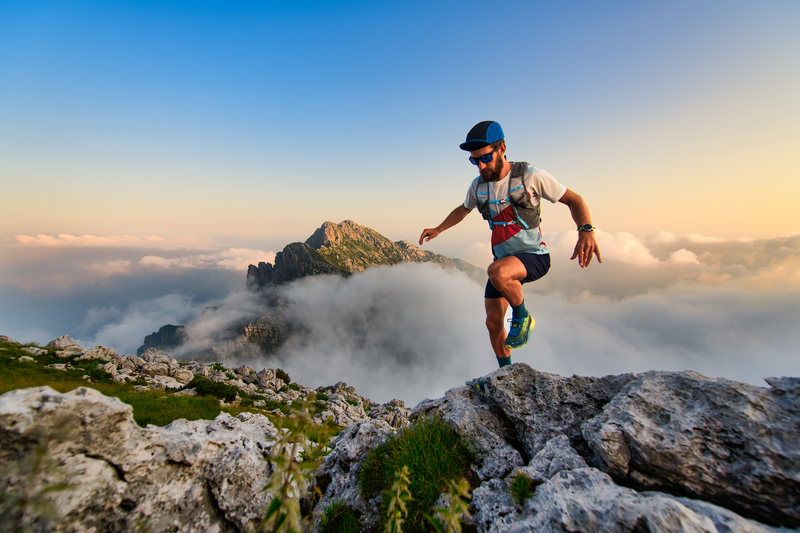
95% of researchers rate our articles as excellent or good
Learn more about the work of our research integrity team to safeguard the quality of each article we publish.
Find out more
ORIGINAL RESEARCH article
Front. Oncol. , 21 November 2024
Sec. Breast Cancer
Volume 14 - 2024 | https://doi.org/10.3389/fonc.2024.1449956
This article is part of the Research Topic Synthetic Biology and Metabolomics: Novel Insight in Oncology Research View all 4 articles
Background: Observational investigations have indicated a notable correlation between body mass index (BMI) and breast cancer (BC). Nevertheless, the precise biological pathways driving this correlation remain ambiguous. Consequently, we utilized Mendelian randomization (MR) techniques to explore the causative link between BMI and genetic predisposition to BC, as well as the potential intermediary influences.
Methods: Utilizing extensive cohorts sourced from publicly accessible genome-wide association studies (GWAS) datasets of European populations, we conducted Mendelian randomization (MR) analysis. The primary method employed was the Inverse Variance Weighted (IVW) model. We evaluated both heterogeneity and horizontal pleiotropy. Our MR analysis unveiled several metabolites and sex hormones as mediators in the association between BMI and BC.
Results: The IVW model indicated significant negative causal correlations between BMI and BC, ER+BC, and ER-BC. Thirty-five metabolites, thirty-three metabolites and sex hormones, and fifteen metabolites respectively mediated the causal effects of BMI on BC, ER+BC, and ER-BC. Furthermore, our study found that BMI influences BC risk through different mediating factors; BMI increases ER+BC risk through the pathway of sex hormones (biologically available testosterone) and decreases the causal relationship of BC risk through multiple metabolite pathways.
Conclusion: This study discovered that BMI increases ER+BC risk through the pathway of sex hormones (biologically available testosterone), and decreases BC risk through multiple metabolite pathways causally. These discoveries could offer insights into the development of preventive strategies and interventions for BC, while further investigations should delve into alternative feasible biological pathways.
Breast cancer (BC) ranks among the most prevalent malignant tumors in women, accounting for a considerable proportion of female malignancies (1). Globally, the incidence of BC is on the rise, despite advancements in early detection and treatment. Nonetheless, BC continues to stand as a prominent contributor to mortality among women attributed to cancer (2). The occurrence of BC involves various factors, including age, family history, reproductive factors, estrogen, and lifestyle (3). In recent years, the widespread utilization of genetic prediction causal inference methods has offered new insights into early screening and prevention strategies for complex diseases. This study aims to utilize genetic instruments to investigate body mass index (BMI) as a potential risk factor for BC and explore its potential mediating effects, offering fresh perspectives on early prevention and screening of BC.
BMI is closely associated with cancer occurrence. Research has indicated a specific association between BMI and the risk of BC, especially among those with obesity (including high BMI) (4). Nonetheless, the intricacy of this association is impacted by diverse elements, such as menopausal status and specific life stages. For postmenopausal individuals, some meta-analyses have highlighted a positive correlation between elevated obesity, adult weight gain, and the risk of hormone receptor-positive BC, including both estrogen receptor-positive (ER+) and progesterone receptor-positive subtypes (5–7). Conversely, epidemiological evidence suggests an inverse relationship or lack of association between high BMI and the risk of premenopausal hormone receptor-positive (8, 9). Studies (10, 11) have demonstrated that testosterone is converted into estrogen within adipocytes, and the upregulation of aromatase further increases the risk of breast cancer. In adipose tissue and normal breast tissue, aromatase is regulated by different promoters, activated by factors such as IL-6, IL-11, and TNF-α. In postmenopausal women, adipose tissue serves as the primary source of estrogen, and a positive correlation between circulating estradiol levels and BC risk has been confirmed (12, 13).
In recent years, modern “omics” technologies, including metabolomics, have significantly contributed to exploration of disease mechanisms. Metabolites, the end products or intermediates of metabolism, play critical roles in human physiology. By revealing altered metabolic pathways and intermediate metabolites, metabolomics provides novel insights into the biological mechanisms underlying diseases (14, 15).
Nevertheless, there is currently no Mendelian Randomization (MR) evidence to establish a causal relationship between BMI and BC, nor to elucidate the mechanisms by which BMI influences breast cancer risk. Building on prior research into sex hormones and metabolites about BC, we employed mediation analysis to investigate the mediating roles of sex hormones and metabolites in the BMI-BC relationship. Mediation analysis is a statistical approach aimed at identifying intermediate factors that may serve as potential intervention targets, thereby enhancing our understanding of the etiological components involved (16). Therefore, our objective is to investigate the causal connection between BMI and the overall susceptibility to BC, as well as the genetic predisposition to estrogen receptor-positive (ER+) and estrogen receptor-negative (ER-) BC. We hypothesize that the influence of BMI on BC risk factors might be mediated through metabolic or sex hormone pathways.
Given the abundance of information garnered from genome-wide association studies (GWAS) and the application of extensive sample sizes, the MR approach has become a valuable tool. MR utilizes genetic variations, frequently single nucleotide polymorphisms (SNPs), as instrumental variables (IVs) to evaluate causal links between exposures and disease outcomes. Furthermore, owing to the stochastic assortment and recombination of alleles during gametogenesis, genetic variation achieves a form of random allocation within the population, mirroring the principles of traditional randomized controlled trials (17). Consequently, the evidentiary standard of MR studies surpasses that of observational studies (18).
In this investigation, we conducted a two-step MR analysis to elucidate the intermediary pathways linking BMI to BC via metabolites and sex hormones. Building upon these premises, we conducted a two-sample MR and mediation analysis utilizing GWAS databases to comprehensively assess the genetic causal association between BMI and the risk of BC.
This study employed summary data sourced from the Bristol University (IEU) Open GWAS project, which encompasses GWAS summary data extracted from published literature, the UK Biobank, and the FinnGen Biobank. All datasets included in this study are anonymous, de-identified, and publicly available, thus not requiring approval from ethics review boards. To achieve credible causal inference, three assumptions must be satisfied for MR analysis IVs: (1) Instrumental variables necessitate a strong association with the exposure. (2) Instrumental variables must demonstrate independence from any potential confounding factors. (3) Instrumental variables must have no direct relationship with the outcome. Figure 1 illustrates the roadmap of this study.
Figure 1. Flowchart of the study design. In this figure, we present our analytical process. In the left half of this figure, we outline four steps of our analysis: (1) examination of the impact of body mass index (BMI) on breast cancer (Step 1; corresponding table is Table 2); (2) analysis of the influence of BMI on hormones (Step 2; corresponding table is Table 2); (3) investigation of the effect of hormones on breast cancer (Step 3; corresponding table is Table 2); (4) determination of intermediate factors (Step 4; corresponding table is shown in Table 2). In the right half of this figure, we illustrate four steps of our analysis: (1) examination of the impact of BMI on breast cancer (Step 1; corresponding table is Table 2); (2) investigation of the influence of BMI on 249 metabolic features (Step 6; corresponding tables are Supplementary Tables S1 and S3); (3) analysis of the impact of 249 metabolic features on breast cancer (Step 5; corresponding tables are Supplementary Tables S2, S4, and S6); (4) determination of intermediate factors, calculation of the ratio of mediation effect to total effect (Step 7; corresponding tables are shown in Tables 3–5, and Supplementary Tables S3, S5). Additionally, some analyses are not depicted in the figure: we selected metabolic features associated with breast cancer based on false discovery rate (FDR)-adjusted p-values (corresponding tables are Supplementary Tables S7-S9).
SNPs related to exposure factors (BMI) and mediator factors (sex hormones, 249 metabolites) were obtained from the IEU GWAS database project. The BMI stems from two datasets released by the Genetic Investigation of Anthropometric Traits (GIANT) consortium in 2015 and 2013, comprising sample sizes of 171,977 and 73,137, respectively. Notably, the dataset unveiled by the GIANT consortium in 2013 is employed as Supplementary Data in this context. Sex hormones in the mediator factors included Bioavailable testosterone levels, Estradiol levels, Sex hormone-binding globulin levels, and Total testosterone levels. GWAS data for BC were obtained from The Breast Cancer Association Consortium (BCAC), comprising datasets for BC, ER+BC, and ER-BC as outcome variables. Additional information is presented in Table 1.
Following the fundamental assumptions of the MR study, SNPs demonstrating correlations meeting the threshold of P < 5 × 10−8 were designated as instrumental variables following screening of the GWAS data. To mitigate the potential impact of linkage disequilibrium (LD) among SNPs on the analytical outcomes, stringent criteria were applied, including a parameter requirement of r2 < 0.001 and a fixed window size of 10,000 kb (19). To mitigate confounding effects, we excluded SNPs (P<10E-8) associated with the outcome during the analysis. Robust associations between instrumental and endogenous variables were ensured, while minimizing the risk of weak instrumental variable bias, through separate computations for each SNP. These computations encompassed the calculation of R2, indicating the proportion of variance explained by each instrumental variable SNP, alongside the utilization of the F statistic to assess the robustness of instrumental variables (20, 21).
We utilized the Inverse Variance Weighted (IVW) method as the primary means to assess causal effects, recognizing its robustness in identifying causal relationships within two-sample MR analysis (22). Our investigation involved a comparative analysis of outcomes obtained from the IVW method alongside those from the Weighted Median and MR-Egger methods. While the Weighted Median method accommodates up to 50% invalid instrumental variables (IVs), the MR-Egger method allows for the possibility of all IVs being invalid. Hence, when the three models are consistent, it is more convincing. We assessed the heterogeneity of the IVW model using Cochran’s Q test, wherein a significance level below p < 0.05 indicates the presence of heterogeneity. It is important to note, however, that the existence of heterogeneity does not necessarily signify the inefficacy of the IVW model. The MR-Egger method, which permits a non-zero intercept, was employed to identify directional pleiotropy. Additionally, we conducted the leave-one-out analysis to evaluate the impact of excluding individual SNPs on the results. The MR-PRESSO approach serves a dual purpose: it identifies outliers and offers a mechanism for assessing causality. Through this approach, it is possible to observe whether the presence of outliers affects the significance of the results. The IVW method, known for its heightened sensitivity in detecting causality, serves as our primary tool to ascertain the presence of a causal relationship. The False Discovery Rate (FDR) is a widely utilized correction method employed in multiple hypothesis testing. It serves to regulate the proportion of false positives within the pool of significant findings, thus enabling a more permissive analytical approach. All analyses were performed utilizing the TwoSampleMR package (23) in R software. Statistical significance in the analysis results was denoted by q<0.05, with q representing the adjusted p-value.
We conducted a two-step MR analysis to investigate mediation. Firstly, we computed the causal effect of BMI on the mediator (β1) and subsequently estimated the causal effect of the mediator on BC (β2). Z-values falling between -1.96 and 1.96, as per the normal distribution table, are commonly interpreted as within the 95% confidence interval, indicating statistically nonsignificant results. Consequently, the mediation effect was estimated based on β1×β2, with the sign of β1×β2 reflected by the Z-value. The significance of the mediation effect was assessed using the Delta method. This study examined the mediating influence of sex hormones and 249 metabolites on the causal association between BMI and BC. Specifically, the study investigated the role of sex hormones and 249 metabolites as mediators in the causal relationship between BMI and BC.
Table 2 describes the MR results from several approaches to analyzing the causal effect of BMI on BC. The IVW results indicate significant negative causal relationships between BMI and BC, ER+ BC, and ER- BC in two-sample MR analyses. The effect estimates for BMI on BC, ER+ BC, and ER- BC were -0.184, -0.183, and -0.349, respectively, with corresponding q-values of 3.32E-03, 4.28E-03, and 1.03E-03.
We initially analyzed the impact of female BMI on BC. Considering sex hormones as potential mediators, we subsequently examined the effects of BMI on sex hormones and their subsequent influence on BC. We used a two-step MR analysis for mediated MR analysis. In the initial stage, we computed the causal effect of BMI on the mediator (β1), while in the subsequent step, we determined the causal effect of the mediator on BC (β2). Ultimately, the mediation effect can be estimated based on β1×β2, with the sign of β1×β2 reflected by the Z-value.
As shown in Table 2, in the two-sample MR analysis of sex hormones and BC, IVW results show a significant positive causal relationship between Bioavailable testosterone, Total testosterone levels, and BC (effect estimates are 0.145 and 0.125, respectively); in the analysis of ER+ BC, Bioavailable testosterone, Total testosterone levels, and Estradiol levels show a positive causal relationship with ER+ BC (with effect estimates of 0.201, 0.399, and 0.172, respectively). However, in the two-sample MR analysis investigating the association between sex hormones and ER-BC, no statistically significant causal relationship between the two variables was observed.
A two-step methodology was utilized to conduct mediation MR analysis. Subsequent to completing the causal MR analyses assessing the influence of BMI on BC and sex hormones on BC, an investigation was initiated to examine whether the causal link between BMI and BC could be mediated through sex hormones (e.g., Bioavailable testosterone levels). It is noteworthy that during our analysis of the effect of BMI (using the dataset released by the GIANT consortium in 2015) on bioavailable testosterone levels, horizontal pleiotropy was detected. Therefore, we utilized BMI data from different years (using the dataset released by the GIANT consortium in 2013) as an alternative. The results, as presented in Table 2, indicate that BMI increases the risk of ER+BC through bioavailable testosterone, with a mediation effect estimate (β1×β2) of 0.025.
From Table 2, it can be observed that increasing BMI is associated with increased levels of bioavailable testosterone and decreased levels of sex hormone-binding globulin (SHBG), while it does not affect estradiol levels and total testosterone levels. Additionally, elevated levels of estrogen and testosterone are correlated with an increased risk of ER+ BC, while showing no association with the risk of ER-BC
In the preliminary analysis, the 249 metabolites were classified into nine main categories, comprising amino acids, low molecular weight metabolites, phospholipids, triglycerides, total lipids, (un)saturated fatty acids, cholesterol esters, free cholesterol, and lipoproteins. Among these metabolites, BMI exhibited a significant causal relationship with 183 metabolites (p < 0.05). Supplementary Tables S2, S4, and S6 respectively present the MR results of 249 metabolites on BC, ER+ BC, and ER- BC. Supplementary Figure S1 illustrates the visual results of MR analysis of BMI on 249 metabolites and 249 metabolites on BC, ER+ BC, and ER- BC. The final analysis results consistently indicate negative mediation effect estimates (β1×β2).
Supplementary Table S2 presents the results of the MR analysis investigating the influence of 249 metabolic traits on BC risk, identified by the BCAC consortium. The two-sample MR outcomes reveal that 79 metabolic traits demonstrate a causal effect on BC. Through screening potential metabolic traits affecting BC based on FDR-adjusted q-values (excluding those with horizontal pleiotropy), Supplementary Table S3 highlights the selected candidates. After FDR correction, we identified 38 metabolic traits with q-values below 0.05 as mediator factors between BMI and BC, as depicted in Supplementary Table S7. Further data processing involved excluding mediators with horizontal pleiotropy and those with q-values below 0.05 in the relationship between BMI and BC. The final analysis results, presented in Table 3, unveil 35 metabolic traits mediating the negative causal association between BMI and BC. These traits predominantly encompass triglyceride levels (especially in VLDL), various cholesterol forms (notably HDL), concentrations of four lipoproteins, three phospholipids, and two total lipids. Figure 2 provides a visual representation of the MR analysis, illustrating the mediation of 35 metabolic traits between BMI and BC causality.
Table 3. The mediators between body mass index and breast cancer were screened according to their P-value (p<0.05) after the FDR corrected (mediators with horizontal pleiotropy were excluded).
Figure 2. The mediator of 35 metabolic traits between BMI and BC causality. We visualized that 35 metabolic traits mediate the effect of BMI on breast cancer. The outermost boundary of the circular heat map consists of 35 metabolic traits and their corresponding identifiers. From the outermost to the innermost rings, the first and second rings of the circular heat map respectively depict the beta values of BMI on a particular metabolic trait and the beta values of the same trait on breast cancer (IVW method), while the third and fourth rings represent the q-values of these two aforementioned effects (IVW method).
Additional MR analysis was performed to investigate metabolic characteristics that mediate the association between BMI and ER+BC. Supplementary Table S4 presents the MR analysis results of the impact of 249 metabolic traits on ER+BC. Preliminary analysis identified 75 metabolic traits exhibiting a causal effect on ER+ BC. Metabolic traits influencing ER+BC were selected based on FDR-adjusted p-values, resulting in the identification of 37 metabolic traits (Supplementary Table S5). Following FDR correction, mediator factors between BMI and ER+BC were identified, leading to the discovery of 33 metabolic traits with q-values below 0.05 (Supplementary Table S8). After excluding mediators with horizontal pleiotropy and those with q-values below 0.05 between BMI and ER+BC, the final results are shown in Table 4. A total of 33 metabolic traits mediate the causal relationship between BMI and ER+BC, predominantly involving various forms of cholesterol (particularly significant in HDL), triglyceride levels, concentrations of four lipoproteins/lipoproteins, and three phospholipids. Figure 3 illustrates the mediation of 33 metabolic traits between BMI and ER+BC causality.
Table 4. The mediators between body mass index and ER+ breast cancer were screened according to their P-value (p<0.05) after the FDR corrected (mediators with horizontal pleiotropy were excluded).
Figure 3. The mediator of 33 metabolic traits between BMI and ER+BC causality. We visualized that 33 metabolic traits mediate the effect of BMI on ER+breast cancer. The outermost boundary of the circular heat map consists of 33 metabolic traits and their corresponding identifiers. From the outermost to the innermost rings, the first and second rings of the circular heat map respectively depict the beta values of BMI on a particular metabolic trait and the beta values of the same trait on ER+ breast cancer (IVW method), while the third and fourth rings represent the q-values of these two aforementioned effects (IVW method).
Similarly, MR analysis was performed to explore metabolic traits mediating the relationship between BMI and ER-BC. The Supplementary Table S6 presents the MR analysis results of the impact of 249 metabolic traits on ER-BC. Mediators between BMI and ER-BC were screened based on their P-values before FDR correction (Supplementary Table S9). Following FDR correction, no statistically significant results were observed. Therefore, potential mediators were screened using uncorrected p-values. The final results, presented in Table 5, reveal 15 metabolic traits mediating the causal relationship between BMI and ER- BC, mainly involving various forms of cholesterol (especially in HDL), triglyceride levels, concentrations of large HDL particles, and large HDL particle concentrations. Additionally, glycine, two unsaturated fatty acids (docosahexaenoic acid and monounsaturated fatty acid), and the r relative proportion of polyunsaturated fatty acids to monounsaturated fatty acids also mediate the causal association between BMI and ER-BC. Figure 4 illustrates the mediation of 15 metabolic traits between BMI and ER-BC causality.
Table 5. The mediators between body mass index and ER- breast cancer were screened according to their P-value (p<0.05) before FDR corrected.
Figure 4. The mediator of 15 metabolic traits between BMI and ER-BC causality. We visualized that 15 metabolic traits mediate the effect of BMI on ER-breast cancer. The outermost boundary of the circular heat map consists of 15 metabolic traits and their corresponding identifiers. From the outermost to the innermost rings, the first and second rings of the circular heat map respectively depict the beta values of BMI on a particular metabolic trait and the beta values of the same trait on ER- breast cancer (IVW method), while the third and fourth rings represent the q-values of these two aforementioned effects (IVW method).
It is noteworthy to mention that in the MR analysis of BC, ER+BC, and ER-BC, the causal relationship between BMI and metabolites is not consistent. For example, in the causal analysis and mediation analysis of BMI and BC, BMI has a negative causal relationship with most cholesterol in HDL but a positive causal relationship with cholesterol in VLDL and triglyceride levels; correspondingly, cholesterol in HDL is positively associated with BC risk, while cholesterol in VLDL and triglyceride levels are negatively associated with BC in MR analysis results. Therefore, BMI has an effect on metabolites, but it is not simply an increase or decrease; it has different effects on metabolites in different lipoproteins (HDL, IDL, VLDL, etc.). Similarly, the effects of metabolites in different lipoproteins (HDL, IDL, VLDL, etc.) on BC are also different.
Previous studies exploring the correlation between BMI and the risk of developing BC using observational research methodologies may be prone to inherent limitations. Our Mendelian randomization study indicates that genetically predicted BMI is linked to a heightened/decreased risk of BC. Furthermore, sex hormones and various metabolites assume significant mediating roles in the causal pathway from BMI to B. We provide causal evidence demonstrating that BMI increases ER+BC risk through bioavailable testosterone levels, while it decreases BC risk through multiple metabolite pathways (mostly various forms of cholesterol, triglyceride levels, etc.). However, this study was conducted at the genetic level, so these results should be interpreted with caution.
In previous studies, significant associations between BMI and several cancers, including colorectal cancer, esophageal cancer, liver cancer, gallbladder cancer, pancreatic cancer, etc., have been observed, showing a positive dose-response relationship (24). However, the impact of BMI on BC is not uniformly established (24). For instance, high BMI during childhood, adolescence, and early adulthood is linked to a diminished risk of premenopausal BC (25–27). Conversely, several investigations have identified an association between adult BMI and postmenopausal BC, particularly in cases of estrogen receptor-positive tumors. Notably, a consistent negative correlation has been observed regarding premenopausal BC (24, 28). High BMI is a well-known risk factor for postmenopausal women with ER+BC. This aligns with our study findings (28–30). We found that BMI appears to affect BC risk through two different pathways. In the sex hormone pathway, we found that elevated BMI is positively associated with increased bioavailable testosterone levels, which in turn increases the risk of ER+ BC. In contrast, in the metabolite pathway study, multiple metabolites mediate the negative causal relationship between BMI and BC, regardless of ER status. Thus, we speculate that premenopausal BMI primarily reduces BC risk through the metabolite pathway, while postmenopausal BMI increases ER+BC risk through the sex hormone pathway. However, this requires further validation through more literature and research.
Our study found that increased BMI is closely associated with elevated levels of bioavailable testosterone and reduced levels of SHBG. Furthermore, higher levels of both estrogen and testosterone have been associated with an increased risk of ER+ BC. These findings are consistent with previous studies (31, 32). A prospective study in premenopausal women demonstrated that elevated total and free estradiol levels, along with higher plasma total and free testosterone levels, were linked to an increased risk of BC (33). These associations appeared to be stronger in women with invasive BC or ER+/PR+ tumors and were independent of other known breast cancer risk factors. ER+BC is one of the more common subtypes and is highly dependent on estrogen. The potential mechanisms connecting testosterone to ER+ breast cancer likely involve its conversion to estradiol (34). Testosterone can be aromatized into estrogen, and estradiol binds to ER, inducing the transcription of growth-promoting genes while downregulating negative growth regulators, ultimately enhancing breast cancer cell proliferation (35). The interaction between high BMI and ER+BC can be partially explained by increased estrogen biosynthesis in adipose tissue (36, 37)and the creation of a pro-tumor environment through the release of various cytokines (38), induction of hypoxia-inducible factor changes (39), and triggering inflammation (40). Additionally, high BMI may increase the sensitivity of breast cells to insulin, leading to excessive production of insulin antibodies, resulting in a high insulin state, ultimately leading to lipid metabolism disorders (41).
The increase in BMI correlates with alterations in the levels of certain metabolites, as high BMI can cause an increase in adipose tissue, leading to metabolic abnormalities. Our study results show that BMI is negatively associated with multiple forms of cholesterol, while in the causality analysis between cholesterol and BC, high cholesterol is linked to a heightened risk of BC. There is evidence to suggest that hypercholesterolemia is considered an autonomous risk factor for postmenopausal women with BC (42). In a recent study, Wen Liu, Binita Chakraborty, et al. (43), found that prolonged exposure to 27-hydroxycholesterol (a major metabolite of cholesterol) selects cells that survive with increased cell uptake and/or lipid biosynthesis. These cells overexpress the iron death negative regulatory factor GPX4, demonstrating stronger tumorigenic and metastatic capabilities. Moreover, several studies (44, 45) have shown that 27-hydroxycholesterol can act as a true endogenous selective estrogen receptor modulator (SERM), thereby promoting the growth of ER-positive BC in luminal BC models. Therefore, high BMI reduces the generation of various forms of cholesterol, and the reduction in cholesterol generation weakens its relationship with the risk of BC. Among the various triglyceride-related metabolites, BMI shows a positive correlation with them, and correspondingly, these metabolites are linked to a diminished risk of BC, although the mechanism behind this is currently unclear. Overall, the relationship between BMI and metabolites is complex and diverse, and different metabolites may have different effects on the risk of BC (46).
Our study results provide a new perspective on early screening and prevention of BC, for populations with high BMI, different prevention strategies can be formulated based on ER status and menopausal status. Postmenopausal women with severe obesity may need to consider the potential risk of BC and take timely intervention measures. For individuals with a high BMI, the decision to pursue weight reduction should be based on the levels of monitored metabolites, such as triglycerides and cholesterol, in order to reduce the risk of breast cancer. Regular lipid monitoring is recommended for individuals with metabolic abnormalities, and appropriate pharmacological treatment may be considered for those with hypercholesterolemia. Multiple large studies (47, 48) have shown that the use of statins (a class of drugs that lower cholesterol) before or after BC diagnosis has significant benefits for overall survival and disease-free survival.
Our study has several strengths. Firstly, the structure of the MR model safeguards against the impact of confounding variables, thereby securing dependable estimations of causal effects derived from observational investigations (49). Additionally, the MR model utilizes extensive-sample Genome-Wide Association Studies (GWAS) datasets, substantially enhancing the analytical efficacy in comparison to small-sample models relying on individual-level data (50). To guarantee the accuracy of the MR analysis, we executed a thorough investigation into pleiotropy. Additionally, we utilized exposure and outcome data sourced from diverse European populations across multiple countries to mitigate potential biases.
Undoubtedly, this study is subject to certain limitations. Firstly, due to the lack of aggregated GWAS data for pre-and postmenopausal women, we were unable to stratify the BC population based on menopausal status. As a result, we analyzed pre-and postmenopausal women as a single group. Given the differences in sex hormone levels between pre- and postmenopausal women, further subgroup analyses are needed to clarify the specific populations in which BMI influences BC risk through bioavailable testosterone. Secondly, given that the data exclusively originate from individuals of European descent, the applicability of the results to other racial or ethnic groups may be limited. Finally, owing to incomplete GWAS data regarding specific exposures, we refrained from conducting reverse MR analysis. Thus, completing bidirectional MR analysis becomes imperative upon meeting future data prerequisites.
In this study, MR analysis revealed that higher BMI decreases the risk of BC, regardless of ER+ or ER- status. Additionally, BMI may increase the likelihood of ER+BC through the pathway of sex hormones (bioavailable testosterone) and decrease the risk of BC through various metabolic pathways. These findings are of significant importance for developing preventive strategies and interventions targeting BC.
Publicly available datasets were analyzed in this study. All GWAS data used in this study are available in the IEU open GWAS project (https://gwas.mrcieu.ac.uk/).
This study used summary-level statistics from published studies and publicly available GWASs. No ethical approval was required for this study.
YY: Formal analysis, Writing – original draft. MC: Conceptualization, Formal analysis, Methodology, Writing – original draft. WY: Formal analysis, Conceptualization, Data curation, Methodology, Supervision, Visualization, Writing – review & editing.
The author(s) declare that no financial support was received for the research, authorship, and/or publication of this article.
Special thanks to the GIANT consortium, the UK Biobank, the BCAC consortium, and the IEU open GWAS project developed by The MRC Integrative Epidemiology Unit (IEU) at the University of Bristol.
The authors declare that the research was conducted in the absence of any commercial or financial relationships that could be construed as a potential conflict of interest.
All claims expressed in this article are solely those of the authors and do not necessarily represent those of their affiliated organizations, or those of the publisher, the editors and the reviewers. Any product that may be evaluated in this article, or claim that may be made by its manufacturer, is not guaranteed or endorsed by the publisher.
The Supplementary Material for this article can be found online at: https://www.frontiersin.org/articles/10.3389/fonc.2024.1449956/full#supplementary-material
1. Akram M, Iqbal M, Daniyal M, Khan AU. Awareness and current knowledge of breast cancer. Biol Res. (2017) 50:33. doi: 10.1186/s40659-017-0140-9
2. Siegel RL, Miller KD, Wagle NS, Jemal A. Cancer statistics, 2023. CA Cancer J Clin. (2023) 73:17–48. doi: 10.3322/caac.21763
3. Sun YS, Zhao Z, Yang ZN, Xu F, Lu HJ, Zhu ZY, et al. Risk factors and preventions of breast cancer. Int J Biol Sci. (2017) 13:1387–97. doi: 10.7150/ijbs.21635
4. Agurs-Collins T, Ross SA, Dunn BK. The many faces of obesity and its influence on breast cancer risk. Front Oncol. (2019) 9:765. doi: 10.3389/fonc.2019.00765
5. Xia X, Chen W, Li J, Chen X, Rui R, Liu C, et al. Body mass index and risk of breast cancer: a nonlinear dose-response meta-analysis of prospective studies. Sci Rep. (2014) 4:7480. doi: 10.1038/srep07480
6. Liu K, Zhang W, Dai Z, Wang M, Tian T, Liu X, et al. Association between body mass index and breast cancer risk: evidence based on a dose-response meta-analysis. Cancer Manag Res. (2018) 10:143–51. doi: 10.2147/CMAR.S144619
7. Afshin A, Reitsma MB, Murray CJL. Health effects of overweight and obesity in 195 countries. N Engl J Med. (2017) 377:1496–7. doi: 10.1056/NEJMc1710026
8. Chen Y, Liu L, Zhou Q, Imam MU, Cai J, Wang Y, et al. Body mass index had different effects on premenopausal and postmenopausal breast cancer risks: a dose-response meta-analysis with 3,318,796 subjects from 31 cohort studies. BMC Public Health. (2017) 17:936. doi: 10.1186/s12889-017-4953-9
9. Suzuki R, Rylander-Rudqvist T, Ye W, Saji S, Wolk A. Body weight and postmenopausal breast cancer risk defined by estrogen and progesterone receptor status among Swedish women: A prospective cohort study. Int J Cancer. (2006) 119:1683–9. doi: 10.1002/ijc.22034
10. Simpson ER. Sources of estrogen and their importance. J Steroid Biochem Mol Biol. (2003) 86:225–30. doi: 10.1016/s0960-0760(03)00360-1
11. Bulun SE, Price TM, Aitken J, Mahendroo MS, Simpson ER. A link between breast cancer and local estrogen biosynthesis suggested by quantification of breast adipose tissue aromatase cytochrome P450 transcripts using competitive polymerase chain reaction after reverse transcription. J Clin Endocrinol Metab. (1993) 77:1622–8. doi: 10.1210/jcem.77.6.8117355
12. Missmer SA, Eliassen AH, Barbieri RL, Hankinson SE. Endogenous estrogen, androgen, and progesterone concentrations and breast cancer risk among postmenopausal women. J Natl Cancer Inst. (2004) 96:1856–65. doi: 10.1093/jnci/djh336
13. Key T, Appleby P, Barnes I, Reeves G. Endogenous sex hormones and breast cancer in postmenopausal women: reanalysis of nine prospective studies. J Natl Cancer Inst. (2002) 94:606–16. doi: 10.1093/jnci/94.8.606
14. Tu J, Wen J, Luo Q, Li X, Wang D, Ye J. Causal relationships of metabolites with allergic diseases: a trans-ethnic Mendelian randomization study. Respir Res. (2024) 25:94. doi: 10.1186/s12931-024-02720-6
15. Xiao G, He Q, Liu L, Zhang T, Zhou M, Li X, et al. Causality of genetically determined metabolites on anxiety disorders: a two-sample Mendelian randomization study. J Transl Med. (2022) 20:475. doi: 10.1186/s12967-022-03691-2
16. Kiyokawa S, Kobayashi K, Kikuchi Y, Kamada H, Harada H. Root-inducing region of mikimopine type Ri plasmid pRi1724. Plant Physiol. (1994) 104:801–2. doi: 10.1104/pp.104.2.801
17. Lawlor DA, Harbord RM, Sterne JA, Timpson N, Davey Smith G. Mendelian randomization: using genes as instruments for making causal inferences in epidemiology. Stat Med. (2008) 27:1133–63. doi: 10.1002/sim.3034
18. Jin T, Huang W, Cao F, Yu X, Guo S, Ying Z, et al. Causal association between systemic lupus erythematosus and the risk of dementia: A Mendelian randomization study. Front Immunol. (2022) 13:1063110. doi: 10.3389/fimmu.2022.1063110
19. Meng L, Wang Z, Ming YC, Shen L, Ji HF. Are micronutrient levels and supplements causally associated with the risk of Alzheimer’s disease? A two-sample Mendelian randomization analysis. Food Funct. (2022) 13:6665–73. doi: 10.1039/d1fo03574f
20. Burgess S, Thompson SG, Collaboration CCG. Avoiding bias from weak instruments in Mendelian randomization studies. Int J Epidemiol. (2011) 40:755–64. doi: 10.1093/ije/dyr036
21. Sanderson E, Spiller W, Bowden J. Testing and correcting for weak and pleiotropic instruments in two-sample multivariable Mendelian randomization. Stat Med. (2021) 40:5434–52. doi: 10.1002/sim.9133
22. Hartwig FP, Davey Smith G, Bowden J. Robust inference in summary data Mendelian randomization via the zero modal pleiotropy assumption. Int J Epidemiol. (2017) 46:1985–98. doi: 10.1093/ije/dyx102
23. Hemani G, Zheng J, Elsworth B, Wade KH, Haberland V, Baird D, et al. The MR-Base platform supports systematic causal inference across the human phenome. Elife. (2018) 7. doi: 10.7554/eLife.34408
24. Lauby-Secretan B, Scoccianti C, Loomis D, Grosse Y, Bianchini F, Straif K, et al. Body fatness and cancer–viewpoint of the IARC working group. N Engl J Med. (2016) 375:794–8. doi: 10.1056/NEJMsr1606602
25. Premenopausal Breast Cancer Collaborative, G, Schoemaker MJ, Nichols HB, Wright LB, Brook MN, Jones ME, et al. Association of body mass index and age with subsequent breast cancer risk in premenopausal women. JAMA Oncol. (2018) 4:e181771. doi: 10.1001/jamaoncol.2018.1771
26. Baer HJ, Tworoger SS, Hankinson SE, Willett WC. Body fatness at young ages and risk of breast cancer throughout life. Am J Epidemiol. (2010) 171:1183–94. doi: 10.1093/aje/kwq045
27. Horn-Ross PL, Canchola AJ, Bernstein L, Neuhausen SL, Nelson DO, Reynolds P. Lifetime body size and estrogen-receptor-positive breast cancer risk in the California Teachers Study cohort. Breast Cancer Res. (2016) 18:132. doi: 10.1186/s13058-016-0790-5
28. Renehan AG, Tyson M, Egger M, Heller RF, Zwahlen M. Body-mass index and incidence of cancer: a systematic review and meta-analysis of prospective observational studies. Lancet. (2008) 371:569–78. doi: 10.1016/S0140-6736(08)60269-X
29. Picon-Ruiz M, Morata-Tarifa C, Valle-Goffin JJ, Friedman ER, Slingerland JM. Obesity and adverse breast cancer risk and outcome: Mechanistic insights and strategies for intervention. CA Cancer J Clin. (2017) 67:378–97. doi: 10.3322/caac.21405
30. Rosenberg LU, Morata-Tarifa C, Valle-Goffin JJ, Friedman ER, Slingerland JM. Risk factors for hormone receptor-defined breast cancer in postmenopausal women. Cancer Epidemiol Biomarkers Prev. (2006) 15:2482–8. doi: 10.1158/1055-9965.EPI-06-0489
31. Albers FEM, Lou MWC, Dashti SG, Swain CTV, Rinaldi S, Viallon V, et al. Sex-steroid hormones and risk of postmenopausal estrogen receptor-positive breast cancer: a case-cohort analysis. Cancer Causes Control. (2024) 35:921–33. doi: 10.1007/s10552-024-01856-6
32. Sieri S, Krogh V, Bolelli G, Abagnato CA, Grioni S, Pala V, et al. Sex hormone levels, breast cancer risk, and cancer receptor status in postmenopausal women: the ORDET cohort. Cancer Epidemiol Biomarkers Prev. (2009) 18:169–76. doi: 10.1158/1055-9965.EPI-08-0808
33. Eliassen AH, Missmer SA, Tworoger SS, Spiegelman D, Barbieri RL, Dowsett M, et al. Endogenous steroid hormone concentrations and risk of breast cancer among premenopausal women. J Natl Cancer Inst. (2006) 98:1406–15. doi: 10.1093/jnci/djj376
34. Simpson ER. Aromatization of androgens in women: current concepts and findings. Fertil Steril. (2002) 77 Suppl:4, S6–10. doi: 10.1016/s0015-0282(02)02984-9
35. Frasor J, Danes JM, Komm B, Chang KC, Lyttle CR, Katzenellenbogen BS. Profiling of estrogen up- and down-regulated gene expression in human breast cancer cells: insights into gene networks and pathways underlying estrogenic control of proliferation and cell phenotype. Endocrinology. (2003) 144:4562–74. doi: 10.1210/en.2003-0567
36. Key TJ, Appleby PN, Reeves GK, Travis RC, Brinton LA, Helzlsouer KJ, et al. Steroid hormone measurements from different types of assays in relation to body mass index and breast cancer risk in postmenopausal women: Reanalysis of eighteen prospective studies. Steroids. (2015) 99:49–55. doi: 10.1016/j.steroids.2014.09.001
37. Liedtke S, Schmidt ME, Vrieling A, Lukanova A, Becker S, Kaaks R, et al. Postmenopausal sex hormones in relation to body fat distribution. Obes (Silver Spring). (2012) 20:1088–95. doi: 10.1038/oby.2011.383
38. Gilbert CA, Slingerland JM. Cytokines, obesity, and cancer: new insights on mechanisms linking obesity to cancer risk and progression. Annu Rev Med. (2013) 64:45–57. doi: 10.1146/annurev-med-121211-091527
39. Trayhurn P. Hypoxia and adipose tissue function and dysfunction in obesity. Physiol Rev. (2013) 93:1–21. doi: 10.1152/physrev.00017.2012
40. Tornatore L, Thotakura AK, Bennett J, Moretti M, Franzoso G. The nuclear factor kappa B signaling pathway: integrating metabolism with inflammation. Trends Cell Biol. (2012) 22:557–66. doi: 10.1016/j.tcb.2012.08.001
41. Capasso I, Esposito E, Pentimalli F, Montella M, Crispo A, Maurea N, et al. Homeostasis model assessment to detect insulin resistance and identify patients at high risk of breast cancer development: National Cancer Institute of Naples experience. J Exp Clin Cancer Res. (2013) 32:14. doi: 10.1186/1756-9966-32-14
42. Danilo C, Frank PG. Cholesterol and breast cancer development. Curr Opin Pharmacol. (2012) 12:677–82. doi: 10.1016/j.coph.2012.07.009
43. Liu W, Chakraborty B, Safi R, Kazmin D, Chang CY, McDonnell DP. Dysregulated cholesterol homeostasis results in resistance to ferroptosis increasing tumorigenicity and metastasis in cancer. Nat Commun. (2021) 12:5103. doi: 10.1038/s41467-021-25354-4
44. Wu Q, Ishikawa T, Sirianni R, Tang H, McDonald JG, Yuhanna IS, et al. 27-Hydroxycholesterol promotes cell-autonomous, ER-positive breast cancer growth. Cell Rep. (2013) 5:637–45. doi: 10.1016/j.celrep.2013.10.006
45. Nelson ER, Wardell SE, Jasper JS, Park S, Suchindran S, Howe MK, et al. 27-Hydroxycholesterol links hypercholesterolemia and breast cancer pathophysiology. Science. (2013) 342:1094–8. doi: 10.1126/science.1241908
46. Ferraro GB, Ali A, Luengo A, Kodack DP, Deik A, Abbott KL, et al. Fatty acid synthesis is required for breast cancer brain metastasis. Nat Cancer. (2021) 2:414–28. doi: 10.1038/s43018-021-00183-y
47. Borgquist S, Broberg P, Tojjar J, Olsson H. Statin use and breast cancer survival - a Swedish nationwide study. BMC Cancer. (2019) 19:54. doi: 10.1186/s12885-018-5263-z
48. Ahern TP, Pedersen L, Tarp M, Cronin-Fenton DP, Garne JP, Silliman RA, et al. Statin prescriptions and breast cancer recurrence risk: a Danish nationwide prospective cohort study. J Natl Cancer Inst. (2011) 103:1461–8. doi: 10.1093/jnci/djr291
49. Emdin CA, Khera AV, Kathiresan S. Mendelian randomization. JAMA. (2017) 318:1925–6. doi: 10.1001/jama.2017.17219
Keywords: breast cancer, body mass index, sex hormones, metabolites, Mendelian randomization
Citation: Yang Y, Chen M and Yang W (2024) Mediation effects of metabolites and sex hormones on the relationship between body mass index and breast cancer: Mendelian randomization analysis and mediation analysis. Front. Oncol. 14:1449956. doi: 10.3389/fonc.2024.1449956
Received: 16 June 2024; Accepted: 28 October 2024;
Published: 21 November 2024.
Edited by:
Ruby Dhar, All India Institute of Medical Sciences, IndiaReviewed by:
Marcos Edgar Herkenhoff, University of São Paulo, BrazilCopyright © 2024 Yang, Chen and Yang. This is an open-access article distributed under the terms of the Creative Commons Attribution License (CC BY). The use, distribution or reproduction in other forums is permitted, provided the original author(s) and the copyright owner(s) are credited and that the original publication in this journal is cited, in accordance with accepted academic practice. No use, distribution or reproduction is permitted which does not comply with these terms.
*Correspondence: Wenwen Yang, MTI0MzAxMjI1NkBxcS5jb20=; Min Chen, bWF5bWluY2hlbkAxNjMuY29t
Disclaimer: All claims expressed in this article are solely those of the authors and do not necessarily represent those of their affiliated organizations, or those of the publisher, the editors and the reviewers. Any product that may be evaluated in this article or claim that may be made by its manufacturer is not guaranteed or endorsed by the publisher.
Research integrity at Frontiers
Learn more about the work of our research integrity team to safeguard the quality of each article we publish.