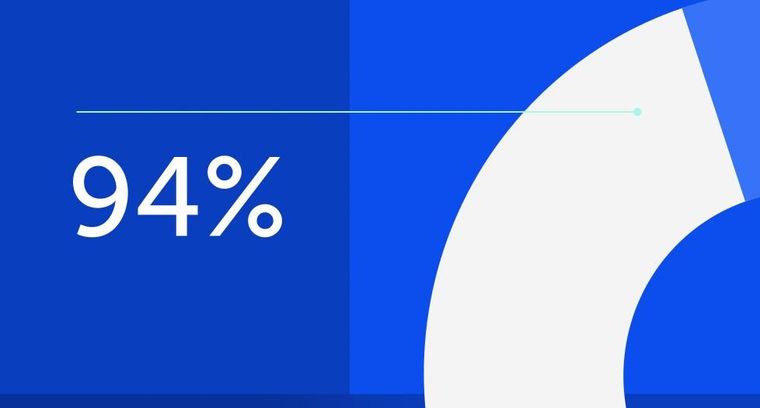
94% of researchers rate our articles as excellent or good
Learn more about the work of our research integrity team to safeguard the quality of each article we publish.
Find out more
ORIGINAL RESEARCH article
Front. Oncol., 21 October 2024
Sec. Thoracic Oncology
Volume 14 - 2024 | https://doi.org/10.3389/fonc.2024.1470827
Purposes: Osimertinib, one of the third-generation EGFR-tyrosine kinase inhibitors (TKIs) designed to target EGFR T790M mutation, significantly improves the prognosis of lung cancer. However, drug resistance still happens and MET amplification is responsible for one of the main causes. Fluorescence in situ hybridization (FISH) is the gold standard for MET amplification detection, but fundamentally limited by observer subjectivity. Herein, we assessed the value of next-generation sequencing (NGS) method in MET amplification detection in non-small cell lung cancer (NSCLC), as well as revealed the mutation profiling of NSCLC patients with osimertinib resistance to provide some valuable clues to the mechanisms of resistance.
Methods: A total of 317 cancer tissue samples from 317 NSCLC patients at time of progression following osimertinib were submitted to NGS and only 96 tissues were tested by FISH simultaneously. With FISH results as gold standard, enumeration algorithm was applied to establish the optimal model for identifying MET amplification using gene copy number (GCN) data.
Results: The optimal model for identifying MET amplification was constructed based on the GCN of MET, BRAF, CDK6 and CYP3A4, which achieved a 74.0% overall agreement with FISH and performed well in identifying MET amplification except polysomy with a sensitivity of 85.7% and a specificity of 93.9%. The inconsistency between NGS and FISH occurred mainly in polysomy subtype, while MET GCN ≥ 5 could be reliably recognized by NGS. Moreover, the most frequently mutated genes in NSCLC patients with osimertinib resistance were EGFR (59.94%), followed by TP53 (43.85%), NRG1 (9.46%), PIK3CA (6.31%), and ATM (5.36%). The known resistance mechanisms, including MET amplification, EGFR (C797S, L718Q/R), TP53, CDK4, CDK6, CDKN2A, BRAF, KRAS, NRAS and PIK3CA mutations were also disclosed in our cohort.
Conclusions: NGS assay can achieve a high concordance with FISH in MET amplification detection and has advantages in portraying various genetic alterations, which is of worthy in clinical promotion.
Lung cancer is the leading cause of cancer-related mortality worldwide. Non–small cell lung carcinoma (NSCLC) constitutes approximately 85% of all the lung cancers and has a poor 5-year survival rate of ~20% (1, 2), despite great efforts made over the past decades. The development of epidermal growth factor receptor (EGFR)-tyrosine kinase inhibitors (TKIs) is an important milestone in the targeted therapy of NSCLC (3). Numerous clinical trials have demonstrated that both the first-generation EGFR-TKIs such as gefitinib and erlotinib, and the second-generation EGFR-TKIs represented by afatinib achieved superior efficacy in the treatment of the EGFR-mutant NSCLC patients (4, 5). However, most NSCLC patients develop drug resistance, with EGFR T790M mutation as the most common resistant mechanism (6). To overcome the T790M-mediated resistance, the third-generation EGFR-TKIs, osimertinib, targeting the T790M mutation emerged as the times require. However, patients also inevitably develop resistance, which limits the long-term efficacy of third-generation EGFR-TKIs in the clinic (7, 8). Of note, osimertinib has been recommended as the preferred first-line treatment option for EGFR-mutated NSCLC at present (9). Therefore, it is necessary to comprehensively explore the resistance mechanisms of osimertinib.
Mesenchymal-epithelial transition (MET) gene located on chromosome 7 (7q31) encodes the receptor tyrosine kinase or hepatocyte growth factor receptor (HGFR). HGFR, along with its ligand, HGF, functions as an important regulator of cell survival, proliferation, motility and migration (10, 11). Dysregulation of MET signaling, such as MET amplification, MET exon 14 skipping mutation, and MET overexpression has been found to be associated with the development of lung cancer (12). MET amplification referring to the MET gene copy number (GCN) gains occurs in 1-6% of newly diagnosed NSCLC patients and is considered as a poor prognostic marker (13, 14). Moreover, growing evidence has demonstrated that MET amplification is a key driver of acquired resistance to EGFR-TKIs in addition to EGFR mutation, especially for patients resistant to the third-generation of EGFR-TKIs (15). Detailly, the incidence of MET amplification in NSCLC patients resistant to third-generation EGFR-TKIs increased from 5-22% to 5-50% compared to the patients’ resistance to the first/second-generation EGFR-TKIs (16). Of note, some clinical studies have demonstrated that the combination therapies of EGFR-TKIs and MET inhibitors improved the outcomes of NSCLC patients with MET amplification (17, 18). Therefore, accurate detection of MET amplification is essential for NSCLC patients with MET amplification.
To date, various techniques, including fluorescence in situ hybridization (FISH), quantitative real-time polymerase chain reaction (qRT-PCR) and next-generation sequencing (NGS), have been developed for detecting MET GCN (19). Both polysomy (multiple copies of chromosome 7) and MET amplification (multiple copies of MET only) cause MET GCN gains. FISH is the gold standard for detecting MET amplification status, but fundamentally limited by reliance on the subjectivity of the observers (20). NGS is increasingly applied in clinical practice for the detection of MET GCN, which offers comprehensive profiling not only MET amplification, but also many other genetic alterations that cannot be detected by FISH but are of high clinical significance. To date, several studies have explored the consistency between NGS and FISH for MET amplification detection. Unfortunately, the concordance between the FISH and NGS was no more than 70% (21, 22). Also, no consensus on the definition of MET amplification is reached, with the cutoff values ranging from GCN 2.3-10 depending on different NGS platforms (16, 22). In addition, MET amplification is regarded as a truly oncogenic driver compared to polysomy (23, 24). Therefore, it is necessary to find out a standardized NGS method to effectively define MET amplification.
In our study, we mainly investigated whether NGS could serve as an alternate method to identify MET amplification status, especially MET amplification, and reported the mutation profiling of the largest cohort of Chinese NSCLC patients with osimertinib resistance to reveal the underlying mechanisms to resistance. To this end, we first constructed the optimal model to identify MET amplification based on the GCN of MET and other genes located on chromosome 7 in 96 NSCLC patients, with FISH results as the gold standard. Then, we described the genetic mutation profile of 317 patients with resistance to osimertinib, and explored the relationship between genetic mutation and MET amplification status. Finally, we disclosed the known mechanisms of resistance to osimertinib in our cohort. Figure 1 showed the experimental flowchart of this study.
A total of 317 NSCLC patients from Shanghai Chest Hospital between August 2021 to October 2022 were enrolled in this study. All cases received first- and/or second-generation EGFR-TKIs as the first-line treatment, followed by third-generation EGFR-TKIs (osimertinib) as the second-line treatment, and eventually showed drug resistance. The tissue samples from 317 NSCLC patients at the time of progression following osimertinib were collected for genetic abnormality analysis, of which all cases were analyzed by NGS and only 96 by FISH simultaneously. Therefore, 96 NSCLC cases could be directly compared using both NGS and FISH regarding the determination of the MET amplification status. Clinic data including the age, gender, stage, and histology type were collected from the medical records. Informed consents were obtained from all patients and this study was carried out in compliance with the Declaration of Helsinki and approved by the ethics committee of Shanghai Chest Hospital (No. KS(Y)22288).
FISH was performed on 4-μm-thick formalin-fixed, paraffin-embedded (FFPE) tissue sections using a MET/CEP7 (centromere of chromosome 7) dual-color FISH probe (Vysis, Abbott Laboratories, Illinois, USA) according to the manufacturer’s instructions. The mean copy number of MET and CEP7 was recorded in at least 50 non-overlapping tumor cell nuclei and then MET/CEP7 ratio was calculated. MET amplification was defined as a mean MET/CEP7 ratio ≥ 2, and polysomy was defined as a mean GCN ≥ 5 and MET/CEP7 ratio < 2 synchronously (25).
Genomic DNA (gDNA) was extracted from FFPE tissues using the QIAamp FFPE DNA Tissue Kit (Qiagen, Germantown, MD, USA). In general, 200 ng gDNA was used to create the sequencing library targeting 84 genes (listed in Supplementary Table 1) (Rightongene, Shanghai, China) using a custom hybrid-capture NGS panel. Briefly, the DNA was fragmented and the fragmented DNA was subjected to end-repairing, A-tailing, and ligation with indexed adapters. Then, the libraries were PCR-amplified and purified for target enrichment. The library concentration was recorded by Qubit 3.0 Flourometer (Thermo, Massachusetts, USA) and the length and purity of the library fragment were measured by Qsep100 automated nucleic acid protein analysis system (BIOptic, Jiangsu, China). 500 ng indexed DNA libraries were pooled to obtain 1.5 μg DNA. Pooled DNA samples were mixed with DNA blocker and dried in a concentrator. Hybridization Master Mix was added to each sample. The mixtures were incubated at 95 °C for 10 min, then combined with probes and incubated at 65 °C for 16 h. Target regions were captured in accordance with the manufacturer’s instructions. The concentration and fragment size distribution of the final library were determined using Qubit 3.0 fluorometer and Qsep100 automated nucleic acid protein analysis system, respectively. Targeted NGS was performed on the Novaseq platform (Illumina, California, USA). The NGS detection sensitivity was approximately 0.1%.
Original sequencing image data file was converted into the sequencing data through base recognition and stored as FASTQ file. The quality of the original sequencing data was evaluated by FastQC (version 1.11.4) software. Trimmatic (version 3.6) software was used to remove joint information, low-quality bases, or undetected bases. After sorting and eliminating repetitive sequences, BAM files were obtained. The data of BAM files including library average length, comparison rate, coverage, capture rate, sequencing depth, homogeneity was used to evaluate the quality of sample library. Based on the BAM results, the SNP and InDel sites were detected by GATK and Mutet2 (26, 27) and annotated by ANNOVAR (28). Germline variants were removed using the ExAC (29), and 1000 Genomes project (30) (>0.1% population frequency). In addition, mutation meeting the following criteria were filtered out: (1) variant allele frequency (VAF) of mutation was less than 1%; (2) mutations predicted as harmless mutation by SIFT (SIFT score > 0.05) (31), and Polyphen2 (Polyphen2 HDAV score ≤ 0.446) (32). The final retained variants may be deleterious, likely deleterious or unknown significance. For the gene copy number identification, we first established a baseline of relative read coverage for capture region using normal tissue samples and then compared clinical samples to this baseline. Variant calling and copy-number variation analysis were performed by the cnvkit (https://github.com/etal/cnvkit).
Enumeration algorithm was applied to construct the optimal model to identify MET amplification using GCN data in 96 patients with FISH results. Receiver operating characteristic (ROC) curves were used to identify cutoff values of CN (MET)/CN (EGFR, BRAF, CDK6, PMS2, ABCB1 and CYP3A4, which were all of other genes on the 84-gene panel that were on chromosome 7) distinguishing between MET amplification subtype and polysomy/negative subtype. Then, enumeration algorithm was used to find out the optimal combination of CN (MET)/CN (other genes on chromosome 7) with the highest consistency with FISH in identifying MET amplification. Finally, the ROC curve was used to determine the cutoff value of MET GCN distinguishing between polysomy subtype and negative subtype. The sensitivity and specificity for identifying MET amplification were calculated as the proportion of MET amplification cases identified by NGS among MET amplification cases identified by FISH, and the proportion of polysomy or negative cases identified by NGS among polysomy or negative cases identified by FISH, respectively. The sensitivity and specificity for identifying polysomy or negative cases were calculated in the same way.
Statistical analysis was performed using R package (version 4.1.2). Categorical variables were evaluated using the chi-squared test or Fisher’s exact test. All tests were two-tailed, p < 0.05 was considered to be significantly different. * represents p < 0.05 and ** represents p < 0.01. Graphs were made in Prism 8, v8.2.0 (GraphPad Software Inc.) and Adobe Illustrator 2021 (Adobe Inc.).
A total of 317 NSCLC patients were enrolled in this study, with a median age of 60 years (range from 32 to 84 years). 135 (42.6%) patients were male and most of patients (94.2%) were diagnosed at an advanced stage of IV. 306 (96.8%) cases were lung adenocarcinoma, 9 (2.8%) cases were squamous cell carcinoma, and 1 (0.3%) case was adenosquamous carcinoma. 96 patients were subjected to FISH testing for detecting MET amplification status, where 14 (14.6%) cases were MET amplification, 16 (16.7%) cases were polysomy and 66 (68.8%) cases were negative. A summary of the clinical characteristics of 317 NSCLC patients was presented in Table 1. There was no significant difference in clinical characteristics between patients with MET amplification and those without MET amplification (Table 2).
Table 2. Differences in clinical characteristics between patients with MET amplification and those without MET amplification.
The commonly accepted criteria for defining MET amplification status via FISH was showed in Figure 2A. With FISH results as a reference, we explored the appropriate model to define MET amplification status based on the GCN of MET and other genes (EGFR, BRAF, CDK6, PMS2, ABCB1 and CYP3A4) located on chromosome 7. The optimal model demonstrated that cases with MET/CYP3A4 ≥ 1.12, MET/CDK6 ≥ 1.20 and MET/BRAF ≥ 1.53 were predicted to be MET amplification. Among the other cases, the cases with MET GCN ≥ 2.8 were predicted to be polysomy; and cases with MET GCN < 2.8 were predicted to be negative (Figure 2B). The overall concordance between NGS and FISH was 74.0% (95% CI 64% - 82.4%), while the sensitivity/specificity for identifying MET amplification, polysomy and MET negative were 85.7%/93.9%, 37.5%/85% and 80.3%/73.3%, respectively (Figure 2C). The model was stronger in identifying MET amplification and negative with a sensitivity of 85.7% and 80.3%, respectively; and weaker in identifying polysomy with a sensitivity of 37.5%. When combining polysomy and negative into one group, the overall concordance between NGS and FISH reached 93.9% (Figure 2D), suggesting that polysomy might be the key factor contributing to the discrepancy between NGS and FISH in the detection of MET amplification status.
Figure 2. Comparison of NGS and FISH for detecting MET gene copy number status. (A) The criteria for defining MET amplification status via fluorescence in situ hybridization (FISH). (B) The optimal model for identifying MET amplification status via next-generation sequencing (NGS). (C) The concordance between NGS and FISH in identifying MET amplification, polysomy and negative. (D) The consistency between NGS and FISH in identifying MET amplification vs. other cases. (E) Distribution of MET gene copy number for concordant and discordant cases. Amp, amplification; GCN, gene copy number.
Next, we investigated whether the concordance between NGS and FISH might be mediated by MET GCN levels. The distribution of MET GCN in all cases ranged from 1.2 to 16.7. The MET GCN of 25 discordant cases between NGS and FISH was predominantly distributed between 2 and 5, and the NGS method showed a 100% concordance with FISH when MET GCN was ≥ 5 (Figure 2E). Overall, our optimal model performed well in identifying MET amplification, especially the high level amplification.
The targeted sequencing was performed in all the patients and the mutation landscape of NSCLC patients resistant to osimertinib was showed in Figure 3A. A total of 66 mutated genes and 488 mutation sites were detected in 82.33% (261 of 317) of the NSCLC patients, among which the most frequently mutated genes were EGFR (59.94%), followed by TP53 (43.85%), NRG1 (9.46%), PIK3CA (6.31%), ATM (5.36%), APC (4.42%), ARID1A (4.42%), BRAF (4.10%), NTRK1 (4.10%), POLE (4.10%) and KRAS (3.79%). Those mutations had multiple mutation forms (missense, nonsense, nonstop, frameshift/inframe and splicing mutations) and mutation types (C→T, C→A, C→G, T→C, T→A and T→G), where the most commonly mutation form and type were missense mutations (424, 62.%) and C→T transitions (260, 41.1%), respectively (Figure 3B). Among the EGFR mutant patients, 95.79% of the patients harbored the reported hotspots, including exon 19 deletion (48.42%), L858R (46.84%) and T790M (17.89%), respectively. Moreover, uncommon mutation sites such as A750P (2.63%), C797S (2.11%), E709K (1.05%) accounted for 20.59% of EGFR mutations (Figure 3C). The majority of patients (65.8%) possessed single EGFR mutation site, a decent number of patients (26.3%) had two EGFR mutation sites, and the minority of patients (7.9%) had more than two EGFR mutation sites (Figure 3D). These mutational analyses may provide some valuable clues to the mechanisms of osimertinib resistance.
Figure 3. Comprehensive mutation analysis of Chinese NSCLC patients with osimertinib resistance. (A) Gene mutational landscapes of NSCLC patients with osimertinib resistance. (B) Classifications of mutation forms and mutation types in NSCLC patients with osimertinib resistance. (C) Bar chart showing the mutation frequencies of EGFR mutation sites. (D) Pie chart showing the percentage of patients carrying different numbers of EGFR mutation sites.
Then, we displayed the mutation landscapes of NSCLC patients with MET amplification, polysomy and MET negative, respectively (Figure 4A), and explored the correlations between genetic mutations and MET amplification status. The results showed that frequencies of mutations such as EGFR L858R (50.0% vs. 68.8% vs. 25.8%, p = 0.004), ARID1A (7.1% vs. 25.0% vs. 4.5%, p = 0.033), ATM (21.4% vs. 0% vs. 3.0%, p = 0.045), NARS (0% vs. 12.5% vs. 0%, p = 0.046) were differed among MET amplification, polysomy and MET negative groups. After multiple comparisons, we found that NSCLC patients with polysomy had a significantly higher incidence of EGFR L858R (p = 0.002), ARID1A (p = 0.025) and NRAS (p = 0.036) and significantly lower incidence of EGFR del19 (p = 0.041) mutation compared with those with MET negative. Moreover, mutation in ATM (p = 0.035) was significantly more common in NSCLC patients with MET amplification compared with those with MET negative. In addition, no association was found between other mutations and MET amplification status (Figure 4B). These findings suggested that there may be a potential link between MET amplification status and gene mutations.
Figure 4. Correlations between genetic mutations and MET amplification status. (A) The gene mutational landscapes of NSCLC patients with MET amplification, polysomy and MET negative. (B) Genes with significantly different mutation frequencies in MET amplification, polysomy and MET negative groups. Amp, amplification. * represents p < 0.05 and ** represents p < 0.01.
Figure 5 summarized the known genetic alterations associated with osimertinib resistance identified in our study. It is well recognized that the osimertinib resistance mechanisms are broadly divided into EGFR-dependent (on-target) and EGFR-independent (off-target) mechanisms. In our cohort, the known on-target mutations in C797S (1.26%), L718Q/R (0.95%), G796S (0.32%), G724S (0.32%), G719X (0.32%) sites were identified in several patients (33). Regarding EGFR-independent mechanisms, the known off-target alterations, such as MET amplification (14 of 96, 14.58%, bypass signaling activation), TP53 mutation (43.85%) (34, 35), PIK3CA mutation (6.31%, PI3K/AKT/PTEN/mTOR pathway activation) (33), BRAF/KRAS/NRAS mutation (5.36%/3.79%/0.95%, RAS-RAF-MEK-MAPK pathway activation) (36), and CDK4/CDK6/CDKN2A mutation (0.63%/0.95%/2.21%, cell cycle gene) (37, 38), were observed in 52.05% of the current cohort.
Figure 5. Schematic representation of the known mechanisms of resistance to osimertinib identified in our cohort. Amp, amplification; Mut, mutation.
The use of reliable methods for the detection of MET amplification status in NSCLC is essential for identifying patients eligible for treatment with MET inhibitors. In the present study, the MET amplification status identified by the optimal model demonstrated excellent agreement with the FISH results, suggesting that NGS may be an alternative method for MET amplification detection. In addition, our study reported the comprehensive mutation profile of a largest cohort of Chinese NSCLC patients with osimertinib resistance, which may contribute to the discovery of potential resistance mechanisms and the development of new EGFR-TKIs.
In recent years, NGS has been widely applied in clinical practice for the detection of comprehensive gene profiles, including gene mutations, gene amplification, rearrangement, and fusion. However, it is still lacking robust evidence regarding the feasibility and appropriateness of NGS as an alternative method to FISH to identify MET amplification. To date, several studies have investigated the performance of NGS in MET amplification detection using FISH as the gold standard (16, 39). Unfortunately, the concordance between the FISH and NGS in identifying MET amplification status was low. For example, Lai et al. (21) reported the low correlation of MET amplification results obtained by NGS and FISH, where of the 18 NSCLC patients identified as FISH-positive (2 MET amplification and 16 polysomy), only 8 (44.4%) were deemed to have MET GCN gain according to NGS. Also, the TATTON study reported that among 47 FISH-positive patients, only 12 (27%) were diagnosed with MET amplification by NGS (40). In addition, the concordance rate among NGS and FISH was only 62.5% (25 of 40) in the study performed by Peng et al. (22). In our study, the optimal model in identifying MET amplification status achieved a relatively high concordance rate of 74.0% with FISH for detecting MET amplification status.
Notably, accumulated evidences have suggested that the MET amplification is the truly oncogenic driver of lung cancer. NSCLC patients with MET amplification presented a more robust response to MET inhibitors compared with those with polysomy in the published results of clinical trials (41, 42). Our study prioritized the identification of MET amplification. In terms of the identification of MET amplification, our NGS method achieved a sensitivity of 85.7% with FISH, providing a reliable measurement of this biomarker in the clinic. In contrast to previous studies, which only used single gene as the determinant to distinguish MET amplification from polysomy (16, 21), we utilized CYP3A4, CDK6, and BRAF genes to determine MET amplification, which allowed for its potential higher resolution in the discrimination of MET amplification status. Moreover, previous studies indicated that high-level amplified MET has been used as a biomarker to predict the benefit of MET inhibitors, emphasizing the importance of identifying high levels of MET amplification (43, 44). In our study, a 100% consistency rate between NGS and FISH was observed in samples harboring MET GCN ≥ 5, which indicated that high-level amplified MET samples can be reliably detected via NGS. This finding was consistent with the previous study performed by Schubart et al. (43), who reported that the NGS method showed the best concordance with FISH when MET GCN was > 10 (80%, 4 of 5). Interestingly, Xiang et al. (41) found that IHC (H-score ≥ 200) showed high overall consistency with FISH in identifying MET amplification. Hartmaier et al. (45) reported that 81.25% (13 of 16) cases of MET amplification by FISH were also IHC positive, and IHC expression tended to increase with increasing MET GCN (≥10) in FISH-positive tumors. These studies indicated the potential relationship between MET amplification by FISH and MET IHC. It would be of interest to look at the correlation of MET amplification by NGS with MET IHC in the future.
Over the past few decades, genetic alterations of cancer driver genes have been identified in NSCLC, and molecular testing and targeted therapies have become standard care for NSCLC patients (46). Osimertinib is currently the preferred first-line therapy in patients with NSCLC with common EGFR mutation and the standard second-line therapy in T790M-positive patients in progression to previous EGFR-TKIs (47, 48). Osimertinib is a highly effective treatment with a high response rate and long-lasting disease control. However, the resistance to the treatment inevitably develops among patients (38, 49). Therefore, a comprehensive understanding of genetic alterations in osimertinib resistant patients is crucial to characterize emerging molecular resistance mechanism and develop novel targeted treatment. Our study presented comprehensive mutation profile of a largest cohort of Chinese NSCLC patients with osimertinib resistance, which may provide some clinically valuable clues to understand the mechanism of osimertinib resistance. To date, a number of studies have revealed that resistance mechanisms to osimertinib are highly complex, including EGFR-dependent and EGFR-independent mechanisms (8, 48, 50). The EGFR-dependent mechanisms include EGFR mutations or amplifications, where the most common EGFR mutation is EGFR C797S, accounts for 0-29% of cases of resistance to osimertinib (48). In our study, the incidence of C797S mutations was 1.26%. Besides C797S mutations, several other known on-target mutations in L718Q/R (0.95%), G796S (0.32%), G724S (0.32%), G719X (0.32%) were also identified. Regarding EGFR-independent mechanisms, it has been reported that osimertinib resistance can be acquired by bypass pathway activation, downstream pathway activation, cell cycle gene mutation, and histologic transformation (33, 35, 36, 51). In our study, the off-target alterations were dominant, mainly in TP53 mutation and MET amplification. It is worth noting that besides the discovery of the known genetic alterations medicating osimertinib resistance, a number of on-target mutations such as EGFR A750P (1.58%), E709K (0.63%), L861Q (0.63%), R776 (0.63%), S752F (0.63%), S758I (0.63%), and V536M as well as off-target mutations such as NRG1 (9.46%), ATM (5.36%), APC (4.42%), ARID1A (4.42%), NTRK1 (4.10%), POLE (4.10%), were also identified in multiple patients in our cohort. This finding may provide important guidance for future oncology efforts, such as exploring the potential resistance mechanisms and developing new EGFR-TKIs or combined strategies for NSCLC patients.
Several limitations of this study should be stated. First, regarding the comparison of FISH and NGS results, this study involved a relatively small sample size and lacked a validation cohort, which may affect the generalizability of our NGS methods for identifying MET amplification status. Second, no treatment response and prognosis data were available in this study. Further research is needed to validate the clinical utility of our NGS methods in determining tumors with MET amplification. We intend to carry out a larger sample size study to further validate our finding and examine the clinical utility of MET amplification by our NGS methods.
Collectively, this study demonstrated the potential of NGS as an alternative method in identifying MET amplification. In addition to MET amplification, DNA-based NGS assay could provide other data, such as characterizing various genetic alterations, which may potentially serve as an effective tool for guiding therapeutic strategies.
The datasets used and/or analyzed during the current study are available from the corresponding author on reasonable request.
The study was carried out based on the Declaration of Helsinki and approved by the Ethics Committee of Shanghai Chest Hospital with No. KS(Y)22288.
XX: Conceptualization, Investigation, Writing – original draft. RX: Investigation, Writing – review & editing. JL: Investigation, Writing – review & editing. BX: Conceptualization, Writing – original draft. CW: Methodology, Software, Writing – review & editing. KZ: Software, Writing – original draft. HZ: Conceptualization, Writing – review & editing. XC: Conceptualization, Writing – review & editing.
The author(s) declare financial support was received for the research, authorship, and/or publication of this article. This study was supported by the grants from Jilin Province Science and Technology Department of young and middle-aged science and technology innovation and entrepreneurship outstanding talents (team) project (20240601041RC).
We thank Shanghai Rightongene Biotechnology Co., Ltd. (Shanghai, China) for performance and analysis of the sequencing data.
Author XX, RX, BX, CW, and KZ were employed by the company Shanghai Rightongene Biotechnology Co., Ltd.
The remaining authors declare that the research was conducted in the absence of any commercial or financial relationships that could be construed as a potential conflict of interest.
All claims expressed in this article are solely those of the authors and do not necessarily represent those of their affiliated organizations, or those of the publisher, the editors and the reviewers. Any product that may be evaluated in this article, or claim that may be made by its manufacturer, is not guaranteed or endorsed by the publisher.
The Supplementary Material for this article can be found online at: https://www.frontiersin.org/articles/10.3389/fonc.2024.1470827/full#supplementary-material
1. Sadeghirad H, Bahrami T, Layeghi SM, Yousefi H, Rezaei M, Hosseini-Fard SR, et al. Immunotherapeutic targets in non-small cell lung cancer. Immunology. (2023) 168:256–72. doi: 10.1111/imm.v168.2
2. Rad HS, Rad HS, Shiravand Y, Radfar P, Arpon D, Warkiani ME, et al. The Pandora's box of novel technologies that may revolutionize lung cancer. Lung Cancer. (2021) 159:34–41. doi: 10.1016/j.lungcan.2021.06.022
3. Wang Q, Yang S, Wang K, Sun SY. MET inhibitors for targeted therapy of EGFR TKI-resistant lung cancer. J Hematol Oncol. (2019) 12:63. doi: 10.1186/s13045-019-0759-9
4. Remon J, Steuer CE, Ramalingam SS, Felip E. Osimertinib and other third-generation EGFR TKI in EGFR-mutant NSCLC patients. Ann Oncol. (2018) 29:i20–7. doi: 10.1093/annonc/mdx704
5. Lee DH. Treatments for EGFR-mutant non-small cell lung cancer (NSCLC): The road to a success, paved with failures. Pharmacol Ther. (2017) 174:1–21. doi: 10.1016/j.pharmthera.2017.02.001
6. Wu SG, Shih JY. Management of acquired resistance to EGFR TKI-targeted therapy in advanced non-small cell lung cancer. Mol Cancer. (2018) 17:38. doi: 10.1186/s12943-018-0777-1
7. Russo A, Franchina T, Ricciardi GRR, Smiroldo V, Picciotto M, Zanghì M, et al. Third generation EGFR TKIs in EGFR-mutated NSCLC: Where are we now and where are we going. Crit Rev Oncol Hematol. (2017) 117:38–47. doi: 10.1016/j.critrevonc.2017.07.003
8. Leonetti A, Sharma S, Minari R, Perego P, Giovannetti E, Tiseo M, et al. Resistance mechanisms to osimertinib in EGFR-mutated non-small cell lung cancer. Br J Cancer. (2019) 121:725–37. doi: 10.1038/s41416-019-0573-8
9. Gomatou G, Syrigos N, Kotteas E. Osimertinib resistance: molecular mechanisms and emerging treatment options. Cancers (Basel). (2023) 15:841. doi: 10.3390/cancers15030841
10. Lutterbach B, Zeng Q, Davis LJ, Hatch H, Hang G, Kohl NE, et al. Lung cancer cell lines harboring MET gene amplification are dependent on Met for growth and survival. Cancer Res. (2007) 67:2081–8. doi: 10.1158/0008-5472.CAN-06-3495
11. Robinson KW, Sandler AB. The role of MET receptor tyrosine kinase in non-small cell lung cancer and clinical development of targeted anti-MET agents. Oncologist. (2013) 18:115–22. doi: 10.1634/theoncologist.2012-0262
12. Lee M, Jain P, Wang F, Ma PC, Borczuk A, Halmos B, et al. MEt alterations and their impact on the future of non-small cell lung cancer (NSCLC) targeted therapies. Expert Opin Ther Targets. (2021) 25:249–68. doi: 10.1080/14728222.2021.1925648
13. Yin W, Cheng J, Tang Z, Toruner G, Hu S, Guo M, et al. MET amplification (MET/CEP7 ratio ≥ 1.8) is an independent poor prognostic marker in patients with treatment-naive non-small-cell lung cancer. Clin Lung Cancer. (2021) 22:e512–8. doi: 10.1016/j.cllc.2020.11.002
14. Schildhaus HU, Schultheis AM, Rüschoff J, Binot E, Merkelbach-Bruse S, Fassunke J, et al. MET amplification status in therapy-naïve adeno- and squamous cell carcinomas of the lung. Clin Cancer Res. (2015) 21:907–15. doi: 10.1158/1078-0432.CCR-14-0450
15. Westover D, Zugazagoitia J, Cho BC, Lovly CM, Paz-Ares L. Mechanisms of acquired resistance to first- and second-generation EGFR tyrosine kinase inhibitors. Ann Oncol. (2018) 29:i10–9. doi: 10.1093/annonc/mdx703
16. Qin K, Hong L, Zhang J, Le X. MET amplification as a resistance driver to TKI therapies in lung cancer: clinical challenges and opportunities. Cancers (Basel). (2023) 15:612. doi: 10.3390/cancers15030612
17. Remon J, Hendriks LEL, Mountzios G, García-Campelo R, Saw SPL, Uprety D, et al. MEt alterations in NSCLC-current perspectives and future challenges. J Thorac Oncol. (2023) 18:419–35. doi: 10.1016/j.jtho.2022.10.015
18. Garon EB, Brodrick P. Targeted therapy approaches for MET abnormalities in non-small cell lung cancer. Drugs. (2021) 81:547–54. doi: 10.1007/s40265-021-01477-2
19. Duncan DJ, Vandenberghe ME, Scott MLJ, Barker C. Fast fluorescence in situ hybridisation for the enhanced detection of MET in non-small cell lung cancer. PLoS One. (2019) 14:e0223926. doi: 10.1371/journal.pone.0223926
20. Clavé S, Jackson JB, Salido M, Kames J, Gerding KMR, Verner EL, et al. Comprehensive NGS profiling to enable detection of ALK gene rearrangements and MET amplifications in non-small cell lung cancer. Front Oncol. (2023) 13:1225646. doi: 10.3389/fonc.2023.1225646
21. Lai GGY, Lim TH, Lim J, Liew PJR, Kwang XL, Nahar R, et al. Clonal MET amplification as a determinant of tyrosine kinase inhibitor resistance in epidermal growth factor receptor-mutant non-small-cell lung cancer. J Clin Oncol. (2019) 37:876–84. doi: 10.1200/JCO.18.00177
22. Peng LX, Jie GL, Li AN, Liu SY, Sun H, Zheng MM, et al. MET amplification identified by next-generation sequencing and its clinical relevance for MET inhibitors. Exp Hematol Oncol. (2021) 10:52. doi: 10.1186/s40164-021-00245-y
23. Sun B, Qiu T, Zeng X, Duan J, Bai H, Xu J, et al. Detection of MET polysomy by next-generation sequencing and its clinical relevance for MET inhibitors. Cancer Res Commun. (2023) 3:532–9. doi: 10.1158/2767-9764.CRC-22-0438
24. Kumaki Y, Olsen S, Suenaga M, Nakagawa T, Uetake H, Ikeda S, et al. Comprehensive genomic profiling of circulating cell-free DNA distinguishes focal MET amplification from aneuploidy in diverse advanced cancers. Curr Oncol. (2021) 28:3717–28. doi: 10.3390/curroncol28050317
25. Bai Q, Shi X, Zhou X, Liang Z, Lu S, Wu Y, et al. Chinese expert consensus on clinical practice of MET detection in non-small cell lung cancer. Zhonghua Bing Li Xue Za Zhi. (2022) 51:1094–103. doi: 10.1177/17588359231216096
26. Cibulskis K, Lawrence MS, Carter SL, Sivachenko A, Jaffe D, Sougnez C, et al. Sensitive detection of somatic point mutations in impure and heterogeneous cancer samples. Nat Biotechnol. (2013) 31:213–9. doi: 10.1038/nbt.2514
27. Boutros PC, Ewing AD, Ellrott K, Norman TC, Dang KK, Hu Y, et al. Global optimization of somatic variant identification in cancer genomes with a global community challenge. Nat Genet. (2014) 46:318–9. doi: 10.1038/ng.2932
28. Wang K, Li M, Hakonarson H. ANNOVAR: functional annotation of genetic variants from high-throughput sequencing data. Nucleic Acids Res. (2010) 38:e164. doi: 10.1093/nar/gkq603
29. Lek M, Karczewski KJ, Minikel EV, Samocha KE, Banks E, Fennell T, et al. Analysis of protein-coding genetic variation in 60,706 humans. Nature. (2016) 536:285–91. doi: 10.1038/nature19057
30. Abecasis GR, Auton A, Brooks LD, DePristo MA, Durbin RM, Handsaker RE, et al. An integrated map of genetic variation from 1,092 human genomes. Nature. (2012) 491:56–65. doi: 10.1038/nature11632
31. Kumar P, Henikoff S, Ng PC. Predicting the effects of coding non-synonymous variants on protein function using the SIFT algorithm. Nat Protoc. (2009) 4:1073–81. doi: 10.1038/nprot.2009.86
32. Adzhubei IA, Schmidt S, Peshkin L, Ramensky VE, Gerasimova A, Bork P, et al. A method and server for predicting damaging missense mutations. Nat Methods. (2010) 7:248–9. doi: 10.1038/nmeth0410-248
33. Zalaquett Z, Catherine Rita Hachem M, Kassis Y, Hachem S, Eid R, Raphael Kourie H, et al. Acquired resistance mechanisms to osimertinib: The constant battle. Cancer Treat Rev. (2023) 116:102557. doi: 10.1016/j.ctrv.2023.102557
34. Vokes NI, Chambers E, Nguyen T, Coolidge A, Lydon CA, Le X, et al. Concurrent TP53 mutations facilitate resistance evolution in EGFR-mutant lung adenocarcinoma. J Thorac Oncol. (2022) 17:779–92. doi: 10.1016/j.jtho.2022.02.011
35. Ibusuki R, Iwama E, Shimauchi A, Tsutsumi H, Yoneshima Y, Tanaka K, et al. TP53 gain-of-function mutations promote osimertinib resistance via TNF-α-NF-κB signaling in EGFR-mutated lung cancer. NPJ Precis Oncol. (2024) 8:60. doi: 10.1038/s41698-024-00557-2
36. He J, Huang Z, Han L, Gong Y, Xie C. Mechanisms and management of 3rd−generation EGFR−TKI resistance in advanced non−small cell lung cancer (Review). Int J Oncol. (2021) 59:90. doi: 10.3892/ijo.2021.5270
37. Volta F, La Monica S, Leonetti A, Gnetti L, Bonelli M, Cavazzoni A, et al. Intrinsic resistance to osimertinib in EGFR mutated NSCLC cell lines induced by alteration in cell-cycle regulators. Target Oncol. (2023) 18:953–64. doi: 10.1007/s11523-023-01005-0
38. Bertoli E, De Carlo E, Del Conte A, Stanzione B, Revelant A, Fassetta K, et al. Acquired resistance to osimertinib in EGFR-mutated non-small cell lung cancer: how do we overcome it? Int J Mol Sci. (2022) 23:6936. doi: 10.3390/ijms23136936
39. Heydt C, Becher AK, Wagener-Ryczek S, Ball M, Schultheis AM, Schallenberg S, et al. Comparison of in situ and extraction-based methods for the detection of MET amplifications in solid tumors. Comput Struct Biotechnol J. (2019) 17:1339–47. doi: 10.1016/j.csbj.2019.09.003
40. Hartmaier RJ, Markovets AA, Ahn MJ, Sequist LV, Han JY, Cho BC, et al. Osimertinib + Savolitinib to overcome acquired MET-mediated resistance in epidermal growth factor receptor-mutated, MET-amplified non-small cell lung cancer: TATTON. Cancer Discovery. (2023) 13:98–113. doi: 10.1158/2159-8290.CD-22-0586
41. Xiang C, Lv X, Chen K, Guo L, Zhao R, Teng H. Unraveling the significance of MET focal amplification in lung cancer: integrative NGS, FISH, and IHC investigation. Mod Pathol. (2024) 37:100451. doi: 10.1016/j.modpat.2024.100451
42. Solomon JP, Yang SR, Choudhury NJ, Ptashkin RN, Eslamdoost N, Falcon CJ, et al. Bioinformatically expanded next-generation sequencing analysis optimizes identification of therapeutically relevant MET copy number alterations in >50,000 tumors. Clin Cancer Res. (2022) 28:4649–59. doi: 10.1158/1078-0432.CCR-22-1321
43. Schubart C, Stöhr R, Tögel L, Fuchs F, Sirbu H, Seitz G, et al. MET amplification in non-small cell lung cancer (NSCLC)-A consecutive evaluation using next-generation sequencing (NGS) in a real-world setting. Cancers (Basel). (2021) 13:5023. doi: 10.3390/cancers13195023
44. Camidge DR, Otterson GA, Clark JW, Ignatius Ou SH, Weiss J, Ades S, et al. Crizotinib in patients with MET-amplified NSCLC. J Thorac Oncol. (2021) 16:1017–29. doi: 10.1016/j.jtho.2021.02.010
45. Hartmaier R, Han JY, Cho BC, Markovets A, Kurian N, Cantarini M, et al. Abstract CT127: Tumor response and MET-detection methods exploratory biomarker analysis of Part B of the Ph 1b TATTON study. Cancer Res. (2021) 81 (13_Supplement):CT127. doi: 10.1158/1538-7445.AM2021-CT127
46. Du Z, Zhang Y, Hesilaiti N, Xia Q, Cui H. Structure-guided strategies of targeted therapies for patients with EGFR-mutant non-small cell lung cancer. Biomolecules. (2023) 13:210. doi: 10.3390/biom13020210
47. Tang ZH, Lu JJ. Osimertinib resistance in non-small cell lung cancer: Mechanisms and therapeutic strategies. Cancer Lett. (2018) 420:242–6. doi: 10.1016/j.canlet.2018.02.004
48. Fu K, Xie F, Wang F, Fu L. Therapeutic strategies for EGFR-mutated non-small cell lung cancer patients with osimertinib resistance. J Hematol Oncol. (2022) 15:173. doi: 10.1186/s13045-022-01391-4
49. Yang Z, Yang N, Ou Q, Xiang Y, Jiang T, Wu X, et al. Investigating novel resistance mechanisms to third-generation EGFR tyrosine kinase inhibitor osimertinib in non-small cell lung cancer patients. Clin Cancer Res. (2018) 24:3097–107. doi: 10.1158/1078-0432.CCR-17-2310
50. Dong RF, Zhu ML, Liu MM, Xu YT, Yuan LL, Bian J, et al. EGFR mutation mediates resistance to EGFR tyrosine kinase inhibitors in NSCLC: From molecular mechanisms to clinical research. Pharmacol Res. (2021) 167:105583. doi: 10.1016/j.phrs.2021.105583
Keywords: next generation sequencing, non-small cell lung cancer, MET amplification, osimertinib resistance, fish
Citation: Xiao X, Xu R, Lu J, Xin B, Wang C, Zhu K, Zhang H and Chen X (2024) The potential role of next-generation sequencing in identifying MET amplification and disclosing resistance mechanisms in NSCLC patients with osimertinib resistance. Front. Oncol. 14:1470827. doi: 10.3389/fonc.2024.1470827
Received: 26 July 2024; Accepted: 07 October 2024;
Published: 21 October 2024.
Edited by:
Guodong Zhao, Zhejiang University of Technology, ChinaReviewed by:
Lara Kujtan, University of Missouri–Kansas City, United StatesCopyright © 2024 Xiao, Xu, Lu, Xin, Wang, Zhu, Zhang and Chen. This is an open-access article distributed under the terms of the Creative Commons Attribution License (CC BY). The use, distribution or reproduction in other forums is permitted, provided the original author(s) and the copyright owner(s) are credited and that the original publication in this journal is cited, in accordance with accepted academic practice. No use, distribution or reproduction is permitted which does not comply with these terms.
*Correspondence: Xinyu Chen, Y2hlbnhpbnl1Y3VzdEAxNjMuY29t; Hao Zhang, emhhbmdoYW9AY3VzdC5lZHUuY24=
Disclaimer: All claims expressed in this article are solely those of the authors and do not necessarily represent those of their affiliated organizations, or those of the publisher, the editors and the reviewers. Any product that may be evaluated in this article or claim that may be made by its manufacturer is not guaranteed or endorsed by the publisher.
Research integrity at Frontiers
Learn more about the work of our research integrity team to safeguard the quality of each article we publish.