- 1Nursing Department, Dazhou Central Hospital, Dazhou, Sichuan, China
- 2Department of Oncology, Dazhou Central Hospital, Dazhou, Sichuan, China
- 3Cardiac Vascular Surgery, Dazhou Central Hospital, Dazhou, Sichuan, China
- 4Department of Oncology, Dazhou Integrated Traditional Chinese Medicine (TCM) and Western Medicine Hospital, Dazhou, Sichuan, China
- 5Department of Oncology, Affiliated Hospital of North Sichuan Medical College, Nanchong, Sichuan, China
Purpose: The paper aims to investigate the factors influencing cancer-related fatigue (CRF) in lung cancer patients and construct a CRF risk prediction model, providing effective intervention strategies for clinical medical staff.
Methods: This paper employs convenience sampling to select 400 lung cancer patients who visited a tertiary hospital in Dazhou, Sichuan Province, from January 2021 to January 2022. A questionnaire survey was conducted using the Revised Piper Fatigue Scale (PFS-R), Pittsburgh Sleep Quality Index (PSQI), and Hospital Anxiety and Depression Scale (HADS) to collect data on patient demographics and sociological characteristics, disease-related information, physiological indicators, sleep quality, mental health, and other relevant factors. To explore the factors influencing CRF in lung cancer patients, single-factor analysis and multiple logistic regression analysis were performed. A CRF risk prediction model was then established, with its predictive performance and calibration evaluated using ROC curves.
Findings: The results of multivariate logistic regression analysis showed that gender, age, education level, living status, daily exercise, clinical stage, course of disease, treatment mode, chronic disease, BMI, hemoglobin, serum albumin, blood glucose, potassium concentration, magnesium concentration, PSQI score and HAD score were the influencing factors of CRF in lung cancer patients (P<0.05). The AUC of the model construction group and the model validation group were 0.863 and 0.838, respectively, and the results of Hosmer-Lemeshow fit test showed that χ2 = 7.540, P=0.378>0.05 of the model construction group and χ2 = 8.120, P=0.320>0.05 of the model validation group indicated that the model had high prediction accuracy.
Originality/value: The risk prediction model for CRF holds significant clinical value. It can help medical staff to promptly identify high-risk patients, develop personalized intervention strategies, alleviate fatigue symptoms, and improve overall patient quality of life.
1 Introduction
As one of the common malignant tumors in clinical practice, lung cancer originates from lung glands or bronchial mucosa (1–3). According to the latest cancer epidemiology data from China for 2022, lung cancer has the highest incidence and mortality rates among both men and women. In 2022, there were 1.0606 million new cases of lung cancer, accounting for 22.0% of all new malignant tumors, and 733,300 deaths, representing 28.5% of all malignant tumors-related deaths (4). It is worth noting that Galvez Nino et al. (5) observed a significant decrease in the median age of diagnosis from 70 years to 36 years, indicating a troubling trend toward younger age groups. This shift emphasizes the urgent need for more attention to be paid to the health management of lung cancer survivors.
Cancer-related fatigue (CRF) is a debilitating condition associated with cancer and its treatment, characterized by persistent, subjective, physical, emotional, or cognitive exhaustion that is disproportionate to recent activity levels and cannot be alleviated by rest or sleep (6). Recent research shows that CRF in lung cancer patients is generally moderate, with lower family income, prolonged chemotherapy cycles, poor psychological resilience, sleep disorders, and high anxiety contributing to more severe CRF (7). Therefore, exploring the factors influencing CRF in lung cancer patients and developing a risk prediction model is crucial. Current studies have identified key factors and trajectories affecting perioperative CRF in lung cancer patients. For instance, patients who are overweight or obese or who have low psychological resilience, are more prone to persistent fatigue. Additionally, patients with a history of smoking and inadequate social support are associated with exacerbated fatigue symptoms (8). A study by Aihua et al. (9) further identified several risk factors for CRF in patients undergoing chemotherapy for non-small cell lung cancer, including age ≥ 60 years, female, living alone, TNM stage III-IV, poor sleep quality, depressive mood, more than two chemotherapy sessions, FEV 1% < 70%, anemia, pain, nausea, and vomiting. Developing a risk prediction model based on these risk factors could enhance screening and prevention strategies for high-risk patients. However, existing research on prediction models is limited, with most studies focusing mainly on clinical indicators and insufficiently addressing the comprehensive physical and mental factors affecting CRF in lung cancer patients (10, 11). This study, therefore, aims to address this gap by adopting a holistic approach, using the Rived Piper Fatigue Scale to evaluate the degree of CRF in lung cancer patients from four dimensions: behavior, cognition, sensation, and emotion. This study takes into account 32 potential risk factors, including demographic and sociological characteristics, disease-related information, physiological indicators, sleep quality, and mental health, in developing a practical risk prediction model. The predictive factors of this model are relatively easy to obtain in clinical practice, reducing the workload of medical staff while enabling timely identification of high-risk patients, and providing new insights into effective intervention strategies for reducing CRF in lung cancer patients.
2 Materials and methods
2.1 Research objects
Convenience sampling was used to select 400 lung cancer patients who met the inclusion criteria and visited the oncology, respiratory medicine, thoracic surgery, and other departments of Dazhou Central Hospital, Sichuan Province, from January 2021 to January 2022. This study has been approved by the Ethics Committee of this hospital, with informed consent from all participants.
2.2 Sample size estimation
Based on the sample size estimation method for multiple factor logistic regression analysis and a review of relevant literature, a total of 32 variables were identified, with a sample size of 5-10 times the number of variables. Considering a 20% rate of invalid samples, the study determined that a minimum of 388 cases was necessary. Of these, 70% (272 cases) were allocated to establish the risk prediction model, while 30% (116 cases) were reserved for model validation.
2.3 Diagnostic criteria
(1) The diagnostic criteria for lung cancer are based on the “Chinese Medical Association Guidelines for Clinical Diagnosis and Treatment of Lung Cancer (Edition 2018)” (12) (2). The pathological classification of lung cancer adheres to the 2015 World Health Organization (WHO) Classification of Tumors of the Lung, Pleura, Thymus and Heart (13) (3). Staging of lung cancer follows the 8th edition of the UICC staging system phases I-IV (14) (4). The diagnostic criteria for CRF are guided by “Chinese Expert Consensus on the Diagnosis and Treatment of Cancer-Associated Fatigue” (15), and are validated using the Revised Piper Fatigue Scale (PFS-R).
2.4 Inclusion criteria (those who meet the following four conditions are included)
(1) Inpatients diagnosed with primary lung cancer or those with a diagnosis of primary lung cancer through clinical histopathology; Age ≥ 18 years (2). Functional status rating criteria (Karnofsky, KPS) ≥ 60 points (3). Diagnosed with CRF (4). Expected survival period greater than 3 months (5). The patient is conscious and voluntarily agrees to participate in the questionnaire survey.
2.5 Exclusion criteria (excluding those who meet any of the following criteria)
(1) Secondary lung cancer or concurrent presence of other cancers (2). Patients with severe complications such as heart, liver, kidney, hematopoietic system (3). Patients with cognitive impairment (4). Patients under confidential medical treatment.
2.6 Research tool
2.6.1 General information survey form
This form collected data on both general and disease-specific characteristics of lung cancer patients namely:
1. Demographic information: Gender (male/female), Age (≥ 65 years/<65 years), Education Level (primary school/junior high school/vocational school or above), Employment Status (employed/retired), Marital Status (unmarried/married/widowed), Living Situation (living alone/with family), Satisfaction with Housing (poor/average/good), Payment Method (self-funded/medical insurance), Monthly Family Income (≥ 5000 yuan/<5000 yuan), Daily Exercise Frequency (≥ 1 time/<1 time).
2. Clinical Information: Pathological Type (squamous cell carcinoma/adenocarcinoma/small cell lung cancer/other), Clinical Stage (phase I/II/III/IV), Disease Course (in days), Necessity of Surgery (yes/no), Treatment Methods (surgery/radiotherapy/chemotherapy/palliative care).
3. Physiological Indicators: Pain Score (using a digital rating scale, ranging from 0-10 points, where 0 indicates no pain and 10 indicates the most severe pain), Complications (yes/no), Chronic Diseases (yes/no), BMI (kg/m2), Erythrocyte (×1012/L), Hemoglobin (g/L), Platelets (×109/L), Serum Albumin (g/L), Lymphocyte Count (×109/L), Lymphocyte Ratio (%), Blood Pressure [systolic blood pressure (mmHg)/diastolic blood pressure (mmHg)], Blood Glucose (mmol/L), Potassium Ion Concentration (mmol/L), Magnesium Ion Concentration (mmol/L).
2.6.2 PFS-R
PFS-R consists of 22 items and 4 dimensions (behavior, emotion, sensation, cognition). It is scored using an 11-point Likert scale, where 0 indicates no change and 10 indicates very severe fatigue. The total score is the average of the scores across these 4 dimensions. Fatigue severity is categorized as follows: mild fatigue (0 to 3.3 points), Moderate fatigue (3.4-6.7 points), and severe fatigue (6.8-10 points). The scale has demonstrated high reliability, with a test-retest reliability of 0.98 and a Cronbach’s alpha coefficient of 0.91.
2.6.3 Pittsburgh sleep quality index
PSQI mainly reflects the patients’ sleep status over the past month, comprising 19 items across 7 dimensions. Each dimension is scored on a 4-point scale ranging from 0 to 3. A total score greater than 7 indicates sleep disorders, with higher scores indicating more severe sleep disorders. The scale has a Cronbach’s alpha coefficient of 0.87, indicating good reliability.
2.6.4 Hospital anxiety and depression scale
HADS consists of 14 questions, with odd-numbered questions assessing anxiety and even-numbered questions assessing depression. Each question offers 4 options, scored from 0 to 3. A score >7 indicates suspicious anxiety or depression, with higher scores indicating more severe symptoms. The overall Cronbach’s alpha coefficient for the scale is 0.785, while the anxiety and depression subscales have Cronbach’s alpha coefficients of 0.72 and 0.68, respectively.
2.7 Data collection
Before the investigation, we formed a team of investigators, consisting of oncology and nursing experts and researchers, who provided standardized training to the team members. The training comprised two components: theoretical and practical skills training. Theoretical training covered relevant topics on lung cancer and CRF, including concepts, epidemiology, clinical manifestations, identification, and evaluation. Practical skills training focused on determining survey questionnaire items, investigation techniques, and necessary precautions. Only after passing the assessment could the investigation work be carried out to ensure standardization and consistency of the investigation. During the survey, with the patient’s informed consent and voluntary participation, the purpose and significance of this study were explained, and patients were informed of their right to voluntarily complete the questionnaire and withdraw at any time. After obtaining consent, the investigators guided the patients to fill out PFS-R, PSQI, and HADS within 3 days of admission, appropriately explaining the meaning, content, and methods of the questionnaire. Patients were instructed to answer truthfully based on their personal situation without fabrication. For patients with difficulty understanding the survey or with many questions, the investigators explained each item and assisted in completing it. This study used a paper version of the questionnaire or Wenjuanxing for data collection. When collecting questionnaires, investigators checked them on the spot for any missing or incomplete items, and promptly inquired about corrections to reduce invalid questionnaires. Starting in January 2022, data from patients admitted for re-examination were collected only once during hospitalization.
2.8 Statistics
This study completed all statistical analyses using SPSS27.0 software. Quantitative data conforming to a normal distribution were represented by ( ± s). Independent sample t-test was used for comparison between two groups, and Pearson correlation analysis was used for correlation analysis. Non-normally distributed quantitative data were represented by M (P25, P75). Mann-Whitney U rank sum test was used for inter-group comparisons, and Spearman correlation analysis was used for correlation analysis. Categorical data were expressed in logarithmic form, with group comparison performed using the χ2 test. Univariate and multivariate logistic regression analyses were conducted to identify the factors influencing CRF in lung cancer patients and to establish a risk prediction model. The discriminative ability of the model was evaluated using the area under the ROC curve (AUC), sensitivity, specificity, Youden index, and other metrics. The Hosmer Lemeshow fit test was used to validate the calibration of the model, with a significant level of P<0.05 considered statistically significant.
3 Results
3.1 General information of lung cancer patients
This study included 400 patients, which met the sample size requirements. Based on the proportion of risk prediction model construction and validation, 280 patients were included in the model construction group and 120 patients were included in the model validation group. There was no statistically significant difference (P>0.05) in all clinical data between the model construction group and the model validation group, as shown in Table 1. The incidence of CRF in the model construction group was 68.8% (193/280). The study subjects were divided into the CRF group (n=192) and NO-CRF group (n=88) based on the occurrence of CRF. In the CRF group, according to the PFS-R scoring criteria, the mild fatigue rate was 17.7% (34/192), the moderate fatigue rate was 51.0% (98/192) and the severe fatigue rate was 31.3% (60/192).
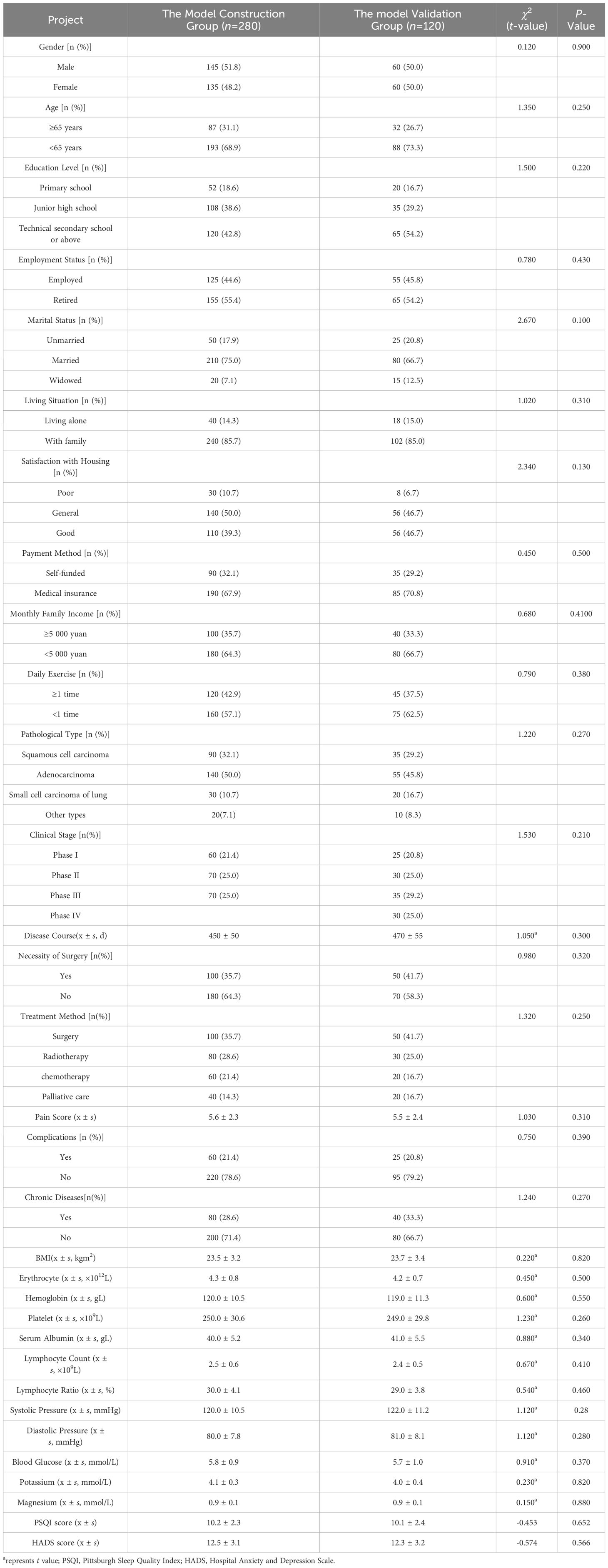
Table 1. The comparison of clinical data between the model construction group and the model validation group.
3.2 Univariate analysis of factors influencing CRF in lung cancer patients in the model construction group
There was a statistically significant difference (P<0.05) between the CRF group and NO-CRF group with respect to gender, age, education level, living situation, Satisfaction with Housing, daily exercise, clinical stage, disease duration, treatment method, presence of chronic diseases, BMI, hemoglobin, serum albumin, blood glucose, potassium ion concentration, magnesium ion concentration, PSQI score, and HADS score, as shown in Table 2.
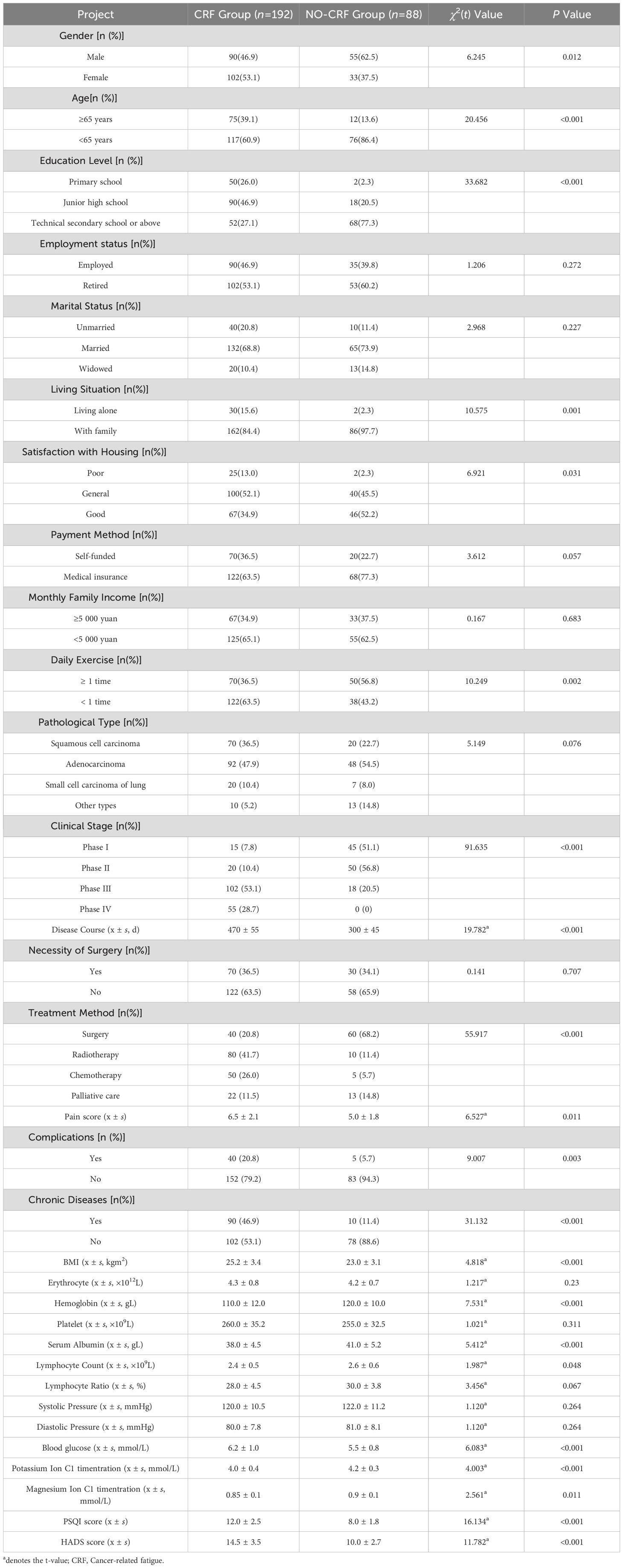
Table 2. Univariate analysis of factors influencing CRF in lung cancer patients in the model construction group.
3.3 Multivariate logistic regression analysis of factors influencing CRF in lung cancer patients in the model construction group
Multiple logistic regression analysis was conducted using the presence of CRF in lung cancer patients as the dependent variable (0=no, 1=yes) and the statistically significant variables from Table 2 as independent variables (assigned values are shown in Table 3). The results showed that gender, age, education level, living situation, daily exercise, clinical stage, disease duration, treatment method, presence of chronic diseases, BMI, hemoglobin, serum albumin, blood glucose, potassium ion concentration, magnesium ion concentration, PSQI score, and HADS score were significant factors influencing CRF in lung cancer patients (P<0.05), as shown in Table 4.
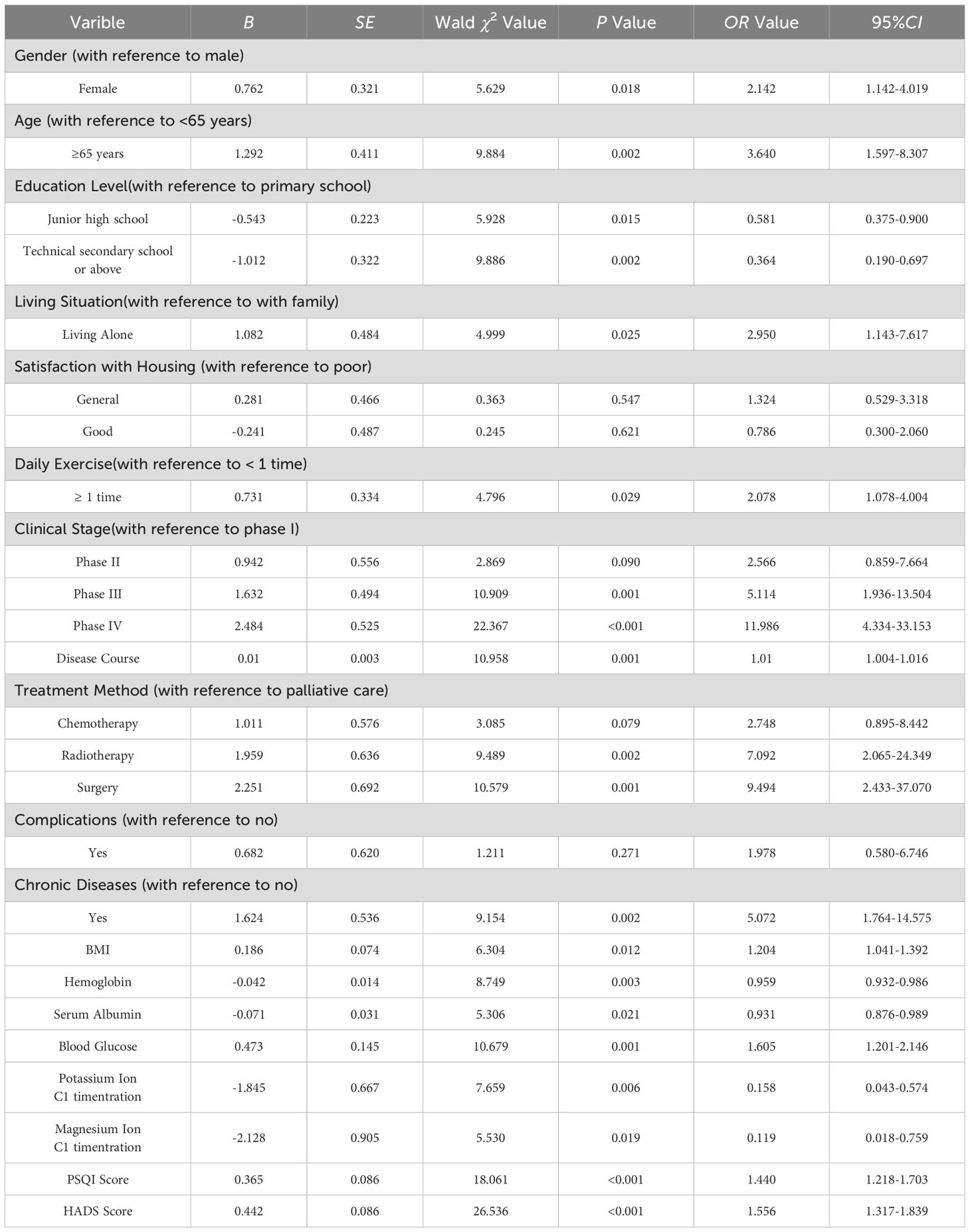
Table 4. Multivariate logistic regression analysis of the factors influencing CRF in lung cancer patients in the model construction group.
According to the results of multiple logistic regression analysis, the risk prediction model for CRF in lung cancer patients can be expressed by the following equation:
logit (P)=0.762 × gender+1.292 × age -0.543 × junior high school education level -1.012 × technical secondary school education level or above+1.082 × living alone+0.731 × daily exercise status+0.942 × phase II +1.632 × phase III +2.484 × phase IV +0.010 × disease course+1.011 × chemotherapy+1.959 × radiotherapy+2.251 × surgery+1.624 × chronic disease+0.186 × BMI -0.042 × hemoglobin -0.071 × serum albumin+0.473 × blood glucose -1.845 × potassium ion concentration -2.128 × magnesium ion concentration+0.365 x PSQI score+0.442 x HADS score.
3.4 Analysis of the predictive effect of CRF prediction model for lung cancer patients
The AUC values for the model construction group and the model validation group were 0.863 and 0.838, respectively. The sensitivity, specificity, and Youden index results are shown in Table 5, with the ROC curves shown in Figures 1 and 2. The Hosmer Lemeshow fitting test results indicated that the model construction group had a χ2 value of 7.54, P=0.378>0.05, while the model validation group had a χ2 value of 8.12, P=0.320>0.05. These results suggest no statistically significant difference between the predicted values and the actual measurement of the model, indicating that the prediction model has good calibration ability.

Table 5. Diagnostic value of CRF prediction model in model construction group and model validation group.
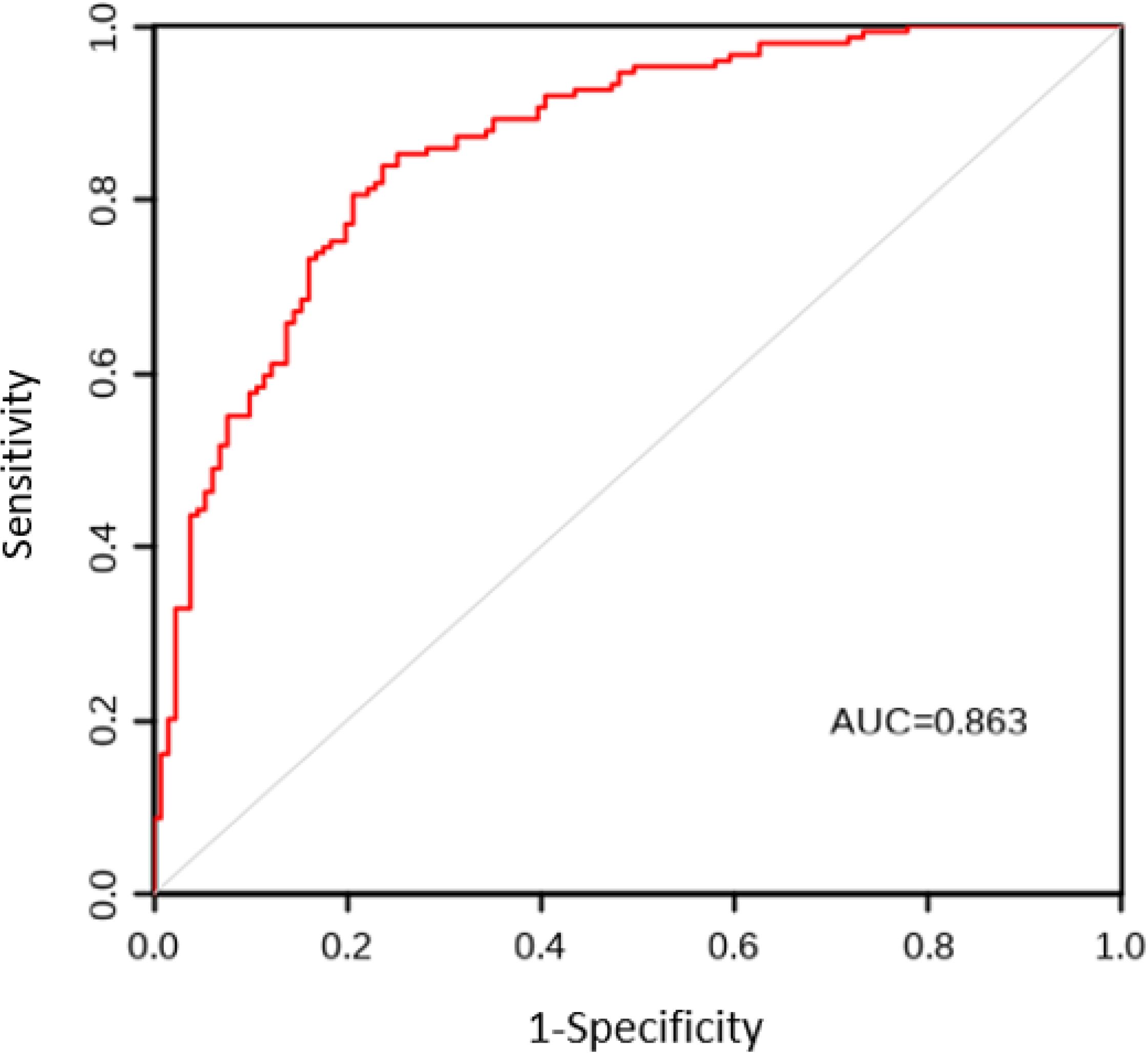
Figure 1. ROC curve of CRF prediction model for lung cancer patients in the model construction group. ROC, Subjects’ Functional Curve; AUC, Area Under ROC Curve; CRF, Cancer-related Fatigue.
4 Discussions
CRF is one of the common adverse reactions in the treatment of cancer patients, which seriously affects their quality of life and treatment effectiveness (16). The mechanism of CRF is complex and involves multiple biological and psychological factors (17). In recent years, an increasing number of studies have explored the biological mechanisms of CRF, including anemia, abnormal cytokine regulation, abnormal hypothalamic pituitary adrenal axis regulation, abnormal regulation of 5-hydroxytryptophan neurotransmitter, and changes in ATP and muscle metabolism (18).The most prominent hypothesis among scholars is the dysregulation of pro-inflammatory cytokines, known as the inflammation hypothesis (19, 20). However, there is still no unified understanding of the exact pathogenesis of CRF.
The occurrence and development of CRF are influenced by multiple factors (21). Weis et al. (22) found that 59% to 100% of cancer patients experience CRF. Common cancer treatments such as surgery, radiotherapy, chemotherapy, and biological therapy have varying degrees of impact on CRF (23). Although CRF may occur before cancer treatment, it typically worsens during radiotherapy, chemotherapy, surgery, or biological therapy. Berger et al. (24) reported that cancer patients undergoing radiotherapy, chemotherapy, and combined treatments had CRF incidence rates of 30% to 91%, 25% to 93%, and 59% to 93%, respectively. However, research into the factors influencing CRF in liver cancer patients remains scarce. In this study, variables such as gender, age, educational level, living situation, daily exercise habits, clinical stage, disease course, treatment method, presence of chronic conditions, BMI, hemoglobin levels, serum albumin, glucose, potassium ion concentration, magnesium ion concentration, PSQI score, and HADS score were incorporated into a multivariate logistic regression analysis to ascertain their influence on CRF in lung cancer patients.
Firstly, gender significantly affects CRF, with female patients being more likely to develop CRF than male patients (25). This may be related to women being more sensitive to pain and fatigue, and having poorer psychological resilience. Yan Wenjing et al. (26) found in a cluster analysis of 220 hospitalized lung cancer chemotherapy patients that female patients exhibited fatigue, negative emotion, pain, and sleep disorder syndrome more frequently. Age is also a significant factor, with older patients (≥ 65 years old) more likely to develop CRF. Elderly patients with decreased physical function are more prone to malnutrition and anemia, coupled with heavy psychological burden and more pronounced fatigue (27). Jacobsen et al. (28) found that the incidence and severity of fatigue in cancer patients with hemoglobin levels<12 g/dL after chemotherapy were significantly positively correlated with hemoglobin levels.
Moreover, the influence of education level on CRF cannot be ignored. Patients with a junior high school education or below are more likely to experience fatigue than those with a technical secondary school education or above. This may be due to lower levels of education and poorer understanding and coping abilities with the disease, as well as insufficient social support (29). Patients living alone are more likely to feel lonely and fatigued due to a lack of family support and social interaction (30). The relationship between daily exercise and CRF has also been validated, with patients with lower exercise frequency having a higher incidence of CRF (31). Appropriate exercise can enhance the body’s immune system, improve mood, and reduce fatigue.
Clinical stage is another important factor, and patients with later staging (phases III-IV) have a higher incidence of CRF. This may be due to the severe condition, high treatment intensity, and heavy physical and psychological burden of late-stage patients (32, 33).
In terms of treatment methods, patients who receive chemotherapy and radiotherapy have a higher incidence of CRF. Although these treatment methods are effective, they have significant side effects that lead to physical weakness and increased fatigue in patients (34). In addition, the presence of chronic diseases increases the risk of CRF. Patients with chronic diseases, due to the presence of underlying conditions experience further limitations in their physical functions, making them more likely to feel tired (35). Physiological indicators such as BMI, hemoglobin, serum albumin, blood glucose, potassium ion concentration, and magnesium ion concentration are also closely related to CRF. Patients with low hemoglobin and serum albumin levels are more prone to fatigue due to the decreased ability of the body to transport oxygen and nutrients. (36) Hypoglycemia and electrolyte imbalances, such as low potassium and magnesium concentrations can also exacerbate fatigue (37). The impact of sleep quality and mental health status on CRF is particularly significant. Patients with poor sleep quality experience more pronounced fatigue due to their inability to recover their energy through sleep. (38)Anxiety and depression can also exacerbate the symptoms of CRF (39). Multiple studies have demonstrated a close relationship between depression and fatigue among the negative emotions affecting CRF. Research by LIN and other researchers has shown that pain, sleep disorders, negative emotions, and CRF interact with each other, leading to an increase in the incidence of CRF in cancer patients and significantly affecting their quality of life (40, 41).
In summary, the occurrence of CRF in lung cancer patients results from multiple interacting factors. Significant factors include gender, age, education level, living situation, daily exercise frequency, clinical stage, disease course, treatment methods, chronic diseases, BMI, hemoglobin, serum albumin, blood glucose, potassium ion concentration, magnesium ion concentration, sleep quality, and mental health status. The risk prediction model constructed based on these factors has important application value in clinical practice. It can help medical staff identify high-risk patients promptly, formulate personalized intervention strategies, alleviate fatigue symptoms, and improve patients’ quality of life.
While this study contributes to addressing an important research gap and offers significant clinical application value, it is not without limitations. Sample size constraints and inherent biases in observational study design may affect the generalizability of the results. Additionally, the limited time points for data collection prevented us from fully capturing all the dynamic factors that are likely to affect the development of CRF, This study only reported short-term effects and lacked long-term follow-up of patients, which is crucial for assessing the long-term effects of cancer-related fatigue. Future research should, therefore, consider larger-scale surveys and incorporating objective indicators, such as biomarkers to further validate the effectiveness and accuracy of the predictive model. Cancer-related fatigue is an important research area, more high-quality, multicenter, standardized studies are needed to provide more effective treatment strategies, exploring the specific mechanisms of CRF across different populations and its impact on personalized intervention measures will provide a more robust evidence base and support for CRF management in lung cancer patients, to improve the quality of life of cancer patients and prolong the overall survival.
Data availability statement
The datasets presented in this study can be found in online repositories. The names of the repository/repositories and accession number(s) can be found in the article/supplementary material.
Ethics statement
The studies involving humans were approved by Ethics Committee of Dazhou Central Hospital. The studies were conducted in accordance with the local legislation and institutional requirements. The participants provided their written informed consent to participate in this study. Written informed consent was obtained from the individual(s) for the publication of any potentially identifiable images or data included in this article.
Author contributions
M-NZ: Conceptualization, Data curation, Formal analysis, Funding acquisition, Investigation, Methodology, Writing – original draft, Writing – review & editing. Y-CZ: Conceptualization, Data curation, Formal analysis, Funding acquisition, Investigation, Methodology, Writing – original draft, Writing – review & editing. ZZ: Data curation, Investigation, Methodology, Writing – review & editing. C-LZ: Data curation, Investigation, Methodology, Writing – review & editing. B-TH: Writing – review & editing. G-RW: Writing – review & editing. QJ: Validation, Writing – review & editing. D-YM: Conceptualization, Data curation, Investigation, Methodology, Writing – original draft, Writing – review & editing.
Funding
The author(s) declare that no financial support was received for the research, authorship, and/or publication of this article.
Conflict of interest
The authors declare that the research was conducted in the absence of any commercial or financial relationships that could be construed as a potential conflict of interest.
Publisher’s note
All claims expressed in this article are solely those of the authors and do not necessarily represent those of their affiliated organizations, or those of the publisher, the editors and the reviewers. Any product that may be evaluated in this article, or claim that may be made by its manufacturer, is not guaranteed or endorsed by the publisher.
References
1. Li. T, Qiao T. Unraveling tumor microenvironment of small-cell lung cancer: Implications for immunotherapy. Semin Cancer Biol. (2022) 86. doi: 10.1016/j.semcancer.2022.09.005
2. Guo. W, Qiao. T, Li T. The role of stem cells in small-cell lung cancer: evidence from chemoresistance to immunotherapy. Semin Cancer Biol. (2022) 87. doi: 10.1016/j.semcancer.2022.11.006
3. Zeng. L, Liang. L, Fang. X, Xiang. S, Dai. C, Zheng. T, et al. Glycolysis induces Th2 cell infiltration and significantly affects prognosis and immunotherapy response to lung adenocarcinoma. Funct Integr Genomics. (2023) 23. doi: 10.1007/s10142-023-01155-4
4. Zheng R, Chen R, Han B, Wang S, Li L, Sun K, et al. Cancer incidence and mortality in China, 2022. Zhonghua zhong liu za zhi [Chinese J oncology]. (2024) 46:221–31. doi: 10.3760/cma.j.cn112152-20240119-00035
5. Galvez-Nino M, Ruiz R, Pinto JA, Roque K, Mantilla R, Raez LE, et al. Lung cancer in the young. Lung. (2020) 198:195–200. doi: 10.1007/s00408-019-00294-5
6. Zhang J, Qian J. Clinical practice guidelines for cancer-related fatigue in China (2021 edition). China Oncol. (2021) 31:852–72. doi: 10.19401/j.cnki.1007-3639.2021.09.012
7. Zhang R, Li M, Rao J. Analysis of cancerrelated fatigue status and influencing factors in patients with lung cancer. Chin J Lung Diseases(Electronic Edition). (2024) 17:111–4. doi: 10.3877/cma.j.issn.1674-6902.2024.01.023
8. Wen S, Liping C, Shuxiang Z, Jinjun Z. Longitudinal study on the trajectory and influencing factors of cancer-related fatigue in patients with lung cancer during perioperative period. Chin J Pract Nursing. (2022) 38:2184–90. doi: 10.3760/cma.j.cn211501-20220104-00019
9. Aihua C, Ying X, Yuping S. Construction and validation of a risk model for cancer-related fatigue in non-small cell lung cancer patients undergoing chemotherapy based on Nomogram model. J Modern Nursing. (2023) 29:1352–60. doi: 10.3760/cma.j.cn115682-20220523-02457
10. Xiang K, Luo T, & Li P. Analysis of risk factors for cancer-related fatigue in elderly patients with lung cancer undergoing chemotherapy. Electronic J Tumor Metab Nutr. (2024) 11:339–43. doi: 10.16689/j.cnki.cn11-9349/r.2024.03.009
11. Li Y, Wu Y, Jin S, Tang L, Yuan Y, & Tao X. A longitudinal study of cancer-related fatigue and its influencing factors in patients with lung cancer undergoing first chemotherapy. Chin J Nurs Manage. (2022) 22:1899–903. doi: 10.3969/j.issn.1672-1756.2022.12.027
12. Association CM. Chinese Medical Association guidelines for clinical diagnosis and treatment of lung cancer (Edition 2018). Chin J Oncol. (2018) 40:935–64. doi: 10.3760/cma.j.issn.0253-3766.2018.12.012
13. Yang X, Lin D. Changes of 2015 WHO histological classiifcation of lung cancer and the clinical signiifcance. Chin J Lung Cancer. (2016) 19:332–6. doi: 10.3779/j.issn.1009-3419.2016.06.06
14. Zhang Y, Bi J, Pi G, Zeng F, Han G, Hu D. An interpretation of revised version of international association for study of lung cancer for forthcoming 8th edition of TNM classification for lung cancer. Cancer Res Prev Treat. (2016) 43:313–8. doi: 10.3971/j.issn.1000-8578.2016.04.016
15. Rehabilitation C, Association PCPCoCAC, Support ECoT, Oncology RTotCSoC. Chinese expert consensus on diagnosis and treatment of cancer-related fatigue. Natl Med J China. (2022) 102:180–9. doi: 10.3760/cma.j.cn112137-20210811-01789
16. Perdigão MMDM, Rodrigues AB, Magalhães TDL, Freitas FMC, Bravo LG, Oliveira PPD. Educational technology for fatigue management related to antineoplastic chemotherapy. Rev Bras Enfermagem. (2019) 72:1519–25. doi: 10.1590/0034-7167-2018-0505
17. Brownstein CG, Twomey R, Temesi J, Medysky ME, Culos-Reed SN, Millet GY. Mechanisms of neuromuscular fatigability in people with cancer-related fatigue. Med Sci Sports Exercise. (2022) 54:1355–63. doi: 10.1249/MSS.0000000000002919
18. Berger AM, Mitchell SA, Jacobsen PB, Pirl WF. Screening, evaluation, and management of cancer-related fatigue: Ready for implementation to practice? CA: Cancer J Clin. (2015) 65:190–211. doi: 10.3322/caac.21268
19. Cai Y, Zhang Q, Gan C, Xu J, Yu S, Tang L, et al. Effects of managing cancer and living meaningfully on cancer-related fatigue and cytokine levels in gastrointestinal cancer patients. Integr Cancer Therapies. (2023) 22:15347354231172511. doi: 10.1177/15347354231172511
20. Li P, Wang Q, Feng L, Ding Z, Fan W. Bibliometric and visualization analyses of cancer-related fatigue research published worldwide from 2001 to 2023. Front Oncol. (2024) 14:1338325. doi: 10.3389/fonc.2024.1338325
21. Fabi A, Bhargava R, Fatigoni S, Guglielmo M, Horneber M, Roila F. Cancer-related fatigue: ESMO Clinical Practice Guidelines for diagnosis and treatment. Ann Oncol. (2020) 31:713–23. doi: 10.1016/j.annonc.2020.02.016
22. Weis J. Cancer-related fatigue: prevalence, assessment and treatment strategies. Expert Rev pharmacoeconomics outcomes Res. (2011) 11:441–6. doi: 10.1586/erp.11.44
23. Bandara V, Capp A, Ahmed G, Arm J, Martin J. Assessment and predictors of fatigue in men with prostate cancer receiving radiotherapy and androgen deprivation therapy. J Med Imaging Radiat Oncol. (2019) 63:683–90. doi: 10.1111/1754-9485.12922
24. Berger AM, Mooney K, Alvarez-Perez A, Breitbart WS, Carpenter KM, Cella D, et al. Cancer-related fatigue, version 2.2015. J Natl Compr Cancer Network. (2015) 13:1012–39. doi: 10.6004/jnccn.2015.0122
25. Gu S, Xu Y, Zhu X, Lam A, Yi D, Gong L, et al. Characteristics of cancer-related fatigue and its correlation with anxiety, depression, and stress-related hormones among Chinese cancer survivors: a cross-sectional study. Front Oncol. (2023) 13:1194673. doi: 10.3389/fonc.2023.1194673
26. Wenjing Y, Yanping L, Li Z, Qingxia H, Zhiyue W, Xing T. The symptom cluster of fatigue, anxiety, pain and sleeping disorder in lung cancer patients received chemotherapy and its influencing factors. Military Nursing. (2019) 36:44–7. doi: 10.3969/j.issn.1672-9676.2023.20.021
27. Yao. Y, Guan. X, Bao. G, Liang. J, Li. T, Zhong X. Whole-exome sequencing and bioinformatics analysis of a case of non-alpha-fetoprotein-elevated lung hepatoid adenocarcinoma. Front Pharmacol. (2022) 13:945038. doi: 10.3389/fphar.2022.945038
28. Jacobsen PB, Garland LL, Booth-Jones M, Donovan KA, Thors CL, Winters E, et al. Relationship of hemoglobin levels to fatigue and cognitive functioning among cancer patients receiving chemotherapy. J Pain Symptom Management. (2004) 28:7–18. doi: 10.1016/j.jpainsymman.2003.11.002
29. Chen W, Ji Y. Analysis of cancer-related fatigue levels and related influencing factors in patients with colorectal cancer after radiotherapy. J Rare Uncommon Diseases. (2023) 30:75–6. doi: 10.3969/j.issn.1009-3257.2023.11.033
30. Na R, Mengbai T, Yitong L, Yuxin S, Yu T. Current status and influencing factors of meaning in life among postoperative lung cancer patients. Chin J Modern Nursing. (2024) 30:806–11. doi: 10.3760/cma.j.cn115682-20230530-02143
31. Ting L, Fangfang B. The effect of family multimodal exercise therapy on cancer-related fatigue in middle-aged and young gynecological chemotherapy patients. Chongqing Med. (2024) 53:1910–4. doi: 10.3969/i.issn.1671-8348.2024.12.028
32. Lulu P, Yufeng R, Xiaoyan C, Qin B. Investigation and analysis of related factors of cancer-related fatigue in elderly patients with breast cancer undergoing chemo-therapy. Geriatrics Health Care. (2024) 30:621–5,32. doi: 10.3969/j.issn.1008-8296.2024.03.011
33. Guo. Q, Li. D, Luo. X, Yuan. Y, Li. T, Liu. H, et al. The regulatory network and potential role of LINC00973-miRNA-mRNA ceRNA in the progression of non-small-cell lung cancer. Front Immunol. (2021) 12:684807. doi: 10.3389/fimmu.2021.684807
34. Jianying Y, Yun D. The impact of hierarchical nursing guided by nursing outcome classification system on the hope level and cancer-related fatigue of lung cancer chemotherapy patients. Int J Nursing. (2024) 43:2314–7. doi: 10.3760/cma.j.cn221370-20230601-00542
35. Yi H, Yanqiong Z, Liujuan L. Research on the current status, influencing factors, and correlation with self transcendence of cancer-related fatigue in lung cancer patients. J Guangxi Univ Chin Med. (2023) 26:58–62. doi: 10.3969/j.issn.2095-4441.2023.03.017
36. Yin J, Wang Z, Zhang H. Genes of cancer-related fatigue: a scoping review. Front Oncol. (2024) 14:1446321. doi: 10.3389/fonc.2024.1446321
37. Zhang C. Prediction of risk factors for cancer-related fatigue and exploration of therapeutic mechanisms under traditional Chinese medicine intervention. Guangzhou University of Chinese Medicine (2021). doi: 10.27044/d.cnki.ggzzu.2021.000722
38. Esquerdo G, Llorca C, Cervera JM, Orts D, Juárez A, Carrato A. Effectiveness of darbepoetin alfa in a cohort of oncology patients with chemotherapy-induced anaemia. Relationship between variation in three fatigue-specific quality of life questionnaire scores and change in haemoglobin level. Clin Transl Oncol. (2011) 13:341–7. doi: 10.1007/s12094-011-0664-3
39. Peirong X, Yan Z, Qiannan S, Daorong W. The mediating effect of cancer-induced fatigue on sleep and cognitive function after gastrointestinal tumor surgery. J Clin Med Practice. (2023) 27:73–7. doi: 10.7619/jcmp.20223117
40. Fabi A, Bhargava R, Fatigoni S, Guglielmo M, Horneber M, Roila F, et al. Cancer-related fatigue: ESMO Clinical Practice Guidelines for diagnosis and treatment. Ann Oncol. (2020) 31:713–23. doi: 10.1016/j.annonc.2020.02.016
Keywords: lung cancer, cancer-related fatigue, sleep quality, anxiety, depression, risk prediction model, logistic regression analysis
Citation: Zhang M-N, Zhou Y-C, Zeng Z, Zeng C-L, Hou B-T, Wu G-R, Jiao Q and Ma D-Y (2025) Actors influencing cancer-related fatigue and the construction of a risk prediction model in lung cancer patients. Front. Oncol. 14:1485317. doi: 10.3389/fonc.2024.1485317
Received: 23 August 2024; Accepted: 27 December 2024;
Published: 24 January 2025.
Edited by:
Mehmet Ali Bedirhan, Yedikule Teaching Hospital, TürkiyeReviewed by:
Feng Liu, Central South University, ChinaSemra Bulbuloglu, Istanbul Aydın University, Türkiye
Copyright © 2025 Zhang, Zhou, Zeng, Zeng, Hou, Wu, Jiao and Ma. This is an open-access article distributed under the terms of the Creative Commons Attribution License (CC BY). The use, distribution or reproduction in other forums is permitted, provided the original author(s) and the copyright owner(s) are credited and that the original publication in this journal is cited, in accordance with accepted academic practice. No use, distribution or reproduction is permitted which does not comply with these terms.
*Correspondence: Dai-Yuan Ma, bWR5bHhAMTYzLmNvbQ==
†These authors share first authorship