- 1College of Medicine, Howard University, Washington, DC, United States
- 2Department of Epidemiology, University of Alabama at Birmingham, Birmingham, AL, United States
- 3School of Nursing, Spoon River College, Canton, IL, United States
Introduction: Since the implementation of the Patient Protection and Affordable Care Act (ACA) and Medicaid expansion, states that adopted the policy have seen reduced uninsured rates. However, it is unclear whether increased healthcare access, particularly for minority and socioeconomically disadvantaged groups, has translated into measurable improvements in health outcomes.
Objective: Our study aims to evaluate the impact of the ACA and Medicaid expansion on breast cancer outcomes in Louisiana, which has implemented the policy, compared to Georgia, which has not, as of 2024.
Methodology: We conducted a retrospective study using SEER registry data from January 2011 to December 2021, including women aged 18-64 diagnosed with breast cancer. The impact of the ACA and Medicaid expansion on cancer-specific survival (CSS), overall survival (OS), and stage at presentation was evaluated. The cohort was divided into pre-ACA (2011-2015) and post-ACA (2017-2021) periods, with a one-year washout (2016). A difference-in-difference (DID) approach compared outcomes between Louisiana and Georgia.
Results: The study analyzed 62,381 women with breast cancer, with 32,220 cases in the pre-ACA period (51.7%) and 30,161 in the post-ACA period (48.3%). In Georgia, 43,279 women were included (52.3% pre-ACA vs. 47.7% post-ACA), while Louisiana had 19,102 women (50.1% pre-ACA vs. 49.9% post-ACA). Medicaid expansion in Louisiana was associated with a 0.26 percentage point reduction in overall deaths (95% CI: -10.9 to 10.4) and a 5.97 percentage point reduction in cancer-specific mortality (95% CI: -26.1 to 14.2). There was also no significant difference in disease stage at presentation compared to Georgia.
Conclusion: This study found no significant differences in overall mortality, cancer-specific mortality, or disease stage at presentation among women with breast cancer in Louisiana, which implemented Medicaid expansion in 2016, compared to Georgia, which has not expanded Medicaid.
Introduction
Breast cancer remains a significant public health concern in the United States, being one of the most commonly diagnosed cancers among women and a leading cause of cancer-related mortality (1, 2). Early detection and timely, effective treatment are critical factors influencing survival rates and overall patient outcomes (3). In recent years, healthcare policies aimed at increasing access to preventive care and medical services have become central to efforts to improve cancer outcomes (4, 5). The Patient Protection and Affordable Care Act (ACA), enacted in 2010, represents one of the most substantial healthcare reforms in U.S. history, with provisions intended to expand healthcare coverage, improve access to preventive services, and reduce healthcare disparities (6–8).
The ACA introduced several key measures, including the expansion of Medicaid, the establishment of health insurance marketplaces, and the mandate for coverage of preventive services such as mammograms without cost-sharing (9). These provisions aimed to reduce financial barriers to healthcare and encourage early detection of diseases, including breast cancer. Prior studies have suggested that these reforms could lead to improved cancer outcomes by facilitating earlier diagnosis and timely treatment (10–12). The significance of the ACA’s impact on breast cancer outcomes (CSS, OS and disease stage at presentation) cannot be overstated.
Georgia and New Jersey provide a unique opportunity to investigate the effects of the ACA. Louisiana implemented Medicaid expansion under the ACA, while Georgia did not. This study leverages this natural experiment to evaluate the ACA’s impact on breast cancer-specific survival and the stage at presentation. The objectives of this study are twofold: first, to determine whether the implementation of the ACA has led to improved breast outcomes in Louisiana compared to Georgia; and second, to assess whether there has been a shift in the stage at presentation of breast cancer diagnoses in these states. By examining these outcomes, this study aims to provide valuable insights into the effectiveness of healthcare policy reforms in improving cancer care and addressing healthcare disparities.
Methodology
Study design
This retrospective comparative cohort study utilized population-based cancer registry data from the SEER registry. The registry provides comprehensive data on cancer incidence, treatment, and outcomes, covering approximately 36.7% of the U.S. population (13–15).
Study population
The study population comprised women aged 18 to 64 years diagnosed with breast cancer between January 1, 2011, and December 31, 2021, in two distinct regions: Georgia, representing a non-Expansion State, and Louisiana, representing a Medicaid Expansion State. The selection of these states facilitated a comparative analysis of the impact of Medicaid expansion on breast cancer outcomes.
Study period and periods of interest
The study analyzed two time periods: pre-ACA (2011–2015) and post-ACA (2017–2021), with a washout period from January to December 2016 to allow for full implementation of the Medicaid expansion in Louisiana, which adopted the policy in 2016.
Georgia vs. Louisiana
We chose Georgia and Louisiana for comparison due to their differing ACA implementation statuses, with Louisiana implementing the ACA with its Medicaid expansion component and Georgia not (8, 16, 17). Despite economic differences, both states share similar key indices crucial for cancer screening, such as cancer rates, and breast cancer screening rates, despite significant differences in uninsured rates (18, 19). The American College of Radiology recommends a breast cancer risk assessment by 25 years for all women and annual mammography screening for women at average risk starting at 40 years (20, 21).
Louisiana implemented the ACA in 2016, under which Medicaid, referred to locally as Healthy Louisiana, was expanded (22, 23). This expansion allows adults aged 19-64 years with incomes up to 138% of the federal poverty level to qualify for Medicaid (23, 24). From 2015 to 2020, Medicaid expansion recorded 468,414 new enrollments, resulting in the uninsured rate dropping from 22.7% to 8.9% (24). Louisiana does not impose work requirements as a condition for Medicaid eligibility (16).
In contrast, Georgia did not adopt the ACA but instead secured a Medicaid waiver to offer health coverage to a subset of low-income adults (25). The Georgia Pathways to coverage program provides government health insurance to individuals earning up to the federal poverty level $15,060 per year for an individual adult conditional upon verification of employment, schooling, or other qualifying activities (26).
Primary outcome of interest
The primary outcomes of interest were cancer-specific survival (CSS), overall deaths (OS), and disease stage at presentation.
Cancer specific survival
The CSS served as a crucial indicator of long-term prognosis and treatment effectiveness among breast cancer patients (27). The CSS was calculated using Cox regression analysis and is defined as the mortality hazard used interchangeably for survival probability as the proportion of patients who survived per unit time (years of follow-up) after their breast cancer diagnosis. The Cox regression, also known as the proportional hazards model, is a statistical technique used to examine the association between the time until an event occurs (cancer-specific deaths) and various predictor variables (states stratified by expansion status (Georgia vs. Louisiana) and period (pre-ACA vs. post-ACA)).
Overall survival
Overall Survival (OS) served as a key measure of general prognosis among breast cancer patients. OS was calculated using Cox regression analysis and is defined as the mortality hazard, representing the probability of survival from any cause of death per unit of time (years of follow-up) after breast cancer diagnosis (28, 29). The Cox regression model, also known as the proportional hazards model, was used to assess the relationship between time until death (from any cause) and predictor variables, including state (stratified by expansion status: Georgia vs. Louisiana) and period (pre-ACA vs. post-ACA).
Disease stage at presentation
The disease stage at the time of breast cancer diagnosis was categorized into three groups based on the SEER registry’s combined summary stage (2004+). This variable allows for consistent stage analysis over time and was derived from the SEER Combined Summary Stage 2000 (2004–2017) and Derived Summary Stage 2018+ variables (30). The stage reflects how far the cancer has spread from its origin:
Localized: Cancer is confined to the primary site (30, 31).
Regional: Cancer has spread beyond the primary site to nearby lymph nodes or tissues (30, 31).
Distant: Cancer has spread to distant organs or remote lymph nodes (30, 31).
Independent variable of interest
The primary variable of interest was the implementation of Medicaid expansion under the ACA. This variable was operationalized as a categorical variable representing two time periods: pre-ACA (2011-2015) and post-ACA (2017-2021). Additionally, the interaction between the Medicaid implementation period and the state expansion status (Georgia vs. Louisiana) was examined to assess the differential impact of the policy on breast cancer outcomes across states.
Covariates
Covariates included demographic factors such as age at diagnosis (categorized as 18-44 years, 45-65 years, and >65 years), race/ethnicity (classified as non-Hispanic White, non-Hispanic Black, Hispanic, non-Hispanic Asian/Pacific Islander), and marital status. Socioeconomic status was assessed using household median income, categorized as <$75,000 and ≥$75,000. Clinical factors such as disease stage at presentation (localized, regional and distant metastasis) and treatment modalities (surgery, chemotherapy, radiation) were also considered as covariates (32, 33).
Theoretical model: Andersen behavioral model
This study uses the Andersen Behavioral Model of Health Services (34, 35) Use to explore the impact of the ACA and Medicaid expansion on breast cancer mortality, comparing Louisiana (which implemented Medicaid expansion in 2016) and Georgia (which has not). The model categorizes factors into predisposing, enabling, and need factors:
Predisposing Factors: These include demographics such as age (18-45, 45-64 years), race/ethnicity (White, Black, Hispanic, etc.), and marital status (single, married, etc.), which influence healthcare-seeking behavior.
Enabling Factors: These are resources that facilitate access to care, including income (<70K, ≥70K), metropolitan status (rural, small, medium, large metropolitan), and the state of residence (Louisiana vs. Georgia), reflecting the impact of Medicaid expansion.
Need Factors: These include clinical variables such as cancer grade (localized, regional, distant) (36), and the receipt of treatments like surgery, chemotherapy, and radiotherapy, all of which influence breast cancer outcomes.
Difference-in-differences specification
The present study used a Difference-in-Differences (DID) model to estimate the impact of Medicaid expansion on breast cancer outcomes, overall survival, overall deaths, and disease stage at presentation by comparing Louisiana (treatment group, which implemented Medicaid expansion) to Georgia (control group, which did not) during the pre-ACA and post-ACA periods. The variable ACA was set to 1 for the post-ACA period (2017–2021) and 0 for the pre-ACA period (2011–2015). The variable State was defined as 1 if the observation was from Louisiana (expansion state) and 0 if from Georgia (non-expansion state).
The DID model is specified as:
y=Xβ+β1·ACA+β2·State + β12· (ACA X State) +u (37).
y represents the breast cancer outcome of interest (e.g., survival, overall mortality, or disease stage at presentation).
X includes covariates such as age, race, marital status, and treatment modalities.
β1 captures the difference in outcomes between the pre- and post-ACA periods across both states.
β2 captures the baseline difference between Louisiana and Texas.
β12 represents the effect of Medicaid expansion on breast cancer outcomes.
The interaction term ACA × State (i.e., β12) estimates the difference in the change in outcomes between Louisiana (the expansion state) and Georgia (the non-expansion state) from the pre- to the post-ACA period. This term provides the key estimate of the impact of Medicaid expansion (37).
Sub-analysis: impact of chemotherapy on CSS and OS
The procedure was conducted using Inverse Probability Weighted Regression Adjustment (IPWRA) to evaluate the impact of chemotherapy on overall survival (OS) and cancer-specific mortality. We implemented the teffects ipwra command in Stata, which combines the benefits of both regression adjustment and propensity score weighting to estimate the Average Treatment Effect (ATE) of chemotherapy on survival outcomes. The treatment model was specified using a logit regression, with chemotherapy as the treatment variable. Covariates included the status of Medicaid expansion (EXPAND), post-ACA period (ACA), age (AGE2), race (i.Race), marital status (i.Married), income (Income2), metropolitan status (i.Metro), hormone receptor status (HER2, PRS, Estrogen), tumor characteristics (primary, Lateral), and cancer grade (i.Grade). This approach ensures that potential confounders are controlled, providing robust estimates of the effect of chemotherapy on survival outcomes in a population-based cohort.
Statistical analysis
Descriptive statistics were used to summarize the characteristics of the study population. Univariate analysis, employing chi-square tests for categorical variables, was conducted to examine the distribution of study variables across different groups. Statistical analyses were performed using appropriate regression models, such as multinomial logistic regression for the disease stage and Cox proportional hazards regression for time-to-event outcomes for the CSS and OS. A margins plot was utilized to visualize the adjusted probabilities of each outcome across states and time periods. Statistical significance was determined using two-tailed tests with a predetermined alpha level (e.g., p < 0.05). All analyses were conducted using STATA 16 statistical software package.
Baseline Characteristics
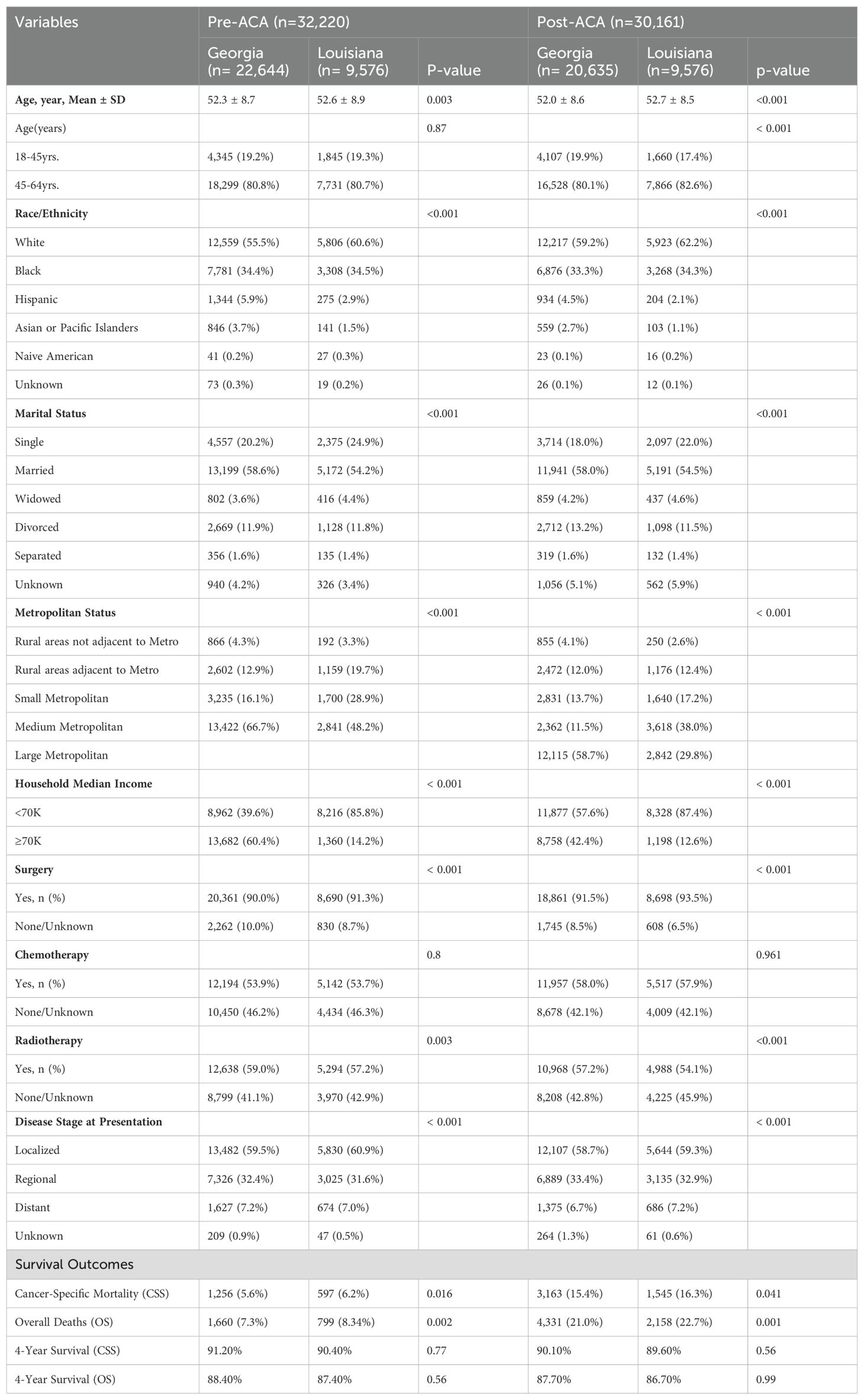
Table 1. Baseline characteristics of women with breast cancer in Georgia and Louisiana Pre-ACA and Post-ACA (2011-2021).
The baseline characteristics of women with breast cancer in Georgia and Louisiana during the pre-ACA (2011–2015) and post-ACA (2017–2021) periods highlight significant demographic and clinical differences between the states (Table 1). The study population consisted of 62,381 women, with 32,220 in the pre-ACA period and 30,161 in the post-ACA period. The mean age was slightly higher in Louisiana compared to Georgia in both periods (pre-ACA: 52.6 ± 8.9 vs. 52.3 ± 8.7, p=0.003; post-ACA: 52.7 ± 8.5 vs. 52.0 ± 8.6, p<0.001). The majority of women were aged 45-64 years in both states and time periods. Racial and ethnic distributions varied significantly, with a higher proportion of White women in Louisiana (pre-ACA: 60.6% vs. 55.5% in Georgia; post-ACA: 62.2% vs. 59.2% in Georgia, p<0.001), while Georgia had a larger proportion of Hispanic and Asian/Pacific Islander women in both periods.
Socioeconomic and Metropolitan Status
In Louisiana, a significantly higher proportion of women reported a household income of less than $70K compared to Georgia (pre-ACA: 85.8% vs. 39.6%, p<0.001; post-ACA: 87.4% vs. 57.6%, p<0.001) (Table 1). Georgia, on the other hand, had a higher percentage of women in the ≥$70K income category (pre-ACA: 60.4% vs. 14.2% in Louisiana; post-ACA: 42.4% vs. 12.6% in Louisiana, p<0.001). Metropolitan status also varied significantly, with the majority of women in Georgia residing in large metropolitan areas in the post-ACA period (58.7% vs. 29.8% in Louisiana, p<0.001), while Louisiana had a higher proportion of women in small and rural metropolitan areas.
Treatment Modalities and Disease Stage at Presentation
The treatment patterns showed significant variations between states (Table 1). Surgical rates were slightly higher in Louisiana during both periods (pre-ACA: 91.3% vs. 90.0% in Georgia; post-ACA: 93.5% vs. 91.5% in Georgia, p<0.001). Chemotherapy usage remained comparable between the states across both periods, while radiotherapy use was higher in Georgia (pre-ACA: 59.0% vs. 57.2% in Louisiana, p=0.003; post-ACA: 57.2% vs. 54.1%, p<0.001). Regarding disease stage at presentation, the proportion of women diagnosed at a localized stage was similar between states and periods (pre-ACA: 59.5% in Georgia vs. 60.9% in Louisiana; post-ACA: 58.7% in Georgia vs. 59.3% in Louisiana, p<0.001). However, there was a slight increase in distant-stage disease in Louisiana post-ACA (7.2% vs. 6.7% in Georgia, p<0.001).
Factors Associated with Cancer-Specific Survival
The Cox regression analysis indicated that the implementation of Medicaid expansioning Louisiana did not significantly affect CSS compared to Georgia (HR=1.070, 95% CI: 0.942-1.216, p=0.297) (Table 2). However, survival worsened in the post-ACA period (HR=1.216, 95% CI: 1.125-1.315, p<0.001), regardless of state. The interaction term between state and the post-ACA period was not significant (HR=0.953, 95% CI: 0.827-1.098, p=0.502), suggesting no differential effect of Medicaid expansion on survival between Louisiana and Georgia.
Demographic and Socioeconomic Factors
Age was not a significant predictor of cancer-specific mortality, but race/ethnicity had a substantial impact (Table 2). Black women had a significantly higher hazard of death compared to White women (HR=1.474, 95% CI: 1.392-1.561, p<0.001). Other racial/ethnic groups, such as Hispanics and Native Americans, did not show a significant difference from the reference group. Women in higher-income households (≥$70K) showed no significant survival benefit (HR=0.962, 95% CI: 0.894-1.034, p=0.291), but married women had a lower hazard of death (HR=0.807, 95% CI: 0.754-0.862, p<0.001) compared to single women.
Disease Stage, Metropolitan Status, and Treatment Modalities
Advanced disease stage was the strongest predictor of cancer-specific mortality, with the highest hazard observed in women with distant-stage disease (HR=12.315, 95% CI: 11.173-13.574, p<0.001) (Table 2). Women residing in large metropolitan areas had a significantly lower hazard of death (HR=0.747, 95% CI: 0.655-0.852, p<0.001) compared to those in rural areas. Treatment modalities also influenced survival, with surgery (HR=0.325, 95% CI: 0.299-0.354, p<0.001) and radiation (HR=0.834, 95% CI: 0.787-0.883, p<0.001) associated with improved outcomes, whereas chemotherapy was linked to an increased hazard of death (HR=1.341, 95% CI: 1.255-1.434, p<0.001).
Factors Associated with Overall Deaths
The Cox regression analysis for overall deaths indicated that the implementation of Medicaid expansion in Louisiana did not significantly impact overall survival compared to Georgia (HR=1.036, 95% CI: 0.928-1.157, p=0.529) (Table 3). However, the hazard of death was significantly higher in the post-ACA period compared to the pre-ACA period (HR=1.113, 95% CI: 1.039-1.192, p=0.002). The interaction term between state and post-ACA period was not significant (HR=0.994, 95% CI: 0.880-1.123, p=0.920), suggesting that the effect of the ACA on overall survival did not differ significantly between Louisiana and Georgia.
Demographic and Socioeconomic Factors
Age and race/ethnicity were significant predictors of overall survival (Table 3). Women aged 45-64 years had a higher hazard of death compared to the reference group (18-45 years) (HR=1.218, 95% CI: 1.148-1.293, p<0.001). Black women had a significantly increased hazard of death compared to White women (HR=1.406, 95% CI: 1.338-1.477, p<0.001). Household income was also associated with survival, as women from higher-income households (≥$70K) had a lower hazard of death (HR=0.909, 95% CI: 0.854-0.968, p=0.003) compared to those with an income of less than $70K. Metropolitan status showed a protective effect, with women residing in large metropolitan areas having a significantly lower hazard of death (HR=0.738, 95% CI: 0.660-0.825, p<0.001) compared to those in rural areas.
Disease Stage, Marital Status, and Treatment Modalities
Advanced disease stage was a strong predictor of overall mortality, with women presenting with distant-stage disease having a significantly higher hazard of death (HR=7.398, 95% CI: 6.812-8.034, p<0.001) compared to those with localized disease (Table 3). Marital status also influenced survival, with married women exhibiting a lower hazard of death (HR=0.735, 95% CI: 0.694-0.778, p<0.001) compared to single women, while widowed women had an elevated hazard of death (HR=1.143, 95% CI: 1.032-1.266, p=0.010). Among treatment modalities, surgery was associated with a significant reduction in mortality risk (HR=0.318, 95% CI: 0.296-0.343, p<0.001), while chemotherapy was associated with an increased hazard of death (HR=1.143, 95% CI: 1.083-1.206, p<0.001). Radiation therapy significantly improved overall survival (HR=0.812, 95% CI: 0.773-0.853, p<0.001).
Comparison of Treatment Patterns Between Georgia and Louisiana
Treatment patterns differed slightly between Georgia and Louisiana in both the pre- and post-ACA periods (Table 4). In the pre-ACA period, the majority of patients in both states received radiation after surgery (Georgia: 53.98% vs. Louisiana: 53.25%), while a significant proportion had no radiation or surgery (Georgia: 44.86% vs. Louisiana: 45.84%). Similar trends persisted in the post-ACA period, with slightly increased proportions of patients receiving no radiation or surgery in both states (Georgia: 45.21% vs. Louisiana: 47.55%, p<0.001). Interestingly, the use of intraoperative radiation was more frequent in Georgia than in Louisiana across both periods, though the overall numbers remained low.
For systemic therapy, the majority of women in both states received systemic therapy after surgery in the pre-ACA period (Georgia: 56.74% vs. Louisiana: 58.81%, p<0.001). However, this trend increased significantly in the post-ACA period, with both states showing higher utilization of systemic therapy after surgery (Georgia: 62.83% vs. Louisiana: 63.17%). The proportion of women receiving systemic therapy both before and after surgery decreased post-ACA in both states (Georgia: 7.81% vs. 6.96% in Louisiana) compared to the pre-ACA period (Georgia: 10.85% vs. 13.07% in Louisiana). Additionally, the number of women receiving systemic therapy before surgery declined post-ACA (Georgia: 9.34% vs. Louisiana: 7.34%, p<0.001).
Overall, the shift in treatment patterns post-ACA, particularly the increased reliance on systemic therapy after surgery, highlights evolving treatment strategies in both states, although the changes were more pronounced in Louisiana.
Baseline Characteristics of Breast Cancer Patients Receiving Chemotherapy Before and After Propensity Score Matching
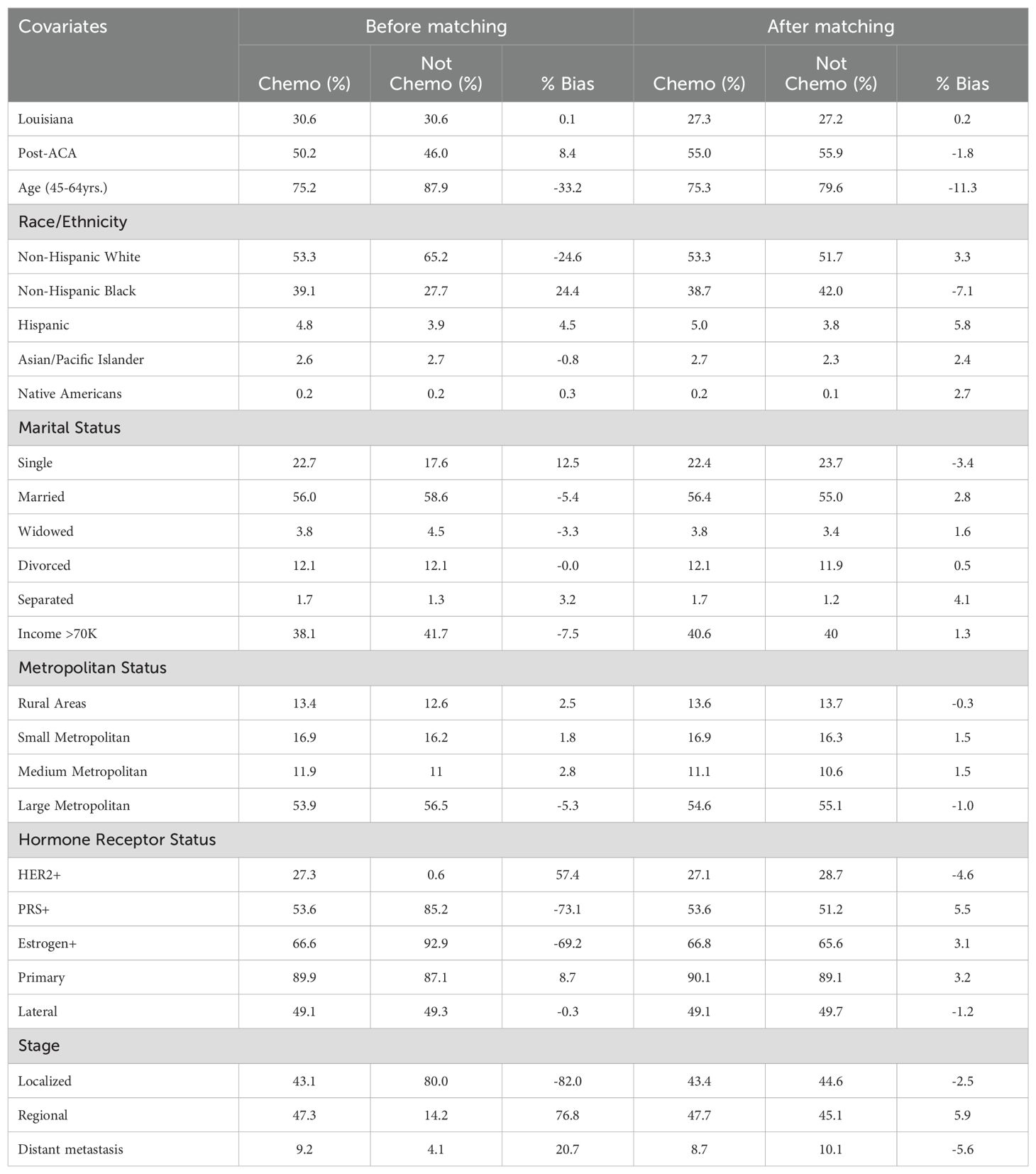
Table 5. Baseline characteristics of breast cancer patients receiving chemotherapy before and after propensity score matching.
Before propensity score matching, significant imbalances were observed across several covariates between patients who received chemotherapy and those who did not (Table 5). For instance, patients in the chemotherapy group had a higher proportion of Non-Hispanic Black individuals (39.1% vs. 27.7%, % bias = 24.4) and were less likely to have localized-stage cancer (43.1% vs. 80%, % bias = -82). Other notable disparities included hormone receptor status such as HER2 (27.3% vs. 6.6%, % bias = 57.4), PRS (53.6% vs. 85.2%, % bias = -73.1), and Estrogen receptor positivity (66.6% vs. 92.9%, % bias = -69.2). After matching, these imbalances were substantially reduced, achieving a closer balance between the two groups. For example, the % bias for the proportion of Non-Hispanic Black individuals dropped to -7.1%, and for localized-stage cancer, the % bias reduced to -2.5%. These results indicate that propensity score matching effectively minimized baseline differences, allowing for a more accurate comparison of outcomes between patients who received chemotherapy and those who did not.
Effect of Chemotherapy on Cancer-Specific Mortality and Overall Survival
The effect of chemotherapy on cancer-specific mortality and overall survival was evaluated using the Average Treatment Effect (ATE) (Table 6). Chemotherapy was significantly associated with a reduction in cancer-specific mortality, showing an 8.38 percentage point decrease compared to those who did not receive chemotherapy (ATE = -0.0838, SE = 0.0113, p < 0.001, 95% CI: -0.1059 to -0.0617). Similarly, chemotherapy was associated with a significant reduction in the risk of overall deaths by 10.53 percentage points (ATE = -0.1053, SE = 0.0115, p < 0.001, 95% CI: -0.1278 to -0.0828). The potential outcome mean (PO Mean) for cancer-specific mortality in the non-chemotherapy group was 19.92% (SE = 0.0112, 95% CI: 17.73% to 22.12%), while for overall deaths, the predicted risk was 25.55% (SE = 0.0114, 95% CI: 23.32% to 27.78%). These findings suggest that chemotherapy not only significantly reduces cancer-specific mortality but also lowers the risk of all-cause mortality in this population.
Impact of Medicaid Expansion on Cancer-Specific Deaths
The DID analysis did not show a significant change in CSS between Louisiana and Georgia across the pre- and post-ACA periods (Table 7). The overall coefficient for the difference in changes between the two states was -5.97 (95% CI: -26.09 to 14.15, p = 0.561), indicating no significant improvement or worsening of survival rates in Louisiana compared to Georgia. When stratified by race, similar patterns were observed across racial and ethnic groups, with coefficients ranging from -4.44 for Asian or Pacific Islanders (95% CI: -19.43 to 10.55, p = 0.561) to -7.33 for Black women (95% CI: -32.04 to 17.38, p = 0.561). The lack of significant change across all racial subgroups suggests that Medicaid expansion in Louisiana did not differentially impact CSS compared to Georgia, regardless of race.
Impact of Medicaid Expansion on Overall Mortality
The DID analysis revealed no significant changes in overall mortality between Louisiana and Georgia in the pre- and post-ACA periods (Table 8). The overall coefficient for the difference in changes between the two states was -0.26 (95% CI: -10.91 to 10.39, p = 0.962), indicating that Medicaid expansion did not result in a measurable difference in overall mortality trends between the two states. When examined by race, similar non-significant results were observed, with coefficients ranging from -0.18 for Asian or Pacific Islanders (95% CI: -7.65 to 7.29, p = 0.962) to -0.34 for Native Americans (95% CI: -14.22 to 13.54, p = 0.962). These findings suggest that the implementation of Medicaid expansion in Louisiana did not significantly influence overall mortality rates compared to Georgia, across all racial and ethnic groups.
Impact of Medicaid Expansion on Disease Stage at Presentation
The DID analysis showed no significant change in the proportion of patients presenting at localized or regional stages in Louisiana compared to Georgia following Medicaid expansion (Table 9). The coefficient for localized stage was -1.27 (95% CI: -3.31 to 0.77, p = 0.221), and for regional stage, the coefficient was 0.47 (95% CI: -1.48 to 2.43, p = 0.635), indicating no significant shifts in early-stage presentations. However, there was a marginally significant increase in the likelihood of distant metastasis at presentation (coefficient: 1.08, 95% CI: -0.01 to 2.17, p = 0.052), suggesting a possible trend toward more advanced stage diagnoses post-ACA in Louisiana relative to Georgia, though this finding did not reach conventional levels of statistical significance.
Discussion
In this study, we sought to evaluate the causal impact of Medicaid expansion in Louisiana, which implemented the policy in 2016, by comparing cancer-specific survival (CSS) and overall mortality among women with breast cancer aged 18-64 years to those in Georgia, a state that has not yet implemented Medicaid expansion. This age group was chosen because individuals aged 18-64 (38) are more likely to benefit from Medicaid expansion, given that individuals over 65 are primarily covered by Medicare (39). Our analysis, which was stratified by income (households earning below the median income of $70,000 in both states), focused on changes in CSS, OS, and disease stage at presentation.
Main findings
We found no statistically significant differences in CSS between Louisiana and Georgia following Medicaid expansion. Specifically, individuals in Louisiana experienced an estimated 6% reduction in CSS relative to their counterparts in Georgia. However, the confidence intervals were wide, ranging from a 26% reduction to a 14.2% increase, indicating high variability and uncertainty. Similarly, no significant differences were observed across different race/ethnic groups, though there was a non-significant trend toward a 7.3% reduction in CSS for non-Hispanic Black women in Louisiana (95% CI: -32.0% to +17.4%).
A potential explanation for the lack of statistical significance is the latency of breast cancer (40, 41). Breast cancer is a disease with a relatively long progression and treatment course, and differences in survival outcomes might take longer to manifest (40, 42, 43). This delayed impact could obscure any immediate differences between the states within the study period.
Moreover, while there was no significant increase in OS, the trends in Louisiana were positive, showing possible improvements in both CCS and OS. This trend, although not statistically significant, suggests that Medicaid expansion may lead to improved outcomes over time (44, 45).
Disease stage at presentation
We examined cancer staging at presentation, classifying breast cancer cases as localized, regional, or distant metastatic. Similar to CSS and OS, there were no significant differences in the stage at presentation between the two states following Medicaid expansion. This may be partly due to the pre-existing national programs such as the National Breast and Cervical Cancer Early Detection Program (46), which predates the ACA and may have blunted the impact of Medicaid expansion on cancer screening and early detection.
Comparison with existing literature
Our findings align with some existing literature that also reports no immediate, significant effect of Medicaid expansion on cancer-specific mortality in the short term (47). For instance, previous studies examining cancer outcomes post-Medicaid expansion have found mixed results, with some studies indicating improvements in early detection and care access but no statistically significant change in survival outcomes during the first few years post-expansion (48, 49).
One key explanation in the literature is that the benefits of policy changes like Medicaid expansion may take time to manifest, particularly for diseases with longer survival curves, such as cancer (10, 48, 50). For instance, research has shown that while expansion improves access to care, it may take several years for this to translate into improved long-term survival, as newly insured patients may not access care immediately, and cancer treatment is often a lengthy process (50, 51). Moreover, socioeconomic factors and pre-existing disparities may further complicate short-term outcomes (52, 53).
The absence of significant differences in survival outcomes between Louisiana and Georgia post-Medicaid expansion could be attributed to several interconnected factors. First, the latent nature of breast cancer might delay observable impacts on survival outcomes. Breast cancer is a disease with a prolonged progression, and changes in mortality outcomes may not manifest until several years after diagnosis and treatment initiation (54). Research shows that it often takes time for the benefits of improved access to healthcare, such as that provided by Medicaid expansion, to influence long-term outcomes like cancer survival (10). Thus, the full effects of Medicaid expansion in Louisiana may not yet be apparent within the study period. Moreover, the capacity of Louisiana’s healthcare system may have played a role. Medicaid expansion often leads to an influx of newly insured individuals, and if the healthcare infrastructure in Louisiana was already strained, particularly in rural or underserved areas, this could limit access to timely and quality cancer care, delaying the potential benefits of the policy (10, 55).
Additionally, national programs predating the Affordable Care Act, such as the National Breast and Cervical Cancer Early Detection Program, may have already been effective in both states, reducing the observable impact of Medicaid expansion. Both Louisiana and Georgia likely benefited from these programs, which have been instrumental in providing low-income and uninsured women access to cancer screening and early detection services (44, 56). Furthermore, differences in baseline health conditions between the populations of Louisiana and Georgia could have influenced the results (57). If Louisiana had a higher burden of comorbidities such as obesity or hypertension, which are known to impact cancer outcomes, the Medicaid expansion may not have fully offset these pre-existing health disparities (58, 59). In addition, persistent racial and socioeconomic disparities likely played a significant role (60–62). Despite improved access to healthcare through Medicaid expansion, Black women continued to experience worse CSS compared to White women, highlighting that access alone does not address deeper social determinants of health, such as poverty, education, and systemic racism (63).
Lastly, limitations in the study design and data may have contributed to the lack of significant findings. The sample size, particularly for subgroups such as racial/ethnic minorities, may have been too small to detect meaningful differences, while variability in outcomes and wide confidence intervals could indicate that the analysis lacked sufficient statistical power (64). Furthermore, differences in how cancer data were recorded and classified between the two states may have introduced inconsistencies, masking real differences in outcomes (65). These methodological challenges, combined with the aforementioned factors, underscore the complexity of evaluating the impact of Medicaid expansion on cancer-specific outcomes (10, 48, 50). Longer-term studies with larger, more diverse samples are likely needed to fully capture the effects of Medicaid expansion on cancer survival.
Chemotherapy and survival outcomes
In the initial Cox regression analysis, chemotherapy was paradoxically associated with worse cancer-specific and overall survival. This counterintuitive finding likely reflects confounding by indication, as patients receiving chemotherapy may have had more advanced disease or unfavorable prognostic factors, leading to poorer survival outcomes. Similar paradoxical associations due to confounding by indication have been observed in other observational studies of cancer treatments (66). However, after applying propensity score matching to balance these baseline differences, chemotherapy was associated with improved cancer-specific and overall survival. This suggests that when accounting for patient characteristics and treatment selection bias, chemotherapy indeed offers a survival benefit, consistent with its intended therapeutic effect (67–69). Our findings align with prior studies that have reported similar associations between chemotherapy and improved outcomes in appropriately matched cohorts, highlighting the importance of adjusting for baseline imbalances when assessing treatment efficacy (70–72). These results underscore the potential misinterpretation of chemotherapy effects without rigorous control for confounding and support the use of propensity score matching in observational analyses to yield more reliable estimates.
Implications for policy
While the results of this study did not reach statistical significance, the trends suggest that Medicaid expansion may contribute to a gradual reduction in cancer-specific mortality and overall deaths. Policymakers should consider that the full effects of expansion might take longer to manifest in populations with complex, long-term health needs, such as cancer patients. Further, efforts to address social determinants of health, beyond just expanding access to healthcare are crucial for reducing racial and socioeconomic disparities in cancer outcomes.
Study limitation
This study has several important limitations. First, although the SEER registry provides a robust source of cancer data, it is limited in capturing other clinical and socioeconomic variables that may impact cancer-specific outcomes, such as comorbidities, healthcare access, and treatment adherence. The SEER database also lacks detailed information on insurance status, limiting our ability to track changes in coverage following Medicaid expansion. Furthermore, SEER is observational in nature, and while our difference-in-differences approach helps mitigate some confounding factors, it does not establish definitive causality. Another key limitation is the follow-up period, which may be too short to observe the full long-term impact of Medicaid expansion on breast cancer outcomes, particularly given the long latency of the disease.
Conclusion
The present study highlights the importance of a long-term perspective when evaluating the effects of Medicaid expansion on cancer survival. Continued monitoring and analysis are necessary to fully understand the potential benefits of Medicaid expansion for underserved populations, especially those facing socioeconomic barriers to care.
Data availability statement
The raw data supporting the conclusions of this article will be made available by the authors, without undue reservation.
Ethics statement
Ethical approval was not required for the study involving humans in accordance with the local legislation and institutional requirements. Written informed consent for participation was not required from the participants or the participants’ legal guardians/next of kin in accordance with the national legislation and institutional requirements.
Author contributions
OA: Conceptualization, Data curation, Formal analysis, Funding acquisition, Investigation, Methodology, Project administration, Resources, Software, Supervision, Validation, Visualization, Writing – original draft, Writing – review & editing. MF: Conceptualization, Data curation, Project administration, Resources, Software, Validation, Visualization, Writing – review & editing. TW: Conceptualization, Methodology, Project administration, Resources, Validation, Visualization, Writing – review & editing. EO: Conceptualization, Methodology, Project administration, Resources, Software, Validation, Visualization, Writing – original draft, Writing – review & editing. IA: Conceptualization, Resources, Validation, Visualization, Writing – review & editing, Data curation, Project administration, Software. KG: Conceptualization, Project administration, Resources, Validation, Visualization, Writing – review & editing, Data curation. MA: Conceptualization, Data curation, Project administration, Validation, Writing – review & editing, Resources, Software, Visualization. MM: Conceptualization, Resources, Validation, Visualization, Writing – review & editing, Funding acquisition, Supervision, Investigation, Methodology, Writing – original draft. KH: Conceptualization, Project administration, Resources, Validation, Visualization, Writing – review & editing, Supervision, Funding acquisition. RW: Conceptualization, Data curation, Project administration, Validation, Writing – review & editing, Investigation, Methodology, Supervision.
Funding
The author(s) declare that no financial support was received for the research, authorship, and/or publication of this article.
Conflict of interest
The authors declare that the research was conducted in the absence of any commercial or financial relationships that could be construed as a potential conflict of interest.
Publisher’s note
All claims expressed in this article are solely those of the authors and do not necessarily represent those of their affiliated organizations, or those of the publisher, the editors and the reviewers. Any product that may be evaluated in this article, or claim that may be made by its manufacturer, is not guaranteed or endorsed by the publisher.
References
1. Menon G, Alkabban FM, Ferguson T. Breast Cancer. StatPearls. Treasure Island (FL: StatPearls Publishing Copyright © 2024, StatPearls Publishing LLC (2024).
2. Xu S, Murtagh S, Han Y, Wan F, Toriola AT. Breast cancer incidence among US women aged 20 to 49 years by race, stage, and hormone receptor status. JAMA Netw Open. (2024) 7:e2353331–e. doi: 10.1001/jamanetworkopen.2023.53331
3. Wilson J, Sule AA. Disparity in Early Detection of Breast Cancer. StatPearls. Treasure Island (FL: StatPearls Publishing Copyright © 2024, StatPearls Publishing LLC (2024).
4. KFF Tisfhpr, polling, and news. Woman’s health policy 2024. Available online at: https://www.kff.org/womens-health-policy/fact-sheet/preventive-services-covered-by-private-health-plans/ (Accessed September 28, 2024).
5. Persaud N, Sabir A, Woods H, Sayani A, Agarwal A, Chowdhury M, et al. Preventive care recommendations to promote health equity. Cmaj. (2023) 195:E1250–e73. doi: 10.1503/cmaj.230237
6. Forum on M, Public Health Preparedness for Catastrophic E, Board on Health Sciences P, Board on Health Care S, Institute of M. The National Academies Collection: Reports funded by National Institutes of Health. In: The Impacts of the Affordable Care Act on Preparedness Resources and Programs: Workshop Summary. National Academies Press (US, Washington DC (2014).
7. Akinyemi O, Weldeslase T, Fasokun M, Odusanya E, Tsion A, Cornwell E, et al. Impact of the affordable care act on revascularization versus amputation in patients presenting with chronic limb-threatening ischemia in Maryland. Am Surgeon™. (2024) 90:2907–12. doi: 10.1177/00031348241259046
8. Akinyemi OA, Weldeslase TA, Fasokun ME, Odusanya E, Mejulu EO, Salihu EY, et al. Causal effects of the affordable care act (ACA) implementation on non-Hodgkin’s lymphoma survival: A difference-in-differences analysis. Cureus. (2024) 16:e52571. doi: 10.7759/cureus.52571
9. Lyon SM, Douglas IS, Cooke CR. Medicaid expansion under the Affordable Care Act. Implications for insurance-related disparities in pulmonary, critical care, and sleep. Ann Am Thorac Soc. (2014) 11:661–7. doi: 10.1513/AnnalsATS.201402-072PS
10. Hotca A, Bloom JR, Runnels J, Salgado LR, Cherry DR, Hsieh K, et al. The impact of medicaid expansion on patients with cancer in the United States: A review. Curr Oncol. (2023) 30:6362–73. doi: 10.3390/curroncol30070469
11. Zhao J, Mao Z, Fedewa SA, Nogueira L, Yabroff KR, Jemal A, et al. The Affordable Care Act and access to care across the cancer control continuum: A review at 10 years. CA: A Cancer J Clin. (2020) 70:165–81. doi: 10.3322/caac.21604
12. Martinez ME, Gomez SL, Canchola AJ, Oh DL, Murphy JD, Mehtsun W, et al. Changes in cancer mortality by race and ethnicity following the implementation of the affordable care act in California. Front Oncol. (2022) 12:916167. doi: 10.3389/fonc.2022.916167
13. Bazzi T, Al-Husseini M, Saravolatz L, Kafri Z. Trends in breast cancer incidence and mortality in the United States from 2004-2018: A surveillance, epidemiology, and end results (SEER)-based study. Cureus. (2023) 15:e37982. doi: 10.7759/cureus.37982
14. NIH NCI. Overview of the SEER Program 2024. In: Seer Is An Authoritative Source For Cancer Statistics In The United States. The Surveillance, Epidemiology, and End Results (SEER) Program (2024). Available at: https://seer.cancer.gov/about/overview.html. SEER is supported by the Surveillance Research Program (SRP) in NCI’s Division of Cancer Control and Population Sciences (DCCPS).
15. Goel N, Hernandez AE, Mazul A. Neighborhood disadvantage and breast cancer–specific survival in the US. JAMA Netw Open. (2024) 7:e247336–e. doi: 10.1001/jamanetworkopen.2024.7336
16. KFF Tisfhpr, polling, and news. Status of State Medicaid Expansion Decisions: Interactive Map (2024). Available online at: https://www.kff.org/affordable-care-act/issue-brief/status-of-state-medicaid-expansion-decisions-interactive-map/ (Accessed September 28, 2024).
17. Sullivan Aoagl J. Entering Their Second Decade, Affordable Care Act Coverage Expansions Have Helped Millions, Provide the Basis for Further Progress (2024). Available online at: https://www.cbpp.org/research/health/entering-their-second-decade-affordable-care-act-coverage-expansions-have-helped (Accessed September 28, 2024).
18. Pruitt SL, Shim MJ, Mullen PD, Vernon SW, Amick BC 3rd. Association of area socioeconomic status and breast, cervical, and colorectal cancer screening: a systematic review. Cancer Epidemiol Biomarkers Prev. (2009) 18:2579–99. doi: 10.1158/1055-9965.EPI-09-0135
19. DPH GDoPh. Breast and Cervical Cancer (2023). Available online at: https://dph.Georgia.gov/BCCP (Accessed September 28, 2024).
20. American College of Radiology. New ACR Breast Cancer Screening Guidelines call for earlier and more-intensive screening for high-risk women 2023. Available online at: https://www.acr.org/Media-Center/ACR-News-Releases/2023/New-ACR-Breast-Cancer-Screening-Guidelines-call-for-earlier-screening-for-high-risk-women (Accessed September 28, 2024).
21. U.S. Preventive Services Task Force yoiH. Breast Cancer: Screening (2024). Available online at: https://www.uspreventiveservicestaskforce.org/uspstf/recommendation/breast-cancer-screening (Accessed September 28, 2024).
22. Jersey SoN, SERVICES DOH, DIVISION OF MEDICAL ASSISTANCE AND HEALTH SERVICE. Affordable Care Act Eligibility Information. New Jersey (2014).
23. Boles W, Kennedy R, Siewert E, Rowland D, Lyons B, Gee RE. A playbook for implementing medicaid expansion: Louisiana’s experience. Milbank Q. (2022) 100:11–37. doi: 10.1111/1468-0009.12540
24. Hamer D, Mandala D, Jones G, Knapp GM, Jagneaux T. Effect of medicaid expansion on visit composition in a Louisiana health care system. Ochsner J. (2022) 22:154–62. doi: 10.31486/toj.21.0106
25. GCDD GCoDD. Georgia Medicaid Waivers (2024). Available online at: https://gcdd.org/Georgia-medicaid-waivers.html (Accessed September 28, 2024).
26. Georgians for a healthy future. Georgia Pathways to Coverage (“Pathways”) Fact Sheet. Available at: https://healthyfuturega.org/ghf_resource/Georgia-pathways-to-coverage-pathways-fact-sheet/ (Accessed September 29, 2024).
27. Wadasadawala T, Patil R, Carlton J, Verma S, Umesh N, Rane P, et al. Long-term outcomes and prognostic factors in elderly patients with breast cancer: single-institutional experience. Ecancermedicalscience. (2023) 17:1542. doi: 10.3332/ecancer.2023.1542
28. Liu X, Morelli D, Littlejohns TJ, Clifton DA, Clifton L. Combining machine learning with Cox models to identify predictors for incident post-menopausal breast cancer in the UK Biobank. Sci Rep. (2023) 13:9221. doi: 10.1038/s41598-023-36214-0
29. Abadi A, Yavari P, Dehghani-Arani M, Alavi-Majd H, Ghasemi E, Amanpour F, et al. Cox models survival analysis based on breast cancer treatments. Iran J Cancer Prev. (2014) 7:124–9. https://pubmed.ncbi.nlm.nih.gov/25250162/.
30. NIH NCI. SEER Summary Staging Manual (2000). Available online at: https://seer.cancer.gov/tools/ssm/ssm2000/ (Accessed September 28, 2024).
31. Wu XC, Yu Q, Andrews PA, Ranganath P, Qiao B, Ajani U, et al. Comparisons of directly coded SEER Summary Stage 2000 and Collaborative Staging Derived SEER Summary Stage 2000. J Registry Manage. (2010) 37:137–40. https://pubmed.ncbi.nlm.nih.gov/21688742/.
32. City of Hope RbDL, MD. Breast Cancer Treatment (2022). Available online at: https://www.cancercenter.com/cancer-types/breast-cancer/treatments (Accessed September 29, 2024).
33. ACS ACoS. Breast Cancer Staging . Available online at: https://www.facs.org/for-patients/the-day-of-your-surgery/breast-cancer-surgery/breast-cancer-types/breast-cancer-staging/ (Accessed September 29, 2024).
34. Alkhawaldeh A, AL M, Rayan A, Abdalrahim A, Musa A, Eshah N, et al. Application and use of Andersen’s behavioral model as theoretical framework: A systematic literature review from 2012-2021. Iran J Public Health. (2023) 52:1346–54. doi: 10.18502/ijph.v52i7.13236
35. Krzyż EZ, Antunez Martinez OF, Lin HR. Uses of Andersen health services utilization framework to determine healthcare utilization for mental health among migrants-a scoping review. Front Public Health. (2023) 11:1284784. https://pmc.ncbi.nlm.nih.gov/articles/PMC10761300/.
36. American Cancer Society. Survival Rates for Breast Cancer (2024). Available online at: https://www.cancer.org/cancer/types/breast-cancer/understanding-a-breast-cancer-diagnosis/breast-cancer-survival-rates.html (Accessed September 29, 2024).
37. Forcasting SMa. Introduction To The Difference-In-Differences Regression Model. Available online at: https://timeseriesreasoning.com/contents/introduction-to-the-difference-in-differences-regression-model/ (Accessed September 29, 2024).
38. Bullinger LR, Meinhofer A. The affordable care act increased medicaid coverage among former foster youth. Health Aff (Millwood). (2021) 40:1430–9. doi: 10.1377/hlthaff.2021.00073
39. Medicare.gov. Get started with Medicare. Available online at: https://www.medicare.gov/basics/get-started-with-medicare (Accessed September 29, 2024).
40. Ho PJ, Cook AR, Binte Mohamed Ri NK, Liu J, Li J, Hartman M. Impact of delayed treatment in women diagnosed with breast cancer: A population-based study. Cancer Med. (2020) 9:2435–44. doi: 10.1002/cam4.2830
41. Olsson H, Baldetorp B, Fernö M, Perfekt R. Relation between the rate of tumour cell proliferation and latency time in radiation associated breast cancer. BMC Cancer. (2003) 3:11. doi: 10.1186/1471-2407-3-11
42. Li Y, Zhou Y, Mao F, Guan J, Lin Y, Wang X, et al. The influence on survival of delay in the treatment initiation of screening detected non-symptomatic breast cancer. Sci Rep. (2019) 9:10158. doi: 10.1038/s41598-019-46736-1
43. Kim K, Marquez-Palencia M, Malladi S. Metastatic latency, a veiled threat. Front Immunol. (2019) 10. doi: 10.3389/fimmu.2019.01836
44. Primm KM, Zhao H, Adjei NN, Sun CC, Haas A, Meyer LA, et al. Effect of Medicaid expansion on cancer treatment and survival among Medicaid beneficiaries and the uninsured. Cancer Med. (2024) 13:e7461. doi: 10.1002/cam4.v13.13
45. Lin Y, Monnette A, Shi L. Effects of medicaid expansion on poverty disparities in health insurance coverage. Int J Equity Health. (2021) 20:171. doi: 10.1186/s12939-021-01486-3
46. Wong FL, Miller JW. Centers for disease control and prevention’s national breast and cervical cancer early detection program: increasing access to screening. J Womens Health (Larchmt). (2019) 28:427–31. doi: 10.1089/jwh.2019.7726
47. KFF Tisfhpr, polling, and news. Building on the Evidence Base: Studies on the Effects of Medicaid Expansion, February 2020 to March 2021 (2021). Available online at: https://www.kff.org/report-section/building-on-the-evidence-base-studies-on-the-effects-of-medicaid-expansion-february-2020-to-march-2021-report/ (Accessed September 28, 2024).
48. Lam MB, Phelan J, Orav EJ, Jha AK, Keating NL. Medicaid expansion and mortality among patients with breast, lung, and colorectal cancer. JAMA Netw Open. (2020) 3:e2024366–e. doi: 10.1001/jamanetworkopen.2020.24366
49. Barnes JM, Johnson KJ, Adjei Boakye E, Schapira L, Akinyemiju T, Park EM, et al. Early medicaid expansion and cancer mortality. J Natl Cancer Inst. (2021) 113:1714–22. doi: 10.1093/jnci/djab135
50. Nogueira LM, Boffa DJ, Jemal A, Han X, Yabroff KR. Medicaid expansion under the affordable care act and early mortality following lung cancer surgery. JAMA Networ Open. (2024) 7:e2351529–e. doi: 10.1001/jamanetworkopen.2023.51529
51. Fund TC. Impact of the Medicaid Coverage Gap: Comparing States That Have and Have Not Expanded Eligibility (2023). Available online at: https://www.commonwealthfund.org/publications/issue-briefs/2023/sep/impact-medicaid-coverage-gap-comparing-states-have-and-have-not (Accessed September 28, 2024).
52. Dreyer MS, Nattinger AB, McGinley EL, Pezzin LE. Socioeconomic status and breast cancer treatment. Breast Cancer Res Treat. (2018) 167:1–8. doi: 10.1007/s10549-017-4490-3
53. Woods LM, Rachet B, Morris M, Bhaskaran K, Coleman MP. Are socio-economic inequalities in breast cancer survival explained by peri-diagnostic factors? BMC Cancer. (2021) 21:485. doi: 10.1186/s12885-021-08087-x
54. Pan H, Gray R, Braybrooke J, Davies C, Taylor C, McGale P, et al. 20-Year Risks of Breast-Cancer Recurrence after Stopping Endocrine Therapy at 5 Years. New Engl J Med. (2017) 377:1836–46. doi: 10.1056/NEJMoa1701830
55. Chu QD, Li T, Hsieh MC, Yi Y, Gibbs JF, Lyons JM 3rd, et al. Positive impact of the Patient Protection and Affordable Care Act Medicaid expansion on Louisiana women with breast cancer. Cancer. (2021) 127:688–99. doi: 10.1002/cncr.v127.5
56. American Cancer Society CAN. The National Breast & Cervical Cancer Early Detection Program (2024). Available online at: https://www.fightcancer.org/policy-resources/national-breast-cervical-cancer-early-detection-program (Accessed September 29, 2024).
57. Katzmarzyk PT, Martin CK, Newton RL Jr., Apolzan JW, Arnold CL, Davis TC, et al. Promoting Successful Weight Loss in Primary Care in Louisiana (PROPEL): Rationale, design and baseline characteristics. Contemp Clin Trials. (2018) 67:1–10. doi: 10.1016/j.cct.2018.02.002
58. Pati S, Irfan W, Jameel A, Ahmed S, Shahid RK. Obesity and cancer: A current overview of epidemiology, pathogenesis, outcomes, and management. Cancers (Basel). (2023) 15:485. doi: 10.3390/cancers15020485
59. Petrelli F, Cortellini A, Indini A, Tomasello G, Ghidini M, Nigro O, et al. Association of obesity with survival outcomes in patients with cancer: A systematic review and meta-analysis. JAMA Netw Open. (2021) 4:e213520–e. doi: 10.1001/jamanetworkopen.2021.3520
60. Jatoi I, Sung H, Jemal A. The emergence of the racial disparity in U.S. Breast-cancer mortality. New Engl J Med. (2022) 386:2349–52. doi: 10.1056/NEJMp2200244
61. Yedjou CG, Sims JN, Miele L, Noubissi F, Lowe L, Fonseca DD, et al. Health and racial disparity in breast cancer. Adv Exp Med Biol. (2019) 1152:31–49. doi: 10.1007/978-3-030-20301-6_3
62. Hirko KA, Rocque G, Reasor E, Taye A, Daly A, Cutress RI, et al. The impact of race and ethnicity in breast cancer—disparities and implications for precision oncology. BMC Med. (2022) 20:72. doi: 10.1186/s12916-022-02260-0
63. Dean HD, Williams KM, Fenton KA. From theory to action: applying social determinants of health to public health practice. Public Health Rep. (2013) 128 Suppl 3:1–4. doi: 10.1177/00333549131286S301
64. Suresh K, Chandrashekara S. Sample size estimation and power analysis for clinical research studies. J Hum Reprod Sci. (2012) 5:7–13. doi: 10.4103/0974-1208.97779
65. Sophia Z, Shalhout DMM. Data Quality Improvement in Cancer Registries- Error-Injection Tests (2021). Available online at: https://www.themillerlab.io/posts/optimizing_rwd_collection-qi-initiative-error_injection_tests/ (Accessed September 29, 2024).
66. Giordano SH, Temin S, Chandarlapaty S, Crews JR, Esteva FJ, Kirshner JJ, et al. Systemic therapy for patients with advanced human epidermal growth factor receptor 2-positive breast cancer: ASCO clinical practice guideline update. J Clin Oncol. (2018) 36:2736–40. doi: 10.1200/JCO.2018.79.2697
67. Sirikul W, Buawangpong N, Pruksakorn D, Charoentum C, Teeyakasem P, Koonrungsesomboon N. The survival outcomes, prognostic factors and adverse events following systemic chemotherapy treatment in bone sarcomas: A retrospective observational study from the experience of the cancer referral center in Northern Thailand. Cancers (Basel). (2023) 15:1979. doi: 10.3390/cancers15071979
68. Tagawa ST, Ramaswamy K, Huang A, Mardekian J, Schultz NM, Wang L, et al. Survival outcomes in patients with chemotherapy-naive metastatic castration-resistant prostate cancer treated with enzalutamide or abiraterone acetate. Prostate Cancer Prostatic Dis. (2021) 24:1032–40. doi: 10.1038/s41391-021-00318-3
69. Récher C, Röllig C, Bérard E, Bertoli S, Dumas PY, Tavitian S, et al. Long-term survival after intensive chemotherapy or hypomethylating agents in AML patients aged 70 years and older: a large patient data set study from European registries. Leukemia. (2022) 36:913–22. doi: 10.1038/s41375-021-01425-9
70. Hoeh B, Würnschimmel C, Flammia RS, Horlemann B, Sorce G, Chierigo F, et al. Effect of chemotherapy on overall survival in contemporary metastatic prostate cancer patients. Front Oncol. (2021) 11. doi: 10.3389/fonc.2021.778858
71. Doleschal B, Taghizadeh H, Webersinke G, Piringer G, Schreil G, Decker J, et al. Real world evidence reveals improved survival outcomes in biliary tract cancer through molecular matched targeted treatment. Sci Rep. (2023) 13:15421. doi: 10.1038/s41598-023-42083-4
Keywords: Georgia, Louisiana, affordable care act, Medicaid expansion, overall mortality, cancer-specific mortality, inverse probability of treatment weighting, difference - in – differences
Citation: Akinyemi O, Fasokun M, Weldeslase T, Odusanya E, Akinyemi I, Geter K, Akula M, Michael M, Hughes K and Williams R (2024) Comparative impact of the affordable care act on breast cancer outcomes among women in two US states. Front. Oncol. 14:1460714. doi: 10.3389/fonc.2024.1460714
Received: 06 July 2024; Accepted: 14 October 2024;
Published: 07 November 2024.
Edited by:
Victoria Seewaldt, City of Hope, United StatesReviewed by:
Saber A. Amin, University of Nebraska Medical Center, United StatesJunlin Liao, The University of Iowa, United States
Copyright © 2024 Akinyemi, Fasokun, Weldeslase, Odusanya, Akinyemi, Geter, Akula, Michael, Hughes and Williams. This is an open-access article distributed under the terms of the Creative Commons Attribution License (CC BY). The use, distribution or reproduction in other forums is permitted, provided the original author(s) and the copyright owner(s) are credited and that the original publication in this journal is cited, in accordance with accepted academic practice. No use, distribution or reproduction is permitted which does not comply with these terms.
*Correspondence: Oluwasegun Akinyemi, YXVzdGluZWFraW55ZW1pQGdtYWlsLmNvbQ==