but the most frequent mutation in lung cancer is G12C (30–32). For many years, despite numerous attempts, KRAS mutations have posed a significant obstacle to the treatment of these patients, in fact, the only therapeutical approach was conventional chemotherapy and the prognosis was poor (33, 34). In recent years, significant advancements in treating lung cancer subtypes with KRAS G12C mutations have led to an increase in patient survival (35–40). However, as for the other targeted therapy, resistance to these therapies has already been observed at the clinical level (41–45). The absence of a fully effective therapies for treating these tumors necessitates further research to explore new therapeutic approaches. Preclinical animal models are a crucial tool for enhancing our understanding of tumors and for exploring new therapeutic strategies (46–50). These models represent an intermediate step in the experimentation process between cell cultures and patients. In particular, transgenic animal models allow for the study of specific mutations that define different tumors (51, 52). Transgenic animal models develop tumors that maintain the same characteristics as patient tumors, allowing the results to be translated into clinical practice (53–55). Furthermore, in these models, is possible to reproduce the different phenotypes of the disease. In this work, we inducted the onset of the tumors in KRAS and KRAS-LKB1 transgenic mice following the pathogenesis over time (53, 56–58). Mice with the co-mutation in KRAS/LKB1 develop more aggressive tumors, as the deletion of LKB1 increases the potential for metastasis (53, 59–62). The goal of these experiments was to observe the motor activity of the mice using the DVC ventilated cage system. DVC system allows the evaluation of these parameters without disturbing the animals. The development of tumors in the lung lobules of the animals over time was monitored using magnetic resonance imaging (MRI) (17). This tool (DVC system) could represent a significant advancement in understanding tumor biology, as well as improving the ethical standards of experiments.
2 Material and methods
2.1 Maintenance of conditional transgenic mice colonies
Conditional transgenic animal models were acquired from the Jackson Laboratory in two different strains: c57-B6.129S4-KRAS<tm4Tyj>/J and c57-Stk11tm1.1Sjm/j. The first strain was modified in KRAS gene for expressing the mutation G12D. The second strain was modified in LKB1 gene to delete the protein. The animals were mated (2 female and 1 male) to expand the colonies of every strain. After 3 weeks postpartum female and male mice were weaned and separated in different cages. The identification of the mice was permitted by inserting a numerical tag into the ear and simultaneously taking a small tissue sample (from the ear); the samples were necessary to genotype the animals. KRAS and LKB1 mutated mice were crossed to generate colonies that harbor both mutations. The genotype of interest was KRAS het/LKB1 ko. However, we also carried out breeding with transgenic mice modified only in the KRAS gene, in order to subsequently develop tumors with the single KRAS mutation (het). Since these models are conditional mice, at this stage the mutations of interest were not yet present; they were only expressed after the administration of Cre-recombinase (SignaGen Laboratories, Rockville, MD, USA). In the case of LKB1, it involves a gene deletion, thus a knock-out, whereas in the case of KRAS, it involves the acquisition of a point mutation, thus a knock-in.
2.2 Genotyping PCR
Tissue samples (small ear pieces) were collected from the mice during weaning. DNA was extracted from the samples using Maxwell ® 16 Tissue DNA Purification (Promega). The DNA extracted was quantified using NanoDrop 2000 (Thermo-Fisher). The 260/280 ratio of the samples ranged between 1.8 and 2.0. PCR was performed using a hot-start DNA polymerase mix (Promega) containing oligonucleotides, reaction buffer with dye, and other reagents important for the function of DNA polymerase. Primer sequences specified for KRAS-LKB1-modified genes were provided directly from Charles River. In the same way, the PCR program was supplied by the company. KRAS-PCR showed a single amplified band (250 bp) when the genotype was wild-type, instead, resulted in two bands (250 bp and 100 bp) when the genotype was heterozygous for G12D mutation. LKB1-PCR highlighted three possible variants: a single band to 348 bp when the genotype was wild-type, two bands to 348 bp and 450 bp when the genotype was heterozygous and, a single band to 450 bp when the genotype was homozygous for the mutation. KRAS transgenic mice were a knock-in model, whereas LKB1 transgenic mice were a knock-out model.
2.3 Lung tumors induction with CRE-recombinase system
After 4 weeks from birth the mice carrying the modified alleles (KRAS het/LKB1 ko) were anesthetized with ketamine (75 mg/kg) and medetomidine (1 mg/kg). The animals were inoculated with adenoviral CRE-recombinase. The viral particles used for the induction were quantified in 5*106 for every animal. The administration of viral particles was performed in intranasal way. The mice were anesthetized with medetomidine and ketamine. Subsequently, under sterile conditions, the virus was delivered directly into the animals’ nostrils. After inoculation, the mice were awakened using an antidote. The animals were then kept in ventilated cages for a few days to allow the viral load to dissipate. For some animals, we attempted to administer the virus directly in the trachea, but due to the difficulties related to animal handling, we decided to continue exclusively with intranasal administration.
2.4 Magnetic resonance to monitor tumor development
During the MRI scans (duration 20-30 min/mouse), animals were kept under controlled anesthesia conditions (100% O2, 2% isoflurane). The animals’ temperature was kept at 36.5 +/-0.5°C using a warmed cradle. Mouse lungs were scanned with a T2w sequence using a respiration triggered spin-echo sequence (12 coronal slices of 1 mm thickness, TR/TE = 1000/25 ms, 117 µm2 in-plane resolution, FOV 3x2 cm2, matrix size = 256 x 172).
2.5 Quantification of the lung volume occupied by the tumor
Images have been analyzed using two free software: Fiji (63) and ITK-SNAP (64). The whole lung has been manually segmented and extracted from the whole chest image stack. The signal of the dark healthy tissue has been set as background. After that a threshold of 3 standard deviations of the background was applied in order to highlight the tumoral tissue with respect to the healthy one. The mask determined in this way covered the tumoral tissue inside the lungs. At the end of the automatic segmentation, an expert researcher has checked the goodness of the segmentation. To consider the different lung sizes between subjects, the volume of the tumor has been expressed as a percentage of the whole lung volume.
2.6 DVC methods
30 animals were housed in a Digital Ventilated Cage (DVC®) rack, a home cage monitoring system capable of continuously tracking animal activity 24/7. This system builds up upon a standard IVC rack by placing an electronic capacitance sensing board beneath each cage to detect the movement of the animals in the area surrounding each of the 12 electrodes that compose the board (65). We used 8 cages, with each cage containing between 3 and 5 animals. Five of these cages contained KRAS/LKB1 mice, while three cages contained KRAS mutant mice. We used the Animal Locomotion Index Smoothed (DVC® Analytics, Tecniplast S.p.A., Buguggiate, Italy) to measure the activity of the mice. This metric is based on the activation density metric defined in (65) which calculates the number of electrodes activations caused by mouse activity, and normalizes it by dividing by the time interval and the number of electrodes (yielding values ranging from 0 to 1). We averaged activity by day and/or week, by distinguishing the light and dark phases of the day. Since the cage numerosity was different between cages and changed over time (because of the separation of some animals due to fight, especially among males), we normalized the animal activity by dividing by the number of the animals in each cage at any given time. Finally, we performed a linear regression analysis on the daily activity data to quantify trends in activity over time.
2.7 Statistical analyses
We used Python to process and visualize DVC data. We used scikit-learn package to fit a linear regression model on daily activity data over time (light and dark separately). We used R to run the corresponding statistics, with significance level α = 0.05. We used lme4 and lmerTest R software packages to model weekly activity data as Linear Mixed Model and test for fixed effects of genotype and time, and a random effect of time (Supplementary Tables S1, S2). We tested the normality assumption of the model residuals and random effects with Shapiro-Wilk tests (p>0.05), and the homoscedasticity of the model residuals with a between-subjects one-way ANOVA (p>0.05).
3 Results
3.1 Generation and maintenance of murine colonies harboring KRAS and KRAS/LKB1 mutation
KRAS and LKB1 mutations frequently characterize NSCLC (17, 22). As reported in the literature, transgenic animal models with LKB1 mutations do not develop tumors. The presence of co-mutation in KRAS and LKB1 instead determined the onset of tumors in the models. Furthermore, these tumors are very aggressive and prone to metastasis. Transgenic animal models with mutations only in the KRAS gene can also develop tumors. Therefore, we decided to study these mutations with transgenic animal models. The animals used were inducible because the modified genes were floxed and only the administration of cre-recombinase can activate the phenotype (66). Therefore, at this stage the animals bred were wild-type. In the LKB1 model, alterations are present in both alleles of the gene, whereas in the KRAS model, the mutation was present in only one allele, because the mice with mutations in both alleles do not survive to birth. The two strains were expanded to determine enough mice to start the crosses. The crosses were maintained for three generations to stabilize the genotype. Before beginning the crosses between the two different mouse strains, the theoretical allele frequencies were determined. After starting the crosses the real allele frequencies were determined to check whether the expected frequencies were confirmed. Table 1 reported the allele frequencies.
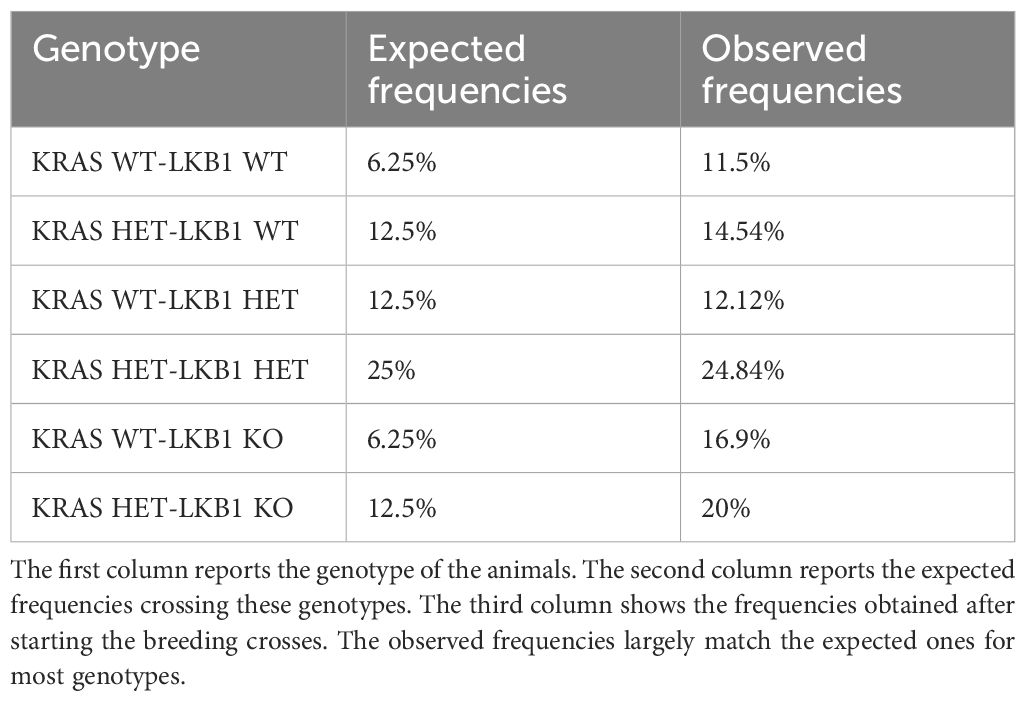
Table 1. The table reported the expected genotypic frequencies on our transgenic models, alongside the real frequencies observed in a total of approximately 200 animals.
3.2 Induction of the mutations and tumor timing
The mutations in the animals were inducted with Cre-recombinase administrating 5*106 viral particles. This number of viral particles was chosen because it results in a level of lesions that is not too extensive and therefore difficult to quantify. Both the strains (KRAS mutated and KRAS-LKB1 mutated) were treated with this number of viral particles. The mice were treated with the virus after 4-6 weeks from birth because older mice were less prone to develop lesions. The timing of the onset of the tumors was determined through pilot experiments using MRI protocol. The mice were weekly monitored identifying the first lesions after 3 weeks from the virus administration in KRAS-LKB1 mice. The mice with only KRAS mutation displayed the first lesions a few weeks later (10-12 weeks) after injection.
3.3 Timing of tumor onset
The onset of the tumors was observed using magnetic resonance (MRI). The injection of the viral particles should be carried out after 4-6 weeks from the birth. This timing was important because older animals could be less susceptible to the onset of the tumor with this method. Furthermore, the presence of elevated adipose tissue may increase anesthetic-induced toxicity (mainly in males). The figure below (Figure 1) shows some images captured by MRI and the presence of the tumor lesions increased after each week. The quantification of the lesions has been performed with two software (ITK-SNAP and Fiji), as reported in the methods. These parameters were measured to plan the main experiment.
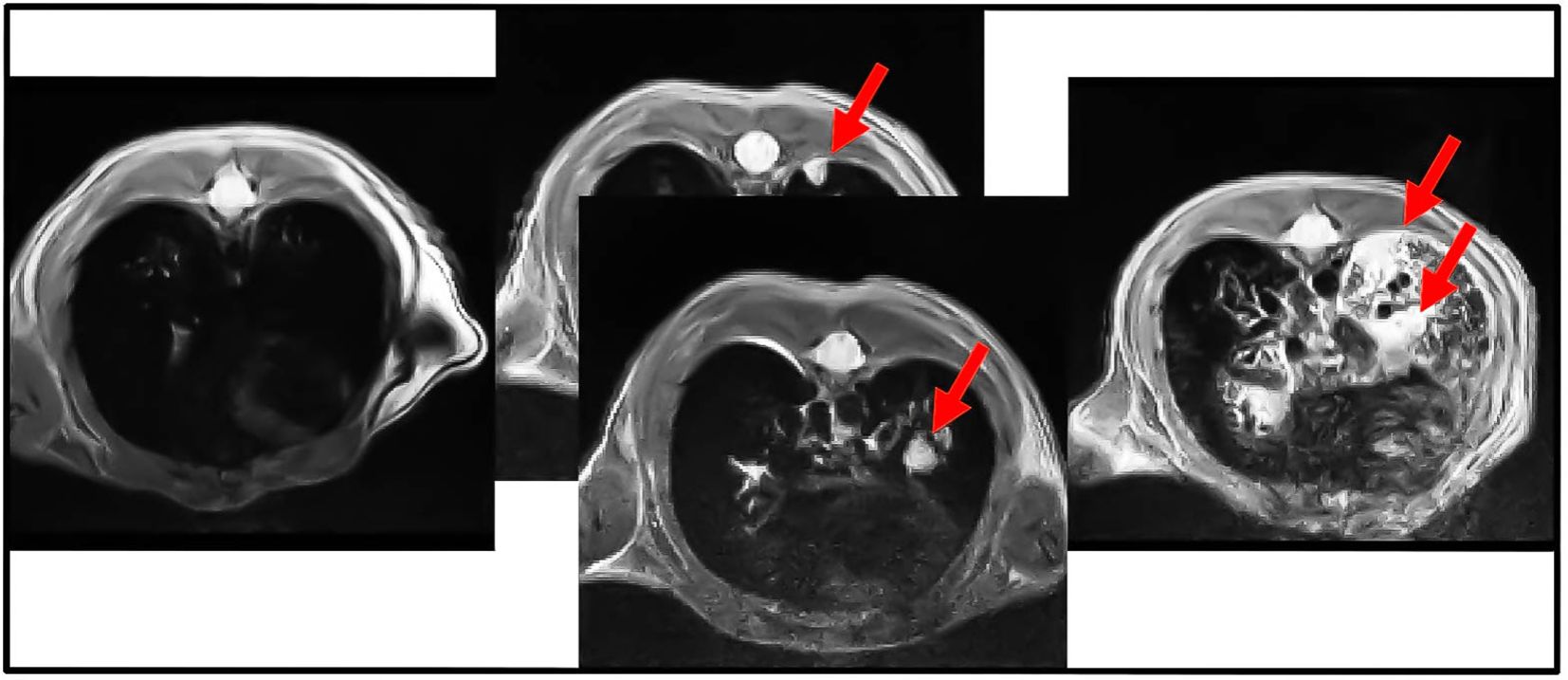
Figure 1. Cross-section of MRI analysis of the lungs from KRAS/LKB1 mutated mice. The arrows show the presence of nodules. Images were acquired weekly for 4 weeks, showing the progression of lesions over time. The arrows indicate the presence of nodules in the lungs. This scan was derived only from KRAS/LKB1 mice. The first scan was referred to T0. The scan in the middle was referred to T1. The last scan was referred to T2.
3.4 Digital ventilated cages system analyses evaluated the locomotion activity of the animals with tumor lesions
Normally, body weight is used as an endpoint to evaluate the health status of animals with tumor lesions in the lungs (17, 67, 68). When body weight decreases, it often indicates the presence of numerous lung nodules, as the weight loss is proportional to the number of lesions. However, this requires handling the animals. DVC analyses provide an alternative by using a different endpoint to monitor disease progression unintrusively. The animals were placed in a ventilated cage system that measures their activity levels. The mice, in which the disease was induced by the injection of an adenoviral vector, were placed in the cage for monitoring. This experiment involved mice with KRAS/LKB1 mutations and those with KRAS mutations alone. The KRAS/LKB1-mutated model developed the disease more slowly than the KRAS-mutated mice. Specifically, the first lesions appeared 10-12 weeks after injection, as determined by MRI analysis. The monitoring of KRAS/LKB1-mutated mice was later placed in the cage due to the delayed onset of lesions.
3.5 Magnetic resonance analyses showed the presence of tumor nodules in the lungs
7 animals were also observed through MRI during the experiment to verify tumor onset. The nodules in the animals lung were quantified using the procedure described in the methods. The Table 2 shows the percentage of lung tissue occupied by the lesions. Figure 1 illustrates the cross-section of the animal lungs, clearly displaying the nodules occupying the lung volume. Figure 2 depicts the frontal sections of the lung from KRAS/LKB1 mice and KRAS mice. Tumor lesions are clearly visible in all the animals observed.
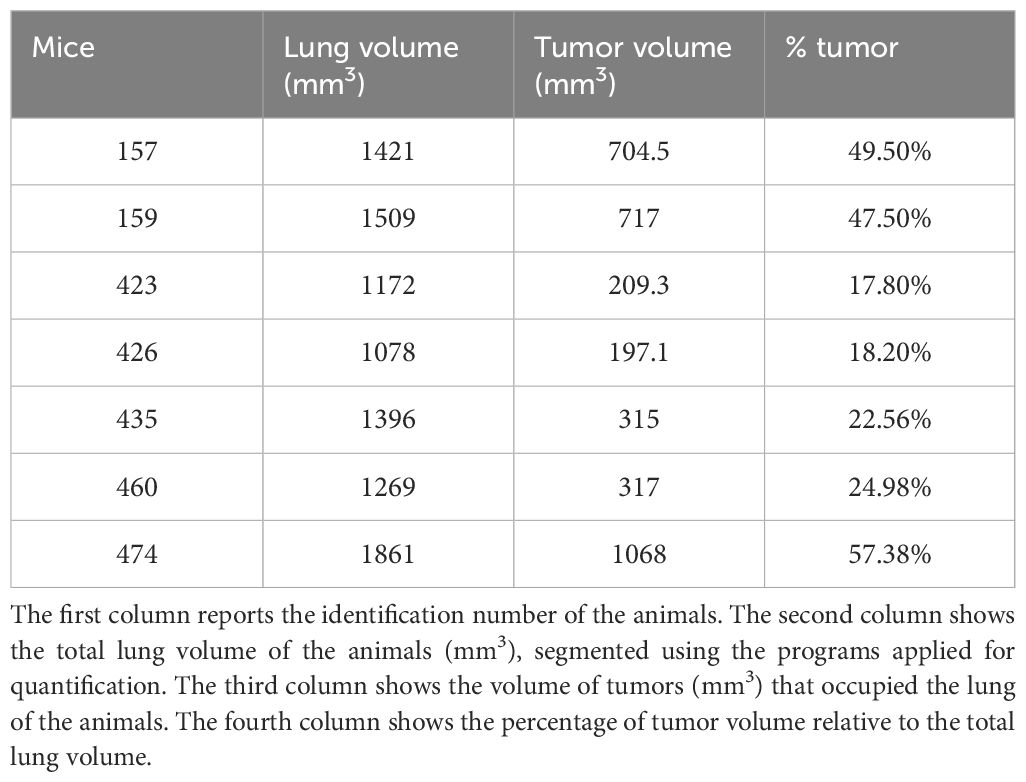
Table 2. The table shows the percentage of the lesions present in the lungs of the animal relative to the lung volume.
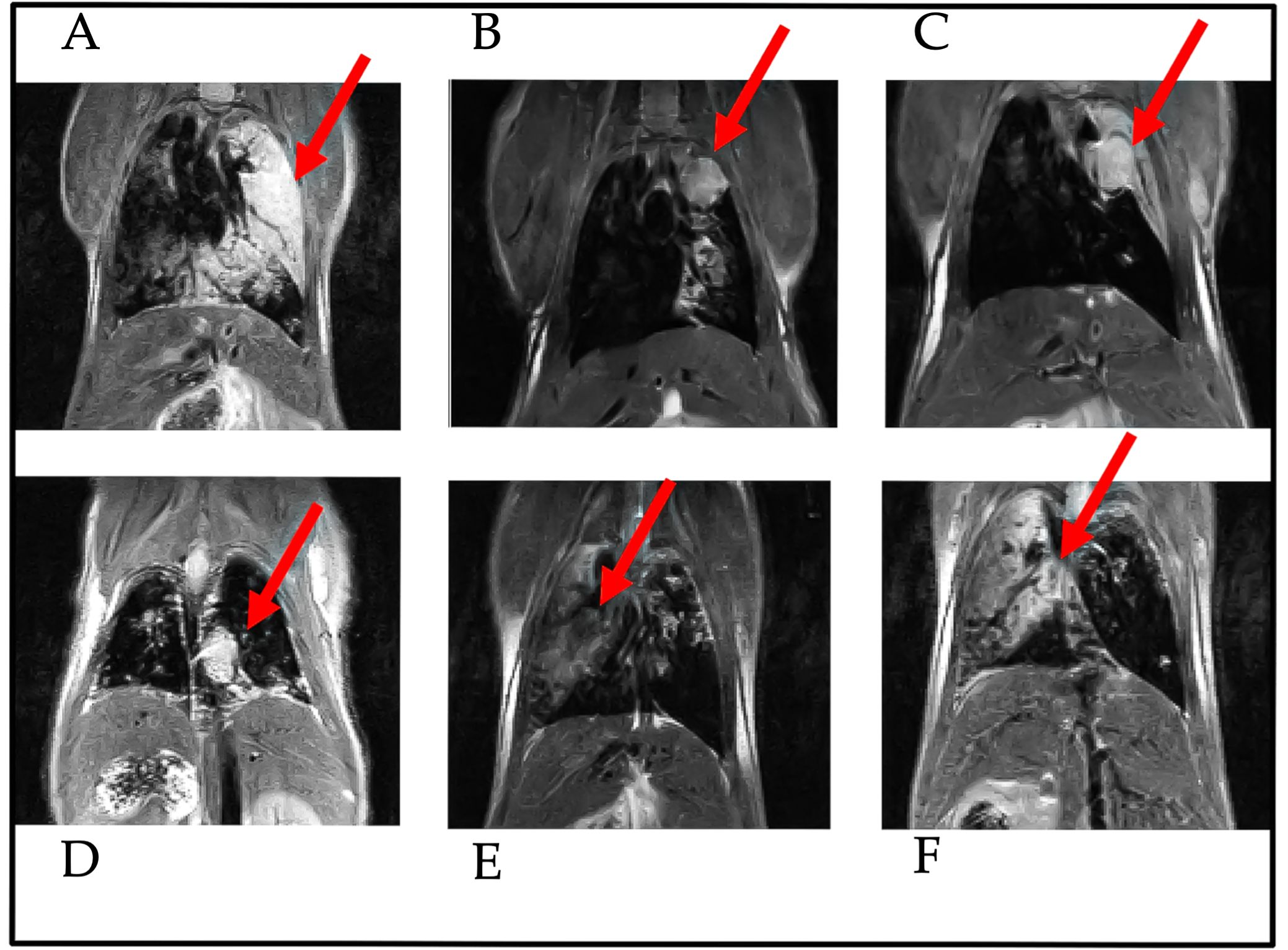
Figure 2. Frontal-section of MRI analysis of the lungs from KRAS/LKB1-mutant mice and KRAS-mutant mice. Images were captured 55 days after the initiation of DVC analysis. The arrows indicate the presence of nodules in the lungs. These scans were performed for both genotypes. The scans A–D were derived from KRAS/LKB1 mice. The last two scans (E, F) were derived from KRAS-mutated mice.
3.6 Activity from home-cage monitoring
The activity of the animals was monitored by the DVC® system 24/7 for 81 days. Figure 3 shows the average daily activity of 5 cages of KRAS/LKB1 mice and 3 cages of KRAS-mutated mice, during the light and dark phases over time. As expected, all the mice displayed a higher activity during the dark phase, which is consistent with their nocturnal behavior (69). The graphs show a reduction in movement starting at around 50-60 days, especially during the dark phase. Figure 4 displays the average weekly activity of the mice during the light and dark phases. The activity has been normalized by the number of mice, due to differences in cage numerosity between units and over time. We observed a decline in activity across individual genotypes in both light conditions (lmerTest, pweek <0.001). During dark phase, KRAS/LKB1 mice generally showed lower activity compared to KRAS-mutated mice (lmerTest, pgenotype <0.05), but a different evolution over time (lmerTest, pgenotype×week <0.05). The details of the Linear Mixed Models are reported in the Supplementary Materials (Supplementary Tables S1, S2). We quantified the decrease in activity over the last observation month by performing a Linear Regression on daily activity data starting from week 8 (considering only the cages present during that period). Figure 5 shows the slope coefficients in light and dark phases. While the decrease of activity during lights-on is absent with a slope close to 0 in both genotypes, during the dark period KRAS/LKB1 exhibit a lower coefficient than KRAS mice. This suggests a faster decrease in activity, although statistical testing was not possible due to the limited sample size in the final observation weeks.
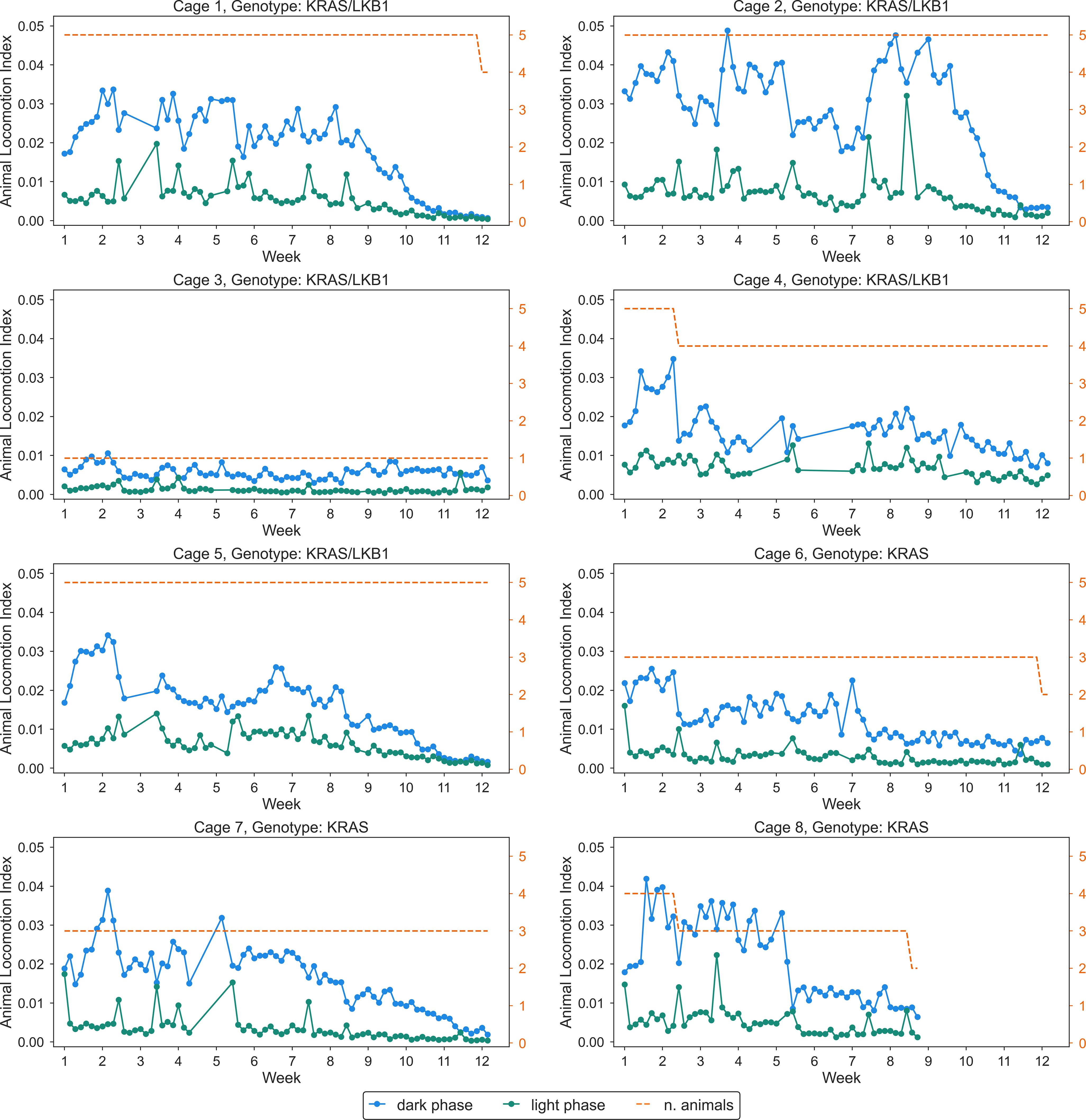
Figure 3. Daily activity of all the cages (KRAS/LKB1 and KRAS) in light and dark conditions. Each panel depicts daily average activity across 81 days, in cages of KRAS/LKB1 and KRAS mice. KRAS/LKB1 mutated mice started the measurement after about 28 days from the birth, while KRAS mice started the measurement after about 60 days from the birth. The blue lines show the activity during the dark phase, while the green lines represent activity during the light phase. The dashed orange line and axis represents the number of animals inside the cage, which changed over time.
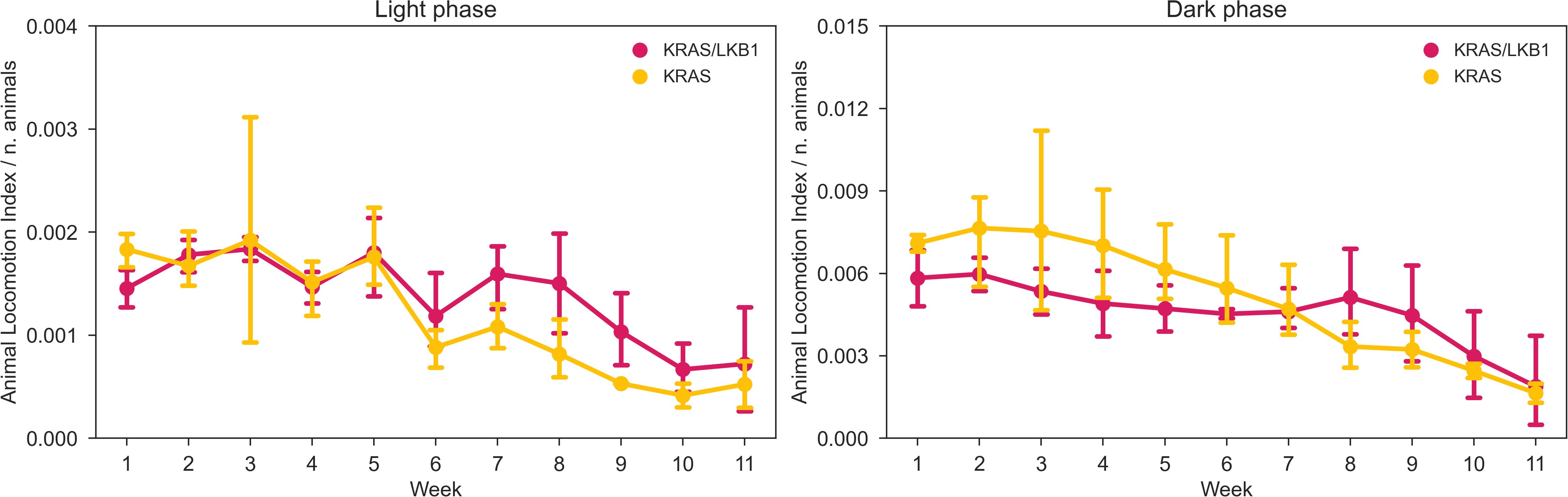
Figure 4. Average weekly activity normalized per cage density. Average weekly normalized activity (± SD) over light (left panel) and dark (right panel) phases across 11 weeks of observation and between two genotypes. N of cages per group: KRAS/LKB1 = 5, KRAS = 3.
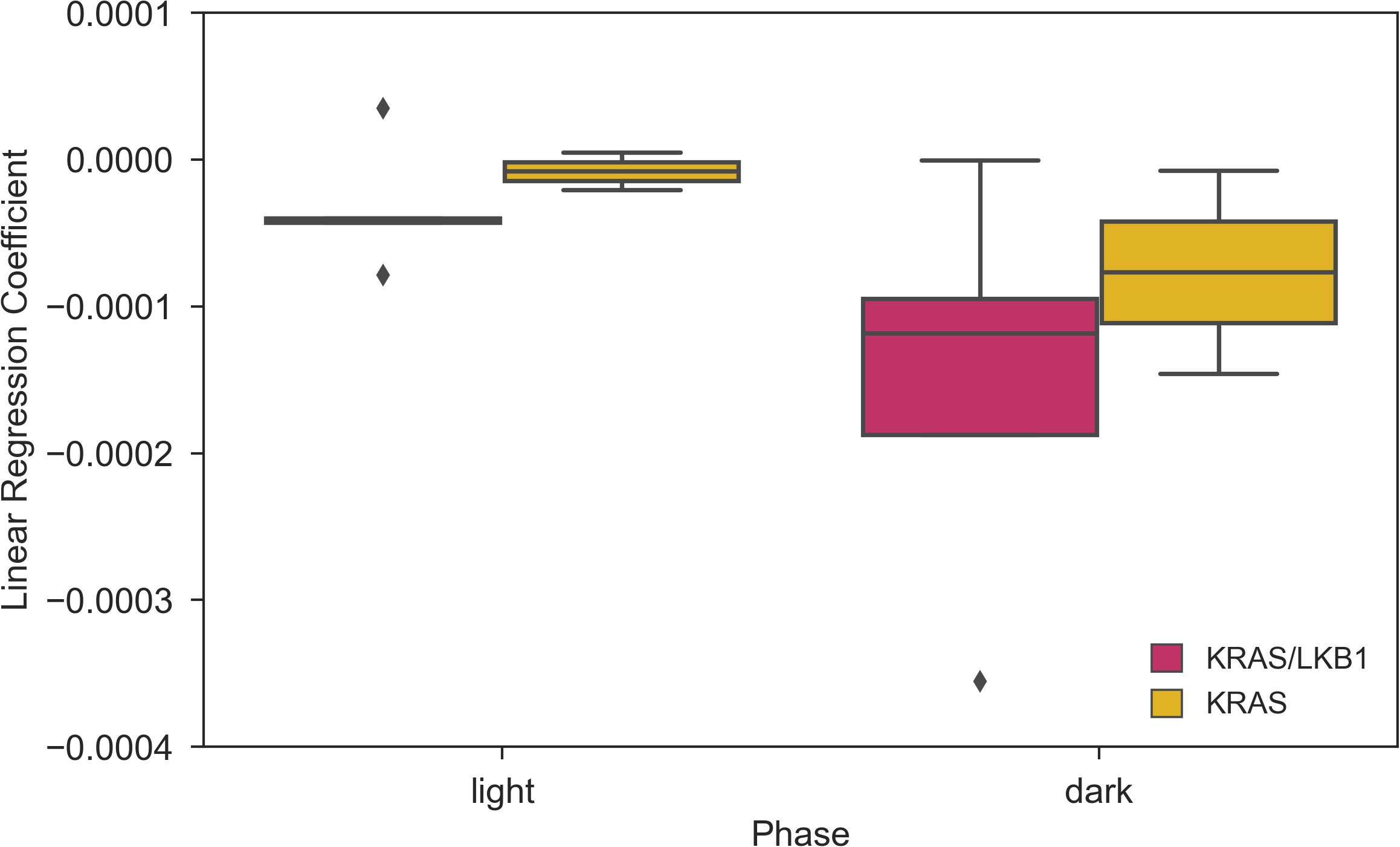
Figure 5. Coefficients of the Linear Regression analysis of daily activity over time during weeks 8-11. Slope of the linear regression computed for the day and night time daily activity points in weeks 8-11. N of cages per group: KRAS/LKB1 = 5, KRAS = 2.
4 Discussion
In this study, transgenic animal models of lung cancer harboring KRAS/LKB1 and KRAS mutations were used. Despite advancements in understanding the molecular biology of these tumors and the development of new therapeutic options, these tumor subtypes remain lethal. In several circumstances, the aggressiveness of the tumors harboring mutations in KRAS/LKB1 precludes the possibility to perform a proper treatment for the patients. It is, therefore, mandatory to have preclinical models able to recapitulate the human tumors. These models can help in defining new treatments for these unfavored tumors (58, 70, 71). Examples of patients derived xenografts, i.e. tumors obtained at surgical level from patients and implanted in immunodeficient animal are an important tool. However, one of the drawbacks of these important models is the lack of a full immune system that does not allow the testing of immune checkpoint inhibitors that represent an important armamentarium for NSCLC patients. In this context, transgenic animal models that are obtained in fully immunological competent mice, represent important tools for enhancing cancer research. Specifically, LKB1/KRAS transgenic animal models serve as translatable models for human lung cancer due to the similar molecular and histological characteristics of the tumors. However, these models can generate different histological subtypes, reflecting the typical heterogeneity of cancer. Additionally, KRAS-mutated transgenic animal models were employed in this study. This model can produce tumors, although they are less aggressive compared to the KRAS/LKB1 model. As expected, tumor onset occurred at different times in the two models. In our study, we also attempted to use a method that can quantify lesions within the animals’ lungs. This quantification method could be useful in the future for studying tumor progression. MRI analysis is a fundamental technique for examining the lungs of animals to assess the presence of lesions; however, this technique requires animal manipulation. The DVC methods represent a home cage monitoring system designed for continuous tracking of animal behavior 24 hours a day, 7 days a week (72–74). Specifically, the Animal Locomotion Index Smoothed was used to measure the activity of the mice. A recent study conducted on lung tumor models demonstrated that DVC methods could determine disease progression by monitoring the activity of individual animals in a cage (75). The analyses conducted with the DVC demonstrate a significant decrease in the locomotor activity of the animals during disease progression. The ability to analyze the animals with MRI also confirmed the presence of tumor lesions within the lungs of the animals. However, the absence of a healthy control group represents a limitation in the interpretation of our data, and a comparison in the evaluation of locomotor activity between animals without lesions and animals with tumors would certainly have strengthened our findings. In KRAS/LKB1 models, the locomotion reduction appeared more pronounced than in KRAS mice, possibly reflecting the aggressive nature of these tumor subtypes. However, this remains a hypothesis, as we lack statistical evidence to confirm that KRAS7LKB1 mice exhibit a greater reduction in locomotion compared to KRAS mice due to the more aggressive nature of the former tumors. This study represents a significant advancement, as the DVC system can detect variations in animal activity related to disease progression while using group-housed animals (76, 77). Importantly, a key feature of the DVC system is its ability to monitor disease progression without disturbing the animals. The possibility of using the DVC on other tumor models is a goal for the future. In particular, considering the high potential of conditional transgenic mouse models, which allow for the replication of all mutations that characterize tumors, the same methodology could be applied to conduct similar evaluations in many other types of cancers, not just lung cancer. For example, a study conducted on breast cancer tests the possibility of using the DVC system to evaluate new analgesics aimed at reducing tumor-induced pain in animals (78). These findings pave the way for developing new therapeutic strategies, whose effectiveness could potentially be detected in early stages, increasing the likelihood of achieving the desired outcomes.
Data availability statement
The raw data supporting the conclusions of this article will be made available by the authors, without undue reservation.
Ethics statement
The animal study was approved by Animal Care Unit of Mario Negri Institute for Pharmacological Research. The study was conducted in accordance with the local legislation and institutional requirements.
Author contributions
MT: Conceptualization, Data curation, Investigation, Methodology, Writing – original draft, Writing – review & editing. FG: Investigation, Methodology, Writing – review & editing. GV: Investigation, Methodology, Writing – review & editing. EM: Data curation, Investigation, Methodology, Writing – review & editing. MR: Data curation, Methodology, Writing – review & editing. FT: Investigation, Methodology, Writing – review & editing. EC: Investigation, Methodology, Writing – review & editing. MM: Investigation, Methodology, Writing – review & editing. MB: Conceptualization, Data curation, Funding acquisition, Investigation, Methodology, Project administration, Writing – original draft, Writing – review & editing.
Funding
The author(s) declare financial support was received for the research, authorship, and/or publication of this article. The authors declare financial support from Associazione Italiana per la Ricerca sul Cancro (IG24347).
Conflict of interest
MR is the lead data scientist at Tecniplast SpA. Tecniplast SpA did not have any decisive role in the study design, data collection and analysis, decision to publish, or preparation of the manuscript.
The remaining authors declare that the research was conducted in the absence of any commercial or financial relationships that could be construed as a potential conflict of interest.
The author(s) declared that they were an editorial board member of Frontiers, at the time of submission. This had no impact on the peer review process and the final decision.
Generative AI statement
The author(s) declare that no Generative AI was used in the creation of this manuscript.
Publisher’s note
All claims expressed in this article are solely those of the authors and do not necessarily represent those of their affiliated organizations, or those of the publisher, the editors and the reviewers. Any product that may be evaluated in this article, or claim that may be made by its manufacturer, is not guaranteed or endorsed by the publisher.
Supplementary material
The Supplementary Material for this article can be found online at: https://www.frontiersin.org/articles/10.3389/fonc.2024.1504938/full#supplementary-material
References
1. Fitzmaurice C, Abate D, Abbasi N, Abbastabar H, Abd-Allah F, Abdel-Rahman O, et al. Global, regional, and national cancer incidence, mortality, years of life lost, years lived with disability, and disability-adjusted life-years for 29 cancer groups, 1990 to 2017: A systematic analysis for the global burden of disease study. JAMA Oncol. (2019) 5:1749–68. doi: 10.1001/jamaoncol.2019.2996
2. Thandra KC, Barsouk A, Saginala K, Aluru JS, Barsouk A. Epidemiology of lung cancer. Contemp Oncol (Pozn). (2021) 25:45–52.
3. de Groot PM, Wu CC, Carter BW, Munden RF. The epidemiology of lung cancer. Transl Lung Cancer Res. (2018) 7:220–33. doi: 10.21037/tlcr.2018.05.06
4. Li C, Wang H, Jiang Y, Fu W, Liu X, Zhong R, et al. Advances in lung cancer screening and early detection. Cancer Biol Med. (2022) 19:591–608. doi: 10.20892/j.issn.2095-3941.2021.0690
5. Goldstraw P, Chansky K, Crowley J, Rami-Porta R, Asamura H, Eberhardt WE, et al. The IASLC lung cancer staging project: proposals for revision of the TNM stage groupings in the forthcoming (Eighth) edition of the TNM classification for lung cancer. J Thorac Oncol. (2016) 11:39–51. doi: 10.1016/j.jtho.2015.09.009
6. Jeon DS, Kim HC, Kim SH, Kim TJ, Kim HK, Moon MH, et al. Five-year overall survival and prognostic factors in patients with lung cancer: results from the korean association of lung cancer registry (KALC-R) 2015. Cancer Res Treat. (2023) 55:103–11. doi: 10.4143/crt.2022.264
7. Siegel RL, Miller KD, Fuchs HE, Jemal A. Cancer statistics, 2022. CA Cancer J Clin. (2022) 72:7–33. doi: 10.3322/caac.21708
8. Alberg AJ, Brock MV, Samet JM. Epidemiology of lung cancer: looking to the future. J Clin Oncol. (2005) 23:3175–85. doi: 10.1200/JCO.2005.10.462
9. Gharibvand L, Shavlik D, Ghamsary M, Beeson WL, Soret S, Knutsen R, et al. The association between ambient fine particulate air pollution and lung cancer incidence: results from the AHSMOG-2 study. Environ Health Perspect. (2017) 125:378–84. doi: 10.1289/EHP124
11. LoPiccolo J, Gusev A, Christiani DC, Jänne PA. Lung cancer in patients who have never smoked - an emerging disease. Nat Rev Clin Oncol. (2024) 21:121–46. doi: 10.1038/s41571-023-00844-0
12. Zhang T, Joubert P, Ansari-Pour N, Zhao W, Hoang PH, Lokanga R, et al. Genomic and evolutionary classification of lung cancer in never smokers. Nat Genet. (2021) 53:1348–59. doi: 10.1038/s41588-021-00920-0
13. Karachaliou N, Mayo C, Costa C, Magrí I, Gimenez-Capitan A, Molina-Vila MA, et al. KRAS mutations in lung cancer. Clin Lung Cancer. (2013) 14:205–14. doi: 10.1016/j.cllc.2012.09.007
14. Westcott PM, To MD. The genetics and biology of KRAS in lung cancer. Chin J Cancer. (2013) 32:63–70. doi: 10.5732/cjc.012.10098
15. Reck M, Carbone DP, Garassino M, Barlesi F. Targeting KRAS in non-small-cell lung cancer: recent progress and new approaches. Ann Oncol. (2021) 32:1101–10. doi: 10.1016/j.annonc.2021.06.001
16. Prior IA, Lewis PD, Mattos C. A comprehensive survey of Ras mutations in cancer. Cancer Res. (2012) 72:2457–67. doi: 10.1158/0008-5472.CAN-11-2612
17. Caiola E, Iezzi A, Tomanelli M, Bonaldi E, Scagliotti A, Colombo M, et al. LKB1 deficiency renders NSCLC cells sensitive to ERK inhibitors. J Thorac Oncol. (2020) 15:360–70. doi: 10.1016/j.jtho.2019.10.009
18. Arbour KC, Jordan E, Kim HR, Dienstag J, Yu HA, Sanchez-Vega F, et al. Effects of co-occurring genomic alterations on outcomes in patients with KRAS-mutant non-small cell lung cancer. Clin Cancer Res. (2018) 24:334–40. doi: 10.1158/1078-0432.CCR-17-1841
19. Hemminki A, Markie D, Tomlinson I, Avizienyte E, Roth S, Loukola A, et al. A serine/threonine kinase gene defective in Peutz-Jeghers syndrome. Nature. (1998) 391:184–7. doi: 10.1038/34432
20. Parker SJ, Svensson RU, Divakaruni AS, Lefebvre AE, Murphy AN, Shaw RJ, et al. LKB1 promotes metabolic flexibility in response to energy stress. Metab Eng. (2017) 43:208–17. doi: 10.1016/j.ymben.2016.12.010
21. Avizienyte E, Loukola A, Roth S, Hemminki A, Tarkkanen M, Salovaara R, et al. LKB1 somatic mutations in sporadic tumors. Am J Pathol. (1999) 154:677–81. doi: 10.1016/S0002-9440(10)65314-X
22. Caiola E, Falcetta F, Giordano S, Marabese M, Garassino MC, Broggini M, et al. Co-occurring KRAS mutation/LKB1 loss in non-small cell lung cancer cells results in enhanced metabolic activity susceptible to caloric restriction: an in vitro integrated multilevel approach. J Exp Clin Cancer Res. (2018) 37:302. doi: 10.1186/s13046-018-0954-5
23. Ndembe G, Intini I, Perin E, Marabese M, Caiola E, Mendogni P, et al. LKB1: can we target an hidden target? Focus on NSCLC. Front Oncol. (2022) 12:889826. doi: 10.3389/fonc.2022.889826
24. Skoulidis F, Goldberg ME, Greenawalt DM, Hellmann MD, Awad MM, Gainor JF, et al. STK11/LKB1 mutations and PD-1 inhibitor resistance in KRAS-mutant lung adenocarcinoma. Cancer Discovery. (2018) 8:822–35. doi: 10.1158/2159-8290.CD-18-0099
25. Bange E, Marmarelis ME, Hwang WT, Yang YX, Thompson JC, Rosenbaum J, et al. Impact of KRAS and TP53 co-mutations on outcomes after first-line systemic therapy among patients with STK11-mutated advanced non-small-cell lung cancer. JCO Precis Oncol. (2019) 3. doi: 10.1200/PO.18.00326
26. Jenne DE, Reimann H, Nezu J, Friedel W, Loff S, Jeschke R, et al. Peutz-Jeghers syndrome is caused by mutations in a novel serine threonine kinase. Nat Genet. (1998) 18:38–43. doi: 10.1038/ng0198-38
27. Kang J, Gallucci S, Pan J, Oakhill JS, Sanij E. The role of STK11/LKB1 in cancer biology: implications for ovarian tumorigenesis and progression. Front Cell Dev Biol. (2024) 12. doi: 10.3389/fcell.2024.1449543
28. Boudeau J, Baas AF, Deak M, Morrice NA, Kieloch A, Schutkowski M, et al. MO25alpha/beta interact with STRADalpha/beta enhancing their ability to bind, activate and localize LKB1 in the cytoplasm. EMBO J. (2003) 22:5102–14. doi: 10.1093/emboj/cdg490
29. Baas AF, Boudeau J, Sapkota GP, Smit L, Medema R, Morrice NA, et al. Activation of the tumour suppressor kinase LKB1 by the STE20-like pseudokinase STRAD. EMBO J. (2003) 22:3062–72. doi: 10.1093/emboj/cdg292
30. Ricciuti B, Mira A, Andrini E, Scaparone P, Michelina SV, Pecci F, et al. How to manage KRAS G12C-mutated advanced non-small-cell lung cancer. Drugs Context. (2022) 11. doi: 10.7573/dic.2022-7-4
31. Rosell R, Codony-Servat J, González J, Santarpia M, Jain A, Shivamallu C, et al. KRAS G12C-mutant driven non-small cell lung cancer (NSCLC). Crit Rev Oncol/Hematol. (2024) 195:104228. doi: 10.1016/j.critrevonc.2023.104228
32. Jones GD, Caso R, Tan KS, Mastrogiacomo B, Sanchez-Vega F, Liu Y, et al. KRAS (G12C) mutation is associated with increased risk of recurrence in surgically resected lung adenocarcinoma. Clin Cancer Res. (2021) 27:2604–12. doi: 10.1158/1078-0432.CCR-20-4772
33. Cox AD, Fesik SW, Kimmelman AC, Luo J, Der CJ. Drugging the undruggable RAS: Mission possible? Nat Rev Drug Discovery. (2014) 13:828–51.
34. Takashima A, Faller DV. Targeting the RAS oncogene. Expert Opin Ther Targets. (2013) 17:507–31. doi: 10.1517/14728222.2013.764990
35. Chen K, Zhang Y, Qian L, Wang P. Emerging strategies to target RAS signaling in human cancer therapy. J Hematol Oncol. (2021) 14:116. doi: 10.1186/s13045-021-01127-w
36. Canon J, Rex K, Saiki AY, Mohr C, Cooke K, Bagal D, et al. The clinical KRAS(G12C) inhibitor AMG 510 drives anti-tumour immunity. Nature. (2019) 575:217–23. doi: 10.1038/s41586-019-1694-1
37. Huang L, Guo Z, Wang F, Fu L. KRAS mutation: from undruggable to druggable in cancer. Signal Transduction Targeted Ther. (2021) 6:386. doi: 10.1038/s41392-021-00780-4
38. Torres-Jiménez J, Espinar JB, de Cabo HB, Berjaga MZ, Esteban-Villarrubia J, Fraile JZ, et al. Targeting KRAS(G12C) in non-small-cell lung cancer: current standards and developments. Drugs. (2024) 84:527–48.
39. Santarpia M, Ciappina G, Spagnolo CC, Squeri A, Passalacqua MI, Aguilar A, et al. Targeted therapies for KRAS-mutant non-small cell lung cancer: from preclinical studies to clinical development-a narrative review. Transl Lung Cancer Res. (2023) 12:346–68. doi: 10.21037/tlcr-22-639
40. Cucurull M, Notario L, Sanchez-Cespedes M, Hierro C, Estival A, Carcereny E, et al. Targeting KRAS in lung cancer beyond KRAS G12C inhibitors: the immune regulatory role of KRAS and novel therapeutic strategies. Front Oncol. (2021) 11:793121. doi: 10.3389/fonc.2021.793121
41. Liu J, Kang R, Tang D. The KRAS-G12C inhibitor: activity and resistance. Cancer Gene Ther. (2022) 29:875–8. doi: 10.1038/s41417-021-00383-9
42. Awad MM, Liu S, Rybkin II, Arbour KC, Dilly J, Zhu VW, et al. Acquired resistance to KRAS(G12C) inhibition in cancer. N Engl J Med. (2021) 384:2382–93. doi: 10.1056/NEJMoa2105281
43. Mohanty A, Nam A, Srivastava S, Jones J, Lomenick B, Singhal SS, et al. Acquired resistance to KRAS G12C small-molecule inhibitors via genetic/nongenetic mechanisms in lung cancer. Sci Adv. (2023) 9:eade3816. doi: 10.1126/sciadv.ade3816
44. Di Federico A, Ricciotti I, Favorito V, Michelina SV, Scaparone P, Metro G, et al. Resistance to KRAS G12C inhibition in non-small cell lung cancer. Curr Oncol Rep. (2023) 25:1017–29. doi: 10.1007/s11912-023-01436-y
45. Lietman CD, Johnson ML, McCormick F, Lindsay CR. More to the RAS story: KRAS(G12C) inhibition, resistance mechanisms, and moving beyond KRAS(G12C). Am Soc Clin Oncol Educ Book. (2022) 42:1–13.
46. Sajjad H, Imtiaz S, Noor T, Siddiqui YH, Sajjad A, Zia M. Cancer models in preclinical research: A chronicle review of advancement in effective cancer research. Anim Model Exp Med. (2021) 4:87–103. doi: 10.1002/ame2.12165
47. Day CP, Merlino G, Van Dyke T. Preclinical mouse cancer models: a maze of opportunities and challenges. Cell. (2015) 163:39–53. doi: 10.1016/j.cell.2015.08.068
48. Gengenbacher N, Singhal M, Augustin HG. Preclinical mouse solid tumour models: status quo, challenges and perspectives. Nat Rev Cancer. (2017) 17:751–65. doi: 10.1038/nrc.2017.92
49. Li L, Wazir J, Huang Z, Wang Y, Wang H. A comprehensive review of animal models for cancer cachexia: Implications for translational research. Genes Dis. (2024) 11:101080. doi: 10.1016/j.gendis.2023.101080
50. Dhumal A, Bendale K, Chaudhari P. Preclinical animal models for cancer research and drug discovery. In: Bose K, Chaudhari P, editors. Unravelling Cancer Signaling Pathways: A Multidisciplinary Approach. Springer Singapore, Singapore (2019). p. 229–54.
51. Shakweer WME, Krivoruchko AY, Dessouki SM, Khattab AA. A review of transgenic animal techniques and their applications. J Genet Eng Biotechnol. (2023) 21:55. doi: 10.1186/s43141-023-00502-z
52. Lampreht Tratar U, Horvat S, Cemazar M. Transgenic mouse models in cancer research. Front Oncol. (2018) 8:268. doi: 10.3389/fonc.2018.00268
53. Jackson EL, Willis N, Mercer K, Bronson RT, Crowley D, Montoya R, et al. Analysis of lung tumor initiation and progression using conditional expression of oncogenic K-ras. Genes Dev. (2001) 15:3243–8. doi: 10.1101/gad.943001
54. Abate-Shen C. A new generation of mouse models of cancer for translational research. Clin Cancer Res. (2006) 12:5274–6. doi: 10.1158/1078-0432.CCR-06-0500
55. Zhou Y, Xia J, Xu S, She T, Zhang Y, Sun Y, et al. Experimental mouse models for translational human cancer research. Front Immunol. (2023) 14:1095388. doi: 10.3389/fimmu.2023.1095388
56. Carretero J, Shimamura T, Rikova K, Jackson AL, Wilkerson MD, Borgman CL, et al. Integrative genomic and proteomic analyses identify targets for Lkb1-deficient metastatic lung tumors. Cancer Cell. (2010) 17:547–59. doi: 10.1016/j.ccr.2010.04.026
57. Ndembe G, Intini I, Moro M, Grasselli C, Panfili A, Panini N, et al. Caloric restriction and metformin selectively improved LKB1-mutated NSCLC tumor response to chemo- and chemo-immunotherapy. J Exp Clin Cancer Res. (2024) 43:6. doi: 10.1186/s13046-023-02933-5
58. Gilbert-Ross M, Konen J, Koo J, Shupe J, Robinson BS, WGt W, et al. Targeting adhesion signaling in KRAS, LKB1 mutant lung adenocarcinoma. JCI Insight. (2017) 2:e90487. doi: 10.1172/jci.insight.90487
59. Ji H, Ramsey MR, Hayes DN, Fan C, McNamara K, Kozlowski P, et al. LKB1 modulates lung cancer differentiation and metastasis. Nature. (2007) 448:807–10. doi: 10.1038/nature06030
60. Sanchez-Cespedes M. The role of LKB1 in lung cancer. Familial Cancer. (2011) 10:447–53. doi: 10.1007/s10689-011-9443-0
61. Mahoney CL, Choudhury B, Davies H, Edkins S, Greenman C, Haaften G, et al. LKB1/KRAS mutant lung cancers constitute a genetic subset of NSCLC with increased sensitivity to MAPK and mTOR signalling inhibition. Br J Cancer. (2009) 100:370–5. doi: 10.1038/sj.bjc.6604886
62. Meuwissen R, Linn SC, van der Valk M, Mooi WJ, Berns A. Mouse model for lung tumorigenesis through Cre/lox controlled sporadic activation of the K-Ras oncogene. Oncogene. (2001) 20:6551–8. doi: 10.1038/sj.onc.1204837
63. Schindelin J, Arganda-Carreras I, Frise E, Kaynig V, Longair M, Pietzsch T, et al. Fiji: an open-source platform for biological-image analysis. Nat Methods. (2012) 9:676–82. doi: 10.1038/nmeth.2019
64. Yushkevich PA, Yang G, Gerig G. ITK-SNAP: An interactive tool for semi-automatic segmentation of multi-modality biomedical images. Annu Int Conf IEEE Eng Med Biol Soc. (2016) 2016:3342–5. doi: 10.1109/EMBC.2016.7591443
65. Iannello F. Non-intrusive high throughput automated data collection from the home cage. Heliyon. (2019) 5:e01454. doi: 10.1016/j.heliyon.2019.e01454
66. Nagy A. Cre recombinase: the universal reagent for genome tailoring. Genesis. (2000) 26:99–109. doi: 10.1002/(SICI)1526-968X(200002)26:2<99::AID-GENE1>3.0.CO;2-B
67. Beaudry AG, Law ML. Variable body and tissue weight reporting in preclinical cachexia literature may alter study outcomes and interpretation. Dis Model Mech. (2023) 16. doi: 10.1242/dmm.050148
68. Wu Q, Zou S, Liu W, Liang M, Chen Y, Chang J, et al. A novel onco-cardiological mouse model of lung cancer-induced cardiac dysfunction and its application in identifying potential roles of tRNA-derived small RNAs. BioMed Pharmacother. (2023) 165:115117. doi: 10.1016/j.biopha.2023.115117
69. Pernold K, Iannello F, Low BE, Rigamonti M, Rosati G, Scavizzi F, et al. Towards large scale automated cage monitoring - Diurnal rhythm and impact of interventions on in-cage activity of C57BL/6J mice recorded 24/7 with a non-disrupting capacitive-based technique. PloS One. (2019) 14:e0211063. doi: 10.1371/journal.pone.0211063
70. Rulli E, Serafini MS, Marabese M, Caiola E, Sozzi G, Moro M, et al. Co-existance of KRAS and LKB1 mutation as predictor of resistance to Erlotinib: Customized next-generation sequencing (NGS) of TAILOR trial. J Clin Oncol. (2017) 35:e20631–e. doi: 10.1200/JCO.2017.35.15_suppl.e20631
71. Meraz IM, Majidi M, Shao R, Meng F, Ha MJ, Shpall E, et al. TUSC2 immunogene enhances efficacy of chemo-immuno combination on KRAS/LKB1 mutant NSCLC in humanized mouse model. Commun Biol. (2022) 5:167. doi: 10.1038/s42003-022-03103-7
72. Baran SW, Bratcher N, Dennis J, Gaburro S, Karlsson EM, Maguire S, et al. Emerging role of translational digital biomarkers within home cage monitoring technologies in preclinical drug discovery and development. Front Behav Neurosci. (2021) 15:758274. doi: 10.3389/fnbeh.2021.758274
73. Gaburro S, Winter Y, Loos M, Kim JJ, Stiedl O. Editorial: home cage-based phenotyping in rodents: innovation, standardization, reproducibility and translational improvement. Front Neurosci. (2022) 16:894193. doi: 10.3389/fnins.2022.894193
74. Voikar V, Gaburro S. Three pillars of automated home-cage phenotyping of mice: novel findings, refinement, and reproducibility based on literature and experience. Front Behav Neurosci. (2020) 14:575434. doi: 10.3389/fnbeh.2020.575434
75. Winn CB, Hwang SK, Morin J, Bluette CT, Manickam B, Jiang ZK, et al. Automated monitoring of respiratory rate as a novel humane endpoint: A refinement in mouse metastatic lung cancer models. PloS One. (2021) 16:e0257694. doi: 10.1371/journal.pone.0257694
76. Do JP, Defensor EB, Ichim CV, Lim MA, Mechanic JA, Rabe MD, et al. Automated and continuous monitoring of animal welfare through digital alerting. Comp Med. (2020) 70:313–27. doi: 10.30802/AALAS-CM-19-000090
77. Carrillo M, Migliorati F, Bruls R, Han Y, Heinemans M, Pruis I, et al. Repeated witnessing of conspecifics in pain: effects on emotional contagion. PloS One. (2015) 10:e0136979. doi: 10.1371/journal.pone.0136979
Keywords: KRAS/LKB1, NSCLC, locomotion, home cage monitoring, biomarker, transgenic animal models, MRI, translational models
Citation: Tomanelli M, Guffanti F, Vargiu G, Micotti E, Rigamonti M, Tumiatti F, Caiola E, Marabese M and Broggini M (2024) Detection of aberrant locomotor activity in a mouse model of lung cancer via home cage monitoring. Front. Oncol. 14:1504938. doi: 10.3389/fonc.2024.1504938
Received: 04 October 2024; Accepted: 05 December 2024;
Published: 23 December 2024.
Edited by:
Oliver Stiedl, Vrije Universiteit, NetherlandsReviewed by:
Leonardo Restivo, Université de Lausanne, SwitzerlandSteven R. Talbot, Hannover Medical School, Germany
Anupam Sah, Innsbruck Medical University, Austria
Anna Kiryk, Polish Academy of Sciences, Poland
Copyright © 2024 Tomanelli, Guffanti, Vargiu, Micotti, Rigamonti, Tumiatti, Caiola, Marabese and Broggini. This is an open-access article distributed under the terms of the Creative Commons Attribution License (CC BY). The use, distribution or reproduction in other forums is permitted, provided the original author(s) and the copyright owner(s) are credited and that the original publication in this journal is cited, in accordance with accepted academic practice. No use, distribution or reproduction is permitted which does not comply with these terms.
*Correspondence: Massimo Broggini, bWFzc2ltby5icm9nZ2luaUBtYXJpb25lZ3JpLml0; Michele Tomanelli, bWljaGVsZXRvbWFuZWxsaTE5ODhAZ21haWwuY29t