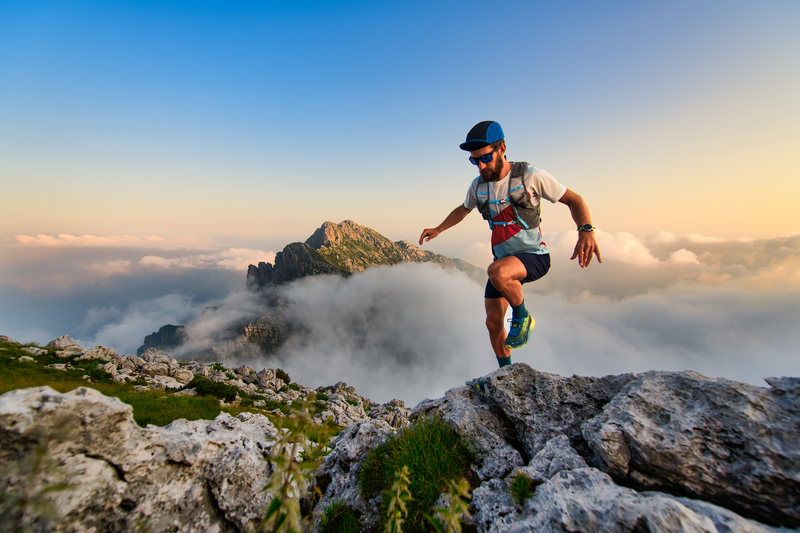
95% of researchers rate our articles as excellent or good
Learn more about the work of our research integrity team to safeguard the quality of each article we publish.
Find out more
REVIEW article
Front. Oncol. , 31 October 2024
Sec. Hematologic Malignancies
Volume 14 - 2024 | https://doi.org/10.3389/fonc.2024.1447001
This article is part of the Research Topic MDS: New Scientific and Clinical Developments View all 14 articles
The unique heterogenous landscape of myelodysplastic syndromes/neoplasms (MDS) has resulted in continuous redefinition of disease sub-entities, in view of the novel translational research data that have clarified several areas of the pathogenesis and the progression of the disease. The new international classifications (WHO 2022, ICC 2022) have incorporated genomic data defining phenotypical alterations, that guide clinical management of specific patient subgroups. On the other hand, for over a decade, multiparameter flow cytometry (MFC) has proven its value as a complementary diagnostic tool for these diseases and although it has never been established as a mandatory test for the baseline evaluation of MDS patients in international guidelines, it is almost universally adopted in everyday clinical practice for the assessment of suspected cytopenias through simplified scoring systems or elaborate analytical strategies for the detection of immunophenotypical dysplastic features in every hematopoietic cell lineage in the bone marrow (BM). In this review, we explore the clinically meaningful interplay of MFC data and genetic profiles of MDS patients, to reveal the currently existing and the potential future role of each methodology for routine clinical practice, and the benefit of the patients. We reviewed the existing knowledge and recent advances in the field and discuss how an integrated approach could lead to patient re-stratification and guide personalized management.
Myelodysplastic syndromes (MDS) or Myelodysplastic Neoplasms are clonal hematopoietic stem cell disorders, characterized by hierarchical somatic mutations establishing ineffective hematopoiesis and by an increased tendency of evolution or transformation to Acute Myelogenous Leukemia (AML) (1–3). From the clinical and hematological point of view, MDS display substantial heterogeneity and a great spectrum of clinical manifestations, depending on the degree of ineffective hematopoiesis/BM failure, the dysregulation of the immune system, the consequences of iron overload and very rarely on potential extramedullary disease infiltration (4–8). The classification of MDS is continuously evolving, as a result of emerging information on the biology and molecular pathogenesis of these diseases, and its prognostic relevance (9–13). The application of the appropriate diagnostic approach and the identification of the patient’s prognostic profile requires the incorporation and evaluation of many clinical and biological parameters of the disease. Cytomorphology has been traditionally and still remains the cornerstone of diagnosis since the initial description of MDS, but cytogenetics and more recently molecular genetics have become an irreplaceable part of the diagnosis, classification and prognostication of these diseases and have been incorporated in several new classification and prognostication systems.
MFC is a useful tool for hematologists, supporting the diagnosis, classification, staging, follow up and the estimation and quantification of measurable clonal residual disease (MRD) in several hematological malignancies (14, 15), such as chronic lymphocytic leukemia (CLL), other mature lymphoproliferative neoplasms, particularly of B-cell origin, as well as all types of acute leukemias (AML and acute lymphoblastic leukemia: ALL) (16–24). The identification of abnormal cellular populations in the peripheral blood, BM or tissue fluids, either at diagnosis or relapse, constitutes a routine and straightforward analysis and highlights the role of MFC in everyday clinical practice for the diagnosis, prognosis and management of the afore-mentioned diseases. However, that is not the case for MDS.
Immunophenotyping dysplastic cells by MFC has become a useful diagnostic tool for MDS mainly the last 15-20 years, and although it can provide a rapid evaluation of dysplastic hematopoiesis in the BM, it has never been considered as a mandatory test for the establishment of diagnosis and/or the evaluation of prognosis for this group of diseases. The description of dyserythropoiesis or dysmyelopoieis in the BM by MFC, in a clinically meaningful way, has been the subject of investigation for more than 20 years and tremendous progress has been reported (25–28). Detection and quantification of the immunophenotypic features of dysplasia has been the endpoint of thorough investigation by several scientific groups, which, on top of reporting their findings, in several occasions have also proposed guidelines for MFC analysis or they have developed various diagnostic and prognostic immunophenotypic scores (29–32). In general, the analytical process and interpretation of the results is clearly easier for patients with excess of BM blasts, and more cumbersome for patients with low blast counts. Most recent recommendations addressing analytical issues for the evaluation of MDS were published in 2023 by European LeukemiaNet (ELN)/international MDS flow cytometry working group (iMDSFlow) (28) and are summarized in Table 1.
Table 1. ELN/international MDS flow cytometry working group 2023 recommendations for the assessment of suspected MDS bone marrow samples.
At the same time the substantial progress in the molecular and pathogenic characterization of the disease has allowed to better understand and to precisely classify and prognostically categorize individual patients, while offering a chance for tailored treatment (33–36). The most recent World Health Organization (WHO) and international consensus classification (ICC) classification systems recognize certain MDS entities exclusively by their molecular background as depicted in Table 2.
Consequently, the major questions regarding the role of MFC in MDS remain: first, are there any individual cases, for which MFC analysis is necessary to establish the diagnosis? and what immunophenotypic findings are the most relevant in each scenario? Second, does MFC analysis hold any independent prognostic added value, regarding the clinical course of the patients? And lastly, can we define the clinical setting, in which MFC findings will affect drastically the management of patients?
In this review, we have explored the relationship between cellular immunophenotypic, molecular and cytogenetic features that have prognostic importance, distinct clinical presentations and outcomes in MDS patients. We have specified the disease sub-entities or sub-groups for which MFC analysis might be more useful and potentially mandatory for the confirmation of the diagnosis. Moreover, we have reviewed areas of diagnosis, treatment evaluation, and prognosis in which MFC analysis contributes to the correct decision-making and analyzed current limitations and future perspectives.
To achieve the above-mentioned aims of this review, a narrative PubMed/Medline review on MDS, flow cytometry and molecular findings with diagnostic or prognostic importance was performed for articles published from January 1980 until March 2024. We searched the Medline database for articles published in English using the search term ‘myelodysplastic syndromes’ adding each of the following keywords: ‘flow cytometry’, ‘immunophenotype’, ‘diagnostic score’, ‘scoring system’, ‘classification’, ‘molecular’, ‘genetics’, ‘karyotype’, ‘prognosis’, ‘diagnosis’, ‘clinical’, ‘erythroid dysplasia’, ‘erythropoiesis’, ‘monocytes’, ‘megakaryocytes’, ‘granulocytes’, ‘immature cells’, ‘peripheral blood’, ‘bone marrow’, ‘algorithm’. Articles were also obtained via cross-reference checking.
Historically, morphology had been the cornerstone of the diagnosis of MDS and the past decades cytogenetics provided new insights into the biology and clinical course of the disease, associating genotype with phenotype. Clonal cytogenetic abnormalities are detected in 40-50% of patients with primary MDS and in 80-95% of patients with secondary MDS (37, 38).
The detection of clonal chromosomal abnormality is a significant diagnostic finding proving the existence of clonal hematopoiesis. Some of the cytogenetic abnormalities, i.e. isolated deletion 5q, are related to the molecular pathogenic mechanisms of MDS, some others are secondary events, related to leukemic transformation while the significance of a small number of them has not yet been completely evaluated (10, 39).
Even though cytogenetics can confirm the diagnosis in a more robust way for the majority of patients, the need for further investigation in certain cases remains, and to this point refined MFC analysis aims to fill this diagnostic gap and/or add new information.
Early applications of Flow Cytometry analysis in the field of MDS included the demonstration or clarification of the lineage of origin of an immature cell population, not easily identifiable by cytochemistry or immunohistochemistry (40, 41), as well as the clarification of the various hematopoietic cell lineages involved in the clonal process (42). It also included the identification of missing expression of characteristic maturation antigens from the cell surface of committed or mature hematopoietic cells or the aberrant expression of such antigens on cells that should not normally be expressed (e.g. lineage infidelity) (43, 44). Flow cytometry analysis has also been used for the determination of DNA ploidy of the abnormal cells or the estimation of median DNA cell content, and for the determination of immature cell growth fraction, by testing S-phase specific biomarkers, such as BrdU, PCNA, CD71 or Ki-67 expression (45, 46). The identification of hypodiploid cells has been associated with dismal prognosis (47, 48). Additional early applications were the determination of an immunophenotypic profile for the various types of clonal cells (erythroblasts, myeloblasts, megakaryocytes), as well as of the mature cells, including erythrocytes, monocytes and platelets, the enumeration of blast cell percentage and the distinguishment of clonal hematopoietic cells from non-clonal cells infiltrating the marrow and from marrow stromal cells, mesenchymal cells and other cell types of the hematopoietic microenvironment (43, 49–53). Moreover, flow cytometry has contributed to the identification of various metabolic abnormalities of the clonal hematopoietic cells (54, 55), resulting in impaired function (54–56), apoptosis (57), oxidative stress (58), inflammatory status, senescence, mitochondrial damage, important regulatory protein expression (TP53, cytochrome c, hemoglobin, growth factor receptors etc) (59–62). Finally, flow cytometry has been used for immunophenotyping of peripheral blood lymphocyte subpopulations and of the bone marrow lymphoid component, as well as for the investigation of hematopoietic cell immunophenotypic alterations, following chemotherapy (particularly with alkylating or hypomethylating agents) and radiotherapy (63–65). Table 3 resumes these initial applications of flow cytometric analysis of MDS.
Since 2008, the WHO classification of hematological neoplasms has recognized the need of extended, secondary diagnostic criteria for the identification of clonal dysplastic hematopoiesis, characterizing MDS, to distinguish borderline MDS cases from benign, non-clonal, reactive or autoimmune conditions mimicking MDS, as well as various pre-neoplastic clinical entities such as idiopathic cytopenia of undetermined significance (ICUS), clonal cytopenia of undetermined significance (CCUS), clonal monocytosis of undetermined significance (CMUS) or idiopathic dysplasia of unknown significance (IDUS) (10, 66).
The study of antigenic expression of clonal hematopoietic cells of MDS patients by MFC has revealed abnormal antigen expression profiles in all the maturation and differentiation stages of hematopoietic cell lines, but no single antigen is considered pathognomonic to myelodysplasia (67–71). These abnormalities are: aberrant antigen expression of myeloid and erythroid cells and abnormal hyper- or hypo-expression of some specific antigens. These abnormal profiles show impressive repeatability in MDS patients and are distinct for each maturation stage (69, 72).
Technically, MFC is capable of producing large datasets from a single sample analysis, providing multi-dimensional data for every cell analyzed. Although one can easily realize why this can be a great advantage, considering the unique capability of depicting complicated patterns of cell surface antigen expression in every stage of cellular maturation in the BM, it might also become a great disadvantage. MFC requires a detailed technical surveillance of both, sample preparation and analytical protocols, and relies vastly on the expertise of the analyst. In addition, different instrumentation and reagents may produce heterogenous results and interlaboratory standardization and harmonization may be needed, in order to overcome data heterogeneity and produce comparable and reproducible results (73, 74).
In 2008, the first ELN working conference on flow cytometry in MDS took place, with the aim to standardize MFC in MDS. To that end, thirty participants from 18 institutes throughout Europe, working within the ELN and 3 experts from outside Europe (USA and Japan) joined this meeting, to define the role of MFC in diagnosis and prognostication of MDS in relation to the validated French-American-British (FAB) and WHO classifications, and to international prognostic scoring system (IPSS) and WHO classification-based prognostic scoring system (WPSS) systems; (b) to discuss the optimal methods of sample processing and handling; (c) to propose a consensual minimal set of monoclonal antibodies capable to assess dysplasia by MFC of BM cells in known or suspected MDS cases; (d) to consider the specificity of MFC analysis for MDS, related to a series of other hematologic benign or malignant diseases and (e) to suggest additional recommendations on MFC, to further optimize analysis for future directions (69). In 2012, after the WHO 2008 acknowledgement that the presence of more than 3 immunophenotypic aberrancies might be indicative of MDS, the ELN working group alongside with an international consortium cooperated to (a) define the minimal requirements to assess BM dysplasia by MFC, both, in immature progenitor cells and in the maturing myelomonocytic lineage in known or suspected MDS; (b) define how these data are to be captured, that is, how to focus on the population of interest and how they should be interpreted objectively; (c) consider the specificity of MFC analysis for MDS, as compared to a series of other either clonal or non-clonal hematological diseases, and (d) define the role of MFC in the diagnosis and prognosis of MDS in relation to the validated prognostic systems, including relevant prognostic cytogenetic and molecular markers (75).
At the same time, the Euroflow consortium (EuroFlow) evaluated the use of MFC for the detection and characterization of abnormal hematopoietic cell populations in the BM of patients with hematological malignancies and suggested an antigenic panel of 8 colors for the detailed immunophenotyping in suspected MDS and AML cases (76).
The proposed analyses constituted the basis for the implementation of multiparametric panels in clinical laboratories for the evaluation of suspected MDS from BM samples. The aim of these guidelines was to provide a common and validated strategy for the detection of marrow myeloid progenitors, the quantification of their percentage and the qualitative assessment of the different cellular populations at various stages of maturation and differentiation, as well as to compare their expression profiles with normal antigen expression patterns and with known alterations suggestive of dysplastic hematopoiesis. Most of these alterations have been thereafter, validated in several studies, providing the basis for MFC diagnostic algorithm for MDS (32, 77). Characteristic examples of antigen expression aberrancies on myeloid progenitors are: the asynchronous expression of mature and immature antigens e.g. co-expression of CD34 and CD10, the expression of infidelity markers (antigens normally expressed on other cell lineages) e.g. expression of CD7, which is a T lymphocyte marker on myeloid progenitors. For maturing cellular stages, dysplasia is commonly depicted by altered scatter characteristics, e.g. decreased side scatter in neutrophils and/or the hypo- or hyper-expression of common myeloid markers (e.g. CD16, CD13, CD11b, CD33, CD10) (68–71). Some characteristic disrupted patterns seen in dysmyelopoiesis are depicted in Figure 1.
Figure 1. Myeloid cell antigen expression patterns of CD16/CD11b/CD13 and CD45 on normal, MDS and blood-contaminated BM samples. Color dot plots of antigenic expression of CD45, CD11b, CD16 and CD13 on bone marrow myeloid cells. Cellular populations depicted: lymphocytes (blue), monocytes (red), myeloid progenitor cell compartment (light blue), lymphoid progenitor cell compartment/hematogones (orange), maturing granulocytes (CD45dim: purple, CD45normal: green). The myeloid progenitor cell compartment and maturing granulocytic compartment constitute the “myeloid” gate. Dot plots of side scatter/front scatter [SSc/FSc] (A), SSc/CD45 (B), CD13/CD16 (C), CD11b/CD16 (D) and CD11b/CD13 (E) from normal bone marrow, MDS and a sample with significant blood contamination exhibit three different maturation/differentiation patterns of maturing granulocytes. (normal B–E): The blood contaminated sample contains only neutrophils (CD45normal to bright, CD16bright, CD11b bright, CD13normal to bright) and every other granulocytic maturation stage is absent from the analysis. The MDS sample exhibits significant variation from normal: maturing granulocytes with decreased MFI of CD11b and CD45 (normal D, B versus MDS D, B), increased CVs of CD45, CD13, CD11b, CD16 and aberrant patterns of co-expression of the three myeloid antigens (normal C–E, MDS C–E). Plots were obtained from Kaluza 2.1 software (Beckman Coulter; RRID: SCR_016182).
These multiparametric analyses have highlighted the diagnostic utility of flow cytometry in MDS, but rely vastly on the analyst’s expertise and are time- and cost-consuming, rendering the development of flow cytometric scores the next rational step for the implementation of practical and easily-obtained diagnostic tools, that would overcome the complexity of the previous protocols (30, 31, 78–86).
The Wells algorithm (87) resulted in a flow cytometry scoring system (FCSS) that allows a simple numerical display of the myeloid and monocytic dyspoiesis in the BM that can be correlated with the IPSS and the outcome following hematopoietic stem cell transplantation. The Ogata score has been one of the most universally applied attempts to develop a clinically useful and easily implemented tool, by minimizing parameters and complexity at the same time, targeting the low grade MDS patient population without distinctive diagnostic features (without excess of blasts or ringed sideroblasts) (29, 88). Ogata score utilizes a minimal MFC panel of four parameters, created from one cell sample stained with two fluorescent antibodies, anti-CD34 and anti-CD45 (29, 85). It is technically simple and reproducible by other groups (83, 89–92), but its sensitivity and specificity in diagnosing low grade MDS is limited. Thus, the authors later proposed a new parameter: the CD33 expression on CD34+ myeloid progenitors and maturing granulocytes (86) which has showed 50% sensitivity and more than 95% specificity in low grade MDS. Our group has proposed a dysmyelopoiesis flow score (DMI) that has 77% sensitivity and 93.6% specificity for low grade MDS, indicating the presence of multilineage dysplasia in certain cases, which were falsely characterized as refractory anemias by cytomorphology evaluation (30).
Erythroid cells exhibit commonly some of the most characteristic dysplastic morphological features in MDS and that is why many groups have tried to incorporate immunophenotypic alterations seen in dyserythropoiesis in flow scores, to increase the sensitivity of low grade MDS detection (31, 82–84, 93, 94).
The detailed description of immunophenotypic aberrancies on the surface or the cytoplasm of cells of different cellular lineages in MDS has been the basis for the development of every diagnostic tool or algorithm, proposed up to date. The majority of the proposed indices of dysplasia have used BM samples since the abnormal expression patterns are recognized, not only on mature and terminally differentiated cells, but also on the immature marrow progenitors.
One of the most common requests in flow cytometric analysis of confirmed or suspected MDS is blast cell quantification. Estimation of blast cell percentage by morphology discriminates MDS from AML and further defines prognostic relevant subcategories, according to both, WHO classification and revised international prognostic scoring system (IPSS-R). MFC can quantify and characterize immature cells, but it is important to note that their percentage although correlates, it is not identical with the morphological findings (95). Visually characterized myeloid blasts do not always correspond to immature cells in MFC, that are traditionally defined as CD45weakCD34+CD117+ HLADR+ (96, 97). This discrepancy can stem from the removal of erythrocytes from the phenotypic analysis but is mainly attributed to the fact that morphologically identified blasts can be more mature and differentiated cells than myeloblasts, sometimes referred to, as blast equivalents, such as neoplastic promonocytes or promyelocytes in certain cases of acute leukemias, a term adopted by the ICC in the latest classification (12). Another confounding factor can be hemodilution of the sample that leads to artificially lower blast percentages. Nonetheless, a multicenter study of the ELN iMDSFlow WG, published in 2023, confirmed that a percentage of ≥3% marrow myeloid progenitors (CD45weak/SSClow/CD34+/CD19) strongly suggests the existence of MDS or myelodysplastic/myeloproliferative neoplasms (MDS/MPN) (77).
The added value of MFC lies in the ability to characterize the abnormal cell compartment for aberrant expression of antigens that can be infidelity markers (CD2 and CD7 expression on myeloid cells), asynchronously expressed, overexpressed or lacking (e.g. lack of CD34 expression on CD117+ cells or overexpression of CD117, which is associated with worse survival) (98). These findings can define myelodysplasia irrespective of the actual blast cell percentage.
The combination of side scatter (SSC) and CD45 expression constitutes the main gating strategy for the myeloid populations that are affected, along with the erythroid lineage in the context of MDS. In some cases, in which these populations are hard to be defined, auxiliary markers, such as CD64 or CD33 can be utilized.
The low SSC exhibited by granulocytes is well-described and correlates with morphological dysplastic features, such as hypogranulation. It is one of the main parameters of the Ogata score, suggesting that the ratio of granulocyte SSC to lymphocyte SSC offers a more sensitive and quantifiable marker of dysplasia (85, 86). Since this is a simple and not reagent-demanding analysis, it can be implemented to the study of any sample suspicious for MDS before any further analysis. Moreover, the Ogata score is recommended by the iMDSFlow as a preliminary assessment for MDS and this has been validated by several publications (89, 91, 99, 100).
In terms of fluorescence, the co-expression patterns of CD13/CD11b and CD16/CD11b can offer very important information, concerning abnormal maturation and differentiation of the myeloid compartment, as they appear, are co-expressed and disappear creating specific patterns in normal BM (30, 76). These patterns are reliable indices of dysplasia, provided that the sample quality is acceptable. Some characteristic disrupted patterns seen in dysmyelopoiesis are depicted in Figure 1, compared to normal findings and a blood contaminated BM sample. Additional insight is offered by the cytoplasmic markers MPO (myeloperoxidase- found in primary granules) and LF (lactoferrin- secondary granules), along with CD10 and CD15, that define mature granulocytes (101). Severely altered expression of MPO/LF has been correlated with low-risk MDS and can distinguish them from normal samples (102). It is also important to delineate the potential aberrant expression of infidelity markers, such as CD56 or other lymphoid indices in dysplastic granulocytes (77). From recent reports, the most sensitive marker for granulocytes, with a specificity of nearly 100% in the dysplastic granulocytic marrow compartment, is an abnormal SSC distribution, whereas abnormal maturation profiles through CD13/CD11b, CD16/CD11b and CD16/CD13 co-expression patterns, along with aberrant expression of CD2 CD7, CD5 and CD19 occur in about 70% of the cases (69, 75).
The monocytic lineage presents a variety of phenotypic disorders. The typically expressed markers CD36, CD14, CD16, CD11b, HLADR, CD13, CD33 can be lacking or have an abnormal expression (77, 89, 103–105).
The aberrant overexpression of CD56 is also frequently observed, although it can be also found in reactive/regenerative conditions of the BM (89). CD14 and CD16 expression defines three distinct monocyte sub-populations (classical monocytes CD14+CD16-, intermediate CD14+CD16+ and atypical CD14weakCD16+), whose relative percentages can be disturbed in dysplasia, chronic myelomonocytic leukemia (CMML) and in reactive/inflammatory conditions. This distribution is the most sensitive marker in CMML and is more pronounced in the peripheral blood, where a cutoff >94% of classical monocytes has been proposed to strongly point to CMML (103).
The erythroid lineage, delineated by negative CD45 and low SSC, is another target for flow cytometric analysis in the context of MDS. Anemia and transfusion dependency remain one of the most frequent presenting features triggering the MDS diagnostic investigations. In classical MFC protocols, RBC lysis preceding analysis, shortens the erythroid compartment that remains, which, along with the paucity of available markers, renders the analysis suboptimal (84). Therefore, efforts have been made to develop non-lysis protocols, that preserve all erythroid cells (83, 94) and several new markers have recently been tested. The initially reported hypoexpression of CD71 and CD235a have been supplemented with the study of CD36, CD117 and CD105, providing new information on the development of the erythroid lineage. In 2013, Mathis et al. developed the RED score for dysplasia, using hemoglobin levels, CD71 and CD36 expression (83), which, when combined with the hepcidin:ferritin ratio appears to predict response to erythropoietin (EPO) in lower-risk MDS (82). In 2017 the iMDS Flow Group reported that an increased coefficient of variation (CV) of CD36 and CD71 constitute the best indicators of dyserythropoiesis (84) and Cremers and al. incorporated their findings to the existing diagnostic MFC scores, to improve their sensitivity (31). In 2019, Violidaki et al. developed a protocol using CD36, CD71, CD105, CD117, CD13 and CD45, combined with a no-lysis protocol, which provided a more reliable evaluation of erythroid dysplasia and led to the integration of artificial intelligence (AI) in the form of Flow-Self Organizing Maps algorithm (FlowSOM) unsupervised analysis, to define distinct clusters within the erythroid compartment in normal BM and subsequently in MDS (94, 106, 107). This analysis demonstrated that the abnormal clones gradually decreased after treatment with azacytidine and were nearly normalized after allogeneic hematopoietic stem cell transplantation (HSCT).
Megakaryocytes, mainly due to their size, fragility and scarcity in the BM aspirates, have not been extensively studied in the context of MDS. Focusing mainly on megakaryoblastic leukemias, the EuroFLOW has included the megakaryocytic/platelet markers CD61, CD41 and CD42a in the AML/MDS panel, albeit not considering them beneficial for the evaluation of suspected MDS (76).
CD41 (or Glycoprotein IIb-GPIIb) has been evaluated in the context of MDS blasts. It may be present at diagnosis or appear during disease progression, has been detected in several cases with unfavorable prognosis and appears to be associated with monosomies or complex karyotype (108, 109).
Due to the above-mentioned difficulty of megakaryocyte analysis, efforts have been made to evaluate peripheral blood platelets as potential indicators of dysplastic thrombopoiesis. From the markers evaluated in a study with 83 participants (44 MDS, 20 healthy subjects and 19 patients with non-MDS conditions), CD61, CD36 and CD42a were found decreased and CD34 was asynchronously expressed within the MDS group (110). Although the authors have proposed a diagnostic score, these observations have not been further validated and widely accepted.
An often-mentioned phenotypic characteristic of the lymphoid BM subpopulations in MDS is the relative decrease of lymphoid progenitors (69). It is included in the Ogata score (as <5% B-progenitors in all CD34+ cells) (59), but has been excluded when the score was revised (86).
The immunological alterations of the dysplastic BM are plenty and can give rise to both, a pro-inflammatory and an immunosuppressive profile (in lower- and higher-risk MDS, respectively (111, 112). This in turn is expressed by altered relative percentages of T cell sub-populations, along with cells of the dendritic cell compartment. CD8+ cytotoxic T-lymphocytes are found increased in the peripheral blood and BM of lower-risk myelodysplastic syndromes, reflecting an anti-tumor attempt that ends up by suppressing both, the malignant and benign hemopoiesis (113–115). In this instance, Th17 cells are increased and Tregs are low, whereas in more advanced stages of the disease this profile shifts to a more immunosuppressive one, with high numbers of Tregs, and Th22 lymphocytes, and low CD8+ T cells, and with immune checkpoint molecules upregulation (116, 117).
The use of markers detecting apoptosis or cell proliferation has long been used as a standard practice in the histopathologic examination of hematologic malignancies but has been less established with MFC. Several types of markers and MFC protocols have been evaluated for the evaluation of proliferation (118, 119) and apoptosis (120–122) but, in contrast to other neoplastic diseases, the pathways and the biology of cell survival and death in MDS are variable and multifactorial. Many studies have demonstrated that in the early stages there is a high apoptotic index, that later shifts to a high proliferation/low apoptosis state, as the leukemic clone is progressing, and this is also correlated with IPSS (123–125). Pathways of pyroptosis, detected cytometrically by the expression of casp-1, appear to participate in the early apoptotic stages (126, 127) possibly through toll-like receptor 4 or CD33. In a review article by Menstrum et al., 18 studies (each of them analyzing >10 subjects) have been assessed for the prognostic potential of proliferation/apoptosis analysis. The results have confirmed the afore-mentioned observations and have detected correlations with OS, PFS and response to treatment for MDS and AML (128).
The recent standardization of MFC by EuroFlow could allow the integration of such diagnostic markers of cytometric evaluation in the clinical setting. Nies et al. proposed an MFC assay to evaluate proliferation rate, during maturation of various hematopoietic lineages in the normal BM (129) and attempts have been made to implement this assay as a diagnostic tool (130).
There are several clinical entities sharing overlapping clinical and/or laboratory features with those of MDS, raising a, sometimes urgent, diagnostic problem. Cytopenias and especially pancytopenia may accompany various infections or other inflammatory conditions, in association with morphologically atypical cells. In these cases, MFC may offer a solution to the diagnostic problem, restricting the need for more advanced, expensive and resource-consuming investigations, by the enumeration and immunophenotypic characterization of myeloid progenitors and the delineation of normal/abnormal or aberrant maturation patterns.
In the case of paroxysmal nocturnal hemoglobinuria (PNH) that can accompany other cytopenia-inducing conditions, including MDS, MFC remains the gold standard for diagnosis, by confirming the loss of the glycosylphosphatidylinositol (GPI)-anchored proteins CD55 or CD59 on the surface of red cells or CD16 and fluorescein-labeled proaerolysin (FLAER) on granulocytes, within hours (131).
Aplastic anemia represents a challenge in morphological evaluation, due to the severe hypoplasia of all BM cellular compartments. Although MDS usually presents with hypercellular or normocellular BM, in 10-15% of cases the biopsy detects hypocellularity (132). Nonetheless, due to the ability of MFC to detect and evaluate large numbers of cells, an accurate percentage of progenitors can be deduced, as well as dysplastic features in the granulocytic and erythroid lineages. Moreover, it is a relatively easy evaluation, which can be repeated whenever any potential disease progression is suspected.
In the context of CMML and pre-CMML conditions, apart from a first evaluation of the BM blast percentage, aiming to exclude AML, and the evaluation of monocyte subsets, as was described earlier, neoplastic monocytes exhibit frequently an aberrant expression of CD56, CD2, CD5, CD10, CD23 or under-express CD13, CD14, CD15, CD33, CD38, CD45, and CD64 (133). Bearing in mind that phenotypically abnormal monocytes may also occur in other myeloid neoplasms, they are nonetheless typically neoplastic cells and warrant further investigation. A special mention should also be made on systemic mastocytosis associated with CMML, in which mast cells invariably express CD25 on flow cytometric analysis (134).
Perhaps the most abundantly discussed problem, concerning MDS, is their differential diagnosis with ICUS and CCUS. Although these entities may indeed represent early stages of MDS, they do not fulfill the morphological criteria of dysplasia, highlighting the ability of MFC to detect -and also monitor by serial testing- abnormal maturation patterns and clone establishment or instead to exclude MDS (135). The usually applied scores (e.g. Ogata or Wells) do not perform well in the differentiation of ICUS vs CCUS but show promise in selecting patient populations with a higher risk of progression (136). When morphological criteria for MDS were not met but MFC features indicated MDS, there was a rate of 50% of progression to overt MDS (137, 138).
For more than 15 years, MFC findings have also been tested as a prognostic tool, either by being correlated with the validated prognostic scoring systems for MDS patients or by assessing their independent prognostic value for overall survival (OS), leukemia free survival (LFS) or post-transplantation outcome in several studies (99, 138–143). These studies highlight the prognostic significance of MFC findings, incorporated in various immunophenotypical scores or sums of abnormal findings instead of single parameters tested (98, 144, 145) but until now immunophenotype is not an established tool for monitoring, staging, and predicting disease and treatment outcomes in international guidelines for MDS (146). Relatively early it has been recognized that a higher expression of HLA-DR in association with low expression of CD11b on total BN cells may predict earlier transformation to AML (147). In other instances, MFC scores have been developed that hold independent prognostic value but do not correlate significantly with IPSS–R, identifying patients with different OS, as reported by Vido-Marques et al (78). Gardikas et al. have reported that a higher rate of apoptosis in the CD34+/CD117+ myeloid compartment is an independent favorable prognostic factor for both, OS and transformation to leukemia (148). Recently, Guarnera et al. have shown that Ogata score ≥2 is significantly associated with the detection of ≥2 mutations, as well as with inferior event-free-survival in MDS patients (149). In a cohort of cytopenic patients (either with MDS or nonclonal cytopenia), without considering cytomorphology diagnosis, iFS (integrated Flow Score) and Ogata score have shown prognostic significance for overall survival. Furthermore, in cytomorphologically characterized MDS patients, multivariable Cox regression analysis including iFS, IPSS-R (as a whole or with its single components) and age has shown that iFS could add independent prognostic information, beyond IPSS-R (32). Oelschlaegel et al. recently published a new MFC strategy for screening MDS, which could be used as a treatment monitoring tool after further validation of its value (150).Future validation of these scores as well as further integration with genetic and molecular data, in tools such as the molecular IPSS would probably clarify the potential prognostic value of immunophenotype in the molecular classification era.
The first classification introducing cytogenetics features was the 3rd classification of myeloid neoplasms by WHO in 2001. In this classification, MDS with isolated deletion of the long arm of chromosome 5 [del(5)q] was identified as a separate entity. Since then, the WHO’s revisions published in 2008 and 2016 have refined additional clinical entities. The introduction of SF3B1 gene mutation in the criteria of diagnosis of MDS with ring sideroblasts and the identification of Clonal Hematopoiesis of Indeterminate Potential (CHIP), an entity without clear MDS features but with gene mutations commonly seen in MDS in the 2016 revision, reflected the great importance of the molecular characteristics (11). The latest WHO edition (2022) incorporated subtypes, based on their molecular characteristics, such as the MDS with biallelic TP53 inactivation (MDS-biTP53), or MDS with low blasts and SF3B1 mutation (MDS-SF3B1), which replaced the previous entity “refractory anemia with ring sideroblasts”, and finally the Clonal Cytopenia of Undetermined Significance (CCUS), which is defined as CHIP in the presence of one or more persistent cytopenias that are otherwise unexplained (13). The same year, the ICC group also suggested a similar classification while redefining the percentage of blast cell boundaries for the characterization of MDS versus AML (12). The continuous accumulation of genetic data in the classification systems in the last decade was resulted from the extended use of Next Generation Sequencing (NGS) (151). These data have been associated with morphological features and, in some cases, with specific immunophenotype alterations (152, 153).
The genetic landscape of MDS is quite complex. Several mutations have been identified in genes that are involved in RNA splicing (SF3B1, U2AF1, SRSF2, and ZRSR2), DNA methylation (DNMT3A, TET2, IDH1/IDH2), chromatin modification (EZH2, ASXL1, KDM6A), transcription (RUNX1, BCOR, ETV6, GATA2), cohesion complex (STAG2), signal transduction (JAK2, KRAS/NRAS, CBL), and tumor suppression (TP53, WT1) (33, 154). Some mutations, such as TP53multihit, FLT3, MLLPTD, ASXL1, BCOR, EZH2, NRAS, RUNX1, STAG2, and U2AF1 show unfavorable outcomes regarding OS, LFS, and AML transformation. Although SF3B1 mutations seem to have favorable outcomes, this is not always the case, when additional mutations coexist (34).
The translation of this knowledge to clinical practice was the incentive to develop the new classification systems, in an effort to more accurately describe subgroups of patients with different biological behavior, clinical presentation or prognosis and individualize our treatment strategies (155). MDS with low blast percentage and SF3B1 mutation (MDS-SF3B1) is a distinct 2022 WHO and ICC classification sub-category, replacing the MDS-RS of the previous WHO revision. The patients usually have a relatively good prognosis, anemia, and a high degree of ineffective erythropoiesis (156). SF3B1 gene encodes a protein that plays a critical role in the spliceosome machinery. Mutant SF3B1 induces errors in the splicing process of mRNA of the genes involved in iron homeostasis, such as the PPOX and ABCB7 genes. This leads to iron accumulation in the mitochondria of the erythroid progenitor cells, resulting in the formation of ring sideroblasts, the morphologic hallmark of sideroblastic anemia. In MDS carrying mutations of the SF3B1 gene, there is also increased erythroid apoptosis due to impaired GATA-1 expression and end-stage erythroid maturation arrest attributed to EIF2AK1 activation (157, 158). The available therapies try to reduce erythoblastic apoptosis and alleviate anemia and its symptoms.
TP53 mutations are present in many types of cancer. The occurrence of these mutations in MDS and AML constitutes a criterion to define separate disease categories according to the new classification systems. Thus, in the 2022 WHO classification, MDS with biallelic TP53 inactivation (MDS-biTP53), which means the presence of two or more TP53 mutations or of one mutation, with evidence of TP53 copy number loss or copy neutral loss of heterozygosity (LOH), when BM or peripheral blood blasts are less than 20% constitutes a new clinical entity. In the ICC classification, the same entity is defined as the myeloid neoplasm with two distinct TP53 mutations (MDS-TP53, MDS/AML-TP53), each with a variant allele frequency (VAF) of >10% or a single TP53 mutation with one of the three following criteria (1): 17p deletion on classical cytogenetics; or (2) VAF of >50%; or (3) copy-neutral LOH at the 17p TP53 locus (33). The MDS with TP53 mutations is characterized by poor prognosis and chemotherapy resistance. It should be noted that patients with a single TP53 mutation have the same prognosis as the wild type (159).
As mentioned above about 50% of patients with MDS have an abnormal karyotype. These abnormalities are mainly consisting of chromosomal deletions, monosomies, trisomies, and more rarely inversions or translocations. One of the reasonably common and well-studied cytogenetic alterations is the del(5)q MDS with del(5q) represent a unique category and was the first genomic aberration included in the WHO classification. Patients without an excess of blasts, exhibiting this abnormality alone or accompanied by an additional one, other than monosomy 7 or del(7q) tend to have several common clinical and morphologic features, steady clinical course and a quite good prognosis (160). Patients usually have macrocytic anemia, without other cytopenias or sometimes exhibit mild to moderate thrombocytosis. Their megakaryocytes have smaller size and have hypolobulated or non-lobulated nuclei. Patients respond favorably to treatment with lenalidomide, possibly due to the haploinsufficient casein kinase-1-alpha-1 (CSNK1A1) gene (161). This gene has a pathogenetic role in the clonal expansion of malignant cells and the disease represents an acquired ribosomopathy, resulting from haploinsufficiency of the crucial Ribosomal Protein of the Small subunit-14 (RPS14) gene, which resides in the deleted 5q region. Haploinsufficiency of the RPS14 affects ribosomal assembly, impairs erythroblastic protein synthesis and results in early cell death and dyserythropoeisis. There are many more genes in the deleted chromosomal locus that contribute to the clinical and laboratory features of the disease. For instance, the haploinsufficient gene coding miR145 through the consequent upregulation of Friend leukemia virus integration 1 (Fli1) gene enables the effective megakaryopoiesis and results in thrombocytosis (162).
The interplay of immunophenotypical findings and genetic alterations partly reveals the association between genotype and phenotype and has been a major field of investigations for the past few years. Some studies have demonstrated an association between genotypic abnormalities and phenotypic patterns in patients with unexplained cytopenia (163). The main findings of these studies are summarized in Table 4. All patients with more than two cytogenetic abnormalities, monosomy 7/del(7q) or del(5q) were shown to have a positive FCSS (163). Guarnera et al. examined 106 patients with MDS and 39 controls, and using the clustering proposed by the EuroMDS score, recently reported that certain immunophenotypic aberrancies are associated with different clusters (149, 155). Ogata score ≥2 in MDS patients was found to correlate with the existence of more than 2 mutations in the molecular testing, as well as with epigenetic modifier gene mutations, such as SRSF2 and TET2 (149). On the other hand, Euro-MDS group 0 (without specific genomic profiles) is associated with Ogata score<2. Regarding specific markers, CD56 expression has been associated with DMNT3A and AML-like mutations (NPM1, FLT3, IDH1, and RUNX1 genes) (Euro-MDS group 7), and CD15 expression with U2AF1 mutations (Euro-MDS group 4). CD38 expression has been specifically associated with TP53 mutations, whereas CD117 expression correlates with Euro-MDS group 2 (TP53 mutations or complex karyotype) (149, 155). Moreover, decreased CD177 positive neutrophils have been associated with specific gene mutations in myeloid neoplasms, such as FLT3-ITD, NPM1, NRAS, RUNX1, TET2 and U2AF1 S34F (135). However, this association has not been confirmed in a separate MDS patient cohort (164).
Table 4. Association of specific genomic alterations with characteristic immunophenotypic findings in MDS patients.
Recently, the combination of fluorescence in situ hybridization (FISH) and flow cytometry cell sorting has identified distinct distributions of cytogenetic abnormalities across different myeloid maturation stages. Patients were divided into three categories: Patients with accumulation of alterations on immature myeloid cells, such as those with monosomy 7, patients with a single cytogenetic abnormality, detected throughout all maturation stages, such as those with good-to-intermediate prognosis (according to IPSS-R) and patients with evidence of clonal evolution. In the latter group, abnormalities representing the founding clone were evenly distributed across stages of myeloid maturation, whereas subclonal abnormalities were mostly encountered in the immature myeloid populations (165).
Various recent reports attempt to describe specific immunophenotypic profiles of key molecular or cytogenetic findings in MDS. The major findings of these studies are summarized in Table 4.
One of the hallmarks of MDS del(5q) immunophenotype is the increased number of myeloid progenitor cells (153). The CD45-MFI-ratio, defined as the intensity of CD45 in reference to the normal lymphocytes (87), is associated with maturation within the progenitor cell compartment and has been found to be normal-to-low in del(5q) MDS patients (153). Even though an abnormally low granulocyte SSC-ratio is observed in almost all del(5q) patients, the low degree of dysgranulopoiesis observed cytomorphologically is consistent with normal granulocyte CD71 and CD10 expression. Moreover, although previous reports have reported CD14 overexpression on the BM granulocytes (166), more recent reports did not confirm this finding (167). Lastly, CD71dim nucleated erythroid cells are distinctly higher in del(5q), compared to normal karyotype MDS patients (153).
Based on these observations, Oelschlaegel et al. proposed the 5-parameter-del(5q)-score, including CD45-MdFI-ratio (lymphocytes vs myeloid precursors; ≤7.0, 10 points), percentage of myeloid precursors (>2.0%, 3 points), granulocytes versus lymphocytes SSC ratio (<6.0, 2 points), CD71 expression on granulocytes (≤20%, 1.5 points), and sex (female, 1.5 points). A score of 15.0 or more can predict 95% of MDS harboring this abnormality whereas a score of less than 10 was not identified in del(5q) MDS (153).
Recent data have shown that myeloid neoplasms with monosomy 7 typically demonstrate multiple immunophenotypic abnormalities on myeloid blasts and maturing myelomonocytic cells (167). In general, immunophenotypic aberrancies are more common in patients with monosomy 7, than in patients with del(7q). Specifically, increased CD14 expression on maturing granulocytic cells is the most frequent aberration associated with monosomy 7, but not with deletion 7q. Increased CD14 is observed in patients with monosomy 7 as a single abnormality, as well as in those with additional cytogenetic abnormalities. In some patients with monosomy 7, this increase in CD14 expression is present uniformly at all stages of maturation, while other patients exhibit variability of CD14 expression at different compartments of granulocytic maturation, with some of them expressing normal levels of CD14 and other demonstrating increased CD14 expression. Chen et al. hypothesized that these patterns may reflect the size of the clones harboring the chromosome abnormality. Granulocyte CD64 retention was also more frequently observed in patients with monosomy 7, than in those with deletion 7q. On the other hand, in both of these chromosome 7 abnormalities, CD13 expression is usually found increased on the granulocytic precursors and on maturing granulocytes in the majority of cases. The same finding has also been observed on granulocytes following G-CSF stimulation.
Regarding myeloid blasts, aberrant CD5, CD7 and CD56 expression are observed with substantial degree of variability in both, chromosome 7 abnormalities, alone or associated with other abnormalities (167).
As mentioned above, MDS -SF3B1 is now classified as a distinct subtype, due to its favorable course and responsiveness to treatment with luspatercept (156). Favorable prognosis is further underlined by the low incidence of high (>2%) CD34+ myeloid progenitors in this MDS subtype. Moreover, Duetz et al. reported that compared to other subtypes, SF3B1-mutant MDS presents significantly higher percentages of erythroid progenitors and mast cells. Erythroid progenitors express less CD71 than other types of MDS, indicating that the CD71-shedding process is severely disturbed. CD71 presents high CV, that is negatively correlated to hemoglobin levels in these patients. High prevalence of ring sideroblasts is accompanied by higher erythrocyte progenitor sideward-light-scatter (SSC). Higher SSC has also been observed in myeloid progenitors and higher expression of CD11b has been reported in neutrophils. Monocytes in SF3B1-mutant MDS also have higher CD11b expression, along with lower expression of CD36 and CD64. Duetz et al. have clarified that this increased CD11b expression in neutrophils and monocytes, as well as higher CD36 expression and higher numbers of mast cells, are more prominent among patients with the K700E SF3B1 mutation. Lastly, in patients with both, SF3B1 mutation and del(5q), erythroid progenitor cell count, CD71 marker CV and mean fluorescent intensity (MFI) resembled more the del(5q) phenotype, suggesting that patients with both mutations might benefit more from lenalidomide than luspatercept (168).
Another gene of the splicing machinery that is of major importance in MDS is the serine and arginine-rich splicing factor 2 (SRSF2). Mutations of SRSF2 are identified in 15-20% and have been associated with increased age and favorable prognosis (169, 170). In SRSF2 mutant MDS, a distinct immunophenotype was identified by Weiß et al., exhibiting a specific CD11b/CD16 co-expression pattern in granulocytes and reduced CD45 expression in myeloid progenitors. Normal granulocytic maturation initiates with an abrupt increase in CD11b expression and a parallel slight increase of CD16 expression. Subsequently, follows a steep increase in CD16 expression, paralleled by only a minimum further rise of CD11b expression (giraffe pattern). Diversely, SRSF2 mutant MDS is characterized by an increase of CD11b without CD16 expression, followed by an increase in CD16 expression without any further increase of CD11b expression (rectangle pattern) and only a dim CD45 expression in myeloid progenitor cells (152).
Immunophenotypic profiles of TP53 mutated MDS and AML patients are very similar, and the only difference is the higher percentage of CD7 expressing blasts, observed in MDS patients (171). Additionally, overall CD38 expression is specifically associated with TP53 mutation and overall CD117 expression correlates with TP53 mutation or with complex karyotype (149). Compared to normal karyotype, TP53 mutated AML blasts exhibit increased expression of CD34, CD13 and CD5, but lower expression of CD10. Moreover, monocytes in complex karyotype or/and TP53 mutated AML patients usually show markedly brighter expression of CD7, CD11b and CD13, as well as aberrant expression of CD34. The granulocytes of these patients demonstrate modestly higher expression of CD3, CD5, CD7 and CD14. Considering the above, aberrant expression of T-cell antigens within the myeloid lineage is characteristic of complex karyotype or/and TP53 mutated MDS/AML (172).
Nucleophosmin (NPM1) mutations are present in about 30% of adult AML patients but are also present in ~4% of MDS patients (173, 174). Current classifications differ in the characterization of these patients, since NPM1 mutation constitutes an adequate criterion for AML diagnosis in WHO 2022 classification, while the ICC requires the 10% blast percentage as a co-criterion for the establishment of AML diagnosis, thus labeling as MDS patients with a lower blast- (or blast equivalent) cell percentage. Immunophenotypic analysis of leukemic myeloid blasts in AML and MDS with excess of blasts (MDS-EB), exhibited a common pattern of leukemia associated immunophenotype (LAIP), which was characterized by CD117+ blasts with low side scatter and decreased or complete absence of CD13, CD34 and/or HLA-DR expression, increased CD33 and/or CD123 expression, as well as absence of CD15 and CD64 expression. This pattern resembles that of normal promyelocytes (CD34-, CD117+, CD33+, HLA-DR-, CD13++, CD15+, and CD64+) and of immature monocytes (CD34-, CD117-, CD33++, HLA-DR++, CD13-, CD15+, CD64+, and higher CD45), but these cells exhibit almost invariably absence of CD15 and CD64 and decreased expression of CD13 or HLA-DR. In a subset of patients leukemic blasts express the lymphoid markers CD7, CD56 or dim CD19, but are negative for CD5 (174).
In 52% of NPM1 mutated MDS/AML an immature monocytic population was detected, which was positive for HLA-DR, variably positive for CD4, CD15, brightly positive for CD33 and CD64, and negative for CD13, CD14, CD16, CD34, or CD117 (174).
Analysis of the immunophenotype of IDH1/2-mutated AML/MDS-EB cases showed a highly significant association between the simultaneous presence of IDH1/2 and NPM1 mutations and confirmed the double negative ‘acute promyelocytic leukemia-like’ myeloid phenotype described above: lacking both, CD34 and HLA-DR and highly expressing MPO and CD33 (175).
In one study improvement of immunophenotypic features was shown in 41% of MDS patients, treated with azacytidine (AZA) and in 68% of them this was accompanied by a hematological response (176). Immunophenotypic improvement was defined as one of the following: a) normalization or reduction of the number of immunophenotypic abnormalities observed on the myeloid CD34+ population, b) reduction by at least 50% of the percentage of CD34+ myeloid cells pre-AZA and normalization of the granulocytic or monocytic pattern of maturation, c) normalization of the granulocytic and monocytic patterns of maturation (without improvement of the immunophenotype of CD34+ myeloid cells), d) improvement of one maturation pattern (granulocytic or monocytic) and improvement of two of the following: erythroid cells abnormalities, normalization of monocyte percentage or correction of reduced granulation of granulocytes (combination of CD45 and SSC).
This improvement in MFC findings was associated with better clinical response after 6 cycles of AZA treatment and the probability of failure or resistance to AZA was much higher among patients not exhibiting any of these findings. Moreover, improved immunophenotypic features following AZA treatment were found to predict longer duration of hematological response in MDS patients (176).
Integration of genomic and immunophenotypic data is still at an early stage, however the potential for personalized approaches is arising. Genetic alterations with a known clinical impact have transformed clinical practice in certain scenarios. Lenalidomide response rates in lower risk MDS patients with del(5q) are 60-65% and transfusion independence is achieved with a median duration of 2-2.5 years. On the contrary, in the same patient population anemia has shown substantially lower response rates and significantly shorter responses to erythropoietin stimulating agents (ESA), compared with other categories of lower-risk MDS (177). The 5-parameter-del(5q)-score proposed by Oelschlaegel et al. can be used to faster identify these patients before their cytogenetic confirmation, with a predictability of 95% and accelerate appropriate treatment selection (153). MDS-SF3B1 also constitutes a distinct subtype due to its favorable course and responsiveness to luspatercept (156). This entity shows specific immunophenotypic features (138) that could contribute to the generation of a diagnostic score for the rapid and accurate identification of candidates for rapid molecular screening. However, MDS patients with both, SF3B1 mutations and del(5q), exhibit immunophenotypic features more consistent with isolated del(5q) MDS, with decreased numbers of erythroid progenitors, higher CD71 expression and lower CD71 CV, suggesting that these patients may gain benefit from lenalidomide, rather than from luspatercept as first-line treatment (168).
Moreover, coexistence of monosomy 7 and TP53 mutation are mostly related to increased CD14 expression on maturing granulocytic cells and to aberrant expression of T-cell antigens within the myeloid lineage, respectively (167, 172). These adverse genomic abnormalities, even when present alone, are usually enough to categorize patients as high-risk and direct treatment decision towards the use of hypomethylating agents. Immunophenotypic monitoring of lower-risk MDS patients would enable the timely suspicion of disease progression and the initiation of the appropriate treatment.
On the other hand, immunophenotypic patterns can provide prognostic information for treatment response. Thus, patients with lower risk MDS, and ≥3% CD117 expressing erythroid precursors are strongly associated with a favorable response to ESA treatment, transfusion independence and longer progression free survival (PFS) (178). Moreover, it was recently shown that erythroblastic predominance without CD41/cyCD41−positive blasts predicts for a favorable prognosis in MDS/AML patients treated with AZA (179) and that emergence of CD41+ blasts, is often accompanied by disease and/or cytogenetic progression (108). Lastly, for both, AZA and lenalidomide treatment, response monitoring using an immunophenotypic approach has been shown to be feasible (153, 176) and improved immunophenotypic features after AZA were found to predict longer duration of hematological response (176). However, these should be further substantiated and confirmed in prospective clinical studies.
Overall, even though several scoring systems for diagnosis and/or prognosis encompassing both, genomic and immunophenotypic data, the latter have not yet implemented and standardized, but steps are being taken to extract valuable information, that MFC can offer and translate our combined knowledge to clinical benefit for our patients.
The journey from CHIP to pre-leukemic syndromes, such as MDS or MPN, and eventually AML, is driven by the progressive selection of mutated clones, a process known as clonal evolution. Conventional “bulk” sequencing is limited by its ability to only analyze entire- cells populations, inevitably falling short for research in MDS, a disease with heterogenous cell populations and rare blast cells in certain cases. Single-cell technologies appear as ideal tools, both, to investigate the highly connected and plastic immune system, as well as to overcome the above problems, arising from clonality and heterogenicity in MDS (180). The high resolution of single-cell technology allows the study of even small cell groups with shared features, while high statistical power is provided by the large throughput of some sequencing platforms (181, 182).
As far as proteomics are concerned, recent sequencing-based techniques simultaneously quantify cell surface protein and transcriptomic data within a single cell readout (183, 184), thus overcoming the RNA-seq error in predicting protein expression. Moreover, mass spectrometry, using antibodies labeled with heavy metals detected by a mass spectrometer, has the advantage of simultaneous detection of around 40 parameters in each cell for millions of cells (180). This technique is considered suitable for tumor immune microenvironment research, since it can characterize subpopulations of immune cells with previously unknown alterations, and it can offer unbiased identification of HLA-neoantigens (185). Behbehani et al. attempted to depict the profile of MDS with mass spectrometry and they reported that it enabled the detection of aberrant surface markers at high resolution, detecting aberrancies in 27/31 surface markers, encompassing almost every previously reported MDS surface marker aberrancy (186).
Epigenomics have also recently adapted to single-cell applications, enabling the elucidation of DNA-methylation patterns, chromatin regions available for transcription factors activity, chromosomal conformation and histone modifications/binding sites in even thousands of individual cells. However, these methods are limited to cover specific regulatory regions (180).
On the other hand, MFC is a well-established technique but has not yet reached its full potential in MDS. AI, machine learning algorithms and other automated clustering techniques, trained from well-defined patient data, are being applied to large datasets enhancing diagnostic accuracy, providing repeatability, objectivity and a high sensitivity and specificity in detecting MDS (107, 187) or even highlight the predictive value of the detection of dysplasia for the response to treatment in AML cases (188) and could prove a new asset in everyday clinical practice in the future.
Even though excessive studies in the field show promising results for the broadened application of MFC scores in routine clinical practice, the standardization of instruments and panels is far from being universally achieved and thus MFC in MDS is still a field of investigation for the implementation of suggested strategies and algorithms in routine clinical practice for the bigger part of the world. Either due to financial reasons or the questioning of the value of extensive MFC panels and time consuming analyses or the lack of expertise in some cases MFC is still heterogeneously used in the diagnostic process or follow up of MDS and suspected MDS cases, acknowledged only as a complementary tool for specific cases (177). Furthermore, the luxury of time in general when investigating suspected MDS; in contrast to AML where MFC is an established diagnostic method mainly for the rapid results, allows for the waiting of the molecular or cytogenetic results to confirm the clonal hematopoiesis. The heterogeneity of the disease, along with the similarities it exhibits with other clinical entities resulting in faulty hematopoiesis, is a co-founding factor for clinicians to rely on more definite tests (such as molecular or cytogenetic alterations) rather than immunophenotypic patterns indicative of dysplasia. Davydova et al (189) has reported that deviations of MFC parameters found in the control group can decrease the specificity of MFC scores when detecting dysplasia. Patients with PNH had increased levels of CD34+CD7+ myeloid cells. Aplastic anemia and PNH were characterized by a high proportion of CD56+ cells among CD34+ precursors and neutrophils. A second problem is that the proportion of MDS-related features increased with the progression of MDS, meaning that flow cytometry can detect more dysplastic immunophenotypic aberrancies when the disease is generally easier to detect. The highest number of CD34+ blasts was found in MDS with excess blasts. On the contrary, in 39 low-grade MDS – in the category where the most borderline cases belong, the sensitivities were 53.8%, 61.5%, and 71.8% for Ogata score, Wells score, and iFS, respectively, not addressing adequately the diagnostic problem that clinicians face when they investigate a suspected MDS case with rare blast cells.
Currently, both, genomic profiling and MFC are in the quiver of the physician for both, diagnosis and prognosis, but since NGS is still a high-cost technique and low-income countries are struggling to adapt (190), the association of specific phenotypic patterns with driver mutations in MDS, could serve as an indication to guide the selection of patients for mutational screening, in an effort to achieve optimal risk stratification.
MFC is a standard of care in the screening of patients with cytopenia, with or without dysplasia. For more than a decade, recommendations from the European LeukemiaNet have incorporated MFC as an additional tool in the diagnostic workup of MDS, compensating for the lack of specificity and repeatability in the morphological evaluation of dysplastic features (191). Bacher et al. showed that clonal chromosomal aberrations were detected in 14.3% of patients without suspicion of MDS by cytomorphologic evaluation during diagnostic work up, demonstrating the need of MFC to exclude MDS when the diagnosis is not confirmed by cytomorphological criteria (192). Various MFC scores have demonstrated up to 80% accuracy in discriminating between MDS and other nonclonal conditions with dysplastic morphological features (146). Further studies have highlighted the association of specific immunophenotypic patterns with cytogenetic or molecular findings, suggesting the ability of MFC to raise suspicion for the existence of specific genetic markers thus guiding further testing and accelerating the establishment of the precise diagnosis perhaps in a more cost effective manner.
On the other hand, the recognition of the prognostic importance of specific immunophenotypic aberrancies, and their incorporation in a composite prognostic scoring system, including clinical, biological and genetic prognostic parameters might further increase the accuracy of patient’s prognostic categorization and treatment response prediction. The integration of prognostically adverse mutational profiles - detected with NGS or other genetic testing- and myelodysplastic features - assessed by MFC scoring- has been implemented in other neoplasms such as systemic mastocytosis and has redefined prognostically different patient groups (193). Although the genetic landscape of MDS is broader and thus, a similar approach is more difficult to be implemented, advances in both the molecular characterization of the syndromes and the technical limitations of MFC analysis might overcome current difficulties in the future. The two methodologies are rather complementary and enable the physician to elaborate the complex phenotype of the disease. To that end, various groups have reported that the distribution of VAFs of individually mutated genes did not correlate with blast percentages in cases of MDS and AML, suggesting that VAF and blast percentage are different metrics of leukemic burden and thus both contribute differently to accurate clinical evaluation (194, 195).
Possibly, the establishment of new technologies and specifically of single cell – omics and a broadened implementation of machine learning algorithms for the analysis of merged data will bridge the gap between genotype and phenotype in the future and advance both, our understanding of the disease and our clinical practice. Until then, MDS poses a complex and delicate clinical challenge and MFC has proven a useful and “handy” tool for everyday screening of suspected MDS patients and an invaluable test for individual cases with no other pathognomonic findings to establish the diagnosis and guide further testing (Figure 2).
Figure 2. Schematic depiction of the interplay of MFC findings with cytogenetic and molecular testing in routine clinical practice. Clonal hematopoiesis is detected in more than 90% of MDS cases by cytogenetic or molecular alterations. These findings will establish the diagnosis but in terms of patient management, only certain disease-defining genetic alterations will constitute the basis of re-stratification of patients and individualize their treatment plan. On the other hand, MFC is currently used to investigate cytopenias and detect the cases in need for further cytogenetic or molecular testing. MFC can confirm or establish the diagnosis of MDS and guide clinical practice in cases with excess of blasts or patients with suspicion of MDS and no MDS-related genomic alterations.
EV: Conceptualization, Investigation, Project administration, Visualization, Writing – original draft, Writing – review & editing. TC: Writing – original draft, Writing – review & editing. VL: Visualization, Writing – original draft, Writing – review & editing. A-LD: Conceptualization, Writing – original draft, Writing – review & editing. AS: Conceptualization, Funding acquisition, Writing – original draft, Writing – review & editing.
The author(s) declare financial support was received for the research, authorship, and/or publication of this article. Funds from the Special Account for Research Funds (ELKE) of University of Patras will cover publication fees [grant number 40620000: “Research of the epidemiology and biological characteristics of myelodysplastic syndromes in Southwestern Greece”].
The authors declare that the research was conducted in the absence of any commercial or financial relationships that could be construed as a potential conflict of interest.
The author(s) declared that they were an editorial board member of Frontiers, at the time of submission. This had no impact on the peer review process and the final decision
All claims expressed in this article are solely those of the authors and do not necessarily represent those of their affiliated organizations, or those of the publisher, the editors and the reviewers. Any product that may be evaluated in this article, or claim that may be made by its manufacturer, is not guaranteed or endorsed by the publisher.
ABCB7: ATP Binding Cassette Subfamily B Member 7
AI: Artificial intelligence
ALL: Acute lymphoblastic leukemia
AML: Acute myelogenous leukemia
ASXL1: Additional sex combs-like 1
BCOR: BCL6 corepressor
BM: Bone marrow
CBL: Casitas B-lineage Lymphoma
CCUS: Clonal cytopenia of undetermined significance
CHIP: Clonal hematopoiesis of indeterminate potential
CLL: Chronic lymphocytic leukemia
CMML: Chronic myelomonocytic leukemia
CSNK1A1: Casein kinase 1 alpha 1
CV: Coefficient of variation
DDX41: DEAD-box RNA helicase-1 gene
DMI: Dysmyelopoiesis flow score
DNMT3A: DNA methyltransferase 3A
EIF2AK1: Eukaryotic translation initiation factor 2-alpha kinase 1
ELN: European LeukemiaNet
EPO: Erythropoietin
ESA: Erythropoietin stimulating agents
ETV6: ETS variant transcription factor 6
EuroFlow: EuroFlow Consortium
EZH2: Enhancer of zeste homolog 2
FAB: French-American-British
FCSS: Flow cytometry scoring system
FISH: Fluorescence in situ hybridization
FLAER: Fluorescein-labeled proaerolysin
Fli1: Friend leukemia virus integration 1
FlowSOM: Flow-Self Organizing Maps algorithm
FLT3: Fms-like tyrosine kinase 3
GATA1/2: GATA-binding factor ½
GPI: Glycosylphosphatidylinositol
GPIIb: Glycoprotein IIb
HLA: Human leukocyte antigens
HSCT: Hematopoietic stem cell transplantation
ICC: International Consensus Classification
ICUS: Idiopathic cytopenia of undetermined significance
IDH1/IDH2: Isocitrate dehydrogenase 1/2
IDUS: Idiopathic dysplasia of unknown significance
iFS: Integrated flow cytometric score
iMDS Flow: International MDS Flow Working Group
IPSS: International prognostic scoring system
IPSS-M: Molecular IPSS
IPSS-R: Revised IPSS
JAK2: Janus kinase 2
KDM6A: Lysine demethylase 6A
KRAS/NRAS: Kirsten/neuroblastoma rat sarcoma viral oncogene homolog
LAIP: Leukemia associated immunophenotype
LF: Lactoferrin
LFS: Leukemia free survival LFS
LOH: Loss of heterozygosity
MDS: Myelodysplastic syndromes/neoplasms
MDS/MPN: Myelodysplastic/myeloproliferative neoplasms
MDS-biTP53: MDS with biallelic TP53 inactivation
MDS-EB: MDS with excess blasts
MDS-SF3B1: MDS with low blasts and SF3B1 mutation
MFC: Multiparameter flow cytometry
MFI: Mean fluorescent intensity
MLL: Mixed-lineage leukemia
MPN: Myeloproliferative neoplasms
MPO: Myeloperoxidase
MRD: Measurable clonal residual disease
NGS: Next Generation Sequencing
NPM1: Nucleophosmin 1
OS: Overall survival
PCNA: Proliferating cell nuclear antigen
PFS: Progression free survival
PNH: Paroxysmal nocturnal hemoglobinuria
PPOX: Protoporphyrinogen oxidase
RBC: Red blood cells
RNA-seq: RNA sequencing
RPS14: Ribosomal Protein S14
RUNX1: Runt-related transcription factor 1
SF3B1: Splicing factor 3B unit 1
SRFS2: Serine and arginine-rich splicing factor 2
SSC: Side scatter
STAG2: Stromal antigen 2
TET2: Tet methylcytosine dioxygenase 2
Th17: T helper type 17 cells
Th22: T helper type 22 cells
TP53: Tumor protein p53
Tregs: Regulatory T cells
U2AF1: U2 small nuclear RNA auxiliary factor 1
UBA1: Ubiquitin like modifier activating enzyme 1
VAF: Variant allele frequency
WHO: World Health Organization
WPSS: WHO classification based prognostic scoring system
WT1: Wilms tumor 1
ZRSR2: Zinc finger CCCH-type, RNA binding motif and serine/arginine rich 2
1. Tefferi A, Vardiman JW. Myelodysplastic syndromes. New Engl J Med. (2009) 361:1872–85. doi: 10.1056/NEJMra0902908
2. Cazzola M. Myelodysplastic syndromes. New Engl J Med. (2020) 383:1358–74. doi: 10.1056/NEJMra1904794
3. Sekeres MA, Taylor J. Diagnosis and treatment of myelodysplastic syndromes: A review. JAMA. (2022) 328:872–80. doi: 10.1001/jama.2022.14578
4. de Roij van Zuijdewijn CLM, Westerweel PE, Schipperus MR, Pruijt JFM, van de Loosdrecht AA, Beeker A. Prevalence and treatment of anemia and secondary iron overload in patients with a myelodysplastic syndrome: Real-world data from a multicenter cohort study. Transfus Clin Biol. (2023) 30:314–8. doi: 10.1016/j.tracli.2023.04.001
5. Kaka S, Jahangirnia A, Beauregard N, Davis A, Tinmouth A, Chin-Yee N. Red blood cell transfusion in myelodysplastic syndromes: A systematic review. Transfus Med. (2022) 32:3–23. doi: 10.1111/tme.12841
6. Fozza C, Murtas A, Caocci G, La Nasa G. Autoimmune disorders associated with myelodysplastic syndromes: clinical, prognostic and therapeutic implications. Leukemia Res. (2022) 117:106856. doi: 10.1016/j.leukres.2022.106856
7. Grignano E, Jachiet V, Fenaux P, Ades L, Fain O, Mekinian A. Autoimmune manifestations associated with myelodysplastic syndromes. Ann Hematol. (2018) 97:2015–23. doi: 10.1007/s00277-018-3472-9
8. Alonso-Fernandez-Gatta M, Martin-Garcia A, Martin-Garcia AC, Lopez-Cadenas F, Diaz-Pelaez E, Jimenez-Solas T, et al. Predictors of cardiovascular events and all-cause of death in patients with transfusion-dependent myelodysplastic syndrome. Br J Haematol. (2021) 195:536–41. doi: 10.1111/bjh.17652
9. Vardiman JW, Harris NL, Brunning RD. The World Health Organization (WHO) classification of the myeloid neoplasms. Blood. (2002) 100:2292–302. doi: 10.1182/blood-2002-04-1199
10. Vardiman JW, Thiele J, Arber DA, Brunning RD, Borowitz MJ, Porwit A, et al. The 2008 revision of the World Health Organization (WHO) classification of myeloid neoplasms and acute leukemia: rationale and important changes. Blood. (2009) 114:937–51. doi: 10.1182/blood-2009-03-209262
11. Arber DA, Orazi A, Hasserjian R, Thiele J, Borowitz MJ, Le Beau MM, et al. The 2016 revision to the World Health Organization classification of myeloid neoplasms and acute leukemia. Blood. (2016) 127:2391–405. doi: 10.1182/blood-2016-03-643544
12. Arber DA, Orazi A, Hasserjian RP, Borowitz MJ, Calvo KR, Kvasnicka H-M, et al. International Consensus Classification of Myeloid Neoplasms and Acute Leukemias: integrating morphologic, clinical, and genomic data. Blood. (2022) 140:1200–28. doi: 10.1182/blood.2022015850
13. Khoury JD, Solary E, Abla O, Akkari Y, Alaggio R, Apperley JF, et al. The 5th edition of the world health organization classification of haematolymphoid tumours: myeloid and histiocytic/dendritic neoplasms. Leukemia. (2022) 36:1703–19. doi: 10.1038/s41375-022-01613-1
14. Craig FE, Foon KA. Flow cytometric immunophenotyping for hematologic neoplasms. Blood. (2008) 111:3941–67. doi: 10.1182/blood-2007-11-120535
15. Riva G, Nasillo V, Ottomano AM, Bergonzini G, Paolini A, Forghieri F, et al. Multiparametric flow cytometry for MRD monitoring in hematologic Malignancies: clinical applications and new challenges. Cancers (Basel). (2021) 13:4582. doi: 10.3390/cancers13184582
16. Cherian S, Soma LA. How I diagnose minimal/measurable residual disease in B lymphoblastic leukemia/lymphoma by flow cytometry. Am J Clin Pathol. (2021) 155:38–54. doi: 10.1093/ajcp/aqaa242
17. Lucas F, Hergott CB. Advances in acute myeloid leukemia classification, prognostication and monitoring by flow cytometry. Clin Lab Med. (2023) 43:377–98. doi: 10.1016/j.cll.2023.04.005
18. Chen X, Cherian S. Acute myeloid leukemia immunophenotyping by flow cytometric analysis. Clin Lab Med. (2017) 37:753–69. doi: 10.1016/j.cll.2017.07.003
19. Craig FE. Flow cytometric evaluation of B-cell lymphoid neoplasms. Clin Lab Med. (2007) 27:487–512. doi: 10.1016/j.cll.2007.05.003
20. DiGiuseppe JA, Wood BL. Applications of flow cytometric immunophenotyping in the diagnosis and posttreatment monitoring of B and T lymphoblastic leukemia/lymphoma. Cytometry B Clin Cytom. (2019) 96:256–65. doi: 10.1002/cyto.b.21833
21. Porwit A, Béné MC. Multiparameter flow cytometry applications in the diagnosis of mixed phenotype acute leukemia. Cytometry B Clin Cytom. (2019) 96:183–94. doi: 10.1002/cyto.b.21783
22. Voso MT, Ottone T, Lavorgna S, Venditti A, Maurillo L, Lo-Coco F, et al. MRD in AML: the role of new techniques. Front Oncol. (2019) 9:655. doi: 10.3389/fonc.2019.00655
23. Wijnands C, Noori S, Donk NWCJV, VanDuijn MM, Jacobs JFM. Advances in minimal residual disease monitoring in multiple myeloma. Crit Rev Clin Lab Sci. (2023) 60:518–34. doi: 10.1080/10408363.2023.2209652
24. D’Arena G, Sgambato A, Volpe S, Coppola G, Amodeo R, Tirino V, et al. Flow cytometric evaluation of measurable residual disease in chronic lymphocytic leukemia: Where do we stand? Hematological Oncol. (2022) 40:835–42. doi: 10.1002/hon.3037
25. Wang W, Khoury JD. Where diagnosis for myelodysplastic neoplasms ( MDS ) stands today and where it will go: The role of flow cytometry in evaluation of MDS. Cytometry Part B Clin. (2023) 104:12–4. doi: 10.1002/cyto.b.22110
26. Bento LC, Correia RP, Pitangueiras Mangueira CL, De Souza Barroso R, Rocha FA, Bacal NS, et al. The use of flow cytometry in myelodysplastic syndromes: A review. Front Oncol. (2017) 7:270. doi: 10.3389/fonc.2017.00270
27. Aanei CM, Picot T, Tavernier E, Guyotat D, Campos Catafal L. Diagnostic utility of flow cytometry in myelodysplastic syndromes. Front Oncol. (2016) 6:161. doi: 10.3389/fonc.2016.00161
28. Porwit A, Béné MC, Duetz C, Matarraz S, Oelschlaegel U, Westers TM, et al. Multiparameter flow cytometry in the evaluation of myelodysplasia: Analytical issues: Recommendations from the European LeukemiaNet/International Myelodysplastic Syndrome Flow Cytometry Working Group. Cytometry Part B Clin. (2023) 104:27–50. doi: 10.1002/cyto.b.22108
29. Ogata K, Porta MGD, Malcovati L, Picone C, Yokose N, Matsuda A, et al. Diagnostic utility of flow cytometry in low-grade myelodysplastic syndromes: a prospective validation study. Haematologica. (2009) 94:1066–74. doi: 10.3324/haematol.2009.008532
30. Verigou E, Lampropoulou P, Smyrni N, Kolliopoulou G, Sakellaropoulos G, Starakis I, et al. Evaluation of a bone marrow dysmyelopoiesis immunophenotypic index for the diagnosis and prognosis of myelodysplastic syndromes. Cardiovasc Hematol Disord Drug Targets. (2015) 15:148–61. doi: 10.2174/1871529x15666150701105822
31. Cremers EMP, Westers TM, Alhan C, Cali C, Visser-Wisselaar HA, Chitu DA, et al. Implementation of erythroid lineage analysis by flow cytometry in diagnostic models for myelodysplastic syndromes. Haematologica. (2017) 102:320–6. doi: 10.3324/haematol.2016.147843
32. Oelschlaegel U, Oelschlaeger L, Von Bonin M, Kramer M, Sockel K, Mohr B, et al. Comparison of five diagnostic flow cytometry scores in patients with myelodysplastic syndromes: Diagnostic power and prognostic impact. Cytometry Part B Clin. (2023) 104:141–50. doi: 10.1002/cyto.b.22030
33. Hoff FW, Madanat YF. Molecular drivers of myelodysplastic neoplasms (MDS)—Classification and prognostic relevance. Cells. (2023) 12:627. doi: 10.3390/cells12040627
34. Bernard E, Tuechler H, Greenberg PL, Hasserjian RP, Arango Ossa JE, Nannya Y, et al. Molecular international prognostic scoring system for myelodysplastic syndromes. NEJM Evidence. (2022) 1:EVIDoa2200008. doi: 10.1056/EVIDoa2200008
35. Kontandreopoulou C-N, Kalopisis K, Viniou N-A, Diamantopoulos P. The genetics of myelodysplastic syndromes and the opportunities for tailored treatments. Front Oncol. (2022) 12:989483. doi: 10.3389/fonc.2022.989483
36. Stahl M, Bewersdorf JP, Xie Z, Porta MGD, Komrokji R, Xu ML, et al. Classification, risk stratification and response assessment in myelodysplastic syndromes/neoplasms (MDS): A state-of-the-art report on behalf of the International Consortium for MDS (icMDS). Blood Rev. (2023) 62:101128. doi: 10.1016/j.blre.2023.101128
37. Schlegelberger B, Göhring G, Thol F, Heuser M. Update on cytogenetic and molecular changes in myelodysplastic syndromes. Leuk Lymphoma. (2012) 53:525–36. doi: 10.3109/10428194.2011.618235
38. Hosono N. Genetic abnormalities and pathophysiology of MDS. Int J Clin Oncol. (2019) 24:885–92. doi: 10.1007/s10147-019-01462-6
39. Malcovati L, Porta MGD, Pascutto C, Invernizzi R, Boni M, Travaglino E, et al. Prognostic factors and life expectancy in myelodysplastic syndromes classified according to WHO criteria: A basis for clinical decision making. JCO. (2005) 23:7594–603. doi: 10.1200/JCO.2005.01.7038
40. Krause R, Penchansky L, Contis L, Kaplan SS. Flow cytometry in the diagnosis of acute leukemia. Am J Clin Pathol. (1988) 89:341–6. doi: 10.1093/ajcp/89.3.341
41. Terstappen LW, Loken MR. Myeloid cell differentiation in normal bone marrow and acute myeloid leukemia assessed by multi-dimensional flow cytometry. Anal Cell Pathol. (1990) 2:229–40.
42. Miura I, Kobayashi Y, Takahashi N, Saitoh K, Miura AB. Involvement of natural killer cells in patients with myelodysplastic syndrome carrying monosomy 7 revealed by the application of fluorescence in situ hybridization to cells collected by means of fluorescence-activated cell sorting. Br J Haematol. (2000) 110:876–9. doi: 10.1046/j.1365-2141.2000.02294.x
43. Ohsaka A, Saionji K, Igari J, Watanabe N, Iwabuchi K, Nagaoka I. Altered surface expression of effector cell molecules on neutrophils in myelodysplastic syndromes. Br J Haematol. (1997) 98:108–13. doi: 10.1046/j.1365-2141.1997.1873007.x
44. Clark RE, Smith SA, Jacobs A. Myeloid surface antigen abnormalities in myelodysplasia: relation to prognosis and modification by 13-cis retinoic acid. J Clin Pathol. (1987) 40:652–6. doi: 10.1136/jcp.40.6.652
45. Riccardi A, Montecucco CM, Danova M, Ucci G, Mazzini G, Giordano PA, et al. Flow cytometric evaluation of proliferative activity and ploidy in myelodysplastic syndromes and acute leukemias. Basic Appl Histochem. (1986) 30:181–92.
46. Maiolo AT, Foa P, Mozzana R, Chiorboli O, Ciani A, Maisto A, et al. Flow cytometric analysis of cellular DNA in human acute nonlymphatic leukemias and dysmyelopoietic syndromes. Cytometry. (1982) 2:265–7. doi: 10.1002/cyto.990020411
47. Hoy TG, Geddes AD, Jacobs A. DNA index and karyotype analysis in myelodysplasia. J Clin Pathol. (1989) 42:498–501. doi: 10.1136/jcp.42.5.498
48. Clark R, Peters S, Hoy T, Smith S, Whittaker K, Jacobs A. Prognostic importance of hypodiploid hemopoietic precursors in myelodysplastic syndromes. N Engl J Med. (1986) 314:1472–5. doi: 10.1056/NEJM198606053142302
49. Jensen IM, Hokland M, Hokland P. A quantitative evaluation of erythropoiesis in myelodysplastic syndromes using multiparameter flow cytometry. Leuk Res. (1993) 17:839–46. doi: 10.1016/0145-2126(93)90149-f
50. Jensen IM. Myelopoiesis in myelodysplasia evaluated by multiparameter flow cytometry. Leukemia Lymphoma. (1995) 20:17–25. doi: 10.3109/10428199509054749
51. Kanter-Lewensohn L, Hellström-Lindberg E, Kock Y, Elmhorn-Rosenborg A, Ost A. Analysis of CD34-positive cells in bone marrow from patients with myelodysplastic syndromes and acute myeloid leukemia and in normal individuals: a comparison between FACS analysis and immunohistochemistry. Eur J Haematol. (1996) 56:124–9. doi: 10.1111/j.1600-0609.1996.tb01330.x
52. Kobayashi Y, Kimura S, Tanaka K, Wada K, Ozawa M, Horiuchi H, et al. Shift in the megakaryocyte ploidy in MDS patients: microcytofluorometry with DAPI staining after destaining of Wright-Giemsa stain. Br J Haematol. (1991) 79:556–61. doi: 10.1111/j.1365-2141.1991.tb08081.x
53. Zhang YZ, WM DA, Huang WR, Gao CJ, Guo B. Bone marrow mesenchymal stem cells derived from patients with myelodysplastic syndrome possess immunosuppressive activity. Zhongguo Shi Yan Xue Ye Xue Za Zhi. (2007) 15:302–5.
54. Nakaseko C, Asai T, Wakita H, Oh H, Saito Y. Signalling defect in FMLP-induced neutrophil respiratory burst in myelodysplastic syndromes. Br J Haematol. (1996) 95:482–8. doi: 10.1111/j.1365-2141.1996.tb08992.x
55. Nakahashi K, Mishima K, Ichikawa Y, Watanabe K, Komatsuda M, Arimori S. Report of a case with chronic myelomonocytic leukemia: demonstration of leukemic monocytes lacking nonspecific esterase by flow cytometry using monoclonal antibodies. Tokai J Exp Clin Med. (1987) 12:275–81.
56. Carulli G, Sbrana S, Minnucci S, Azzarà A, Angiolini C, Gullaci AR, et al. Actin polymerization in neutrophils from patients affected by myelodysplastic syndromes-a flow cytometric study. Leuk Res. (1997) 21:513–8. doi: 10.1016/s0145-2126(97)00009-x
57. Park SJ, Min WS, Yang IH, Kim HJ, Min CK, Eom HS, et al. Effects of mixed chimerism and immune modulation on GVHD, disease recurrence and survival after HLA-identical marrow transplantation for hematologic Malignancies. Korean J Intern Med. (2000) 15:224–31. doi: 10.3904/kjim.2000.15.3.224
58. Tsurumi H, Shimazaki M, Takahashi T, Moriwaki H, Muto Y. Flow cytometric determination of active oxygen (hydroperoxide) produced by peripheral blood neutrophils in patients with hematological disorders. Int J Hematol. (1993) 57:213–9.
59. Prodan M, Tulissi P, Perticarari S, Presani G, Franzin F, Pussini E, et al. Flow cytometric assay for the evaluation of phagocytosis and oxidative burst of polymorphonuclear leukocytes and monocytes in myelodysplastic disorders. Haematologica. (1995) 80:212–8.
60. Ogata K, An E, Kamikubo K, Tamura H, Yokose N, Dan K, et al. Cell cycle modulation by hematopoietic growth factors in myelodysplastic syndromes: analysis by three-color flow cytometry. Exp Hematol. (1997) 25:8–18.
61. Darley RL, Hoy TG, Baines P, Padua RA, Burnett AK. Mutant N-RAS induces erythroid lineage dysplasia in human CD34+ cells. J Exp Med. (1997) 185:1337–47. doi: 10.1084/jem.185.7.1337
62. Danova M, Giordano M, Mazzini G, Riccardi A. Expression of p53 protein during the cell cycle measured by flow cytometry in human leukemia. Leuk Res. (1990) 14:417–22. doi: 10.1016/0145-2126(90)90027-7
63. Iwase O, Aizawa S, Kuriyama Y, Yaguchi M, Nakano M, Toyama K. Analysis of bone marrow and peripheral blood immunoregulatory lymphocytes in patients with myelodysplastic syndrome. Ann Hematol. (1995) 71:293–9. doi: 10.1007/BF01697982
64. Maciejewski JP, Hibbs A JR SK. P Y, N.S. Bone marrow and peripheral blood lymphocyte phenotype in patients with bone marrow failure. Exp Hematol. (1994) 22:1102–10.
65. Pallis M, Syan J, Russell NH. Flow cytometric chemosensitivity analysis of blasts from patients with acute myeloblastic leukemia and myelodysplastic syndromes: the use of 7AAD with antibodies to CD45 or CD34. Cytometry. (1999) 37:308–13. doi: 10.1002/(sici)1097-0320(19991201)37:4<308::aid-cyto8>3.0.co;2-z
66. Valent P, Horny H-P. Minimal diagnostic criteria for myelodysplastic syndromes and separation from ICUS and IDUS: update and open questions. Eur J Clin Invest. (2009) 39:548–53. doi: 10.1111/j.1365-2362.2009.02151.x
67. Loken MR, Van De Loosdrecht A, Ogata K, Orfao A, Wells DA. Flow cytometry in myelodysplastic syndromes: Report from a working conference. Leukemia Res. (2008) 32:5–17. doi: 10.1016/j.leukres.2007.04.020
68. Elghetany MT. Surface marker abnormalities in myelodysplastic syndromes. Haematologica (1998) 83:1104–15
69. Van De Loosdrecht AA, Alhan C, Bene MC, Della Porta MG, Drager AM, Feuillard J, et al. Standardization of flow cytometry in myelodysplastic syndromes: report from the first European LeukemiaNet working conference on flow cytometry in myelodysplastic syndromes. Haematologica. (2009) 94:1124–34. doi: 10.3324/haematol.2009.005801
70. Del Cañizo MC, Fernández ME, López A, Vidriales B, Villarón E, Arroyo JL, et al. Immunophenotypic analysis of myelodysplastic syndromes. Haematologica. (2003) 88:402–7.
71. Ossenkoppele GJ, Van De Loosdrecht AA, Schuurhuis GJ. Review of the relevance of aberrant antigen expression by flow cytometry in myeloid neoplasms. Br J Haematol. (2011) 153:421–36. doi: 10.1111/j.1365-2141.2011.08595.x
72. Della Porta MG, Lanza F, Del Vecchio L, for the Italian Society of Cytometry (GIC). Flow cytometry immunophenotyping for the evaluation of bone marrow dysplasia. Cytometry Part B Clin. (2011) 80:201–11. doi: 10.1002/cyto.b.20607
73. Satoh C, Dan K, Yamashita T, Jo R, Tamura H, Ogata K. Flow cytometric parameters with little interexaminer variability for diagnosing low-grade myelodysplastic syndromes. Leukemia Res. (2008) 32:699–707. doi: 10.1016/j.leukres.2007.08.022
74. Rothe G, Schmitz G. Consensus protocol for the flow cytometric immunophenotyping of hematopoietic Malignancies. Working Group on Flow Cytometry and Image Analysis. Leukemia. (1996) 10:877–95.
75. Westers TM, Ireland R, Kern W, Alhan C, Balleisen JS, Bettelheim P, et al. Standardization of flow cytometry in myelodysplastic syndromes: a report from an international consortium and the European LeukemiaNet Working Group. Leukemia. (2012) 26:1730–41. doi: 10.1038/leu.2012.30
76. on behalf of the EuroFlow Consortium (EU-FP6, LSHB-CT-2006-018708), Van Dongen JJM, Lhermitte L, Böttcher S, Almeida J, van der Velden VHJ, et al. EuroFlow antibody panels for standardized n-dimensional flow cytometric immunophenotyping of normal, reactive and Malignant leukocytes. Leukemia. (2012) 26:1908–75. doi: 10.1038/leu.2012.120
77. Kern W, Westers TM, Bellos F, Bene MC, Bettelheim P, Brodersen LE, et al. Multicenter prospective evaluation of diagnostic potential of flow cytometric aberrancies in myelodysplastic syndromes by the ELN iMDS flow working group. Cytometry Part B Clin. (2023) 104:51–65. doi: 10.1002/cyto.b.22105
78. Vido-Marques JR, Reis-Alves SC, Saad STO, Metze K, Lorand-Metze I. A simple score derived from bone marrow immunophenotyping is important for prognostic evaluation in myelodysplastic syndromes. Sci Rep. (2020) 10:20281. doi: 10.1038/s41598-020-77158-z
79. Alayed K, Meyerson JB, Osei ES, Blidaru G, Schlegelmilch J, Johnson M, et al. CD177 enhances the detection of myelodysplastic syndrome by flow cytometry. Am J Clin Pathol. (2020) 153:554–65. doi: 10.1093/ajcp/aqz196
80. Duetz C, Westers TM, van de Loosdrecht AA. Clinical implication of multi-parameter flow cytometry in myelodysplastic syndromes. Pathobiology. (2019) 86:14–23. doi: 10.1159/000490727
81. Muyldermans A, Florin L, Devos H, Cauwelier B, Emmerechts J. Diagnostic utility of the lymphoid screening tube supplemented with CD34 for Ogata score calculation in patients with peripheral cytopenia. Hematology. (2019) 24:166–72. doi: 10.1080/10245332.2018.1535536
82. Park S, Kosmider O, Maloisel F, Drenou B, Chapuis N, Lefebvre T, et al. Dyserythropoiesis evaluated by the RED score and hepcidin : ferritin ratio predicts response to erythropoietin in lower-risk myelodysplastic syndromes. Haematologica. (2019) 104:497–504. doi: 10.3324/haematol.2018.203158
83. Mathis S, Chapuis N, Debord C, Rouquette A, Radford-Weiss I, Park S, et al. Flow cytometric detection of dyserythropoiesis: a sensitive and powerful diagnostic tool for myelodysplastic syndromes. Leukemia. (2013) 27:1981–7. doi: 10.1038/leu.2013.178
84. Westers TM, Cremers EMP, Oelschlaegel U, Johansson U, Bettelheim P, Matarraz S, et al. Immunophenotypic analysis of erythroid dysplasia in myelodysplastic syndromes. A report from the IMDSFlow working group. Haematologica. (2017) 102:308–19. doi: 10.3324/haematol.2016.147835
85. Porta MGD, Picone C, Pascutto C, Malcovati L, Tamura H, Handa H, et al. Multicenter validation of a reproducible flow cytometric score for the diagnosis of low-grade myelodysplastic syndromes: results of a European LeukemiaNET study. Haematologica. (2012) 97:1209–17. doi: 10.3324/haematol.2011.048421
86. Ogata K, Sei K, Saft L, Kawahara N, Porta MGD, Chapuis N, et al. Revising flow cytometric mini-panel for diagnosing low-grade myelodysplastic syndromes: Introducing a parameter quantifying CD33 expression on CD34+ cells. Leukemia Res. (2018) 71:75–81. doi: 10.1016/j.leukres.2018.07.009
87. Wells DA, Benesch M, Loken MR, Vallejo C, Myerson D, Leisenring WM, et al. Myeloid and monocytic dyspoiesis as determined by flow cytometric scoring in myelodysplastic syndrome correlates with the IPSS and with outcome after hematopoietic stem cell transplantation. Blood. (2003) 102:394–403. doi: 10.1182/blood-2002-09-2768
88. Ogata K, Kishikawa Y, Satoh C, Tamura H, Dan K, Hayashi A. Diagnostic application of flow cytometric characteristics of CD34+ cells in low-grade myelodysplastic syndromes. Blood. (2006) 108:1037–44. doi: 10.1182/blood-2005-12-4916
89. Bardet V, Wagner-Ballon O, Guy J, Morvan C, Debord C, Trimoreau F, et al. Multicentric study underlining the interest of adding CD5, CD7 and CD56 expression assessment to the flow cytometric Ogata score in myelodysplastic syndromes and myelodysplastic/myeloproliferative neoplasms. Haematologica. (2015) 100:472–8. doi: 10.3324/haematol.2014.112755
90. Kárai B, Bedekovics J, Miltényi Z, Gergely L, Szerafin L, Ujfalusi A, et al. A single-tube flow cytometric procedure for enhancing the diagnosis and prognostic classification of patients with myelodysplastic syndromes. Int J Lab Hematol. (2017) 39:577–84. doi: 10.1111/ijlh.12700
91. Grille Montauban S, Hernandez-Perez CR, Velloso EDRP, Novoa V, Lorand-Metze I, Gonzalez J, et al. Flow cytometry “Ogata score” for the diagnosis of myelodysplastic syndromes in a real-life setting. A Latin American experience. Int J Lab Hematol. (2019) 41:536–41. doi: 10.1111/ijlh.13047
92. Porwit A, van de Loosdrecht AA, Bettelheim P, Brodersen LE, Burbury K, Cremers E, et al. Revisiting guidelines for integration of flow cytometry results in the WHO classification of myelodysplastic syndromes—proposal from the International/European LeukemiaNet Working Group for Flow Cytometry in MDS. Leukemia. (2014) 28:1793–8. doi: 10.1038/leu.2014.191
93. Lu Y, Chen X, Zhang L. CD36 relative mean fluorescence intensity of CD105+ nucleated erythroid cells can be used to differentiate myelodysplastic syndrome from megaloblastic anemia. Sci Rep. (2023) 13:8930. doi: 10.1038/s41598-023-35994-9
94. Violidaki D, Axler O, Jafari K, Bild F, Nilsson L, Mazur J, et al. Analysis of erythroid maturation in the nonlysed bone marrow with help of radar plots facilitates detection of flow cytometric aberrations in myelodysplastic syndromes. Cytometry Part B Clin. (2020) 98:399–411. doi: 10.1002/cyto.b.21931
95. Johansson U, McIver-Brown N, Cullen M, Duetz C, Dunlop A, Oelschlägel U, et al. The flow cytometry myeloid progenitor count: A reproducible parameter for diagnosis and prognosis of myelodysplastic syndromes. Cytometry Part B Clin. (2023) 104:115–27. doi: 10.1002/cyto.b.22048
96. Aldawood A, Kinkade Z, Rosado F, Esan O, Gibson L, Vos J. A novel method to assess bone marrow purity is useful in determining blast percentage by flow cytometry in acute myeloid leukemia and myelodysplasia. Ann Hematol Oncol (2015) 2:1038.
97. Sandes AF, Kerbauy DMB, Matarraz S, Chauffaille M de LLF, López A, Orfao A, et al. Combined flow cytometric assessment of CD45, HLA-DR, CD34, and CD117 expression is a useful approach for reliable quantification of blast cells in myelodysplastic syndromes. Cytometry Part B: Clin Cytometry. (2013) 84:157–66. doi: 10.1002/cyto.b.21087
98. Alhan C, Westers TM, Cremers EMP, Cali C, Witte BI, Ossenkoppele GJ, et al. The myelodysplastic syndromes flow cytometric score: a three-parameter prognostic flow cytometric scoring system. Leukemia. (2016) 30:658–65. doi: 10.1038/leu.2015.295
99. Dhingra G, Dass J, Arya V, Gupta N, Saraf A, Langer S, et al. Evaluation of multiparametric flow cytometry in diagnosis & prognosis of myelodysplastic syndrome in India. Indian J Med Res. (2020) 152:254. doi: 10.4103/ijmr.IJMR_924_18
100. Rajab A, Porwit A. Screening bone marrow samples for abnormal lymphoid populations and myelodysplasia-related features with one 10-color 14-antibody screening tube. Cytometry Part B: Clin Cytometry. (2015) 88:253–60. doi: 10.1002/cyto.b.21233
101. Raskovalova T, Berger MG, Jacob M-C, Park S, Campos L, Aanei CM, et al. Flow cytometric analysis of neutrophil myeloperoxidase expression in peripheral blood for ruling out myelodysplastic syndromes: a diagnostic accuracy study. Haematologica. (2019) 104:2382–90. doi: 10.3324/haematol.2018.202275
102. Vikentiou M, Psarra K, Kapsimali V, Liapis K, Michael M, Tsionos K, et al. Distinct neutrophil subpopulations phenotype by flow cytometry in myelodysplastic syndromes. Leukemia Lymphoma. (2009) 50:401–9. doi: 10.1080/10428190902755497
103. Selimoglu-Buet D, Wagner-Ballon O, Saada V, Bardet V, Itzykson R, Bencheikh L, et al. Characteristic repartition of monocyte subsets as a diagnostic signature of chronic myelomonocytic leukemia. Blood. (2015) 125:3618–26. doi: 10.1182/blood-2015-01-620781
104. Barreau S, Green AS, Dussiau C, Alary A, Raimbault A, Mathis S, et al. Phenotypic landscape of granulocytes and monocytes by multiparametric flow cytometry: A prospective study of a 1-tube panel strategy for diagnosis and prognosis of patients with MDS. Cytometry Part B Clin. (2020) 98:226–37. doi: 10.1002/cyto.b.21843
105. Stachurski D, Smith BR, Pozdnyakova O, Andersen M, Xiao Z, Raza A, et al. Flow cytometric analysis of myelomonocytic cells by a pattern recognition approach is sensitive and specific in diagnosing myelodysplastic syndrome and related marrow diseases: emphasis on a global evaluation and recognition of diagnostic pitfalls. Leuk Res. (2008) 32:215–24. doi: 10.1016/j.leukres.2007.06.012
106. Béné MC, Axler O, Violidaki D, Lacombe F, Ehinger M, Porwit A. Definition of erythroid differentiation subsets in normal human bone marrow using flowSOM unsupervised cluster analysis of flow cytometry data. Hemasphere. (2020) 5:e512. doi: 10.1097/HS9.0000000000000512
107. Porwit A, Violidaki D, Axler O, Lacombe F, Ehinger M, Béné MC. Unsupervised cluster analysis and subset characterization of abnormal erythropoiesis using the bioinformatic Flow-Self Organizing Maps algorithm. Cytometry Part B Clin. (2022) 102:134–42. doi: 10.1002/cyto.b.22059
108. Ogata K, Mochimaru Y, Sei K, Kawahara N, Ogata M, Yamamoto Y. Myeloblasts transition to megakaryoblastic immunophenotypes over time in some patients with myelodysplastic syndromes. PLoS One. (2023) 18:e0291662. doi: 10.1371/journal.pone.0291662
109. Ogata K, Sei K, Kawahara N, Yamamoto Y. Clinical significance of CD41-positive blasts in association with a monosomal karyotype in patients with myelodysplastic syndrome treated with azacitidine. Br J Haematology. (2020) 189:e144–7. doi: 10.1111/bjh.16565
110. Sandes AF, Yamamoto M, Matarraz S, Chauffaille M de LLF, Quijano S, López A, et al. Altered immunophenotypic features of peripheral blood platelets in myelodysplastic syndromes. Haematologica. (2012) 97:895–902. doi: 10.3324/haematol.2011.057158
111. Barakos GP, Hatzimichael E. Microenvironmental features driving immune evasion in myelodysplastic syndromes and acute myeloid leukemia. Diseases. (2022) 10:33. doi: 10.3390/diseases10020033
112. Winter S, Shoaie S, Kordasti S, Platzbecker U. Integrating the “Immunome” in the stratification of myelodysplastic syndromes and future clinical trial design. JCO. (2020) 38:1723–35. doi: 10.1200/JCO.19.01823
113. Micheva I, Thanopoulou E, Michalopoulou S, Kakagianni T, Kouraklis-Symeonidis A, Symeonidis A, et al. Impaired generation of bone marrow CD34-derived dendritic cells with low peripheral blood subsets in patients with myelodysplastic syndrome. Br J Haematol. (2004) 126:806–14. doi: 10.1111/j.1365-2141.2004.05132.x
114. Tsoplou P, Kouraklis-Symeonidis A, Thanopoulou E, Zikos P, Orphanos V, Zoumbos N. Apoptosis in patients with myelodysplastic syndromes: differential involvement of marrow cells in ‘good’ versus ‘poor’ prognosis patients and correlation with apoptosis-related genes. Leukemia. (1999) 13:1554–63. doi: 10.1038/sj.leu.2401538
115. Symeonidis A, Kourakli A, Katevas P, Perraki M, Tiniakou M, Matsouka P, et al. Immune function parameters at diagnosis in patients with myelodysplastic syndromes: Correlation with the FAB classification and prognosis. Eur J Haematology. (1991) 47:277–81. doi: 10.1111/j.1600-0609.1991.tb01571.x
116. Shao L, Zhang L, Hou Y, Yu S, Liu X, Huang X, et al. Th22 cells as well as th17 cells expand differentially in patients with early-stage and late-stage myelodysplastic syndrome. PLoS One. (2012) 7:e51339. doi: 10.1371/journal.pone.0051339
117. Kotsianidis I, Bouchliou I, Nakou E, Spanoudakis E, Margaritis D, Christophoridou AV, et al. Kinetics, function and bone marrow trafficking of CD4+CD25+FOXP3+ regulatory T cells in myelodysplastic syndromes (MDS). Leukemia. (2009) 23:510–8. doi: 10.1038/leu.2008.333
118. Lyons AB. Divided we stand: Tracking cell proliferation with carboxyfluorescein diacetate succinimidyl ester. Immunol Cell Biol. (1999) 77:509–15. doi: 10.1046/j.1440-1711.1999.00864.x
119. Smith PJ, Wiltshire M, Davies S, Patterson LH, Hoy T. A novel cell permeant and far red-fluorescing DNA probe, DRAQ5, for blood cell discrimination by flow cytometry. J Immunol Methods. (1999) 229:131–9. doi: 10.1016/S0022-1759(99)00116-7
120. Darzynkiewicz Z, Galkowski D, Zhao H. Analysis of apoptosis by cytometry using TUNEL assay. Methods. (2008) 44:250–4. doi: 10.1016/j.ymeth.2007.11.008
121. van Engeland M, Ramaekers FCS, Schutte B, Reutelingsperger CPM. A novel assay to measure loss of plasma membrane asymmetry during apoptosis of adherent cells in culture. Cytometry. (1996) 24:131–9. doi: 10.1002/(SICI)1097-0320(19960601)24:2<131::AID-CYTO5>3.0.CO;2-M
122. Ludwig LM, Maxcy KL, LaBelle JL. Flow cytometry-based detection and analysis of BCL-2 family proteins and mitochondrial outer membrane permeabilization (MOMP). Methods Mol Biol. (2019) 1877:77–91. doi: 10.1007/978-1-4939-8861-7_5
123. Matarraz S, Teodosio C, Fernandez C, Albors M, Jara-Acevedo M, López A, et al. The proliferation index of specific bone marrow cell compartments from myelodysplastic syndromes is associated with the diagnostic and patient outcome. PLoS One. (2012) 7:e44321. doi: 10.1371/journal.pone.0044321
124. Parker JE, Mufti GJ, Rasool F, Mijovic A, Devereux S, Pagliuca A. The role of apoptosis, proliferation, and the Bcl-2–related proteins in the myelodysplastic syndromes and acute myeloid leukemia secondary to MDS. Blood. (2000) 96:3932–8. doi: 10.1182/blood.V96.12.3932
125. Invernizzi R, Travaglino E. Increased apoptosis as a mechanism of ineffective erythropoiesis in myelodysplastic syndromes. Clin Leukemia. (2008) 2:113–20. doi: 10.3816/CLK.2008.n.014
126. Masters SL, Gerlic M, Metcalf D, Preston S, Pellegrini M, O’Donnell JA, et al. NLRP1 inflammasome activation induces pyroptosis of hematopoietic progenitor cells. Immunity. (2012) 37:1009–23. doi: 10.1016/j.immuni.2012.08.027
127. Sallman DA, Cluzeau T, Basiorka AA, List A. Unraveling the pathogenesis of MDS: the NLRP3 inflammasome and pyroptosis drive the MDS phenotype. Front Oncol. (2016) 6:151. doi: 10.3389/fonc.2016.00151
128. Mestrum SGC, Hopman AHN, Ramaekers FCS, Leers MPG. The potential of proliferative and apoptotic parameters in clinical flow cytometry of myeloid Malignancies. Blood Adv. (2021) 5:2040–52. doi: 10.1182/bloodadvances.2020004094
129. Nies KPH, Kraaijvanger R, Lindelauf KHK, Drent RJMR, Rutten RMJ, Ramaekers FCS, et al. Determination of the proliferative fractions in differentiating hematopoietic cell lineages of normal bone marrow. Cytometry Part A. (2018) 93:1097–105. doi: 10.1002/cyto.a.23564
130. Mestrum SGC, de Wit NCJ, Drent RJM, Hopman AHN, Ramaekers FCS, Leers MPG. Proliferative activity is disturbed in myeloproliferative neoplasms (MPN), myelodysplastic syndrome (MDS), and MDS/MPN diseases. Differences between MDS and MDS/MPN. Cytometry Part B: Clin Cytometry. (2021) 100:322–30. doi: 10.1002/cyto.b.21946
131. Borowitz MJ, Craig FE, DiGiuseppe JA, Illingworth AJ, Rosse W, Sutherland DR, et al. Guidelines for the diagnosis and monitoring of paroxysmal nocturnal hemoglobinuria and related disorders by flow cytometry. Cytometry Part B Clin. (2010) 78:211–30. doi: 10.1002/cyto.b.20525
132. Toyama K, Ohyashiki K, Yoshida Y, Abe T, Asano S, Hirai H, et al. Clinical and cytogenetic findings of myelodysplastic syndromes showing hypocellular bone marrow or minimal dysplasia, in comparison with typical myelodysplastic syndromes. Int J Hematol. (1993) 58:53–61.
133. Valent P, Orazi A, Savona MR, Patnaik MM, Onida F, van de Loosdrecht AA, et al. Proposed diagnostic criteria for classical chronic myelomonocytic leukemia (CMML), CMML variants and pre-CMML conditions. Haematologica. (2019) 104:1935–49. doi: 10.3324/haematol.2019.222059
134. Escribano L, Garcia Montero AC, Núñez R, Orfao A. Flow cytometric analysis of normal and neoplastic mast cells: role in diagnosis and follow-up of mast cell disease. Immunol Allergy Clinics North America. (2006) 26:535–47. doi: 10.1016/j.iac.2006.05.008
135. Steensma DP. Dysplasia has A differential diagnosis: distinguishing genuine myelodysplastic syndromes (MDS) from mimics, imitators, copycats and impostors. Curr Hematol Malig Rep. (2012) 7:310–20. doi: 10.1007/s11899-012-0140-3
136. Dimopoulos K, Hansen OK, Sjö LD, Saft L, Schjødt IM, Werner Hansen J, et al. The diagnostic and prognostic role of flow cytometry in idiopathic and clonal cytopenia of undetermined significance (ICUS/CCUS): A single-center analysis of 79 patients. Cytometry Part B Clin. (2020) 98:250–8. doi: 10.1002/cyto.b.21842
137. Cremers EMP, Westers TM, Alhan C, Cali C, Wondergem MJ, Poddighe PJ, et al. Multiparameter flow cytometry is instrumental to distinguish myelodysplastic syndromes from non-neoplastic cytopenias. Eur J Cancer. (2016) 54:49–56. doi: 10.1016/j.ejca.2015.11.013
138. Kern W, Bacher U, Haferlach C, Alpermann T, Schnittger S, Haferlach T. Multiparameter flow cytometry provides independent prognostic information in patients with suspected myelodysplastic syndromes: A study on 804 patients. Cytometry Part B: Clin Cytometry. (2015) 88:154–64. doi: 10.1002/cyto.b.21224
139. Van De Loosdrecht AA, Westers TM, Westra AH, Dräger AM, van der Velden VHJ, Ossenkoppele GJ. Identification of distinct prognostic subgroups in low- and intermediate-1–risk myelodysplastic syndromes by flow cytometry. Blood. (2008) 111:1067–77. doi: 10.1182/blood-2007-07-098764
140. Scott BL, Wells DA, Loken MR, Myerson D, Leisenring WM, Deeg HJ. Validation of a flow cytometric scoring system as a prognostic indicator for posttransplantation outcome in patients with myelodysplastic syndrome. Blood. (2008) 112:2681–6. doi: 10.1182/blood-2008-05-153700
141. Matarraz S, López A, Barrena S, Fernandez C, Jensen E, Flores-Montero J, et al. Bone marrow cells from myelodysplastic syndromes show altered immunophenotypic profiles that may contribute to the diagnosis and prognostic stratification of the disease: A pilot study on a series of 56 patients. Cytometry Part B Clin. (2010) 78:154–68. doi: 10.1002/cyto.b.20513
142. Guo J, Wang H, Xiong S, Zhang X, Zhang C, Yang D, et al. Simplified flow cytometry scoring for diagnosis and prognosis of myelodysplastic symptom. Am J Transl Res. (2020) 12:7449–58.
143. Falco P, Levis A, Stacchini A, Ciriello MM, Geuna M, Notari P, et al. Prognostic relevance of cytometric quantitative assessment in patients with myelodysplastic syndromes: Prognostic role of flow cytometry in MDS. Eur J Haematology. (2011) 87:409–18. doi: 10.1111/j.1600-0609.2011.01676.x
144. Majcherek M, Kiernicka-Parulska J, Mierzwa A, Barańska M, Matuszak M, Lewandowski K, et al. The diagnostic and prognostic significance of flow cytometric bone marrow assessment in myelodysplastic syndromes according to the European LeukemiaNet recommendations in single-centre real-life experience. Scand J Immunol. (2021) 94:e13028. doi: 10.1111/sji.13028
145. Chu S-C, Wang T-F, Li C-C, Kao R-H, Li D-K, Su Y-C, et al. Flow cytometric scoring system as a diagnostic and prognostic tool in myelodysplastic syndromes. Leukemia Res. (2011) 35:868–73. doi: 10.1016/j.leukres.2011.02.016
146. Van De Loosdrecht AA, Kern W, Porwit A, Valent P, Kordasti S, Cremers E, et al. Clinical application of flow cytometry in patients with unexplained cytopenia and suspected myelodysplastic syndrome: A report of the European LeukemiaNet International MDS-Flow Cytometry Working Group. Cytometry Part B Clin. (2023) 104:77–86. doi: 10.1002/cyto.b.22044
147. Mittelman M, Karcher DS, Kammerman LA, Lessin LS. High la (HLA-DR) and low CD11b (Mo1) expression may predict early conversion to leukemia in myelodysplastic syndromes. Am J Hematol. (1993) 43:165–71. doi: 10.1002/ajh.2830430302
148. Gardikas N, Vikentiou M, Konsta E, Kontos CK, Papageorgiou SG, Spathis A, et al. Immunophenotypic profile of CD34+ Subpopulations and their role in the diagnosis and prognosis of patients with de-novo, particularly low-grade myelodysplastic syndromes. Cytometry Part B Clin. (2019) 96:73–82. doi: 10.1002/cyto.b.21725
149. Guarnera L, Fabiani E, Attrotto C, Hajrullaj H, Cristiano A, Latagliata R, et al. Reliability of flow-cytometry in diagnosis and prognostic stratification of myelodysplastic syndromes: correlations with morphology and mutational profile. Ann Hematol. (2023) 102:3015–23. doi: 10.1007/s00277-023-05384-2
150. Oelschlaegel U, Winter S, Sockel K, Epp K, SChadt J, Röhnert MA, et al. MDS-PB13 Score - Blood based detection of aberrancies by flow cytometry in patients with suspected and confirmed Myelodysplastic Neoplasms. Leukemia. (2024) 38:446–50. doi: 10.1038/s41375-024-02141-w
151. Papaemmanuil E, Gerstung M, Malcovati L, Tauro S, Gundem G, Van Loo P, et al. Clinical and biological implications of driver mutations in myelodysplastic syndromes. Blood. (2013) 122:3616–27. doi: 10.1182/blood-2013-08-518886
152. Weiß E, Walter W, Meggendorfer M, Baer C, Haferlach C, Haferlach T, et al. Identification of a specific immunophenotype associated with a consistent pattern of genetic mutations including SRFS2and gene expression profile in MDS. Cytometry Part B Clin. (2023) 104:173–82. doi: 10.1002/cyto.b.22057
153. Oelschlaegel U, Westers TM, Mohr B, Kramer M, Parmentier S, Sockel K, et al. Myelodysplastic syndromes with a deletion 5q display a characteristic immunophenotypic profile suitable for diagnostics and response monitoring. Haematologica. (2015) 100:e93–6. doi: 10.3324/haematol.2014.115725
154. Maurya N, Mohanty P, Dhangar S, Panchal P, Jijina F, Mathan SLP, et al. Comprehensive analysis of genetic factors predicting overall survival in Myelodysplastic syndromes. Sci Rep. (2022) 12:5925. doi: 10.1038/s41598-022-09864-9
155. Bersanelli M, Travaglino E, Meggendorfer M, Matteuzzi T, Sala C, Mosca E, et al. Classification and personalized prognostic assessment on the basis of clinical and genomic features in myelodysplastic syndromes. JCO. (2021) 39:1223–33. doi: 10.1200/JCO.20.01659
156. Malcovati L, Stevenson K, Papaemmanuil E, Neuberg D, Bejar R, Boultwood J, et al. SF3B1-mutant MDS as a distinct disease subtype: a proposal from the International Working Group for the Prognosis of MDS. Blood. (2020) 136:157–70. doi: 10.1182/blood.2020004850
157. Adema V, Ma F, Kanagal-Shamanna R, Thongon N, Montalban-Bravo G, Yang H, et al. Targeting the EIF2AK1 signaling pathway rescues red blood cell production in SF3B1-mutant myelodysplastic syndromes with ringed sideroblasts. Blood Cancer Discovery. (2022) 3:554–67. doi: 10.1158/2643-3230.BCD-21-0220
158. Jiang M, Chen M, Liu Q, Jin Z, Yang X, Zhang W. SF3B1 mutations in myelodysplastic syndromes: A potential therapeutic target for modulating the entire disease process. Front Oncol. (2023) 13:1116438. doi: 10.3389/fonc.2023.1116438
159. Sallman DA, McLemore AF, Aldrich AL, Komrokji RS, McGraw KL, Dhawan A, et al. TP53 mutations in myelodysplastic syndromes and secondary AML confer an immunosuppressive phenotype. Blood. (2020) 136:2812–23. doi: 10.1182/blood.2020006158
160. List A, Ebert BL, Fenaux P. A decade of progress in myelodysplastic syndrome with chromosome 5q deletion. Leukemia. (2018) 32:1493–9. doi: 10.1038/s41375-018-0029-9
161. Fink EC, Ebert BL. The novel mechanism of lenalidomide activity. Blood. (2015) 126:2366–9. doi: 10.1182/blood-2015-07-567958
162. Fuchs O. Important genes in the pathogenesis of 5q- syndrome and their connection with ribosomal stress and the innate immune system pathway. Leuk Res Treat. (2012) 2012:179402. doi: 10.1155/2012/179402
163. Cutler JA, Wells DA, van de Loosdrecht AA, de Baca ME, Kalnoski MH, Zehentner BK, et al. Phenotypic abnormalities strongly reflect genotype in patients with unexplained cytopenias. Cytometry Part B: Clin Cytometry. (2011) 80:150–7. doi: 10.1002/cyto.b.20582
164. Alayed K, Meyerson HJ. Decreased CD177pos neutrophils in myeloid neoplasms is associated with NPM1, RUNX1, TET2, and U2AF1 S34F mutations. Leukemia Res. (2022) 112:106752. doi: 10.1016/j.leukres.2021.106752
165. Cutler JA, Pugsley HR, Bennington R, Fritschle W, Hartmann L, Zaidi N, et al. Integrated analysis of genotype and phenotype reveals clonal evolution and cytogenetically driven disruption of myeloid cell maturation in myelodysplastic syndromes. Cytometry Part B Clin. (2023) 104:183–94. doi: 10.1002/cyto.b.22036
166. Keerthivasan G, Mei Y, Zhao B, Zhang L, Harris CE, Gao J, et al. Aberrant overexpression of CD14 on granulocytes sensitizes the innate immune response in mDia1 heterozygous del(5q) MDS. Blood. (2014) 124:780. doi: 10.1182/blood-2014-01-552463
167. Chen X, Wood BL, Cherian S. Immunophenotypic features of myeloid neoplasms associated with chromosome 7 abnormalities. Cytometry B Clin Cytom. (2019) 96:300–9. doi: 10.1002/cyto.b.21775
168. Duetz C, Westers TM, In ’T Hout FEM, Cremers EMP, Alhan C, Venniker-Punt B, et al. Distinct bone marrow immunophenotypic features define the splicing factor 3B subunit 1 ( SF3B1 )-mutant myelodysplastic syndromes subtype. Br J Haematol. (2021) 193:798–803. doi: 10.1111/bjh.17414
169. Cabezón M, Bargay J, Xicoy B, García O, Borrás J, Tormo M, et al. Impact of mutational studies on the diagnosis and the outcome of high-risk myelodysplastic syndromes and secondary acute myeloid leukemia patients treated with 5-azacytidine. Oncotarget. (2018) 9:19342–55. doi: 10.18632/oncotarget.25046
170. Haferlach T, Nagata Y, Grossmann V, Okuno Y, Bacher U, Nagae G, et al. Landscape of genetic lesions in 944 patients with myelodysplastic syndromes. Leukemia. (2014) 28:241–7. doi: 10.1038/leu.2013.336
171. Dutta S, Moritz J, Pregartner G, Thallinger GG, Brandstätter I, Lind K, et al. Comparison of acute myeloid leukemia and myelodysplastic syndromes with TP53 aberrations. Ann Hematol. (2022) 101:837–46. doi: 10.1007/s00277-022-04766-2
172. Dannheim KC, Pozdnyakova O, Dal Cin P, Weinberg OK. Immunophenotypic dysplasia and aberrant T-cell antigen expression in acute myeloid leukaemia with complex karyotype and TP53 mutations. J Clin Pathol. (2018) 71:1051–9. doi: 10.1136/jclinpath-2018-205348
173. Falini B, Brunetti L, Sportoletti P, Martelli MP. NPM1-mutated acute myeloid leukemia: from bench to bedside. Blood. (2020) 136:1707–21. doi: 10.1182/blood.2019004226
174. Bains A, Luthra R, Medeiros LJ, Zuo Z. FLT3 and NPM1 mutations in myelodysplastic syndromes: Frequency and potential value for predicting progression to acute myeloid leukemia. Am J Clin Pathol. (2011) 135:62–9. doi: 10.1309/AJCPEI9XU8PYBCIO
175. Papadopoulou V, Schoumans J, Basset V, Solly F, Pasquier J, Blum S, et al. Single-center, observational study of AML/MDS-EB with IDH1/2 mutations: genetic profile, immunophenotypes, mutational kinetics and outcomes. Hematology. (2023) 28:2180704. doi: 10.1080/16078454.2023.2180704
176. Subirá D, Alhan C, Oelschlaegel U, Porwit A, Psarra K, Westers TM, et al. Monitoring treatment with 5-Azacitidine by flow cytometry predicts duration of hematological response in patients with myelodysplastic syndrome. Ann Hematol. (2021) 100:1711–22. doi: 10.1007/s00277-021-04411-4
177. Fenaux P, Haase D, Santini V, Sanz GF, Platzbecker U, Mey U. Myelodysplastic syndromes: ESMO Clinical Practice Guidelines for diagnosis, treatment and follow-up†☆. Ann Oncol. (2021) 32:142–56. doi: 10.1016/j.annonc.2020.11.002
178. Raimbault A, Itzykson R, Willems L, Rousseau A, Chapuis N, Mathis S, et al. The fraction of CD117/c-KIT-expressing erythroid precursors predicts ESA response in low-risk myelodysplastic syndromes. Cytometry Part B Clin. (2019) 96:215–22. doi: 10.1002/cyto.b.21781
179. Matsuoka A, Tanibuchi M, Yamazaki I, Taoka T. Erythroblast predominance without CD41/cyCD41-positive blasts predicts favorable prognosis in patients with myelodysplastic syndromes and acute myeloid leukemias treated with azacitidine. Int J Hematol. (2022) 115:852–9. doi: 10.1007/s12185-022-03317-9
180. Caprioli C, Nazari I, Milovanovic S, Pelicci PG. Single-cell technologies to decipher the immune microenvironment in myeloid neoplasms: perspectives and opportunities. Front Oncol. (2021) 11:796477. doi: 10.3389/fonc.2021.796477
181. Navin NE. Cancer genomics: one cell at a time. Genome Biol. (2014) 15:452. doi: 10.1186/s13059-014-0452-9
182. Giladi A, Amit I. Single-cell genomics: A stepping stone for future immunology discoveries. Cell. (2018) 172:14–21. doi: 10.1016/j.cell.2017.11.011
183. Stoeckius M, Hafemeister C, Stephenson W, Houck-Loomis B, Chattopadhyay PK, Swerdlow H, et al. Simultaneous epitope and transcriptome measurement in single cells. Nat Methods. (2017) 14:865–8. doi: 10.1038/nmeth.4380
184. Peterson VM, Zhang KX, Kumar N, Wong J, Li L, Wilson DC, et al. Multiplexed quantification of proteins and transcripts in single cells. Nat Biotechnol. (2017) 35:936–9. doi: 10.1038/nbt.3973
185. Berlin C, Kowalewski DJ, Schuster H, Mirza N, Walz S, Handel M, et al. Mapping the HLA ligandome landscape of acute myeloid leukemia: a targeted approach toward peptide-based immunotherapy. Leukemia. (2015) 29:647–59. doi: 10.1038/leu.2014.233
186. Behbehani GK, Finck R, Samusik N, Sridhar K, Fantl WJ, Greenberg PL, et al. Profiling myelodysplastic syndromes by mass cytometry demonstrates abnormal progenitor cell phenotype and differentiation. Cytometry Part B Clin. (2020) 98:131–45. doi: 10.1002/cyto.b.21860
187. Duetz C, Van Gassen S, Westers TM, Van Spronsen MF, Bachas C, Saeys Y, et al. Computational flow cytometry as a diagnostic tool in suspected-myelodysplastic syndromes. Cytometry Pt A. (2021) 99:814–24. doi: 10.1002/cyto.a.24360
188. Duchmann M, Wagner-Ballon O, Boyer T, Cheok M, Fournier E, Guerin E, et al. Machine learning identifies the independent role of dysplasia in the prediction of response to chemotherapy in AML. Leukemia. (2022) 36:656–63. doi: 10.1038/s41375-021-01435-7
189. Davydova YO, Parovichnikova EN, Galtseva IV, Kokhno AV, Dvirnyk VN, Kovrigina AM, et al. Diagnostic significance of flow cytometry scales in diagnostics of myelodysplastic syndromes. Cytometry Part B Clin. (2021) 100:312–21. doi: 10.1002/cyto.b.21965
190. Anwar N, Memon FA, Shahid S, Shakeel M, Irfan M, Arshad A, et al. The Dawn of next generation DNA sequencing in myelodysplastic syndromes- experience from Pakistan. BMC Genomics. (2021) 22:903. doi: 10.1186/s12864-021-08221-w
191. Malcovati L, Hellström-Lindberg E, Bowen D, Adès L, Cermak J, Del Cañizo C, et al. Diagnosis and treatment of primary myelodysplastic syndromes in adults: recommendations from the European LeukemiaNet. Blood. (2013) 122:2943–64. doi: 10.1182/blood-2013-03-492884
192. Bacher U, Haferlach T, Kern W, Weiss T, Schnittger S, Haferlach C. The impact of cytomorphology, cytogenetics, molecular genetics, and immunophenotyping in a comprehensive diagnostic workup of myelodysplastic syndromes. Cancer. (2009) 115:4524–32. doi: 10.1002/cncr.24501
193. Mannelli F, Gesullo F, Rotunno G, Pacilli A, Bencini S, Annunziato F, et al. Myelodysplasia as assessed by multiparameter flow cytometry refines prognostic stratification provided by genotypic risk in systemic mastocytosis. Am J Hematol. (2019) 94:845–52. doi: 10.1002/ajh.25506
194. Chen X, Othus M, Wood BL, Walter RB, Becker PS, Percival M-E, et al. Comparison of myeloid blast counts and variant allele frequencies of gene mutations in myelodysplastic syndrome with excess blasts and secondary acute myeloid leukemia. Leuk Lymphoma. (2021) 62:1226–33. doi: 10.1080/10428194.2020.1861267
195. Toth LN, Green D, Peterson J, Deharvengt SJ, de Abreu FB, Loo EY. Variant allele frequencies do not correlate well with myeloblast counts in a clinically validated gene sequencing panel for routine acute myeloid leukemia workup. Leuk Lymphoma. (2019) 60:2415–22. doi: 10.1080/10428194.2019.1587757
Keywords: myelodysplastic syndromes, myelodysplastic neoplasms, flow cytometry, immunophenotype, molecular genetics, classification, diagnosis, prognosis
Citation: Verigou E, Chatzilygeroudi T, Lazaris V, de Lastic A-L and Symeonidis A (2024) Immunophenotyping myelodysplastic neoplasms: the role of flow cytometry in the molecular classification era. Front. Oncol. 14:1447001. doi: 10.3389/fonc.2024.1447001
Received: 10 June 2024; Accepted: 09 October 2024;
Published: 31 October 2024.
Edited by:
Chung Hoow Kok, University of Adelaide, AustraliaReviewed by:
Teresa de Souza Fernandez, National Cancer Institute (INCA), BrazilCopyright © 2024 Verigou, Chatzilygeroudi, Lazaris, de Lastic and Symeonidis. This is an open-access article distributed under the terms of the Creative Commons Attribution License (CC BY). The use, distribution or reproduction in other forums is permitted, provided the original author(s) and the copyright owner(s) are credited and that the original publication in this journal is cited, in accordance with accepted academic practice. No use, distribution or reproduction is permitted which does not comply with these terms.
*Correspondence: Argiris Symeonidis, YXJnaXJpcy5zeW1lb25pZGlzQHlhaG9vLmdy; Evgenia Verigou, ai52ZXJpZ291QGdtYWlsLmNvbQ==
†These authors have contributed equally to this work
Disclaimer: All claims expressed in this article are solely those of the authors and do not necessarily represent those of their affiliated organizations, or those of the publisher, the editors and the reviewers. Any product that may be evaluated in this article or claim that may be made by its manufacturer is not guaranteed or endorsed by the publisher.
Research integrity at Frontiers
Learn more about the work of our research integrity team to safeguard the quality of each article we publish.