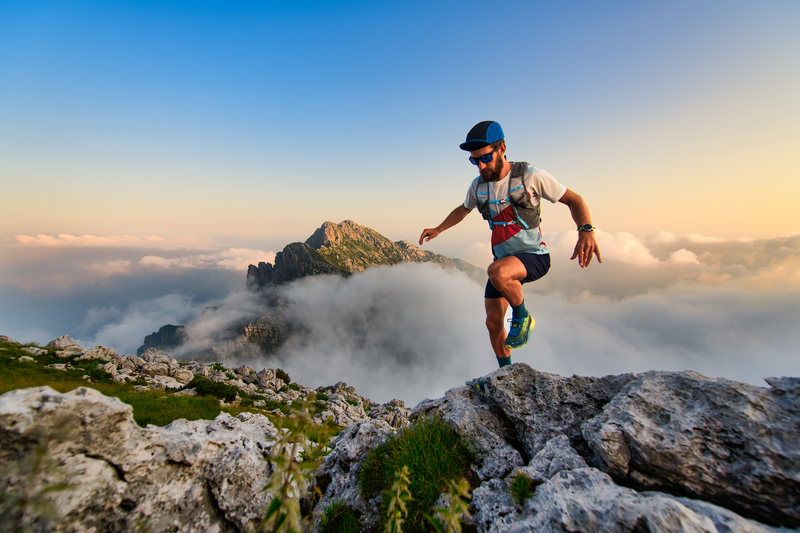
95% of researchers rate our articles as excellent or good
Learn more about the work of our research integrity team to safeguard the quality of each article we publish.
Find out more
ORIGINAL RESEARCH article
Front. Oncol. , 16 August 2024
Sec. Breast Cancer
Volume 14 - 2024 | https://doi.org/10.3389/fonc.2024.1443399
This article is part of the Research Topic Successful Breast Cancer Survivorship Among Racial/Ethnic Minorities View all 9 articles
Background: Breast cancer (BC) affects racial and ethnic groups differently, leading to disparities in clinical presentation and outcomes. It is unclear how Hispanic ethnicity affects BC outcomes based on geographic location and proximity to the United States (U.S.)/Mexico border. We hypothesized that the impact of race/ethnicity on BC outcomes depends on geographic location and country of origin within each BC subtype.
Methods: We analyzed BC data from the Texas Cancer Registry by race/ethnicity/birthplace according to BC subtype (luminal A/luminal B/human epidermal growth factor receptor 2 [HER2]/triple-negative breast cancer[TNBC]). Other covariates included age, geographic location (U.S., Mexico), residency (border, non-border), treatments, and comorbidities. Crude and adjusted effects of race/ethnicity and birthplace on overall survival (OS) were analyzed using Cox regression methods.
Results: Our analysis of 76,310 patient records with specific BC subtypes revealed that Hispanic and non-Hispanic Black (NHB) patients were diagnosed at a younger age compared with non-Hispanic White (NHW) patients for all BC subtypes. For the 19,748 BC patients with complete data on race/ethnicity/birthplace/residency, Hispanic patients had a higher mortality risk in the Luminal A subtype, regardless of birthplace, whereas U.S.-born Hispanics had a higher risk of death in the TNBC subtype. In contrast, NHB patients had a higher mortality risk in the Luminal A and HER2 subtypes. Residence along the U.S./Mexico border had little impact on OS, with better outcomes in Luminal A patients and worse outcomes in Luminal B patients aged 60–74 years.
Conclusion: Race/ethnicity, geographic birth location, and residency were significant predictors of survival in BC. Migration, acculturation, and reduced healthcare access may contribute to outcome differences.
In 2020, breast cancer (BC) became the leading cause of global cancer incidence, overtaking lung cancer with 2.3 million new cases and ranking as the fifth cause of death worldwide (1). Among women in the United States (U.S.), BC is the most frequently diagnosed cancer and remains the second leading cause of cancer-related deaths, with 297,790 new cases and 43,700 deaths in 2023 alone (2). Developing countries exhibit higher BC mortality rates relative to their developed counterparts despite having lower incidence rates, which is likely due to reduced screening measures (3, 4). Despite a decade of declining BC mortality rates, disparities persist among racial and ethnic minorities in the U.S. The BC mortality rate has dropped more slowly for Hispanics than non-Hispanic White (NHW) women (5), and racial/ethnic groups are more frequently diagnosed with advanced-stage tumors due to lower utilization of mammography services and delays in follow-up care (6, 7). Moreover, foreign-born Hispanic patients had lower incidence and mortality rates compared with U.S.-born Hispanics (5). Whereas Hispanic women in the U.S. have demonstrated lower BC incidence and mortality rates compared with NHW and non-Hispanic Black (NHB) women (8), BC remains the primary cause of cancer-related deaths for female Hispanics. Similarly, NHB women have a 4% lower BC incidence rate compared with NHW women, but face a 40% higher chance of death (9). In fact, aggressive BC subtypes like triple-negative breast cancer (TNBC) are more prevalent in non-White populations (10, 11). New strategies and treatments will be required to improve outcomes for minority populations with BC.
BC is a clinically and genetically heterogeneous disease characterized by multiple different subtypes. Molecular subtyping has provided a valuable tool for predicting clinical outcomes in BC (12). Thus, the BC subtype is an important factor that needs to be accounted for in the analysis of overall survival (OS). Breast cancer (BC) can be classified into four major subtypes based on hormone receptor (HR) status: estrogen receptor (ER), progesterone receptor (PR), and HER2. The subtypes are Luminal A (HR+/HER2-), Luminal B (HR+/HER2+), HER2+/ER-/PR-, and triple-negative (HR-/HER2-) (13–16). Among these subtypes, Luminal A has the best prognosis, followed by Luminal B (15, 16). On the other hand, TNBCs are associated with the worst prognosis (16, 17). HER2-positive can be diagnosed at a younger age compared with Luminal A (15, 18). TNBCs are more prevalent among Hispanic women compared with NHW women, and they are also common among NHB women. Luminal A is more common among white women (19–21). The high prevalence of TNBC among NHB and Hispanic women could be the reason for the poor prognosis in these populations (2, 22–24).
There is a dearth of information available about cancer rates along the U.S./Mexico border region. One study reported increased cancer mortality among younger Hispanic Americans in the U.S./Mexico border region spanning California, Arizona, New Mexico, and Texas (25). Similarly, using data from the Texas Cancer Registry (TCR), we demonstrated that Hispanic patients with certain hematologic malignancies located along the Texas/Chihuahua border (Health Service Region 10 [HSR10]) were diagnosed at younger ages, presented with increased numbers of comorbidities, and had worse OS compared with the rest of Texas (26). Although racial and ethnic disparities persist in the presentation and outcomes of BC, the influence of race and ethnicity on survival status in the different BC subtypes remains unclear. Moreover, the impact of Hispanic ethnicity on BC outcomes concerning birth country and proximity to the U.S./Mexico border requires further examination, especially within each BC subtype. Previous studies of the border region have reported higher BC presentation and mortality rates for women who grew up in the U.S. or immigrated at a young age compared with Mexican Hispanics or those who immigrated at older ages (27). In addition, a link between diabetes, obesity, and BC health disparities among Hispanic women has been established (28). Hispanic and Black women have a higher prevalence of obesity (29, 30), but their comorbidities and biomarkers can be completely different (30–33). Weight gain could be associated with Hispanics compared to other ethnicities (28, 30), negatively affecting metabolic-related disorder outcomes (30, 34); due to this, insulin resistance and metabolic syndrome are common among Hispanics (30, 35, 36), which is linked to increased aggressive BC subtypes (30, 37, 38). Obesity and other comorbidities can affect treatment (30, 39–41), but not much is known about their contribution among Hispanics. Quality of life substantially reduces post-diagnosis among Hispanics (30, 42, 43). Moreover, obesity can impact the dosages required for therapeutic intervention (30, 44, 45), and can also affect surgery, radiotherapy, and adjuvant chemotherapy (30, 46–49).
In the present study, we aimed to evaluate differences in BC presentation and survival between NHW, NHB, and Hispanic patients (born in Mexico versus the U.S.), and comparing the border region with the rest of Texas. We also assessed covariates associated with mortality in BC subtypes throughout Texas. We hypothesized that the impact of race/ethnicity on BC outcomes depends on a combination of BC subtype, country of origin (U.S. vs. Mexico), and residency (HSR10 vs. rest of Texas). Specifically, we sought to understand which BC subtypes have poor presentation and worse OS among Mexican Hispanics compared with non-Hispanics. Additionally, we wanted to pinpoint the BC subtypes demonstrating worse outcomes in the border region to prioritize appropriate screening measures and treatment for these patients.
BC data (International Classification of Disease: ICD-O-3 histology codes 8500–8509) were obtained from the Texas Cancer Registry (TCR), which serves as a statewide population-based registry of cancer prevalence and burden in Texas (https://www.dshs.texas.gov/tcr/). All breast cancer data available (i.e., 1995 to 2016) from the TCR were reviewed to understand the clinical presentation and survival of BC for NHW, NHB, and Hispanic patients, as well as the population at the Texas/Chihuahua border in HSR10. HSR10 refers to communities at the U.S./Mexico border near Chihuahua, Mexico. The region is medically underserved, with significant barriers to healthcare access (50). Hispanics in our dataset were divided by birth country (Mexico or U.S.). The following BC subtypes were analyzed: Luminal A (estrogen and/or progesterone receptor-positive (ER+ or PR+) and human epidermal growth factor receptor 2-negative (HER2-)); Luminal B (ER+ and/or PR+ and HER2+), HER2 positive (ER-, PR-, and HER2+), and TNBC (ER-, PR- and HER2-). We included only female BC patients with sufficient data to classify their BC subtypes, called sub-cohort 1. In secondary criteria, we only included BC patients from sub-cohort 1 with appropriate race/ethnicity/birthplace/age classifications, called sub-cohort 2. This study was approved by the Institutional Review Boards at Texas Tech University Health Sciences Center El Paso (E19045) and the TCR (19–006). Figure 1 illustrates the data selection process. The study sample included 289,593 female BC records from the TCR. BC data not having complete hormone receptor information (213,283 records) were excluded from the study. Baseline characteristics for the excluded BC patients are available in Supplementary Table S1. The remaining 76,310 records (sub-cohort 1) had a specific classification by subtype (Table 1 and Supplementary Table S2). In contrast to data from the Surveillance, Epidemiology, and End Results (SEER) Program, entries without a reported ethnicity in the TCR are not assumed to be non-Hispanic but instead coded as unknown, thereby reducing the potential for perceived bias from self-reporting of ethnicity (51). Patients identified as Other non-Hispanic (46,280) or Other Hispanic (10,282) who were not born in the U.S. or Mexico were excluded from survival calculations due to a lack of confidence surrounding ethnicity. In total, 19,748 records (sub-cohort 2) had complete information regarding classification by race, ethnicity, BC subtype, and birthplace (Table 2 and Supplementary Table S3).
Figure 1. Sample selection diagram and patient characteristics for the current study. The selection of breast cancers from the Texas Cancer Registry was performed using the International Classification of Diseases for Oncology third edition (ICD-O-3) histology codes for the following diseases: ductal and lobular carcinomas, followed by stratification into ER+, PR+, HER2+, and TNBC subtypes.
Table 1. Breast cancer distribution of sub-cohort 1 (76,310 breast cancer patients from the Texas Cancer Registry from 1995–2016) according to subtype, based on age, race, ethnicity, geographic location, and socioeconomic indicators.
Table 2. Sub-cohort 2 consisted of 19,748 patients with complete data on breast cancer subtype, race, ethnicity, birthplace, and age.
OS for each BC subtype was the primary outcome, with race/ethnicity, birthplace (U.S.-born NHW, U.S.-born NHB, U.S.-born Hispanic, Mexico-born Hispanic), geographic location (HSR10, rest of Texas), and residency (HSR10, rest of Texas) as the independent variables. OS was measured from the year of diagnosis to the year of vital status. Patients without a date of death or last contact but were alive after December 2016 were assumed to be alive.
The following covariates were considered as possible confounders of the relationship between race/ethnicity and OS for patients with BC: age (18–39, 40–59, 60–74, 75+ years), grade (I, II, III, IV), SEER stage (localized, regional, distant), treatment (hormone therapy, chemotherapy, radiation therapy, mastectomy, distal lymph or tissue/organ surgery), urbanization (rural, semi-urban, urban), and number of comorbidities (1–2, 3–4, 5–6, 7–8, 9–10). We chose not to control for measures of poverty and stage at diagnosis since these variables are on the causal pathway between our exposure variable (race/ethnicity) and the outcome (time between cancer diagnosis and death) (52). Controlling for causal intermediates using standard regression methods results in biased estimates of the total effect of race/ethnicity (52, 53). Since race and ethnicity are closely linked to socioeconomic status (SES) (52), poverty indicators and primary payer at diagnosis were excluded from the analysis. Based on the literature, Hispanic patients are often diagnosed with BC at a younger age, which likely affects OS. To investigate the potential impact of age on survival, patients were categorized into four age groups (in years) for each BC subtype: 18–39, 40–59, 60–74, and ≥75. Differences in OS among NHW, NHB, and Hispanic patients due to treatment effects were evaluated by stratifying patients based on their intervention(s).
We summarized continuous variables with mean and standard deviation (SD), whereas categorical variables were summarized with frequency and percentage. All covariates were summarized in frequencies with percentages by BC subtypes. The average age of patients was compared between race/ethnicity by one-way analysis of variance within each BC subtype. All the categorical variables were compared by race/ethnicity/country of birth using a chi-square test. The association of each exposure and covariate with OS in each BC diagnosis was determined using a Cox proportional hazards regression analysis. Multivariable Cox proportional hazards regression was used to determine the adjusted association between primary exposures and OS for each BC diagnosis. The multivariable analysis included all the relevant covariates simultaneously in the analysis. A priori, critical covariates were adjusted regardless of their significance level in the multivariable analyses as per the study objectives (54). The results of Cox models were summarized with hazard ratio (HR) and 95% confidence interval (CI). All hazard ratio (HR) calculations in the multivariable analysis were adjusted for the remaining variables listed in the table. The assumption of proportional hazards was tested by inspecting log-log plots for each predictor variable. Severe violations (as indicated by non-parallel lines) were not detected. Kaplan-Meier curves were constructed to describe survival for Hispanic and non-Hispanic patients according to diagnosis or regional location and were compared using log-rank tests. P-values less than or equal to 5% were considered statistically significant results. All the statistical analyses were conducted using SAS version 7 (SAS Institute Inc., Cary, NC, USA) and followed the statistical analysis and reporting checklists (55).
The total number of BC patients in our cohort (sub-cohort 1) included 53,777 Luminal A patients (70.5%), 8,790 Luminal B patients (11.5%), 3,699 HER2 patients (4.8%), and 10,044 TNBC patients (13.2%) (Table 1). For survival calculations, patients who did not identify as NHW, NHB, or Hispanic born in the U.S. or Mexico (Other) were excluded from the analysis (56,562). Of the remaining 19,748 BC patients (sub-cohort 2), 12,650 (64.1%) identified as NHW, 2,958 (15.0%) identified as NHB, 2,484 (12.6%) identified as U.S.-born Hispanic, and 1,656 (8.4%) identified as Mexico-born Hispanic (Table 2 and Figure 1). There were no differences in reporting standards by ethnicity, as 47.5% of non-Hispanics and 47.8% of Hispanics had a specific diagnosis (not shown).
Table 1 shows the distribution of patients with known BC subtypes based on age, race, ethnicity, geographic location, and socioeconomic indicators. The Luminal A subtype of BC was more common in patients older than 60 years, whereas the other BC subtypes were more common in patients 40–59 years of age. NHW patients were more likely to be diagnosed with the Luminal A or Luminal B subtypes of BC. Hispanic patients were more likely to be diagnosed with the HER2 subtype, whereas NHB patients were more likely to be diagnosed with TNBC (Table 1). Proportionally, Hispanics born in the U.S. had higher rates of all BC subtypes than those born in Mexico. Overall, about half of the patients had private insurance; however, many patients were covered by Medicaid/Medicare. Finally, the highest poverty rate was observed in TNBC patients (29.7%) (Table 1).
Supplementary Table S2 illustrates patient distribution between subtypes based on tumor grade, treatment, and comorbidity burden. TNBC patients had the most significant proportion of patients diagnosed at grade III/IV (74.9%), followed by HER2 (69.0%), Luminal B (47.4%) and Luminal A (19.8%). However, HER2 had the most significant proportion of patients with regional and distant metastasis (50.1%). Over half of the patients had no comorbidities across all subtypes. However, the comorbidities most highly associated with all BC subtypes were cardiovascular diseases, endocrine/nutritional/metabolic/immune disorders, and genitourinary diseases.
Most Hispanic patients developed BC between 40 and 59 years of age, regardless of birthplace (Supplementary Table S3). However, in that age group, Hispanic patients born in Mexico developed proportionally more BC than those born in the U.S. (55.8% versus 41.0%). In contrast, NHW patients developed BC older than 60 years (63.9%). Many Hispanic patients resided in HSR10 compared with other racial/ethnic groups (9.3% and 24.3% for Hispanics born in the U.S. and Mexico, respectively). The bottom of Table 2 shows the age at diagnosis for each disease, comparing NHW, NHB, and Hispanic BC patients throughout Texas. NHB and Hispanic patients in Texas were diagnosed at younger ages than NHW patients for all diseases analyzed (P<0.0001). When we compared the distribution of BC among Hispanics born in the U.S. versus Mexico, Hispanics born in Mexico developed BC at younger ages (Table 2). As expected, Hispanics born in Mexico had the highest prevalence of uninsured patients (42.7%), while NHW had the highest proportion of private insurance coverage (42.4%, Supplementary Table S3). Most patients born in the U.S. were enrolled in Medicaid/Medicare. Finally, 53.3% of Hispanic patients born in Mexico were classified as impoverished, followed by NHB (42.9%), Hispanics born in the U.S. (42.3%), and NHW (15.3%) (Supplementary Table S3).
Table 3 describes the tumor grade, treatment, and comorbidity distribution for U.S.-born Hispanics, Mexico-born Hispanics, NHW, and NHB patients. NHB patients had the highest percentages of grade III/IV tumors (50.9%) with distant metastasis (17.4%), followed by Hispanics born in Mexico (42.8% and 15.8%), Hispanics born in the U.S. (39.5% and 12.6%), and NHW (32.8% and 10.1%). Similar comorbidities were noted across races and ethnicities, except for Hispanics born in Mexico, who presented with higher rates of 1–2 or 3–4 comorbidities (Table 3). Hispanics born in the U.S. had the highest prevalence of endocrine, nutritional, metabolic, or immune disorders (24.5%), followed by NHB (23.9%), Hispanics born in Mexico (23.6%), and NHW (18.6%). NHBs were most likely to be observed with cardiovascular diseases (30.2%), followed by Hispanic patients born in the U.S. (24.7%), Hispanic patients born in Mexico (22.7%), and NHW (21.2%). Hispanics born in Mexico had a higher proportion of genitourinary comorbidities (17.3%), followed by NHB (14.3%), Hispanics born in the U.S. (11.6%), and NHW (10.6%). Finally, NHB patients had the highest rate of mortalities (48.9%), followed by Hispanics born in the U.S. (40.2%), NHW (39.0%), and Hispanics born in Mexico (35.8%).
Table 3. Demographic and socioeconomic characteristics of sub-cohort 2 (19,748 breast cancer patients with complete data on subtype/race/ethnicity/birthplace/age) based on tumor grade, treatment, and comorbidity burden.
In terms of treatment by subtype, Supplementary Table S2 shows that patients with the Luminal A (27.7%) and Luminal B (22.7%) subtypes of breast cancer received the highest rates of hormone therapy, compared to HER2 (2.7%) and TNBC (1.7%) subtypes, who generally do not qualify for hormone therapy. Over half of patients with HER2 (51.3%), TNBC (49.0%), and Luminal B (45.0%) subtypes received chemotherapy, whereas <20% of patients across all subtypes received radiation therapy. Mastectomies were performed with a high percentage in all patients across all subtypes, with 66.8% for Luminal A, 60.7% for Luminal B, 60.5% for TNBC, and 57.4% for HER2. Distal surgeries were only performed in 2–3% of patients across all BC subtypes.
We next compared BC treatment based on race, ethnicity, and birthplace (Table 3). The use of hormone therapy was higher among NHW and Hispanics born in Mexico (26.1% and 23.4%) compared with Hispanics born in the U.S. and NHB (21.5% and 21.0%). Hispanics born in Mexico had the highest rates of chemotherapy (47.3%), followed by NHB (40.0%), Hispanics born in the U.S. (37.9%), and NHW (30.6%). NHW patients had the highest rates of radiation therapy (21.7%), whereas NHB patients had the lowest rates of radiation therapy (18.1%). Lower rates of mastectomies were observed for NHB patients (50.2%) compared with 55–63% for Hispanic and NHW (Table 3). However, patients who did not have a mastectomy may have received other forms of surgical intervention.
In unadjusted analyses, the outcomes for Hispanic BC patients initially appeared better than those for non-Hispanics diagnosed with the Luminal A and TNBC subtypes (Supplementary Table S4). Black race, on the other hand, was associated with worse outcomes for BC patients diagnosed with the Luminal A, Luminal B, and TNBC subtypes (Supplementary Table S4). Figures 2–4 depict the OS by BC subtype based on race/ethnicity, birthplace, and geographic location, segmented by age. In the Luminal A subtype of BC, worse survival outcomes were observed in every age category associated with race/ethnicity (Figure 2A). In contrast, race had a more significant impact than ethnicity in the Luminal B subtype (Figure 2B). Race/ethnicity did not affect survival in the HER2 subtype of BC (Figure 2C), whereas it negatively affected OS in the younger TNBC patients (Figure 2D). Birthplace had little effect on OS in any BC subtype (Figures 3A–D), except for TNBC patients aged 40–59 years, where Hispanic patients born in Mexico demonstrated better OS than those born in the U.S. (Figure 3D). Lastly, residence in HSR10 near the U.S./Mexico border had little impact on OS (Figures 4A–D), except for Luminal A and Luminal B patients aged 60–74 years. Interestingly, patients in this category residing in HSR10 have better OS than the rest of Texas for Luminal A patients (Figure 4A) but worse for Luminal B patients (Figure 4B). These data underscore the importance of subtype, birthplace, and age in analyzing the effect of race, ethnicity, and geographic location on BC outcomes.
Figure 2. Overall survival (OS) for the different BC subtypes based on race/ethnicity and stratified by age. (A–D) Kaplan Meier curves show the OS for patients diagnosed with the Luminal A (A), Luminal B (B), HER2 (C), and TNBC (D) subtypes of BC based on race and ethnicity. All subtypes were stratified by the following age groups from left to right: 18–39 years, 40–59 years, 60–74 years, and 75+ years.
Figure 3. Overall survival (OS) for Hispanic BC patients based on birthplace and stratified by age. (A–D) Kaplan Meier curves show the OS for Hispanic patients diagnosed with the Luminal A (A), Luminal B (B), HER2 (C), and TNBC (D) subtypes of BC based on birthplace. All subtypes were stratified by the following age groups from left to right: 18–39 years, 40–59 years, 60–74 years, and 75+ years.
Figure 4. Overall survival (OS) for BC patients based on geographic location near the U.S./Mexico border. (A–D) Kaplan Meier curves show the OS for patients diagnosed with the Luminal A (A), Luminal B (B), HER2 (C), and TNBC (D) subtypes of BC based on geographic location near the U.S./Mexico border (HSR10). All subtypes were stratified by the following age groups from left to right: 18–39 years, 40–59 years, 60–74 years, and 75+ years.
Luminal A. For patients with the Luminal A subtype of BC, NHB patients had an 18% increase in mortality (p<.0001). Hispanic ethnicity had a similar effect, regardless of birthplace. Residence near the U.S./Mexico border (HSR10), on the other hand, had no significant impact on mortality compared with residence in other areas of Texas (p=0.066). Comorbidities were associated with worse outcomes for patients with the Luminal A subtype of BC. In contrast, hormone therapy, radiation therapy, mastectomy, and urbanization were associated with better survival outcomes (Table 4).
Table 4. Factors contributing to the overall survival (OS) of sub-cohort 2 in multivariate Cox regression analyses.
Luminal B. For patients with the Luminal B subtype of BC, race, ethnicity, and geographic location did not affect mortality rates. Regional or distant metastases and comorbidities were associated with worse outcomes for patients with the Luminal B subtype of BC, whereas hormone therapy, chemotherapy, and mastectomy were associated with better outcomes (Table 4).
HER2. Mortality risk increased by >2-fold in patients aged 75 years and older (p<0.0001). We observed no differences in mortality comparing patients by ethnicity, birthplace, and residency. However, NHB patients had a 56% increase in mortality risk compared with NHWs (p=0.003). Regional and distant metastasis correlated with worse outcomes, whereas mastectomy significantly reduced mortality risk for patients with HER2+ BC (Table 4).
TNBC. Age did not affect mortality risk in the most aggressive TNBC subtype. While race did not affect mortality risk in TNBC, Hispanic patients from the U.S. had a 25% increase in mortality risk compared with NHW patients (p=0.006). Birthplace in Mexico, on the other hand, had no significant effect. Regional and distant metastasis correlated with worse outcomes for TNBC patients, whereas chemotherapy and mastectomy were associated with better outcomes (Table 4).
Prior work has demonstrated that racial disparities differ by tumor subtype (18, 56, 57). Our data confirmed our hypothesis that the effect of race/ethnicity on breast cancer outcomes depends on the BC subtype and country of origin but not geographic location near the U.S./Mexico border. Ethnic and racial disparities in cancer outcomes, particularly breast cancer, persist despite progress in understanding contributing factors (58,) (59). This is especially true for cancer patients living near the U.S./Mexico border (25, 26, 60). The Hispanic population is the largest minority group in the U.S., representing >18% of the population (61). Hispanic/Latinx refers to individuals with Latin American roots, erroneously generalized to a single homogenous group due to commonalities in language, values, and migration history (61). This study focuses on BC disparities among Hispanic populations, emphasizing the need to analyze subgroups separately due to diverse biological, behavioral, sociocultural, and socioeconomic factors. Our investigation compared BC presentation and outcomes for U.S.-born and Mexico-born Hispanic patients compared with NHW and NHB populations. We also examined the effects of border proximity on BC outcomes and analyzed the impact of various treatment interventions on OS. Our findings revealed worse outcomes for U.S.-born Hispanic patients with TNBC compared with all other racial and ethnic groups. In contrast, U.S.-born Hispanic, Mexico-born Hispanic, and NHB patients with the Luminal A subtype had worse outcomes compared with NHW patients. Surprisingly, proximity to the U.S./Mexico border improved OS for Luminal A patients aged 60–74 years but reduced OS for Luminal B patients aged 60–74 years. Understanding factors that influence the presentation and survival of BC patients is crucial for prioritizing resources, addressing systemic deficiencies, and developing targeted approaches for prevention, screening, treatment, and adherence within these high-risk communities.
Individuals living in the border region face many challenges, such as lower median income, higher poverty rates, and reduced educational attainment leading to lower utilization of screening services (62, 63). Hispanic women with lower educational attainment have difficulty communicating with healthcare providers and may view BC as a terminal disease, unaware of effective treatment options (64). Patients in this region are more likely to be uninsured, and Hispanic patients are more frequently covered by Medicaid than NHWs (62). Previous studies have noted that Hispanics are more likely to be diagnosed with aggressive subtypes of BC, such as TNBC, and present at more advanced stages than NHWs (20). However, differences in survival for Hispanic patients are only partially explained by tumor characteristics. Low SES is linked to increased mortality due to financial concerns, lack of insurance coverage, and limited access to care and screening services (20).
Despite these challenges, some studies have reported lower BC incidence rates and better survival among Hispanics, underscoring the complexity of these disparities (27). The influence of acculturation on higher BC presentation and mortality may reflect the adoption of unhealthy behaviors (such as poor diet and smoking) by those who have been in the U.S. for a more extended period of time. Still, it may also be due to self-selection, where unacculturated immigrants are healthy when they enter the U.S. and may leave the country if diagnosed with cancer and become lost to follow-up (8, 27). This finding may be due to disparities in insurance coverage, as Hispanics are more likely to be underinsured, resulting in problems with healthcare access (65).
Delays in treatment and adherence issues also affect survival rates (58, 66). Hispanics are more likely to experience delayed initiation of chemotherapy and longer wait times (over 30 days) for surgery than NHWs (58, 66). Possible reasons for delays in treatment include SES, insurance status, problems accessing care, and geographic distance from cancer centers (58, 66). Additionally, poor adherence to treatment leads to cancer relapse, higher rates of hospitalization, and shorter OS (67). A survey of Hispanics in the border region found medium-to-low compliance with long-term adjuvant endocrine therapy (67). Frequently cited reasons were forgetfulness, avoidance of medication side effects, and high cost, but educational programs and frequent reassessment were identified as potential strategies for improvement (67). Smaller sample sizes of patients in HSR10 and loss to follow-up, primarily ages 18–39, may affect the interpretation of results. Further investigation is required to identify additional factors affecting OS for aggressive BC subtypes.
In our study, NHB and Hispanic patients in Texas were diagnosed at younger ages than NHW patients for all diseases analyzed (P<0.0001), consistent with previous reports (68, 69). NHB, U.S.-born Hispanic, and Mexico-born Hispanic patients had worse outcomes in the Luminal A subtype, indirectly supporting the potential role of genetic factors associated with Indigenous and African American ancestry in South American populations (70). Notably, BC patients aged 40–59 with the Luminal A subtype showed better OS, possibly due to tissue maturation after breastfeeding (71). Patients 40–59 years old have most likely had one or more children and a longer duration of breastfeeding compared with younger patients and most likely accumulated fewer comorbidities than older patients (71). Foreign-born women also exhibit lower BC mortality rates, often attributed to fewer risk factors like obesity, hormone replacement therapy, alcohol consumption, and a sedentary lifestyle (72). For example, a study analyzing BC mortality rates of women residing in California found that women born in Mexico had a 28% lower mortality rate than those born in the U.S. (12.9 versus 18.0 per 100,000, respectively) (73–75). In our analysis, race remains a significant risk factor, with Black patients experiencing a higher risk of death across all BC subtypes (76).
A major strength of our population-based study is a comprehensive focus on Mexican Hispanic populations by birthplace. Additionally, we included multiple BC subtypes based on the effects of ethnicity, race, border proximity, and age (52). We did not control for the stage at diagnosis, since it is most likely on the causal path between race, ethnicity, and OS (52). Controlling for causal intermediates using standard statistical methods will lead to biased estimates of the total effect (52, 77). Limitations to our study include using a single-state registry without critical behavioral and genomic data and the large number of exclusions based on our stringent eligibility criteria (i.e., lack of BC subtype or ethnicity information). While time to treatment between Hispanic and non-Hispanic patients was another potential limitation, the analysis was only possible with extensive data loss due to irregularities with the reporting of dates within the dataset, preventing us from calculating the time from diagnosis to initiation of treatment.
Greater racial and ethnic diversity in biobanks and genome-wide association studies will allow for improved risk stratification and clinical decision-making (78, 79). Future studies should focus on enhancing diversity, equity, inclusion, and accessibility in healthcare, as well as increased clinical trial participation from diverse backgrounds, to address the needs of at-risk communities (80). On top of that, future studies should examine the effects of various treatment protocols and adherence rates on relapse rate and OS across different racial/ethnic groups. Furthermore, to establish a clear understanding of the socioeconomic factors that impact the pathways leading to breast cancer in minority women, it is essential to conduct genetic, epigenomic, and transcriptomic studies, which may provide novel approaches for diagnosing and treating breast cancer among vulnerable populations. Increasing minority participation in clinical trials will be critical for learning the pathophysiological aspects of treatment. Finally, immediate caregivers, such as nurses and family members, significantly impact treatment outcomes. Therefore, enhancing diversity among healthcare providers would be highly beneficial.
The original contributions presented in the study are included in the article/Supplementary Material. Further inquiries can be directed to the corresponding authors.
The studies involving humans were approved by Institutional Review Boards at Texas Tech University Health Sciences Center El Paso and the Texas Cancer Registry. The studies were conducted in accordance with the local legislation and institutional requirements. Written informed consent for participation was not required from the participants or the participants’ legal guardians/next of kin in accordance with the national legislation and institutional requirements.
VN: Formal analysis, Investigation, Methodology, Software, Visualization, Writing – original draft, Writing – review & editing. AB-A: Conceptualization, Data curation, Investigation, Methodology, Project administration, Supervision, Visualization, Writing – original draft, Writing – review & editing. LA: Formal analysis, Investigation, Methodology, Software, Supervision, Validation, Writing – original draft. MKi: Investigation, Writing – original draft, Writing – review & editing. MG-H: Conceptualization, Data curation, Investigation, Supervision, Validation, Visualization, Writing – original draft, Writing – review & editing. IO: Investigation, Supervision, Visualization, Writing – original draft, Writing – review & editing. MKe: Visualization, Writing – review & editing. SG: Conceptualization, Data curation, Resources, Writing – review & editing. ZM: Conceptualization, Investigation, Methodology, Software, Supervision, Validation, Writing – original draft, Writing – review & editing. AD: Conceptualization, Data curation, Formal analysis, Investigation, Methodology, Project administration, Software, Writing – original draft, Writing – review & editing. SSG: Conceptualization, Data curation, Investigation, Project administration, Supervision, Validation, Visualization, Writing – original draft, Writing – review & editing. AE: Conceptualization, Data curation, Formal analysis, Funding acquisition, Investigation, Methodology, Project administration, Resources, Software, Supervision, Validation, Visualization, Writing – original draft, Writing – review & editing.
The author(s) declare financial support was received for the research, authorship, and/or publication of this article. SG is a CPRIT scholar in cancer research. SG is supported by a first-time faculty recruitment award from the Cancer Prevention and Research Institute of Texas (CPRIT; RR170020). SG is also supported by the NIH 1RO1AI175837–01, Lizanell and Colbert Coldwell Foundation, The Edward N. and Margaret G. Marsh Foundation, the American Cancer Society (RSG-22–170-01-RMC), NIH 1R16GM149497–01, and CPRIT-TREC (RP230420). AE is supported by the American Cancer Society (RSG-23–1025480–01-IBCD), a CPRIT-TREC Pilot Study Award (RP230444), CPRIT-TREC (RP230420), and NIH 1R15HL172157–01.
The authors are grateful to Dr. Rajkumar Lakshmanaswamy for the critical review of the manuscript.
The authors declare that the research was conducted in the absence of any commercial or financial relationships that could be construed as a potential conflict of interest.
The author(s) declared that they were an editorial board member of Frontiers, at the time of submission. This had no impact on the peer review process and the final decision.
All claims expressed in this article are solely those of the authors and do not necessarily represent those of their affiliated organizations, or those of the publisher, the editors and the reviewers. Any product that may be evaluated in this article, or claim that may be made by its manufacturer, is not guaranteed or endorsed by the publisher.
The Supplementary Material for this article can be found online at: https://www.frontiersin.org/articles/10.3389/fonc.2024.1443399/full#supplementary-material
1. Arnold M, Morgan E, Rumgay H, Mafra A, Singh D, Laversanne M, et al. Current and future burden of breast cancer: Global statistics for 2020 and 2040. Breast. (2022) 66:15–23. doi: 10.1016/j.breast.2022.08.010
2. ACS. Breast Cancer Facts & Figures 2022-2024. American Cancer Society. 2022. Atlanta: American Cancer Society, Inc (2022).
3. Ferlay J, Colombet M, Soerjomataram I, Parkin DM, Piñeros M, Znaor A, et al. Cancer statistics for the year 2020: An overview. Int J Cancer. (2021) 149:778–89. doi: 10.1002/ijc.33588
4. Sung H, Ferlay J, Siegel RL, Laversanne M, Soerjomataram I, Jemal A, et al. Global cancer statistics 2020: GLOBOCAN estimates of incidence and mortality worldwide for 36 cancers in 185 countries. CA Cancer J Clin. (2021) 71:209–49. doi: 10.3322/caac.21660
5. Miller KD, Ortiz AP, Pinheiro PS, Bandi P, Minihan A, Fuchs HE, et al. Cancer statistics for the US Hispanic/Latino population, 2021. CA Cancer J Clin. (2021) 71:466–87. doi: 10.3322/caac.21695
6. Press R, Carrasquillo O, Sciacca RR, Giardina EG. Racial/ethnic disparities in time to follow-up after an abnormal mammogram. J Womens Health (Larchmt). (2008) 17:923–30. doi: 10.1089/jwh.2007.0402
7. Stuver SO, Zhu J, Simchowitz B, Hassett MJ, Shulman LN, Weingart SN. Identifying women at risk of delayed breast cancer diagnosis. Jt Comm J Qual Patient Saf. (2011) 37:568–75. doi: 10.1016/S1553-7250(11)37073-0
8. ACS. Cancer Facts & Figures for Hispanics/Latinos 2018-2020 Vol. 2018. Atlanta, GA, USA: American Cancer Society (2018), p. 1–48.
9. ACS. Incidence - NAACCR. Mortality - National Center for Health Statistics (NCHS), Center for Disease Control and Prevention Vol. 2022. American Cancer Society, Inc., Surveillance and Health Equity Science, Atlanta, GA, USA (2022).
10. Prakash O, Hossain F, Danos D, Lassak A, Scribner R, Miele L. Racial disparities in triple negative breast cancer: A review of the role of biologic and non-biologic factors. Front Public Health. (2020) 8:576964. doi: 10.3389/fpubh.2020.576964
11. Davis MB. Genomics and cancer disparities: the justice and power of inclusion. Cancer Discovery. (2021) 11:805–9. doi: 10.1158/2159-8290.CD-21-0225
12. Hung SK, Yang HJ, Lee MS, Liu DW, Chen LC, Chew CH, et al. Molecular subtypes of breast cancer predicting clinical benefits of radiotherapy after breast-conserving surgery: a propensity-score-matched cohort study. Breast Cancer Res. (2023) 25:149. doi: 10.1186/s13058-023-01747-9
13. Carey LA, Cheang MCU, Perou CM. Chapter 29: Genomics, Prognosis, and Therapeutic Interventions. In: Harris JR, Lippman ME, Morrow M, Osborne CK, editors. Diseases of the Breast, 5th Edition. Lippincott Williams & Wilkins, Philadelphia, PA, USA (2014).
14. Foukakis T, Bergh J. Prognostic and predictive factors in early, non-metastatic breast cancer. Burstein HJ, Vora SR, editors. UpToDate, Inc., Waltham, MA (2022).
15. Metzger-Filho O, Sun Z, Viale G, Price KN, Crivellari D, Snyder RD, et al. Patterns of Recurrence and outcome according to breast cancer subtypes in lymph node-negative disease: results from international breast cancer study group trials VIII and IX. J Clin Oncol. (2013) 31:3083–90. doi: 10.1200/JCO.2012.46.1574
16. McGuire A, Lowery AJ, Kell MR, Kerin MJ, Sweeney KJ. Locoregional recurrence following breast cancer surgery in the trastuzumab era: A systematic review by subtype. Ann Surg Oncol. (2017) 24:3124–32. doi: 10.1245/s10434-017-6021-1
17. Anders CK, Carey LA. ER/PR-negative, HER2-negative (triple-negative) breast cancer. Heyes DF, Burstein HJ, Vora SR, editors. UpToDate, Inc., Waltham, MA (2022).
18. Partridge AH, Hughes ME, Warner ET, Ottesen RA, Wong YN, Edge SB, et al. Subtype-dependent relationship between young age at diagnosis and breast cancer survival. J Clin Oncol. (2016) 34:3308–14. doi: 10.1200/JCO.2015.65.8013
19. Scott LC, Mobley LR, Kuo TM, Il’yasova D. Update on triple-negative breast cancer disparities for the United States: A population-based study from the United States Cancer Statistics database, 2010 through 2014. Cancer. (2019) 125:3412–7. doi: 10.1002/cncr.32207
20. Martínez ME, Gomez SL, Tao L, Cress R, Rodriguez D, Unkart J, et al. Contribution of clinical and socioeconomic factors to differences in breast cancer subtype and mortality between Hispanic and non-Hispanic white women. Breast Cancer Res Treat. (2017) 166:185–93. doi: 10.1007/s10549-017-4389-z
21. Rey-Vargas L, Sanabria-Salas MC, Fejerman L, Serrano-Gómez SJ. Risk factors for triple-negative breast cancer among Latina women. Cancer Epidemiol Biomarkers Prev. (2019) 28:1771–83. doi: 10.1158/1055-9965.EPI-19-0035
22. Troester MA, Sun X, Allott EH, Geradts J, Cohen SM, Tse CK, et al. Racial differences in PAM50 subtypes in the Carolina breast cancer study. J Natl Cancer Inst. (2018) 110:176–82. doi: 10.1093/jnci/djx135
23. Chollet-Hinton L, Olshan AF, Nichols HB, Anders CK, Lund JL, Allott EH, et al. Biology and etiology of young-onset breast cancers among premenopausal African American women: results from the AMBER consortium. Cancer Epidemiol Biomarkers Prev. (2017) 26:1722–9. doi: 10.1158/1055-9965.EPI-17-0450
24. Walsh SM, Zabor EC, Stempel M, Morrow M, Gemignani ML. Does race predict survival for women with invasive breast cancer? Cancer. (2019) 125:3139–46. doi: 10.1002/cncr.32296
25. Paulozzi LJ, McDonald JA, Sroka CJ. A Disparity beneath a Paradox: Cancer Mortality among Young Hispanic Americans in the US-Mexico Border Region. J Racial Ethn Health Disparities. (2021) 8:1556–62. doi: 10.1007/s40615-020-00920-6
26. Bencomo-Alvarez AE, Gonzalez MA, Rubio AJ, Olivas IM, Lara JJ, Padilla O, et al. Ethnic and border differences on blood cancer presentation and outcomes: A Texas population-based study. Cancer. (2021) 127:1068–79. doi: 10.1002/cncr.33347
27. Yanez B, McGinty HL, Buitrago D, Ramirez AG, Penedo FJ. Cancer outcomes in Hispanics/Latinos in the United States: an integrative review and conceptual model of determinants of health. J Lat Psychol. (2016) 4:114–29. doi: 10.1037/lat0000055
28. Lopez R, Agullo P, Lakshmanaswamy R. Links between obesity, diabetes and ethnic disparities in breast cancer among Hispanic populations. Obes Rev. (2013) 14:679–91. doi: 10.1111/obr.12030
29. Flegal KM, Kruszon-Moran D, Carroll MD, Fryar CD, Ogden CL. Trends in obesity among adults in the United States, 2005 to 2014. Jama. (2016) 315:2284–91. doi: 10.1001/jama.2016.6458
30. Bandera EV, Hong CC, Qin B. Impact of Obesity and Related Factors in Breast Cancer Survivorship Among Hispanic Women. In: Ramirez AG, Trapido EJ, editors. Advancing the Science of Cancer in Latinos: Building Collaboration for Action. Springer, Cham (CH (2023). p. 163–76.
31. Morimoto Y, Conroy SM, Ollberding NJ, Kim Y, Lim U, Cooney RV, et al. Ethnic differences in serum adipokine and C-reactive protein levels: the multiethnic cohort. Int J Obes (Lond). (2014) 38:1416–22. doi: 10.1038/ijo.2014.25
32. Goran MI. Ethnic-specific pathways to obesity-related disease: the Hispanic vs. African-American paradox Obes (Silver Spring). (2008) 16:2561–5. doi: 10.1038/oby.2008.423
33. Romero-Corral A, Somers VK, Sierra-Johnson J, Thomas RJ, Collazo-Clavell ML, Korinek J, et al. Accuracy of body mass index in diagnosing obesity in the adult general population. Int J Obes (Lond). (2008) 32:959–66. doi: 10.1038/ijo.2008.11
34. Keum N, Greenwood DC, Lee DH, Kim R, Aune D, Ju W, et al. Adult weight gain and adiposity-related cancers: a dose-response meta-analysis of prospective observational studies. J Natl Cancer Inst. (2015) 107:djv088. doi: 10.1093/jnci/djv088
35. Velasco-Mondragon E, Jimenez A, Palladino-Davis AG, Davis D, Escamilla-Cejudo JA. Hispanic health in the USA: a scoping review of the literature. Public Health Rev. (2016) 37:31. doi: 10.1186/s40985-016-0043-2
36. Cortes-Bergoderi M, Goel K, Murad MH, Allison T, Somers VK, Erwin PJ, et al. Cardiovascular mortality in Hispanics compared to non-Hispanic whites: a systematic review and meta-analysis of the Hispanic paradox. Eur J Intern Med. (2013) 24:791–9. doi: 10.1016/j.ejim.2013.09.003
37. Agresti R, Meneghini E, Baili P, Minicozzi P, Turco A, Cavallo I, et al. Association of adiposity, dysmetabolisms, and inflammation with aggressive breast cancer subtypes: a cross-sectional study. Breast Cancer Res Treat. (2016) 157:179–89. doi: 10.1007/s10549-016-3802-3
38. Davis AA, Kaklamani VG. Metabolic syndrome and triple-negative breast cancer: a new paradigm. Int J Breast Cancer. (2012) 2012:809291. doi: 10.1155/2012/809291
39. Lee K, Kruper L, Dieli-Conwright CM, Mortimer JE. The impact of obesity on breast cancer diagnosis and treatment. Curr Oncol Rep. (2019) 21:41. doi: 10.1007/s11912-019-0787-1
40. Minicozzi P, Van Eycken L, Molinie F, Innos K, Guevara M, Marcos-Gragera R, et al. Comorbidities, age and period of diagnosis influence treatment and outcomes in early breast cancer. Int J Cancer. (2019) 144:2118–27. doi: 10.1002/ijc.31974
41. Hong CC, Ambrosone CB, Goodwin PJ. Comorbidities and their management: potential impact on breast cancer outcomes. Adv Exp Med Biol. (2015) 862:155–75. doi: 10.1007/978-3-319-16366-6_11
42. Yanez B, Thompson EH, Stanton AL. Quality of life among Latina breast cancer patients: a systematic review of the literature. J Cancer Surviv. (2011) 5:191–207. doi: 10.1007/s11764-011-0171-0
43. Connor AE, Baumgartner RN, Pinkston CM, Boone SD, Baumgartner KB. Obesity, ethnicity, and quality of life among breast cancer survivors and women without breast cancer: the long-term quality of life follow-up study. Cancer Causes Control. (2016) 27:115–24. doi: 10.1007/s10552-015-0688-z
44. Griggs JJ, Liu Y, Sorbero ME, Jagielski CH, Maly RC. Adjuvant chemotherapy dosing in low-income women: the impact of Hispanic ethnicity and patient self-efficacy. Breast Cancer Res Treat. (2014) 144:665–72. doi: 10.1007/s10549-014-2869-y
45. Griggs JJ, Sorbero ME, Lyman GH. Undertreatment of obese women receiving breast cancer chemotherapy. Arch Intern Med. (2005) 165:1267–73. doi: 10.1001/archinte.165.11.1267
46. Louwman WJ, Janssen-Heijnen ML, Houterman S, Voogd AC, van der Sangen MJ, Nieuwenhuijzen GA, et al. Less extensive treatment and inferior prognosis for breast cancer patient with comorbidity: a population-based study. Eur J Cancer. (2005) 41:779–85. doi: 10.1016/j.ejca.2004.12.025
47. Vulto AJ, Lemmens VE, Louwman MW, Janssen-Heijnen ML, Poortmans PH, Lybeert ML, et al. The influence of age and comorbidity on receiving radiotherapy as part of primary treatment for cancer in South Netherlands, 1995 to 2002. Cancer. (2006) 106:2734–42. doi: 10.1002/cncr.21934
48. Harlan LC, Klabunde CN, Ambs AH, Gibson T, Bernstein L, McTiernan A, et al. Comorbidities, therapy, and newly diagnosed conditions for women with early stage breast cancer. J Cancer Surviv. (2009) 3:89–98. doi: 10.1007/s11764-009-0084-3
49. Land LH, Dalton SO, Jensen MB, Ewertz M. Influence of comorbidity on the effect of adjuvant treatment and age in patients with early-stage breast cancer. Br J Cancer. (2012) 107:1901–7. doi: 10.1038/bjc.2012.472
50. de Heer HD, Balcazar HG, Morera OF, Lapeyrouse L, Heyman JM, Salinas J, et al. Barriers to care and comorbidities along the U.S.-Mexico border. Public Health Rep. (2013) 128:480–8. doi: 10.1177/003335491312800607
51. Clegg LX, Reichman ME, Hankey BF, Miller BA, Lin YD, Johnson NJ, et al. Quality of race, Hispanic ethnicity, and immigrant status in population-based cancer registry data: implications for health disparity studies. Cancer Causes Control. (2007) 18:177–87. doi: 10.1007/s10552-006-0089-4
52. Kaufman JS, Cooper RS. Commentary: considerations for use of racial/ethnic classification in etiologic research. Am J Epidemiol. (2001) 154:291–8. doi: 10.1093/aje/154.4.291
53. Schisterman EF, Cole SR, Platt RW. Overadjustment bias and unnecessary adjustment in epidemiologic studies. Epidemiology. (2009) 20:488–95. doi: 10.1097/EDE.0b013e3181a819a1
54. Dwivedi AK. How to write statistical analysis section in medical research. J Investig Med. (2022) 70:1759–70. doi: 10.1136/jim-2022-002479
55. Dwivedi AK, Shukla R. Evidence-based statistical analysis and methods in biomedical research (SAMBR) checklists according to design features. Cancer Rep (Hoboken). (2020) 3:e1211. doi: 10.1002/cnr2.1211
56. O’Brien KM, Cole SR, Tse CK, Perou CM, Carey LA, Foulkes WD, et al. Intrinsic breast tumor subtypes, race, and long-term survival in the Carolina Breast Cancer Study. Clin Cancer Res. (2010) 16:6100–10. doi: 10.1158/1078-0432.CCR-10-1533
57. Lipsyc-Sharf M, Ballman KV, Campbell JD, Muss HB, Perez EA, Shulman LN, et al. Age, body mass index, tumor subtype, and racial and ethnic disparities in breast cancer survival. JAMA Netw Open. (2023) 6:e2339584. doi: 10.1001/jamanetworkopen.2023.39584
58. Serrano-Gomez SJ, Sanabria-Salas MC, Fejerman L. Breast cancer health disparities in hispanics/latinas. Curr Breast Cancer Rep. (2020) 12:175–84. doi: 10.1007/s12609-020-00370-3
59. Zavala VA, Bracci PM, Carethers JM, Carvajal-Carmona L, Coggins NB, Cruz-Correa MR, et al. Cancer health disparities in racial/ethnic minorities in the United States. Br J Cancer. (2021) 124:315–32. doi: 10.1038/s41416-020-01038-6
60. Castellanos MI, Oluyomi AO, Chambers TM, Gramatges MM, Winestone LE, Lupo PJ, et al. Ethnic disparities in childhood leukemia survival by border residence: A Texas population-based analysis. Cancer. (2023) 129:1276–86. doi: 10.1002/cncr.34636
61. Zamora SM, Pinheiro PS, Gomez SL, Hastings KG, Palaniappan LP, Hu J, et al. Disaggregating Hispanic American cancer mortality burden by detailed ethnicity. Cancer Epidemiol Biomarkers Prev. (2019) 28:1353–63. doi: 10.1158/1055-9965.EPI-18-0872
62. United States Census Bureau. QuickFacts El Paso County, Texas. Texas (2019). Available online at: https://www.census.gov/quickfacts/fact/table/elpasocountytexas/.
63. Larsen IK, Myklebust TA, Babigumira R, Vinberg E, Moller B, Ursin G. Education, income and risk of cancer: results from a Norwegian registry-based study. Acta Oncol. (2020) 59:1300–7. doi: 10.1080/0284186X.2020.1817548
64. Bird Y, Moraros J, Banegas MP, King S, Prapasiri S, Thompson B. Breast cancer knowledge and early detection among Hispanic women with a family history of breast cancer along the U.S.-Mexico border. J Health Care Poor Underserved. (2010) 21:475–88. doi: 10.1353/hpu.0.0292
65. Monnat SM. The new destination disadvantage: disparities in Hispanic health insurance coverage rates in metropolitan and nonmetropolitan new and established destinations. Rural Sociol. (2017) 82:3–43. doi: 10.1111/ruso.12116
66. Smith EC, Ziogas A, Anton-Culver H. Delay in surgical treatment and survival after breast cancer diagnosis in young women by race/ethnicity. JAMA Surg. (2013) 148:516–23. doi: 10.1001/jamasurg.2013.1680
67. Philipovskiy A, Campbell A, Heydarian R, et al. Adherence to adjuvant aromatase inhibitor therapy among postmenopausal Hispanic/Latino women with breast cancer. Anticancer Res. (2020) 40:857–64. doi: 10.21873/anticanres.14018
68. Stringer-Reasor EM, Elkhanany A, Khoury K, Simon MA, Newman LA. Disparities in breast cancer associated with African American identity. Am Soc Clin Oncol Educ Book. (2021) 41:e29–46. doi: 10.1200/EDBK_319929
69. Keegan THM, Abrahão R, Alvarez EM. Survival trends among adolescents and young adults diagnosed with cancer in the United States: comparisons with children and older adults. J Clin Oncol. (2024) 42:631–41. doi: 10.1007/978-3-319-16366-6_11
70. De Oliveira TC, Secolin R, Lopes-Cendes I. A review of ancestrality and admixture in Latin America and the caribbean focusing on native American and African descendant populations. Front Genet. (2023) 14:1091269. doi: 10.3389/fgene.2023.1091269
71. Sangaramoorthy M, Hines LM, Torres-Mejía G, Phipps AI, Baumgartner KB, Wu AH, et al. A pooled analysis of breastfeeding and breast cancer risk by hormone receptor status in Parous Hispanic women. Epidemiology. (2019) 30:449–57. doi: 10.1097/EDE.0000000000000981
72. Siegel RL, Miller KD, Fuchs HE, Jemal A. Cancer statistics, 2022. CA Cancer J Clin. (2022) 72:7–33. doi: 10.3322/caac.21708
73. Stern MC, Fejerman L, Das R, Setiawan VW, Cruz-Correa MR, Perez-Stable EJ, et al. Variability in cancer risk and outcomes within US Latinos by national origin and genetic ancestry. Curr Epidemiol Rep. (2016) 3:181–90. doi: 10.1007/s40471-016-0083-7
74. Chiang KV, Li R, Anstey EH, Perrine CG. Racial and ethnic disparities in breastfeeding initiation ─ United States, 2019. MMWR Morb Mortal Wkly Rep. (2021) 70:769–74. doi: 10.15585/mmwr.mm7021a1
75. Pinheiro PS, Callahan KE, Stern MC, de Vries E. Migration from Mexico to the United States: A high-speed cancer transition. Int J Cancer. (2018) 142:477–88. doi: 10.1002/ijc.31068
76. Yedjou CG, Sims JN, Miele L, Noubissi F, Lowe L, Fonseca DD, et al. Health and racial disparity in breast cancer. Adv Exp Med Biol. (2019) 1152:31–49. doi: 10.1007/978-3-030-20301-6_3
77. Bandoli G, Palmsten K, Flores KF, Chambers CD. Constructing causal diagrams for common perinatal outcomes: benefits, limitations and motivating examples with maternal antidepressant use in pregnancy. Paediatr Perinat Epidemiol. (2016) 30:521–8. doi: 10.1111/ppe.12302
78. Staaf J, Glodzik D, Bosch A, Vallon-Christersson J, Reuterswärd C, Häkkinen J, et al. Whole-genome sequencing of triple-negative breast cancers in a population-based clinical study. Nat Med. (2019) 25:1526–33. doi: 10.1038/s41591-019-0582-4
79. Martini R, Chen Y, Jenkins BD, Elhussin IA, Cheng E, Hoda SA, et al. Investigation of triple-negative breast cancer risk alleles in an International African-enriched cohort. Sci Rep. (2021) 11:9247. doi: 10.1038/s41598-021-88613-w
Keywords: race/ethnicity, breast cancer (BC), United States/Mexico border, population-based study, cancer health disparities
Citation: Nhim V, Bencomo-Alvarez AE, Alvarado L, Kilcoyne M, Gonzalez-Henry MA, Olivas IM, Keivan M, Gaur S, Mulla ZD, Dwivedi AK, Gadad SS and Eiring AM (2024) Racial/ethnic differences in the clinical presentation and survival of breast cancer by subtype. Front. Oncol. 14:1443399. doi: 10.3389/fonc.2024.1443399
Received: 04 June 2024; Accepted: 22 July 2024;
Published: 16 August 2024.
Edited by:
Kate E. Dibble, Dana–Farber Cancer Institute, United StatesReviewed by:
Andrea Sabina Llera, IIBBA-CONICET Leloir Institute Foundation, ArgentinaCopyright © 2024 Nhim, Bencomo-Alvarez, Alvarado, Kilcoyne, Gonzalez-Henry, Olivas, Keivan, Gaur, Mulla, Dwivedi, Gadad and Eiring. This is an open-access article distributed under the terms of the Creative Commons Attribution License (CC BY). The use, distribution or reproduction in other forums is permitted, provided the original author(s) and the copyright owner(s) are credited and that the original publication in this journal is cited, in accordance with accepted academic practice. No use, distribution or reproduction is permitted which does not comply with these terms.
*Correspondence: Shrikanth S. Gadad, c2hyaWthbnRoLmdhZGFkQHR0dWhzYy5lZHU=; Anna M. Eiring, YW5uYS5laXJpbmdAdHR1aHNjLmVkdQ==
Disclaimer: All claims expressed in this article are solely those of the authors and do not necessarily represent those of their affiliated organizations, or those of the publisher, the editors and the reviewers. Any product that may be evaluated in this article or claim that may be made by its manufacturer is not guaranteed or endorsed by the publisher.
Research integrity at Frontiers
Learn more about the work of our research integrity team to safeguard the quality of each article we publish.