- 1Department of Orthopedics, Orthopaedic Research Institute, West China Hospital, Sichuan University, Chengdu, China
- 2Department of Operating Room, West China Hospital, Sichuan University/Nursing Key Laboratory of Sichuan Province, Sichuan University, Chengdu, China
Osteosarcoma stands as the most prevalent bone tumor, characterized by a heightened tendency for local recurrence and distant metastasis, resulting in a bleak prognosis. Presently, there exists a shortage of novel markers to effectively determine the prognosis of osteosarcoma patients. Recent research indicates that hematological markers partially mirror an individual’s microenvironment, offering potential insights into predicting patient prognosis. However, prior studies predominantly focused on the prognostic significance of singular hematological indices, failing to comprehensively represent the tumor microenvironment of patients. In our investigation, we meticulously gathered data on 22 hematological and electrolyte markers, utilizing LASSO Cox regression analysis to devise an Electrolyte Prognostic Scoring System (EPSS). The EPSS encompasses various indicators, including immunity, inflammation, coagulation, and electrolyte levels. Our findings indicate that the EPSS stands as an independent prognostic factor for overall survival among osteosarcoma patients. It serves as a valuable addition to clinical characteristics, adept at discerning high-risk patients from those deemed clinically low-risk. Furthermore, EPSS-based nomograms demonstrate commendable predictive capabilities.
1 Introduction
Osteosarcoma, comprising 20% to 40% of all bone tumors (1–3), stands as the most prevalent bone tumor with a pronounced propensity for local recurrence and distant metastasis, resulting in a dismal prognosis. The introduction of chemotherapy treatment in the 1970s notably augmented the five-year survival rate for patients with nonmetastatic osteosarcoma (4). As comprehensive treatments advance, the 5-year overall survival (OS) rate has improved to 60%–70%. However, this rate decreases significantly to 20%–30% when metastasis occurs. At the time of presentation, around 15%–20% of affected patients already exhibit metastases, and those with metastatic disease face notably low short- and long-term survival rates (5–8). Furthermore, tumor recurrence and the development of chemoresistance are recognized as crucial prognostic factors (9, 10). These clinical characteristics play a pivotal role in identifying high-risk patients and guiding treatment decisions (11). Nonetheless, disease progression might vary among patients sharing similar clinical traits. Hence, it becomes imperative to consider additional factors to enable precise and tailored treatment approaches.
Recent studies have revealed that certain preoperative hematological markers, such as the neutrophil-to-lymphocyte ratio (NLR), platelet-to-lymphocyte ratio (PLR), LDH, HBDH, and lymphocyte-to-macrophage ratio (LMR), offer insights into an individual’s tumor microenvironment, and these markers have shown promise in predicting the prognosis of cancer patients (12–16). They are easily accessible, cost-effective, and serve as valuable prognostic indicators. Numerous recent studies have underscored their significance in predicting survival and treatment response in cancer, including osteosarcoma (17, 18). Moreover, recent literature suggests that the presence of hyponatremia, hypochloremia, hypocalcemia, and hyperuricemia correlates with poorer survival rates. Surprisingly, there remains a dearth of studies investigating the relationship between electrolyte levels and the prognosis of osteosarcoma (19).
The LASSO model is an estimation method that enables the reduction of the set of indicators. LASSO regression has the advantages of ridge regression and subset selection at the same time, which makes it superior to other methods in terms of prediction accuracy and model interpretability for high dimensional multicollinearity problems. These advantages make LASSO regression an important tool in cancer biomarker research and prognostic model construction. In our study, we gathered established prognostic hematologic and electrolyte markers, utilizing iterative least absolute contraction and selection operator (LASSO) COX proportional hazards regression analysis to devise the Electrolyte Prognostic Scoring System (EPSS). Our findings indicate that EPSS addresses drawbacks inherent in single hematological markers, such as inadequate predictive power and instability, making it a valuable complement to clinical features.
2 Methods
2.1 Patients
The flow chart through this study is presented in Figure 1. With the approval of the Medical Ethics Committee, we reviewed the clinical data of osteosarcoma patients from January 2012 to January 2022 in the database of the Musculoskeletal Tumor Center of West China Hospital. During the review process, we included and excluded patients according to the following criteria: 1) patients with high grade osteosarcoma confirmed by histopathology; 2) patients have complete hematological test results before neoadjuvant chemotherapy; 3) patient received standard treatment at West China Hospital. The exclusion criteria:1) Patients with histopathologically confirmed low-grade osteosarcoma (intramedullary and bone surface) and periosteal osteosarcoma; 2) Patients who had received neoadjuvant chemotherapy before their first-time consultancy in our hospital; 3) patients with hematological diseases; 4) patients with other malignancies; 5) patients not received standard treatment (patients who are misdiagnosed and mistreated or fail to complete postoperative chemotherapy). Finally, 150 patients were included in our study after passing the inclusion and exclusion criteria. Each patient was followed up regularly until death or January 2022. The following follow-up principles were followed: reexamination every 3 months within 1 year after surgery; reexamination every 4 months 1-2 years after surgery; reexamination every 5 months 2-3 years after surgery; reexamination every 6 months 3-5 years after surgery; reexamination every year more than 5 years after surgery. All patients were randomly divided into a training set (n=105, 70%) and external validation set (n=45, 30%) using a random seed set in 2022.
2.2 Date collection and processing
We gathered various hematological and biochemical parameters from the initial blood routine, coagulation function tests, and liver and kidney function assessments of 150 patients before neoadjuvant chemotherapy. These included Hemoglobin (HB), Platelet count (Plt), Leukocyte count (Leut), Neutrophilic granulocyte percentage (Neup), Lymphocyte percentage (LYMp), Neutrophil count (Neut), Lymphocyte count (LYMPH), Monocyte count (MONO), Alanine aminotransferase (ALT), Aspartate aminotransferase (AST), Albumin (Protein), Alkaline phosphatase (ALP), Glutamyl transpeptidase (Glu), Creatine kinase (CK), Lactate dehydrogenase (LDH), Hydroxybutyrate dehydrogenase (HBDH), Natrium ion (Na), Anion gap (AG), Serum beta-hydroxybutyric acid (serum β), and Phosphorus (P).
Formulas were employed to compute several ratios including Neutrophil-to-Lymphocyte Ratio (NLR), Platelet-to-Lymphocyte Ratio (PLR), Lymphocyte-to-Monocyte Ratio (LMR), and derived Neutrophil-to-Leukocyte Ratio (dNLR), determined as follows: NLR = Neut/LYMPH, PLR = PLT/LYMPH, LMR = LYMPH/MONO, and dNLR = Neut/(Leut-Neut). Additionally, patient demographics such as age, gender, tumor location, and pathological fracture status were abstracted from medical records.
Overall survival (OS) was calculated from the date of tumor resection until the last follow-up or date of death. Optimal cutoff values for each hematological marker were determined using time-dependent receiver operating curve (tdROC) analysis, which were then converted into binary variables based on these cutoff values in the overall patient cohort.
2.3 Establishment and validation of EPSS (electrolyte prognostic scoring system)
We initiated our analysis by employing univariate Cox regression analysis to identify potential prognostic indicators within the overall patient cohort. Subsequently, leveraging the identified prognostic hematological markers, we conducted LASSO Cox regression analysis on the training set to ascertain the optimal Hematological Prognostic Scoring System (EPSS). EPSS scores were computed for each patient in both the training and validation sets based on coefficients derived from the LASSO Cox regression analysis.
To assess the predictive performance of EPSS compared to individual hematological markers, receiver operating curves (ROCs) were utilized in both the training and validation sets. Within the training set, the optimal cutoff value for EPSS was determined using the survival-ROC package, subsequently stratifying patients into high-risk and low-risk groups based on this cutoff value, which was then applied to the validation set. Kaplan-Meier survival curves were generated to visualize the disparity in Overall Survival (OS) between the two groups of patients.
The independent prognostic capacity of EPSS in predicting OS among osteosarcoma patients was evaluated using multivariate Cox regression analysis. Additionally, ROC curves were plotted for EPSS and clinical variables, spanning from 1 to 5 years, in both the training and validation sets using the time-ROC package, allowing for comparison.
2.4 Construction and evaluation of the nomogram
A nomogram was developed by combining EPSS with clinical features within the training set. Harrell’s Concordance Index was utilized to evaluate the nomograms’ discrimination ability, while calibration curves were employed to assess accuracy. Decision curve analysis (DCA) was conducted to appraise the clinical usefulness of the nomogram. Additionally, the established nomogram was applied to forecast overall survival in the validation cohort, affirming the stability and predictive capacity of the model.
2.5 Statistical analysis
The Kolmogorov–Smirnov test was used to assess whether continuous variables were normally distributed, and the Mann-Whitney U test or Spearman correlation analysis was used to assess differences between continuous variables according to the results. Categorical variables were evaluated using the chi-square test and Fisher’s exact test based on the number of individuals in each group. All statistical analyses were conducted using R software, version 4.3.1 (Institute for Statistics and Mathematics, Vienna, Austria). P-values < 0.05 were considered to indicate statistical significance.
3 Results
3.1 Patient characteristics
The study encompassed a cohort of 150 osteosarcoma patients, comprising 95 males and 55 females. Patients’ ages ranged from 8 to 72 years, with a mean age of 24.9 years. The majority of patients had tumors located in the extremities, while only 12 patients presented with tumors in non-extremity sites. Six patients had pathological fractures upon presentation. The 150 patients were randomly divided into a training cohort and a validation cohort. Table 1 displays the demographic and clinical characteristics of both the training and validation cohorts. No significant differences were observed between the two groups in terms of these characteristics. Supplementary Table 1 includes the optimal cutoff values for 22 hematological and electrolyte markers (HB, Plt, Leut, Neup, LYMp, Neut, LYMPH, MONO, NLR, PLR, LMR, dNLR, Protein, ALP, Glu, CK, LDH, HBDH, Na, AG, serumβ, P). Notably, the distributions of all variables in both the training and validation sets showed no significant differences, as demonstrated in Table 1.
3.2 Establishment and validation of electrolyte risk model for osteosarcoma
We initiated our study with univariate Cox regression analysis, evaluating the association between hematologic and electrolyte markers with OS in osteosarcoma patients within the overall cohort. Figure 2A illustrates that nine hematological markers demonstrated statistical significance in the univariate Cox regression analysis. Subsequently, employing LASSO Cox regression analysis in the training set using these nine hematological indicators, we identified an EPSS composed of eight significant hematological indicators (Table 1). The risk formula can be expressed as: Riskscore=HB*(-0.56) +Plt*0.55+Neup*0.27+dNLR*0.36+ALP*0.19+Na*(-0.70) +AG*0.67+P*0.57.
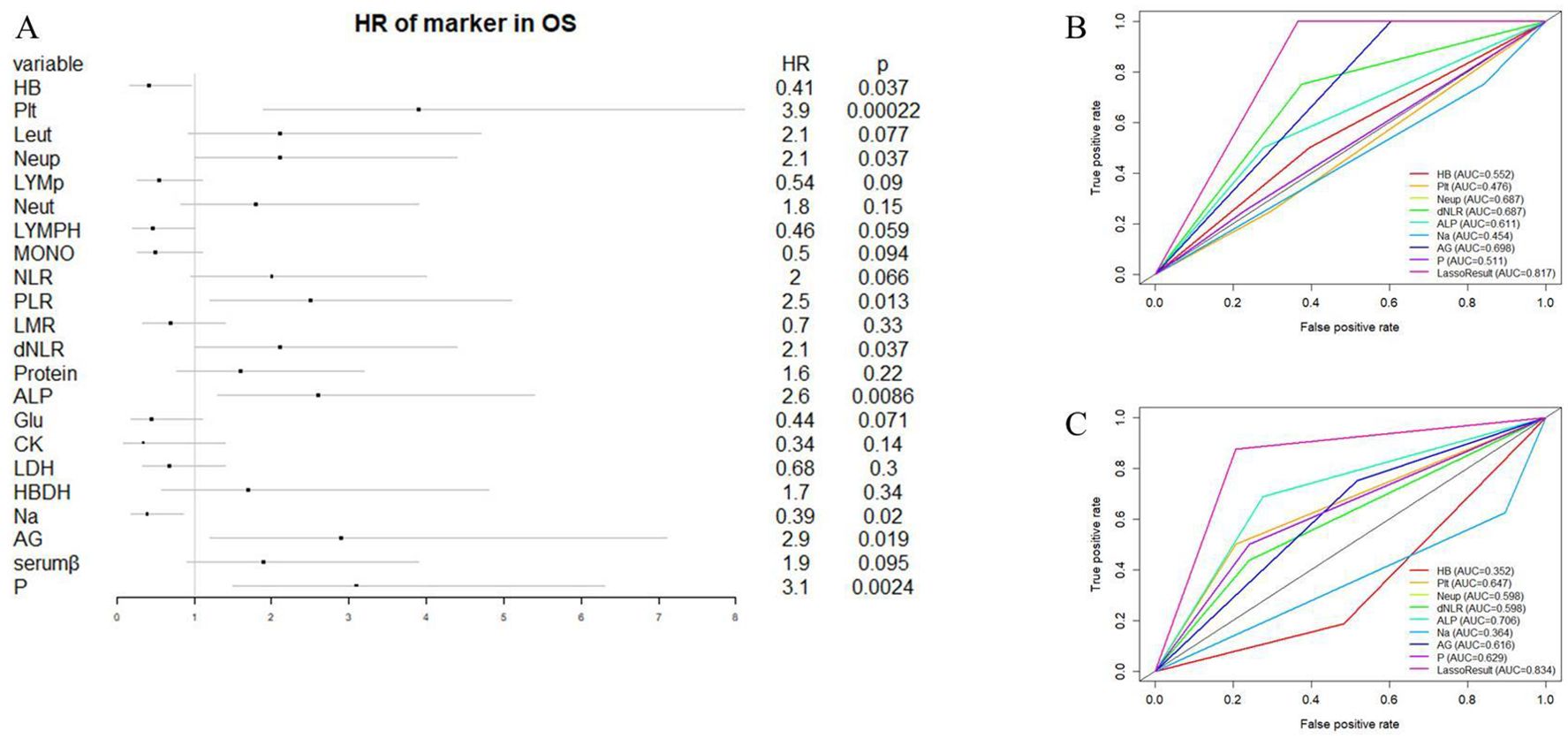
Figure 2. Construction of EPSS and its comparison with individual hematological and electrolyte parameters. (A) Forest plot showing the results of univariate cox regression analysis of 22 hematological and electrolyte markers; (B) ROC curves showing the predictive power of EPSS in the training set versus a single hematology or electrolyte indicator; (C) ROC curves showing the predictive power of EPSS in the validation set versus a single hematology or electrolyte indicator.
The coefficients assigned to each indicator in the EPSS are detailed in Table 1, facilitating the calculation of EPSS for each patient based on these coefficients. Our ROC curve analysis demonstrated a notably enhanced predictive ability of EPSS compared to individual hematological markers in both the training and validation cohorts (0.817 vs 0.454-0.698; 0.834 vs 0.352-0.706, Figures 2B, C).
We also determined optimal cutoff values for EPSS, stratifying the training cohort and validating it in the validation cohort. As depicted in Figures 3A, B, patients in the high EPSS risk group exhibited significantly lower overall survival rates in both cohorts (P < 0.001).
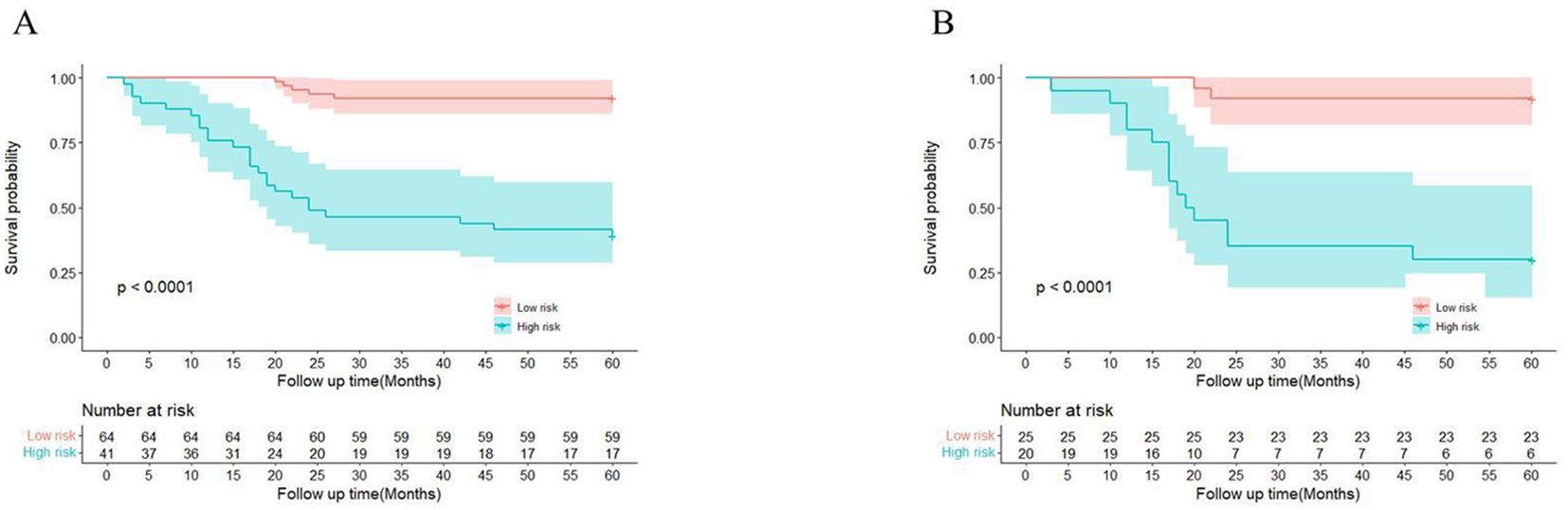
Figure 3. There are significant differences between patients in EPSS risk groups. (A) High-risk patients in the training set had significantly lower overall survival than low-risk patients; (B) High-risk patients in the validation set had significantly lower overall survival than low-risk patients.
Further analysis through multivariate Cox regression confirmed EPSS as an independent prognostic factor for overall survival in osteosarcoma patients within both the training and validation cohorts (training cohort: HR: 11.47(4.11-31.98); validation cohort: HR: 13(2.7-59), Figure 4A–D).
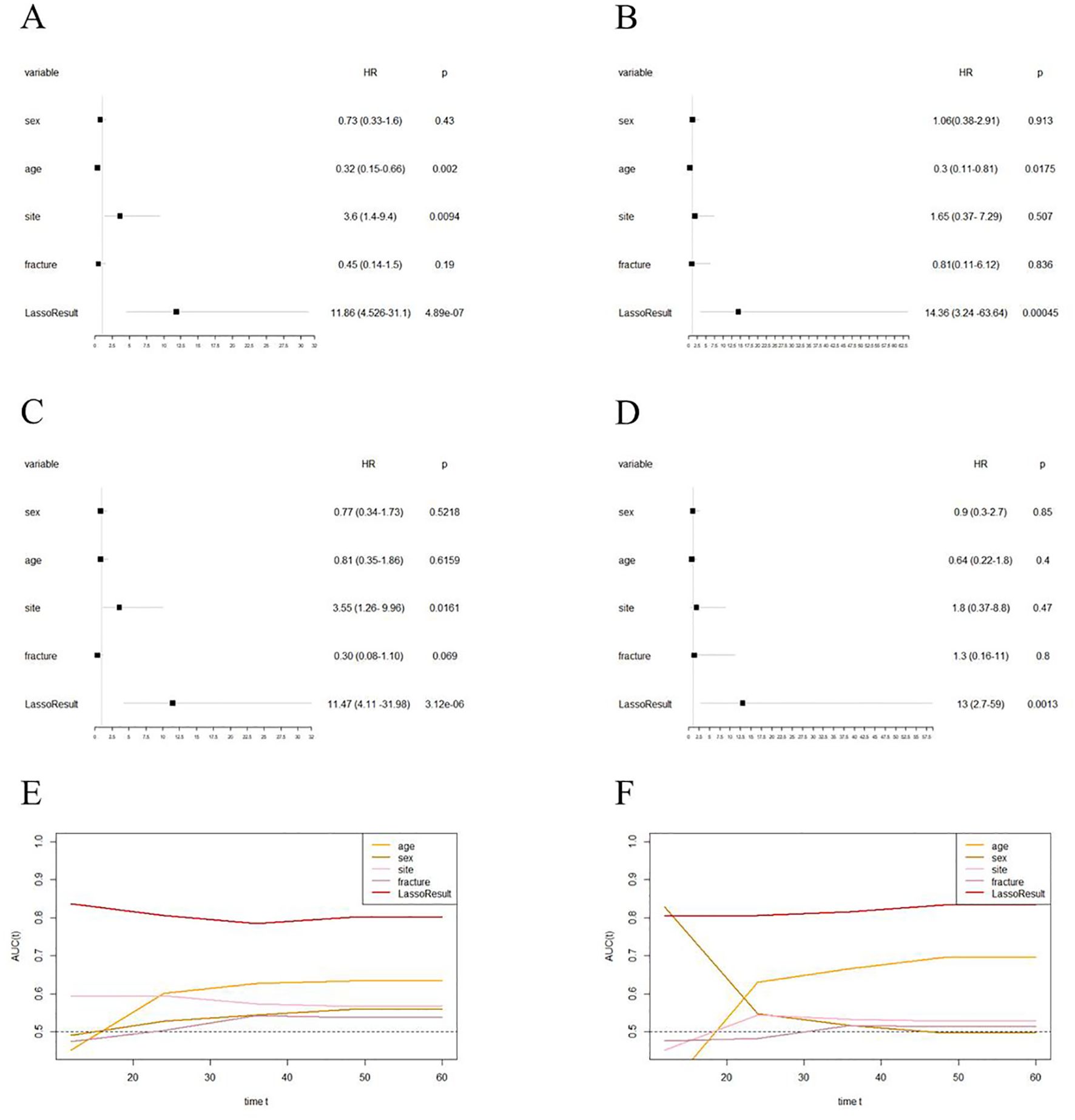
Figure 4. EPSS is an independent prognostic factor for overall survival in patients with osteosarcoma and has certain advantages compared with clinical characteristics. (A) Forest plot showing the results of univariate COX regression analysis of EPSS and clinical characteristics in the training set; (B) Forest plot showing the results of univariate COX regression analysis of EPSS and clinical characteristics in the validation set; (C) Forest plot showing the results of multivariate COX regression analysis of EPSS and clinical characteristics in the training set; (D) Forest plot showing the results of multivariate COX regression analysis of EPSS and clinical characteristics in the validation set; (F) Time-dependent ROC curves showing the predictive power of EPSS and clinical features in the training set; (F) Time-dependent ROC curves showing the predictive power of EPSS and clinical features in the training set; It can be seen that the predictive power of each variable varies over time.
Lastly, we plotted time-dependent ROC curves to compare the predictive ability of EPSS with clinical features such as tumor site, metastatic status, and pathological fractures. Figures 4E, F illustrate similar predictive abilities of EPSS over time in both the training and validation cohorts, indicating a gradual improvement in its predictive capacity.
3.3 Construction and validation of EPSS-based nomograms
To facilitate the clinical applicability of EPSS, we developed a nomogram by integrating EPSS with clinical characteristics based on the training cohort. Cox proportional hazards regression assigned a score corresponding to the hazard ratio for each covariate in the nomogram. The cumulative scores of these covariates constituted the nomogram’s total score. With a C-index of 0.78, the constructed nomogram exhibited good predictive accuracy for 36-month and 60-month overall survival in the training cohort, as depicted in Figures 5A and 5B through calibration curves.
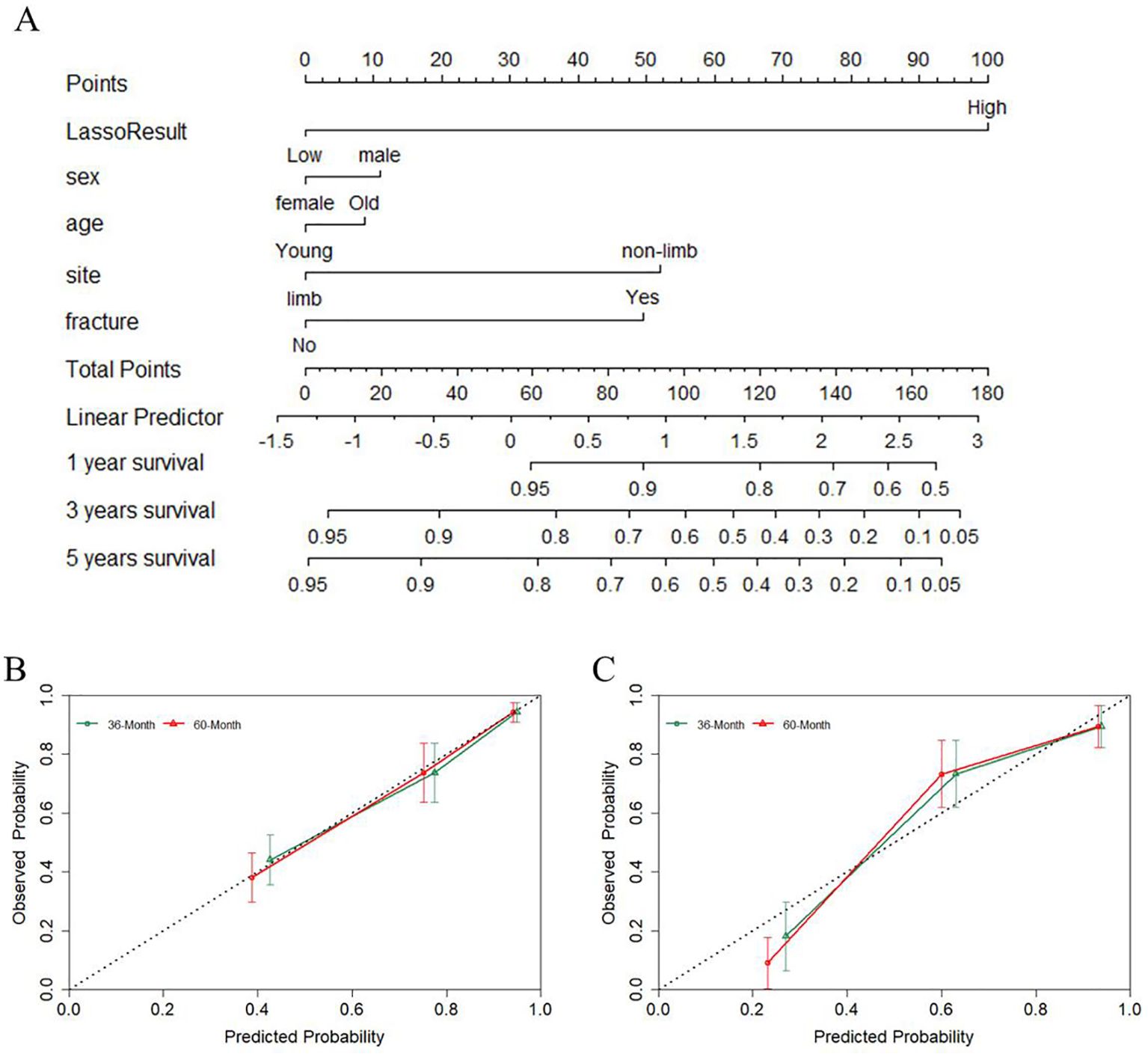
Figure 5. A nomogram was constructed combining EPSS with clinical features and the predictive power of the nomogram was assessed. (A) The nomogram of the overall survival of patients with osteosarcoma shows that EPSS score and site are the two most important variables; (B) Calibration curves for nomogram predicting 36-Month and 60-Month survival of patients in the training set; (C) Calibration curves for nomogram predicting 36-Month and 60-Month survival of patients in the validation set.
To validate the robustness of the nomogram, we assessed its performance in the validation cohort. With a C-index of 0.79, the nomogram displayed commendable predictive ability in the validation set, as indicated by the calibration curve in Figure 5C.
3.4 Association between EPSS and clinical features
Finally, we further assessed the relationship between EPSS and clinical characteristics. The results of the violin plot indicated that there was no significant difference in EPSS among patients with different gender, tumor site and pathological fracture (Figures 6A–C).
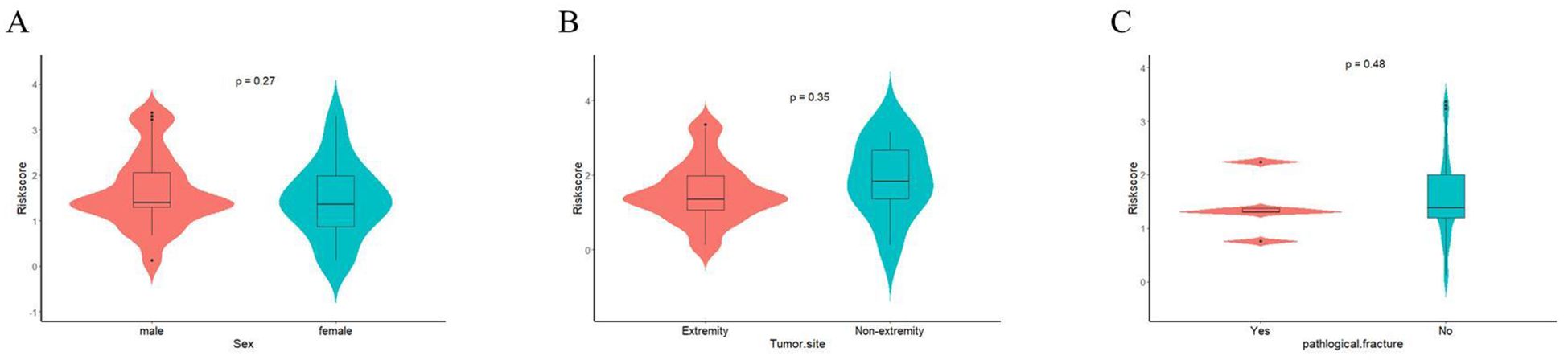
Figure 6. The relationship between EPSS and clinical characteristics were assessed. (A) The relationship between EPSS and gender; (B) The relationship between EPSS and Tumor-site; (C) The relationship between EPSS and pathological fracture.
4 Discussion
As surgical techniques advance and novel treatments emerge, the mortality rates among cancer patients have shown a declining trend. However, despite these advancements, the overall survival rates for osteosarcoma patients have plateaued since the 1970s (1, 11, 20). The evolution of precision medicine emphasizes the imperative need for tailored treatment strategies, offering the potential to enhance patient prognosis through individualized management (21, 22). Growing evidence underscores the pivotal role of genetic changes and epigenetic modifications in tumor onset and progression. Genetic testing, particularly assessing response to drug therapy, has gained traction in clinical applications. However, many of these tests rely on patient tissue and are cost-intensive. Encouragingly, recent research has unveiled that several preoperative hematological markers hold promise in predicting the prognosis of cancer patients (23–28). Contrary to genetic testing, hematological markers offer cost-effective and easily accessible prognostic insights. Remarkably, these markers originate from routine tests conducted upon patient admission. Prior studies often highlighted the prognostic value of singular hematological markers in cancer patients (29–31). However, due to the intricate nature of the tumor microenvironment, a single hematological marker struggles to comprehensively reflect tumor characteristics and accurately predict tumor progression. While previous studies indicated the prognostic value of Lymphocyte-to-Monocyte Ratio (LMR) in osteosarcoma patients, our cohort found LMR to exhibit limited predictive power (16, 32–34). Recent literature highlights associations between electrolyte imbalances (hyponatremia, hypochloremia, hypocalcemia, hyperuricemia) and poorer survival among tumor patients. Surprisingly, there’s a lack of exploration into the relationship between electrolytes and osteosarcoma prognosis.
Our study extensively gathered hematological markers with established prognostic value in osteosarcoma and uniquely combined electrolytes with these markers to construct the EPSS. Compared to singular hematological parameters, EPSS demonstrates superior predictive ability, potentially overcoming the instability of single hematological markers. EPSS demonstrated better predictive power (AUC>0.8) compared with previously published hematological prognostic models that did not include electrolytes (AUC<0.8) (28). Moreover, EPSS surpasses clinical characteristics in predicting long-term patient survival. The EPSS-based nomogram exhibits robust predictive capability, serving as a valuable complement to clinical features. When combined with clinical characteristics, EPSS facilitates further differentiation among patients categorized as clinically low risk.
Inflammation associated with tumors stands recognized as a significant hallmark of cancer. Numerous studies have elucidated how inflammatory processes foster cancer growth, activate oncogenic signaling pathways, and potentially contribute to immune resistance in cancer patients (35, 36). Immune cells, through intricate interactions with cancer cells, exert a pivotal role in shaping the tumor microenvironment (37).While evidence presents a paradoxical role for neutrophils in both impeding and advancing tumor progression, in solid tumors, their proliferation within the tumor microenvironment and systemically often correlates with a poorer prognosis (16, 38, 39). Conversely, lymphocytes in the tumor microenvironment are deemed crucial for antitumor immunity, orchestrating cytokine production and inducing tumor cell apoptosis (40). Platelets play a role in altering the tumor microenvironment by secreting vascular growth factors, promoting tumor cell growth, vascular proliferation, shielding tumor cells from immune cell eradication, and facilitating tumor cell metastasis (41, 42). Lactate dehydrogenase, a classical inflammatory marker in cancer, has undergone extensive study for its prognostic value (15, 43). Notably, within the EPSS framework, Platelet count (Plt) emerged as a cornerstone, exhibiting a coefficient of 0.552. This reaffirms the association between higher Plt levels and poorer patient prognosis, corroborating prior findings.
Serum ALP levels are often positively correlated with osteoblast activity, and serum ALP is common in fractures, physiological growth and bone tumors. Studies on the prognostic value of serum ALP in osteosarcoma date back even before the era of chemotherapy (44, 45). Now, it is generally believed that elevated serum ALP is associated with a worse prognosis in osteosarcoma patients (46). As an important part of constituting the EPSS, the coefficient of ALP was 0.194, indicating that elevated ALP is associated with poor patient prognosis. This is consistent with previous findings. We believe that the introduction of serum ALP makes EPSS more suitable for patients with bone tumors and enhances its predictive ability in patients with bone tumors.
EPSS exhibited superior predictive potency compared to individual hematological markers in both our training and validation cohorts. Our review of previous studies highlighted variations in the prognostic value of different hematological parameters across diverse cohorts, posing challenges in their clinical application. This variability likely stems from the incapacity of single hematological markers to comprehensively address the intricate tumor microenvironment. In response, our study extensively collected multiple hematological and electrolyte markers, culminating in the construction of EPSS to enhance predictive ability. Our aim was to mitigate the inherent instability of individual hematological markers. Notably, EPSS showcased higher predictive power than clinical features. Consequently, we assert EPSS’s effectiveness as a valuable complement to clinical features, particularly in distinguishing high-risk patients within the cohort initially categorized as low-risk based on clinical features.
We recommend utilizing hematological parameters obtained before chemotherapy to calculate EPSS for patients diagnosed with osteosarcoma. These pre-chemotherapy hematological parameters offer a more accurate reflection of the patient’s tumor microenvironment, as the results obtained after chemotherapy might be influenced by the treatment and may not faithfully represent the true tumor microenvironment.
We acknowledge several limitations in our study. Firstly, its retrospective nature may introduce selection bias. Secondly, the composition and calculation of EPSS, involving five hematological and three electrolyte parameters, pose complexity compared to single hematological markers. Notably, the relationship between electrolytes and osteosarcoma prognosis, explored for the first time in this study, lacks previous literature support. Further research is essential to validate our findings. Additionally, exploring whether EPSS can guide osteosarcoma treatment warrants attention. For instance, assessing whether patients with high EPSS, not developing lung metastases, benefit from increased frequency of lung CT follow-ups or if those with high EPSS benefit from increased chemotherapy cycles requires further investigation.
5 Conclusions
Our study confirms the prognostic value of the comprehensive hematological score EPSS in patients with osteosarcoma. EPSS is an independent prognostic factor in patients with osteosarcoma. The nomogram constructed based on EPSS has good predictive ability. The EPSS is a valid addition to clinical characteristics and is suitable for further identification of high risk patients from low clinical risk patients.
Data availability statement
The data analyzed in this study is subject to the following licenses/restrictions: nothing. Requests to access these datasets should be directed to xnliuyedao@163,com.
Ethics statement
The studies involving humans were approved by Ethics Committee of West China Hospital, Sichuan University. The studies were conducted in accordance with the local legislation and institutional requirements. The human samples used in this study were acquired from primarily isolated as part of your previous study for which ethical approval was obtained. Written informed consent for participation was not required from the participants or the participants’ legal guardians/next of kin in accordance with the national legislation and institutional requirements.
Author contributions
HL: Formal analysis, Supervision, Writing – original draft, Writing – review & editing. HK: Data curation, Formal analysis, Investigation, Writing – review & editing. LL: Data curation, Investigation, Writing – review & editing. ZL: Data curation, Investigation, Writing – review & editing. XH: Investigation, Methodology, Software, Writing – review & editing. YZ: Data curation, Investigation, Writing – review & editing. LM: Funding acquisition, Methodology, Resources, Supervision, Writing – review & editing. CT: Funding acquisition, Investigation, Methodology, Formal analysis, Resources, Writing – review & editing. ML: Data curation, Investigation, Writing – review & editing.
Funding
The author(s) declare financial support was received for the research, authorship, and/or publication of this article. This study is funded by Qingdao research institutes of Sichuan University, Research of biomedical materials and 3D printing related products (20GZ30301).
Acknowledgments
Thanks to the support of West China Hospital, Sichuan University for the research.
Conflict of interest
The authors declare that the research was conducted in the absence of any commercial or financial relationships that could be construed as a potential conflict of interest.
Publisher’s note
All claims expressed in this article are solely those of the authors and do not necessarily represent those of their affiliated organizations, or those of the publisher, the editors and the reviewers. Any product that may be evaluated in this article, or claim that may be made by its manufacturer, is not guaranteed or endorsed by the publisher.
Supplementary material
The Supplementary Material for this article can be found online at: https://www.frontiersin.org/articles/10.3389/fonc.2024.1466912/full#supplementary-material
References
1. Isakoff MS, Bielack SS, Meltzer P, Gorlick R. Osteosarcoma: current treatment and a collaborative pathway to success. J Clin Oncol Off J Am Soc Clin Oncol. (2015) 33:3029–35. doi: 10.1200/JCO.2014.59.4895
2. Valery PC, Laversanne M, Bray F. Bone cancer incidence by morphological subtype: a global assessment. Cancer Causes Control CCC. (2015) 26:1127–39. doi: 10.1007/s10552-015-0607-3
3. Beck J, Ren L, Huang S, Berger E, Bardales K, Mannheimer J, et al. Canine and murine models of osteosarcoma. Vet Pathol. (2022) 59(3):399–414. doi: 10.1177/03009858221083038
4. Mirabello L, Troisi RJ, Savage SA. Osteosarcoma incidence and survival rates from 1973 to 2004: data from the Surveillance, Epidemiology, and End Results Program. Cancer. (2009) 115:1531–43. doi: 10.1002/cncr.24121
5. Kempf-Bielack B, Bielack SS, Jürgens H, Branscheid D, Berdel WE, Exner GU, et al. Osteosarcoma relapse after combined modality therapy: an analysis of unselected patients in the Cooperative Osteosarcoma Study Group (COSS). J Clin Oncol Off J Am Soc Clin Oncol. (2005) 23(3):559–68. doi: 10.1200/JCO.2005.04.063
6. Aljubran AH, Griffin A, Pintilie M, Blackstein M. Osteosarcoma in adolescents and adults: survival analysis with and without lung metastases. Ann Oncol Off J Eur Soc Med Oncol. (2009) 20:1136–41. doi: 10.1093/annonc/mdn731
7. Gorlick R, Janeway K, Lessnick S, Randall RL, Marina N, COG Bone Tumor Committee. Children’s Oncology Group’s 2013 blueprint for research: bone tumors. Pediatr Blood Cancer. (2013) 60:1009–15. doi: 10.1002/pbc.24429
8. Ferguson JL, Turner SP, Barracks S. Bone cancer: diagnosis and treatment principles. Bone Cancer. (2018) 98(4):205–13.
9. Saraf AJ, Fenger JM, Roberts RD. Osteosarcoma: accelerating progress makes for a hopeful future. Front Oncol. (2018) 8:4. doi: 10.3389/fonc.2018.00004
10. Meyers PA, Schwartz CL, Krailo M, Kleinerman ES, Betcher D, Bernstein ML, et al. Osteosarcoma: a randomized, prospective trial of the addition of ifosfamide and/or muramyl tripeptide to cisplatin, doxorubicin, and high-dose methotrexate. J Clin Oncol Off J Am Soc Clin Oncol. (2005) 23(9):2004–11. doi: 10.1200/JCO.2005.06.031
11. Strauss SJ, Whelan JS. Current questions in bone sarcomas. Curr Opin Oncol. (2018) 30:252–9. doi: 10.1097/CCO.0000000000000456
12. Li LQ, Bai ZH, Zhang LH, Zhang Y, Lu XC, Zhang Y, et al. Meta-analysis of hematological biomarkers as reliable indicators of soft tissue sarcoma prognosis. Front Oncol. (2020) 10:30. doi: 10.3389/fonc.2020.00030
13. Zhang L, Li L, Liu J, Wang J, Fan Y, Dong B, et al. Meta-analysis of multiple hematological biomarkers as prognostic predictors of survival in bladder cancer. Med (Baltimore). (2020) 99(30):e20920. doi: 10.1097/MD.0000000000020920
14. Xia WK, Liu ZL, Shen D, Lin QF, Su J, Mao WD. Prognostic performance of pre-treatment NLR and PLR in patients suffering from osteosarcoma. World J Surg Oncol. (2016) 14:127. doi: 10.1186/s12957-016-0889-2
15. Fu Y, Lan T, Cai H, Lu A, Yu W. Meta-analysis of serum lactate dehydrogenase and prognosis for osteosarcoma. Med (Baltimore). (2018) 97:e0741. doi: 10.1097/MD.0000000000010741
16. Peng LP, Li J, Li XF. Prognostic value of neutrophil/lymphocyte, platelet/lymphocyte, lymphocyte/monocyte ratios and Glasgow prognostic score in osteosarcoma: A meta-analysis. World J Clin Cases. (2022) 10:2194–205. doi: 10.12998/wjcc.v10.i7.2194
17. Suazo-Zepeda E, Bokern M, Vinke PC, Hiltermann TJN, de Bock GH, Sidorenkov G. Risk factors for adverse events induced by immune checkpoint inhibitors in patients with non-small-cell lung cancer: a systematic review and meta-analysis. Cancer Immunol Immunother CII. (2021) 70:3069–80. doi: 10.1007/s00262-021-02996-3
18. Li YJ, Yao K, Lu MX, Zhang WB, Xiao C, Tu CQ. Prognostic value of the C-reactive protein to albumin ratio: a novel inflammation-based prognostic indicator in osteosarcoma. OncoTargets Ther. (2017) 10:5255–61. doi: 10.2147/OTT.S140560
19. Grikyte I, Ignatavicius P. Postoperative hypophosphatemia as a prognostic factor for postoperative pancreatic fistula: A systematic review. Med Kaunas Lith. (2023) 59:274. doi: 10.3390/medicina59020274
20. Kager L, Tamamyan G, Bielack S. Novel insights and therapeutic interventions for pediatric osteosarcoma. Future Oncol Lond Engl. (2017) 13:357–68. doi: 10.2217/fon-2016-0261
21. Forrest SJ, Geoerger B, Janeway KA. Precision medicine in pediatric oncology. Curr Opin Pediatr. (2018) 30:17–24. doi: 10.1097/MOP.0000000000000570
22. Tirtei E, Campello A, Asaftei SD, Mareschi K, Cereda M, Fagioli F. Precision medicine in osteosarcoma: MATCH trial and beyond. Cells. (2021) 10:281. doi: 10.3390/cells10020281
23. Wang PF, Meng Z, Song HW, Yao K, Duan ZJ, Yu CJ, et al. Preoperative changes in hematological markers and predictors of glioma grade and survival. Front Pharmacol. (2018) 9:886. doi: 10.3389/fphar.2018.00886
24. Charrier M, Mezquita L, Lueza B, Dupraz L, Planchard D, Remon J L, Planchard D, Remon J, et al. Circulating innate immune markers and outcomes in treatment-naïve advanced non-small cell lung cancer patients. Eur J Cancer Oxf Engl. (2019) 108:88–96. doi: 10.1016/j.ejca.2018.12.017
25. Xu Z, Xu W, Cheng H, Shen W, Ying J, Cheng F, et al. The prognostic role of the platelet-lymphocytes ratio in gastric cancer: A meta-analysis. PloS One. (2016) 11(9):e0163719. doi: 10.1371/journal.pone.0163719
26. Li L, Wang Y, He X, Li Z, Lu M, Gong T, et al. Hematological prognostic scoring system can predict overall survival and can indicate response to immunotherapy in patients with osteosarcoma. Front Immunol. (2022) 13:879560. doi: 10.3389/fimmu.2022.879560
27. He X, Lu M, Hu X, Li L, Zou C, Luo Y, et al. Osteosarcoma immune prognostic index can indicate the nature of indeterminate pulmonary nodules and predict the metachronous metastasis in osteosarcoma patients. Front Oncol. (2022) 12:952228. doi: 10.3389/fonc.2022.952228
28. He X, Wang Y, Ye Q, Wang Y, Min L, Luo Y, et al. Lung immune prognostic index could predict metastasis in patients with osteosarcoma. Front Surg. (2022) 9:923427. doi: 10.3389/fsurg.2022.923427
29. Liang YJ, Mei XY, Zeng B, Zhang SE, Yang L, Lao XM, et al. Prognostic role of preoperative D-dimer, fibrinogen and platelet levels in patients with oral squamous cell carcinoma. BMC Cancer. (2021) 21(1):122. doi: 10.1186/s12885-021-07841-5
30. Wang S, Ma L, Wang Z, He H, Chen H, Duan Z, et al. Lactate dehydrogenase-A (LDH-A) preserves cancer stemness and recruitment of tumor-associated macrophages to promote breast cancer progression. Front Oncol. (2021) 11:654452. doi: 10.3389/fonc.2021.654452
31. Li X, Xu J, Zhu L, Yang S, Yu L, Lv W, et al. A novel nomogram with preferable capability in predicting the overall survival of patients after radical esophageal cancer resection based on accessible clinical indicators: A comparison with AJCC staging. Cancer Med. (2021) 10(13):4228–39. doi: 10.1002/cam4.3878
32. Hu H, Deng X, Song Q, Lv H, Chen W, Xing X, et al. Prognostic value of the preoperative lymphocyte-to-C-reactive protein ratio and albumin-to-globulin ratio in patients with osteosarcoma. OncoTargets Ther. (2020) 13:12673–81. doi: 10.2147/OTT.S287192
33. Liu B, Huang Y, Sun Y, Zhang J, Yao Y, Shen Z, et al. Prognostic value of inflammation-based scores in patients with osteosarcoma. Sci Rep. (2016) 6:39862. doi: 10.1038/srep39862
34. Pre-operative lymphocyte-to-monocyte ratio as a predictor of overall survival in patients suffering from osteosarcoma - PubMed . Available online at: https://pubmed.ncbi.nlm.nih.gov/26380812/ (Accessed January 8, 2024).
35. Grivennikov SI, Greten FR, Karin M. Immunity, inflammation, and cancer. Cell. (2010) 140:883–99. doi: 10.1016/j.cell.2010.01.025
36. Tsai HC, Cheng SP, Han CK, Huang YL, Wang SW, Lee JJ, et al. Resistin enhances angiogenesis in osteosarcoma via the MAPK signaling pathway. Aging. (2019) 11(21):9767–77. doi: 10.18632/aging.102423
37. Dunn GP, Old LJ, Schreiber RD. The immunobiology of cancer immunosurveillance and immunoediting. Immunity. (2004) 21:137–48. doi: 10.1016/j.immuni.2004.07.017
38. Araki Y, Yamamoto N, Hayashi K, Takeuchi A, Miwa S, Igarashi K, et al. Pretreatment neutrophil count and platelet-lymphocyte ratio as predictors of metastasis in patients with osteosarcoma. Anticancer Res. (2022) 42(2):1081–9. doi: 10.21873/anticanres.15570
39. Powell DR, Huttenlocher A. Neutrophils in the tumor microenvironment. Trends Immunol. (2016) 37:41–52. doi: 10.1016/j.it.2015.11.008
40. Galon J, Bruni D. Tumor immunology and tumor evolution: intertwined histories. Immunity. (2020) 52:55–81. doi: 10.1016/j.immuni.2019.12.018
41. Gay LJ, Felding-Habermann B. Contribution of platelets to tumour metastasis. Nat Rev Cancer. (2011) 11:123–34. doi: 10.1038/nrc3004
42. Shu Y, Peng J, Feng Z, Hu K, Li T, Zhu P, et al. Osteosarcoma subtypes based on platelet-related genes and tumor microenvironment characteristics. Front Oncol. (2022) 12:941724. doi: 10.3389/fonc.2022.941724
43. Petrelli F, Cabiddu M, Coinu A, Borgonovo K, Ghilardi M, Lonati V, et al. Prognostic role of lactate dehydrogenase in solid tumors: a systematic review and meta-analysis of 76 studies. Acta Oncol Stockh Swed. (2015) 54:961–70. doi: 10.3109/0284186X.2015.1043026
44. Bacci G, Longhi A, Fagioli F, Briccoli A, Versari M, Picci P. Adjuvant and neoadjuvant chemotherapy for osteosarcoma of the extremities: 27 year experience at Rizzoli Institute, Italy. Eur J Cancer Oxf Engl. (2005) 41:2836–45. doi: 10.1016/j.ejca.2005.08.026
45. Bramer JAM, Abudu AA, Tillman RM, Carter SR, Sumathi VP, Grimer RJ. Pre- and post-chemotherapy alkaline phosphatase levels as prognostic indicators in adults with localised osteosarcoma. Eur J Cancer Oxf Engl. (2005) 41:2846–52. doi: 10.1016/j.ejca.2005.07.024
Keywords: osteosarcoma, hematological markers, prognostic nomograms, electrolyte, OS
Citation: Liu H, Kang H, Li L, Li Z, He X, Zhang Y, Lu M, Min L and Tu C (2024) Electrolyte prognosis scoring system can predict overall survival in patients with osteosarcoma. Front. Oncol. 14:1466912. doi: 10.3389/fonc.2024.1466912
Received: 18 July 2024; Accepted: 19 September 2024;
Published: 09 October 2024.
Edited by:
Wenle Li, Xiamen University, ChinaReviewed by:
Xiaodong Tang, Peking University People’s Hospital, ChinaYulou Luo, Xinjiang Medical University, China
Copyright © 2024 Liu, Kang, Li, Li, He, Zhang, Lu, Min and Tu. This is an open-access article distributed under the terms of the Creative Commons Attribution License (CC BY). The use, distribution or reproduction in other forums is permitted, provided the original author(s) and the copyright owner(s) are credited and that the original publication in this journal is cited, in accordance with accepted academic practice. No use, distribution or reproduction is permitted which does not comply with these terms.
*Correspondence: Li Min, jacky-min@163.com; Chongqi Tu, tucq@scu.edu.cn
†These authors share first authorship