- 1Section of Innovation Biomedicine – Oncology Area, Department of Engineering for Innovation Medicine (DIMI), University of Verona and University and Hospital Trust/Azienda Ospedaliero-Universitaria Integrata (AOUI), Verona, Italy
- 2Otolaryngology-Head and Neck Surgery Department, University of Verona Hospital Trust, Verona, Italy
- 3Department of Diagnostics and Public Health, Section of Pathology, University and Hospital Trust of Verona, Verona, Italy
- 4Medical Oncology Unit, Azienda Ospedaliera (AO) San Giovanni Addolorata Hospital, Rome, Italy
- 5Medical Oncology Unit, San Giuseppe Moscati Hospital, Taranto, Italy
- 6Section of Oncology, Azienda Ospedaliera (AO) Ospedali Riuniti “Villa Sofia- V. Cervello”, Palermo, Italy
- 7Department of Clinical Oncology, San Bortolo General Hospital, Azienda ULSS8 Berica, Vicenza, Italy
- 8Department of Experimental Medicine, Sapienza University, Rome, Italy
- 9Medical Oncology Department, Fondazione Istituti di Ricovero e Cura a Carattere Scientifico (IRCCS) Istituto Nazionale Dei Tumori, Milan, Italy
- 10Division of Medical Oncology B, Policlinico Umberto I, Rome, Italy
- 11Medical Oncology Department, Santa Chiara Hospital, Trento, Italy
- 12Lung Unit, Ospedale Pederzoli, Peschiera del Garda, Verona, Italy
- 13Medical Oncology, Azienda Ospedaliero Universitaria Policlinico “G. Rodolico-S. Marco”, Catania, Italy
- 14Department of Medical Oncology, Unità Operativa Complessa (UOC) Oncologia, Taormina, Italy
- 15Medical Oncology Unit, San Giovanni di Dio e Ruggi d’Aragona, Salerno, Italy
- 16AdRes Health Economics and Outcome Research, Turin, Italy
- 17Roche S.p.A., Monza, Italy
- 18Department of Public Health, University Federico II of Naples, Naples, Italy
- 19Department of Oncology, San Luigi Gonzaga Hospital, University of Turin, Orbassano, Italy
- 20Comprehensive Cancer Center, Fondazione Policlinico Universitario Agostino Gemelli Istituti di Ricovero e Cura a Carattere Scientifico (IRCCS), Roma, Italy
- 21Medical Oncology, Università Cattolica del Sacro Cuore, Roma, Italy
Introduction: To date, for all non-small cell lung cancer (NSCLC) cases, it is recommended to test for driver alterations to identify actionable therapeutic targets. In this light, comprehensive genomic profiling (CGP) with next generation sequencing (NGS) has progressively gained increasing importance in clinical practice. Here, with the aim of assessing the distribution and the real-world frequency of gene alterations and their correlation with patient characteristics, we present the outcomes obtained using FoundationOne (F1CDx) and FoundationLiquid CDx (F1L/F1LCDx) NGS-based profiling in a nationwide initiative for advanced NSCLC patients.
Methods: F1CDx (324 genes) was used for tissue samples, and F1L (70 genes) or F1LCDx (324 genes) for liquid biopsy, aiming to explore the real-world occurrence of molecular alterations in aNSCLC and their relationship with patients’ characteristics.
Results: Overall, 232 advanced NSCLC patients from 11 Institutions were gathered [median age 63 years; never/former or current smokers 29.3/65.9%; adenocarcinoma/squamous 79.3/12.5%; F1CDx/F1L+F1LCDx 59.5/40.5%]. Alterations were found in 170 different genes. Median number of mutated genes per sample was 4 (IQR 3–6) and 2 (IQR 1–3) in the F1CDx and F1L/F1LCDx cohorts, respectively. TP53 (58%), KRAS (22%), CDKN2A/B (19%), and STK11 (17%) alterations were the most frequently detected. Actionability rates (tier I and II) were comparable: 36.2% F1CDx vs. 34% ctDNA NGS assays (29.5% and 40.9% F1L and F1LCDx, respectively). Alterations in KEAP1 were significantly associated with STK11 and KRAS, so as TP53 with RB1. Median tumor mutational burden was 6 (IQR 3–10) and was significantly higher in smokers. Median OS from metastatic diagnosis was 23 months (IQR 18.5–19.5) and significantly lower in patients harboring ≥3 gene mutations. Conditional three-year survival probabilities increased over time for patients profiled at initial diagnosis and exceeded those of individuals tested later in their clinical history after 12 months.
Conclusion: This study confirms that NGS-based molecular profiling of aNSCLC on tissue or blood samples offers valuable predictive and prognostic insights.
1 Background
Lung cancer is the second most common cancer in the world and the first cause of cancer-related deaths (1). In Italy, lung cancer ranks second and third in incidence in men (15% of all tumors) and women (6% of all tumors), respectively, and accounted for 34,000 estimated deaths in 2021 (2). Histologically, most cases are categorized as non-small-cell lung cancer (NSCLC) and approximately half of the patients are diagnosed with metastatic disease (3).
The therapeutic landscape has evolved to encompass oncogene-addicted (15–20%), for whom molecularly targeted agents are available over multiple lines of treatment, and non-oncogene addicted disease (80–85%), for whom immune checkpoint blockade, alone or combined with chemotherapy, is the preferred treatment path (4), resulting in a dramatic change in survival and quality of life for advanced NSCLC patients.
Most actionable oncogenic alterations occur in lung adenocarcinoma (LUAD), the most common NSCLC subtype (5, 6). Actionable drivers in lung LUAD involve the epidermal growth factor receptor (EGFR), KRAS, ALK genes and, less commonly, ROS1, BRAF, MET, RET, ERBB2, NTRK, and NRG1 (5, 6). Drivers found in squamous cell carcinoma (SCC, accounting for 35% of NSCLC patients) are much less frequently actionable and include TP53, PIK3CA, CDKN2A, SOX2, and CCND1 alterations (4).
Testing for driver alterations is recommended for all NSCLC cases to find actionable therapeutic targets (6). To this purpose, different techniques may be used: immunohistochemistry (IHC), fluorescence in situ hybridization (FISH), multiplex reverse transcriptase-polymerase chain reaction (RT-PCR) panel assays, in situ hybridization (ISH), or comparative genomic hybridization. However, all relevant molecular alterations may also be detected by next generation sequencing (NGS). In that respect, ESMO guidelines (6) state that “if available, multiplex platforms (NGS) for molecular testing are preferable”, favoring the use of RNA- or DNA-based NGS designed to capture gene fusions (7).
Comprehensive genomic profiling (CGP) with NGS allows for multigene sequencing in a unique sample, identification of structural variants and genomic signatures and has predictive, prognostic, and therapeutic impact. Although the benefit of broad-based genomic sequencing, defined as any multigene panel testing more than 30 genes, remains debated (8), CGP is becoming increasingly easy and cheap, thus growing in utility with a larger number of patients (9). Previously unreported alterations are now routinely found, thus making it difficult to interpret genomic testing, as only 2% of somatic alterations are known oncogenic events (9). Moreover, non-invasive testing using blood as the source biological material and circulating free DNA (cfDNA) or circulating tumor DNA (ctDNA) as the substrate for CGP (liquid biopsy) is becoming increasingly used as an alternative to tissue-based profiling (10).
FoundationOne®CDx (F1CDx; Foundation Medicine, Inc., Cambridge, MA, USA) is a qualitative, NGS-based in vitro diagnostic test (11). Using a targeted high throughput hybridization-based capture technology, it is able to detect substitutions, insertion and deletion alterations (indels), copy number alterations (CNAs), and gene rearrangements in 324 genes, genomic biomarkers including microsatellite instability (MSI), and tumor mutational burden (TMB) (11). Source material is formalin-fixed, paraffin-embedded (FFPE) tumor tissue specimens (11) FoundationOne®Liquid (F1L; Foundation Medicine, Inc., Cambridge, MA, USA) and FoundationOne®Liquid CDx (F1LCDx; Foundation Medicine, Inc., Cambridge, MA, USA) are other NGS-based in vitro diagnostic tests (12) that use targeted high throughput hybridization-based capture technology to detect and report alterations in 70 and 324 genes, respectively, from plasma derived from anti-coagulated peripheral whole blood.
The IMpact of broad genoMic profIling oN advancEd NSCLC ouTcome (IMMINENT) study aimed at assessing the distribution and the real-world frequency of gene alterations and their correlation with patient characteristics in a population of advanced NSCLC patients; it also sought to evaluate the clinical impact of molecular profiling and the timing of CGP with respect to the line of treatment.
2 Materials and methods
2.1 Study design and informed consent statement
This retrospective real-world data analysis leveraged a clinical-genomic database including anonymized patient-level data of NSCLC patients who underwent CGP within a national FoundationOne access program in 11 Italian Oncological centers between May 2019 and November 2022. Data had been previously collected and stored by the University Hospital Trust in Verona in accordance with the Helsinki declaration, as all the patients signed the informed consent form (ICF) for secondary use of their data for research purposes. Data included clinico-pathological features, treatment history, and tumor profiling results. Genomic analysis was performed using the F1CDx assay on tumor tissue specimens or using circulating tumor DNA (ctDNA) profiling assays, including F1L and F1LCDx (the latter launched in August 2020) on DNA from blood sampling.
2.2 Inclusion and exclusion criteria
Patients included in this study were adults with histologically confirmed advanced NSCLC diagnosis who were profiled by NGS test using tissue biopsies (F1CDx) or blood samples (F1L and F1LCDx).
Exclusion criteria were patients<18 years of age, lack of medical reports, and unwillingness or impossibility to sign the written ICF.
2.3 NGS analysis
F1CDx analyzes DNA extracted from formalin-fixed paraffin-embedded (FFPE) tumor samples. Specimens with at least 50 ng of DNA were used for library construction. Hybridization capture and multiplex sequencing were performed to a mean coverage depth of >550X for 324 cancer-related genes. Sequencing data were processed using a proprietary bioinformatics pipeline, which was designed to detect base substitutions, indels, CNAs, gene rearrangements, TMB, and MSI (13–15).
For F1L and its new version F1LCDx assays, circulating free DNA (cfDNA) was obtained from plasma derived from peripheral whole blood. Extracted cfDNA underwent whole-genome shotgun library construction and hybridization-based capture of 70 and 324 genes. Then, selected libraries were sequenced with deep coverage (median depth >6000x).
Testing were conducted to evaluate the impact of a range of cfDNA input masses (50% below the lower limit and 33% above the upper limit) for F1LCDx using an updated library construction input range (20-60ng).
The assays reported base substitutions, indels, and selected CNAs and gene rearrangements (16, 17). F1LCDx also detected genomic signatures, such as MSI and blood TMB (bTMB), while F1L reported only MSI.
Only known or likely pathogenic alterations were considered in this study.
TMB was measured by counting somatic, non-driver coding mutations per megabase (mut/Mb) of coding genome (14, 17). The MSI status was based on genome wide analysis across >2000 microsatellite loci, and was generated by calculating the fraction of unstable ones (containing a repeated length not present in an internal database generated using >3000 clinical samples) (14, 17).
2.4 Actionability classification
Genomic alterations classified as tier I-III mutations according to ESCAT (6, 7) were analyzed.
2.5 Statistical analysis
Statistical analysis was performed using R (version 4.1.2). Descriptive statistics included percentages and frequencies for categorical variables, and median and interquartile range (IQR) for continuous variables. Categorical variables were compared using the χ2 or Fisher exact test when appropriate, while continuous variables were compared using the Mann-Whitney U or Kruskal Wallis test. Comparisons between proportions were analyzed by Fisher’s exact test. Multiple testing correction was applied using the Benjamin-Hochberg (BH) method. The overall survival from histological (hOS) and metastatic (mOS) diagnosis were analyzed using Kaplan-Meier (KM) method, and the log rank test was used to compare the survival curves among the groups. Conditional survival (CS) was computed using the multiplicative law of probability. CS(y|x) can be defined as the probability of surviving additional y years, given that the patient has already survived x years. It can be expressed as:
A p-value<0.05 was considered statistically significant.
3 Results
3.1 Patient characteristics
A total of 246 NSCLC patients were profiled by tissue- and ctDNA-based NGS assays (F1CDx, F1L, and F1LCDx). Tissue-based F1CDx assay was performed in 146 (59.3%) patients, while the remaining 100 (40.7%) were liquid biopsy samples, 78 (78%) and 22 (22%) of which tested by F1L and F1LCDx, respectively. The proportion of testing failure (no results) was 5.5% (8 out of 146) for tissue sequencing and 6% (6 out of 100) for ctDNA testing (all with F1L). Overall, analyses were completed for 232 samples, with a success rate of 94.3%. For those patients in whom molecular profiling failed, further analysis through liquid biopsy was not proposed, as per protocol.
Table 1 and Supplementary Data 1, Supplementary Figure S1, summarize patients’ clinico-pathological characteristics; differences between the patients tested with tissue- and ctDNA-based NGS were statistically significant.
3.2 Mutational profile of NSCLC
A total of 923 genomic alterations were detected in 170 genes, with a median number of altered genes per sample of 3 (IQR: 2–5). Of the 232 successfully tested samples, 219 (94.4%) exhibited one or more genomic alterations, with a significantly lower number of altered genes per sample detected by F1L, as compared to F1CDx and F1LCDx (p<0.001, Supplementary Data 1, Supplementary Figure S2). The genomic profile of the analyzed NSCLC samples is presented in Figure 1A.
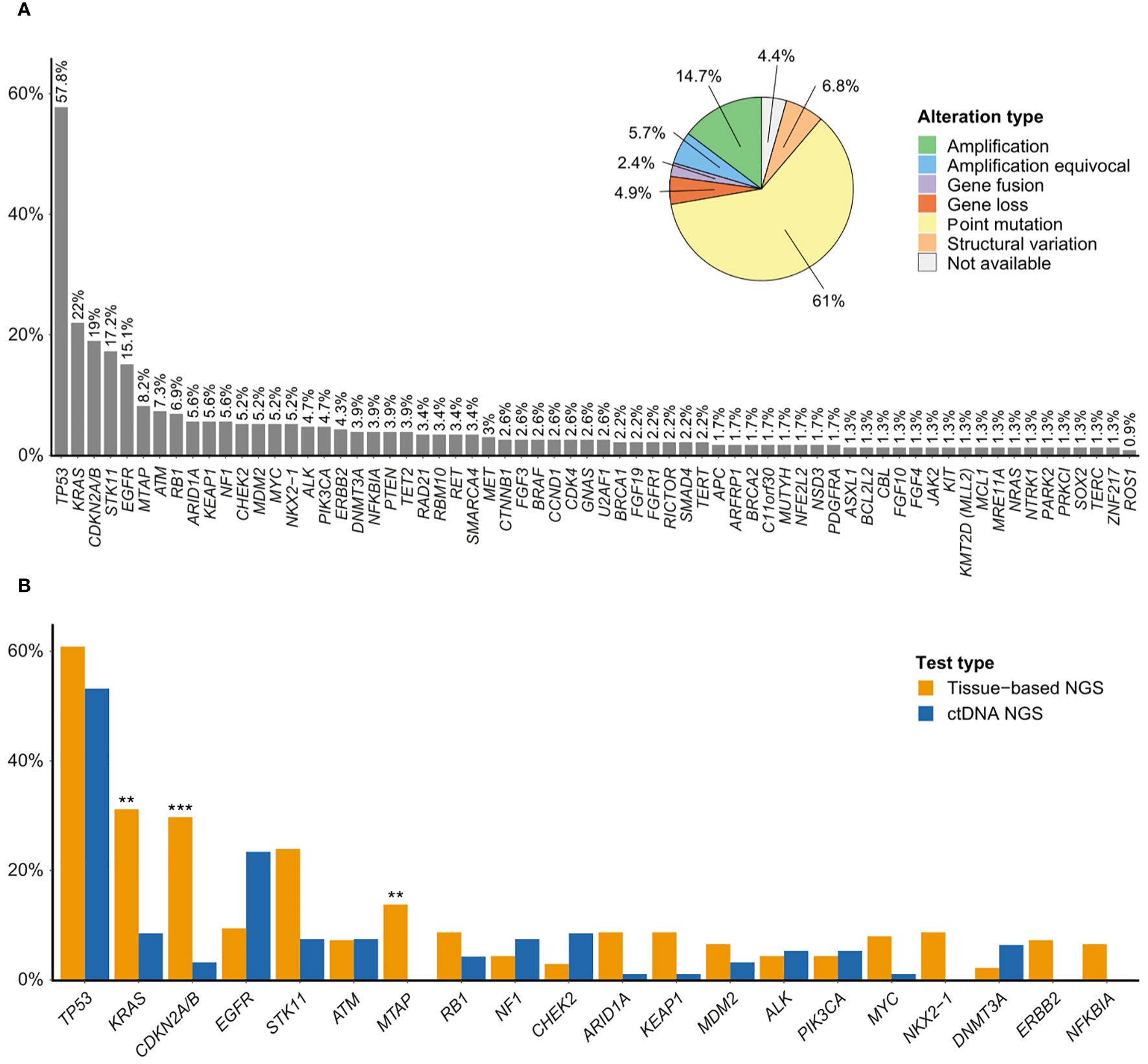
Figure 1 (A) Mutation profile of NSCLC. Figure shows driver genes and genes mutated in at least 1% of the patients, and distribution of alteration types. (B) Comparison of gene frequencies between tissue-based NGS (n=138) and ctDNA NGS (n=94) (top-20 mutated genes). The Y-axis indicates the percentage of patients with the mutated genes across the X-axis for groups under study. **Adjusted p-value<0.01; ***Adjusted p-value<0.001.
The top frequently altered genes included TP53 (57.8%), KRAS (22%), CDKN2A/B (19%; comprising 50% gene loss and 9.1% structural variation), STK11 (17.2%), and EGFR (15.1%). Most variant types were point mutations (61%), followed by amplifications (14.7%), and structural variations (6.8%), which included insertions, deletions, truncations, large-scale gene losses, and gene rearrangements.
The comparison of gene frequencies between solid and liquid biopsy is presented in Figure 1B and Supplementary Data 1, Supplementary Table S1. Notable differences between tissue-based and ctDNA NGS included KRAS (31.2% vs. 8.5%, p<0.01), CDKN2A/B (29.7% vs. 3.2%, p<0.01), and MTAP (13.8% vs. 0%, p<0.01). Furthermore, ctDNA sequencing did not detect any alterations in NKX2-1, ERBB2, and NFKBIA. Mutations in EGFR were detected more frequently in liquid biopsy samples compared to tissue specimens, albeit the differences were non-significant, after adjusting for multiple comparisons.
For the top-20 mutated genes, associations between mutations and clinical characteristics are depicted in Supplementary Figures S3A–C. KRAS and STK11 mutation rates were more frequent in ever- than in never-smokers (KRAS: 29.4% ever-smoker vs. 7.4% never-smoker, p=0.03; STK11: 22.2% vs. 4.4%, p=0.06). Conversely, ALK alterations were more frequent in never-smokers, although this difference did not reach statistical significance (2.3% in ever-smokers vs. 11.8% in never-smokers, p=0.09). Additional information on the comparison of gene alteration frequencies is presented in Supplementary Data 1, Supplementary Tables S2–S4.
3.3 Actionability
Frequencies and types of oncogenic driver alterations across all samples are presented in Supplementary Data 1, Supplementary Table S1 and S5. Based on the ESCAT actionability scale, we identified 82 cases (35.3%) harboring at least one actionable gene alteration (74 [31.9%] tier I and 8 [3.5%] tier II), and 10 (4.3%) additional cases had an ESCAT tier III biomarker (Figure 2). Identified targetable genomic variants were: EGFR mutations (3.4%) and structural variations (including exon 19 deletion and exon 20 insertion, 8.6%; considering that in some patients both were detected, the total patients involved by EGFR mutations were 11.6%), KRAS mutations (G12C: 9.1%), ALK fusions (3.9%) and rearrangements (0.4%), RET fusions (3.4%, with 0.4% of patients carrying also a rearrangement in the same gene), MET exon 14 skipping mutations (2.2%) and amplifications (0.9%), BRAF mutations (V600E: 0.9%), NTRK1 fusions (0.9%, with 0.4% of patients carrying also a rearrangement in the same gene), ROS1 fusions (0.9%), and ERBB2 mutations (3%). The frequency of ESCAT tier III mutations was 3.4% for PIK3CA, 1.7% for BRCA1, and 1.3% for BRCA2.
Actionability rates (tier I and II) were comparable across panel types: 36.2% F1CDx vs. 34% ctDNA NGS assays (29.5% and 40.9% F1L and F1LCDx, respectively).
3.4 TMB and MSI status
TMB was evaluable for 123 (89.1%) and 22 (100%) patients profiled by F1CDx and F1LCDx respectively. The difference in TMB between the two groups was on the verge of statistical significance, with a higher TMB in tissue specimens compared to liquid biopsy samples (median TMB: 6 for F1CDx vs. 3.5 for F1LCDx, p=0.051) (Figure 3A). In the whole cohort, the median TMB was 6 mut/Mb (IQR: 3–10) and 28.3% of patients exhibited TMB ≥10 mut/Mb. Significantly higher TMB was observed in ever-smokers (Figure 3B); TMB also tended to be higher in SCC tumors (vs. LUAD; Supplementary Figure S4).
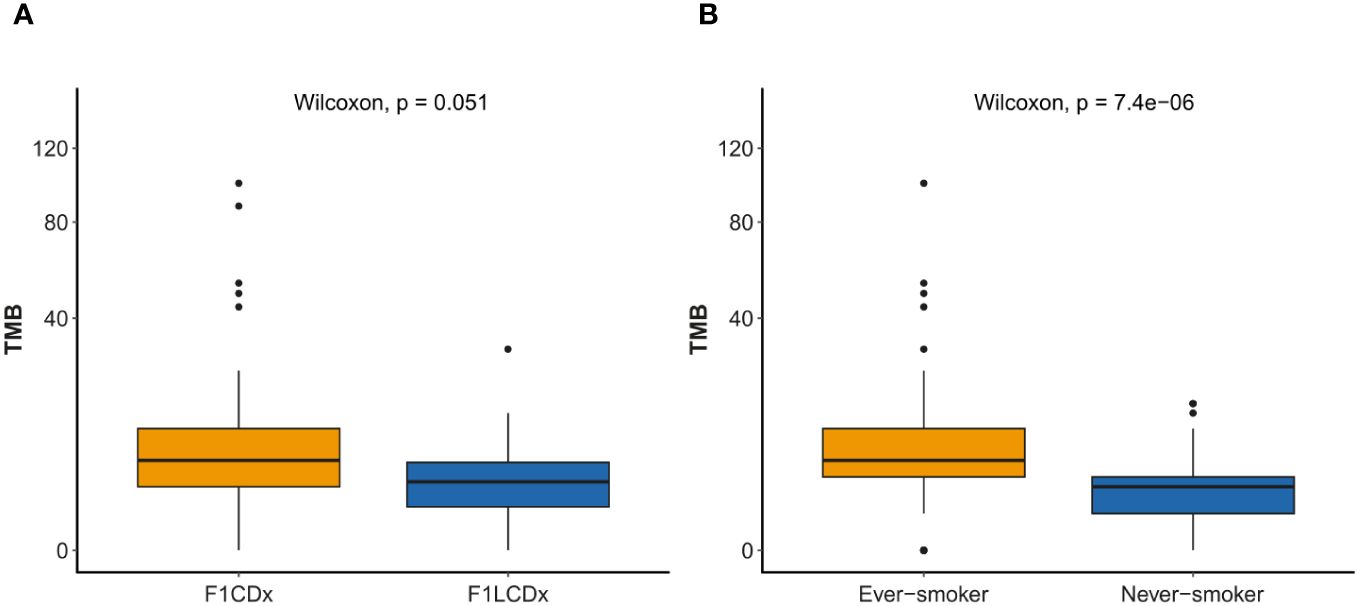
Figure 3 TMB comparison of genomic testing and smoking status. Y-axis presented in square root transformation. (A) F1CDx (n=123) vs. F1LCDx (n=22). (B) Ever-smoker (n=101) vs. never-smoker (n= 37). TMB, tumor mutational burden.
Except for 2 cases with microsatellite instability, all tumors for which MSI status was available (135, 58.2%) were stable. The proportion of undetermined MSI status was significantly higher in liquid biopsy samples compared to tissue specimens (Supplementary Data 1, Supplementary Figure S5, p<0.001), as, by design, specimens assayed using F1L are reported as “Unknown” if MSI-H (high) is not detected.
3.5 Co-occurrence
We investigated the distribution of co-occurring alterations in driver genes (Supplementary Data 1, Supplementary Figure S6) and analyzed the statistical significance of mutual exclusivity and co-occurrence of the most frequent variants in this cohort (Figure 4).
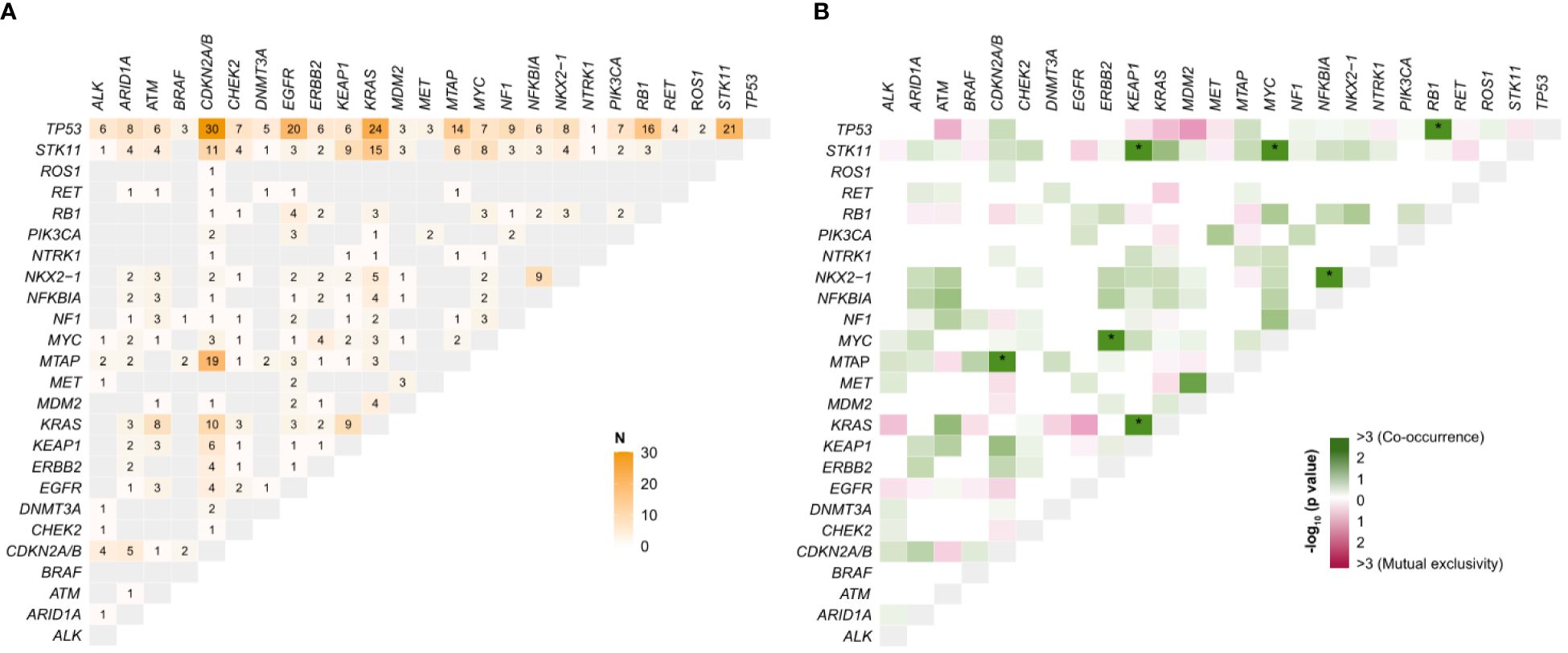
Figure 4 (A) Number of co-occurrences between gene pairs. (B) Mutual exclusivity and co-occurrence of top-20 genes/driver genes in 232 NSCLC tumors. P-values (not adjusted) were calculated using Fisher’s exact test and transformed into a score, -log10(P-value). *Adjusted p-value<0.05; N, number.
Concurrently mutated genes were identified in 187 (80.6%) patients. TP53 represented the most prevalent co-alteration in all tumors (Figure 4A) and significantly co-occurred with RB1 (Figure 4B, p<0.01). KEAP1 co-mutations were highly represented in tumors with KRAS (9/51, 17.6%; p=0.01) and STK11 (9/40, 22.5%; p<0.01) alterations. Despite the propensity for mutual exclusivity, we discovered 3 (5.9%) and 24 (47.1%) KRAS-mutant patients harboring EGFR and TP53 alterations, respectively. The spectrum of KRAS co-mutations also comprised aberrations in STK11 (15/51, 29.4%) and CDKN2A/B (10/51, 19.6%) (Supplementary Data 1, Supplementary Figure S6A). Among EGFR-mutant patients, TP53 alterations were detected in 57.1% (20/35) of cases, followed by PTEN (5/35, 14.3%), CDKN2A/B (4/35, 11.4%), and RB1 (4/35, 11.4%) (Supplementary Data 1, Supplementary Figure S6B). ERBB2-mutant patients were significantly enriched with MYC (4/10, 40%; p=0.03), whereas ALK mostly co-occurred with TP53 (6/11, 54.5%) and CDKN2A/B (4/11, 36.7%) (Supplementary Data 1, Supplementary Figures S6C, D). MTAP/CDKN2A/B, MYC/STK11, and NFKBIA/NKX2-1 were other co-occurrent variants with statistical significance. These results mostly apply to patients who underwent tissue biopsy. Due to the low number of cases, patients profiled by F1L/F1LCDx exhibited a less obvious pattern (Supplementary Data 1, Supplementary Figure S7), with no significant co-occurrences and a non-significant tendency of MET-mutated tumors towards MDM2 enrichment.
3.6 Overall survival
Survival data were available for 229 (98.7%) patients, 217 of whom (94.6%) with metastatic disease. The median hOS and mOS were 27.8 (95% CI: 24.2–42.7) and 23 (95% CI: 18.5–26.7) months, respectively, with a 5-year survival rate of 29.3% (hOS; 95% CI: 22.1–39%) and 18.6% (mOS; 95% CI: 12.1–28.7%). In an explorative univariate analysis, a higher number of altered genes was associated with worse hOS and mOS (p<0.01; Figure 5, Supplementary Figure S8G).
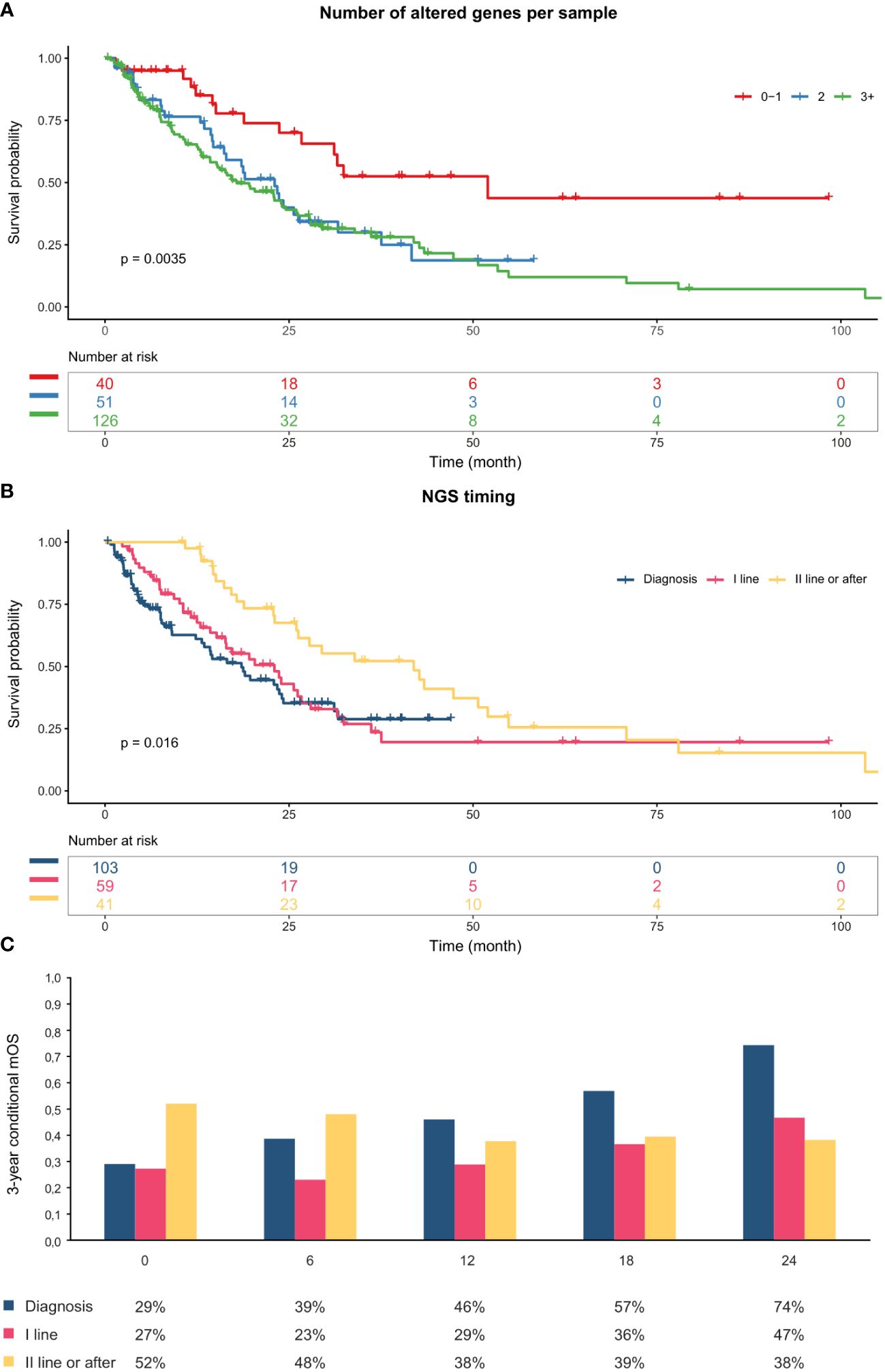
Figure 5 (A) Kaplan-Meier Curves indicating overall survival from metastatic diagnosis stratified by number of altered genes per sample. (B) Kaplan-Meier Curves indicating overall survival from metastatic diagnosis stratified by NSG timing. (C) Three-year conditional mOS by NGS timing. The x-axis represents the duration of survival to date (6-month intervals). All patients in the “Diagnosis” group died within 42 months; therefore, they were assumed to have a constant survival rate from that point onwards. mOS, overall survival from metastatic diagnosis.
Never-smokers had longer hOS than those with a smoking history (p = 0.041; Supplementary Data 1, Supplementary Figure S8E), and patients with stage at diagnosis I-II had longer hOS than patients who were diagnosed with stage III or IV (p<0.0001; Supplementary Data 1, Supplementary Figure S8A). Kaplan-Meier curves also indicated poorer survival in patients with higher ECOG PS (p<0.0001; Supplementary Data 1, Supplementary Figures S8C, D).
We also analyzed the impact of the timing of NGS tests on OS. The majority of the patients underwent single-gene analysis, at least for EGFR evaluation (94% of patients), at the time of diagnosis. Most NGS tests (114, 49.1%) were performed after initial diagnosis or before starting the first line of therapy; 59 (25.4%) were performed when first-line treatment was ongoing; all others were performed before or during subsequent lines (41, 17.7%). For 18 (7.8%), this information was not available.
Patients who were profiled at or after second line display longer mOS, as compared with subjects tested at the time of diagnosis or during the first-line treatment (Figure 5B). However, conditional one- and three-year survival probabilities increased over time for patients profiled at initial diagnosis and exceeded those of individuals tested later in their clinical history after 36 and 12 months, respectively (Figure 5C, Supplementary Data 1, Supplementary Figure S9).
4 Discussion
In this study, we analyzed the results of CGP performed on either tissue or liquid biopsy samples in a real-world population of Italian NSCLC patients. The patient population analyzed was strikingly similar to the NSCLC population recently reported in the context of the RATIONAL Italian registry study (18), encompassing advanced disease, 85% adenocarcinoma histology, and approximately 45% of tests performed at diagnosis or at I-line treatment start. These figures reflect current indications for NGS testing in Italy (5). In line with indications to liquid biopsy at the time of testing, the population of patients who underwent ctDNA NGS was significantly skewed towards younger, female, adenocarcinoma patients, less heavily exposed to cigarette smoke, who were metastatic at diagnosis. Regardless of the sample source (tissue or ctDNA), NGS tools had a very high success rate in sequencing, i.e., a mean of 94.3%, which is in line with the relevant technical information and other reported series (11, 12, 19, 20).
The number and types of gene alterations (in particular those falling in ESCAT tiers I/II) reported in our experience are in line with the literature (6, 7). MET alterations were slightly less prevalent in our cohort, primarily due to the lower occurrence of high-level amplifications (0.9% compared with 2-4% reported in the literature (21, 22)). This discrepancy may be due to the rarity of these mutations and the low sample size of our cohort, as well as to the lower ability of ct-DNA-based methods (employed in approximately 41% of our cohort) to detect MET amplifications (23). Exon 14 skipping mutation rate (2.2%) was consistent with that reported in the literature (3%) (6).
Significant differences between tissue-based and ctDNA NGS included KRAS, CDKN2A/B, and MTAP. KRAS frequency was significantly higher in cases tested with the tissue-based F1CDx than in those tested by ctDNA-based panels (F1L/F1LCDx). This may be due to a selection bias skewing the population subjected to liquid testing towards second or further lines and enriching it for EGFR-mutant cases progressing on TKI treatment; indeed, the prevalence of smokers was significantly lower (74.6% vs 53.2%) and that of EGFR mutations was higher (9.4% vs 23.4%) in patients tested by liquid biopsy. Genes like CDKN2A/B and MTAP may have an artificially low prevalence in this type of study, due to copy number deletions not being detected by liquid assays. Similarly, no alterations in NKX2-1, ERBB2, and NFKBIA were found using ctDNA sequencing, possibly due to lower sensitivity of liquid biopsy to detect ERBB2 copy number alterations (23) and the fact that NKX2-1 and NFKBIA are not baited on F1L test. Part of the differences in detection rates observed with liquid, as opposed to tissue-based tests, may also be attributable to the size of the panel used; indeed, even though the sample size in the F1LCDx was low, we observed a higher proportion of actionable genes detected by this test compared to the older F1L (40.9% vs. 29.5%), presumably due to the greater number of genes analyzed (324 vs. 70). It seems that the larger the gene panel, the higher the proportion of actionable genes detected (24, 25). The increase in the percentage of actionable genes ranged from 31% (24) to 45% (25). In addition, F1LCDx was shown to have a higher success rate than F1L (26).
In addition to IHC-based PD-L1 expression, high TMB, generally defined as TMB ≥ 10 mutations/Mb, may predict response to immunotherapy (7, 27), although this is not yet a recognized regulatory biomarker in Europe (7). In our cohort, 28% of 145 TMB-assessable patients had high TMB (30.1% in tissue biopsies); such frequency is slightly lower than that reported in the literature for NSCLC (36%), possibly due to differences between tests. Indeed, greater TMB was detected by F1CDx than by F1LCDx; such difference may be explained by a relatively lower prevalence of smokers in the liquid cohort (a greater frequency of high TMB is observed among ever-smokers in our cohort, consistent with other reports (28)) and/or by the possibility that a low tumor fraction may lead to TMB underestimation in blood samples (29). MSI status is another predictive biomarker for immunotherapy (30). In our study, a greater proportion of patients (86%) had undetermined MSI status in liquid specimens when compared to tissue ones (12%). This is due to the design of F1L assay, which reports only MSI-H status. Genomic space covered and panel composition may result in a different efficiency in detecting MSI. However, in NSCLC MSI is rare (1%) and only a few studies have evaluated its impact on response to immunotherapy (31).
Another potential advantage of NGS is the ability to detect co-occurrences that may affect the response to anticancer therapies in NSCLC (32). TP53 is one of the most important tumor suppressors (33), frequently mutated in almost all types of cancers, including NSCLC (34). Thus, it is not surprising that concomitant alterations of TP53 and actionable genes may affect responsiveness to TKI and immunotherapy. In the IMMINENT cohort, TP53 was the most prevalent co-alteration in all tumors, particularly in EGFR- and KRAS-mutated tumors. Co-occurrence of TP53 and EGFR mutations is generally associated with worse prognosis in patients treated with TKIs (35, 36). In the IMMINENT cohort, co-occurrences were also significant with RB1; patients with EGFR/RB1/TP53 co-mutant lung cancer are at risk of small or large cell neuroendocrine lung cancer transformation (37, 38). Although usually mutually exclusive, co-occurrence of EGFR and KRAS alterations (found in three patients in the IMMINENT cohort) can be found with highly sensitive methods and usually portends primary and/or acquired resistance to EGFR TKIs (39). In the IMMINENT dataset, STK11 was the second most frequently co-mutated gene, among KRAS-mutant patients, although this association did not reach statistical significance. STK11/KEAP1 and KRAS/KEAP1 co-mutations have been reported by other studies (40), with variable association with clinical outcome (41, 42). To our knowledge, this is the first study reporting co-occurrences of MYC/STK11 and MYC/ERBB2 and were preliminarily confirmed as statistically significant in the TCGA pan-lung cancer cohort (43). Overall, the concept that co-mutations are associated with worse outcome is supported by an exploratory univariate analysis showing, in the IMMINENT cohort, a significantly better survival for patients whose tumors harbored no or a single genomic driver, as compared to those characterized by a more complex genomic landscape. These findings, however, need further analysis to exclude potential confounders: smoking history may play a role in the association between the number of altered genes and worse OS, as smokers display both a higher number of mutations and worse prognosis. Moreover, the association between a higher number of altered genes and worse hOS and mOS was not observed when the analysis was restricted to tissue specimens; in blood samples, the absence of mutations may indicate minimal or no shedding of tumor DNA, which may have positive prognostic value (44).
Survival outcomes for the IMMINENT population compare favorably with those reported in a recent Italian real-world experience (45). Better figures in the IMMINENT cohort, as compared to registry data, probably reflect a relative selection of patients, as well as the inclusion of patients who had been treated with first-line chemo-immunotherapy combinations (reimbursed in Italy since 2019). The clear impact of well-known prognostic factors (such as stage at diagnosis for hOS, ECOG PS, and smoking status for both h and mOS) attests to the representativeness of the population studied and to the generalizability of the results.
Current clinical guidelines advocate for the molecular genotyping of patients newly diagnosed with metastatic NSCLC. Real-world evidence underscores the significant survival benefits associated with comprehensive molecular profiling performed either at the time of diagnosis or before initiating first-line treatment. This approach ensures that patients receive tailored therapies targeting specific genetic alterations, thereby optimizing treatment efficacy and patient outcomes (46, 47). In our study, somewhat contrary to expectations, both h and mOS appeared to be significantly longer for patients tested in second or further lines; such finding may be explained in part by the fact that the probability of surviving for a defined further period of time is higher for patients who have already survived up to a certain landmark (conditional survival (48)); indeed, in our series conditional 3-year survival probability did not differ for patients tested with NGS at different times in their disease course, provided that they had already survived 1 year.
Additionally, single gene testing was conducted for the majority of the analyzed patients, allowing for targeted therapy initiation at diagnosis in many cases. However, previous single gene testing may have, in some instances, compromised the amount of available material for comprehensive genomic profiling (CGP) analysis (49).
This study has some limitations, which include its retrospective nature and a relatively small sample size. Another significant limitation is the unavailability of tumor fraction (TF) data in the liquid biopsy cohort. Specifically, for patients who underwent the F1L test, the TF data is not specified in the report. On the other hand, for patients who were subjected to the F1LCdx test, TF data was available for only 13 patients, out of which only 6 patients had high TF levels. This lack of comprehensive TF data is relevant given the clinical implications of TF levels. Patients with who have negative liquid biopsy results and a ctDNA TF of 1% or higher are unlikely to have a driver mutation detected on subsequent tissue testing. Therefore, these patients might benefit from starting treatment immediately. On the other hand, those with a negative liquid biopsy and a ctDNA TF of less than 1% often have a driver mutation identified in follow-up tissue testing and should be prioritized for additional analysis (50). Our limited availability of TF data constrains the ability to draw comprehensive conclusions about the correlation between TF levels and clinical outcomes in the study population. Of note, high-risk clonal hematopoiesis (CH) is often unexpectedly detected in solid tumor patients undergoing plasma cell-free DNA sequencing. These findings could lead to further hematologic diagnostic tests and uncover an occult hematologic malignancy (51). These insights pose a limitation to our study as we did not evaluate monoclonal components due to data insufficiency and the primary objectives of the study.
In that respect, other data sources such as the ongoing ATLAS registry [https://biomarkersatlas.com (52)], prospectively collecting data on the molecular characterization of NSCLC in the majority of Italian Centers, may overcome many of such limitations. Moreover, currently ongoing prospective trials, such as the Liquid-First trial (NCT05846594), will contribute to define the place of ctDNA-based NGS tests in the upfront molecular characterization of newly diagnosed, advanced NSCLC.
5 Conclusions
In conclusion, the IMMINENT study, analyzing data coming from both liquid and tissue NGS tests performed on 246 patients affected by NSCLC in the period May 2019–November 2022, confirms the utility of CGP in the upfront molecular characterization of advanced NSCLC. Further analysis will help gaining insights into prognostic and predictive value of specific genomic alterations or combinations thereof.
Data availability statement
The datasets presented in this study can be found in online repositories. The names of the repository/repositories and accession number(s) can be found in the article/Supplementary Material.
Ethics statement
The study was conducted in accordance with the Declaration of Helsinki, and approved by the Institutional Ethics Committee of clinical research of AOUI Verona (protocol code 59115 of 11/10/2021). The studies were conducted in accordance with the local legislation and institutional requirements. The participants provided their written informed consent to participate in this study.
Author contributions
MaS: Conceptualization, Data curation, Investigation, Methodology, Resources, Validation, Writing – original draft, Writing – review & editing. LB: Conceptualization, Data curation, Investigation, Methodology, Resources, Validation, Writing – original draft, Writing – review & editing. RN: Conceptualization, Data curation, Investigation, Methodology, Resources, Validation, Writing – original draft, Writing – review & editing. JI: Data curation, Writing – review & editing. IS: Data curation, Writing – review & editing. JM: Data curation, Writing – review & editing. MiS: Data curation, Writing – review & editing. AL: Data curation, Writing – review & editing. FB: Data curation, Writing – review & editing. FV: Data curation, Writing – review & editing. FS: Data curation, Writing – review & editing. GA: Data curation, Writing – review & editing. LC: Data curation, Writing – review & editing. MO: Data curation, Writing – review & editing. DM: Data curation, Writing – review & editing. AV: Data curation, Writing – review & editing. FL: Data curation, Writing – review & editing. HP: Data curation, Writing – review & editing. FF: Data curation, Writing – review & editing. CS: Data curation, Writing – review & editing. CP: Data curation, Formal analysis, Methodology, Writing – original draft, Writing – review & editing. LP: Data curation, Formal analysis, Methodology, Writing – original draft, Writing – review & editing. ES: Conceptualization, Data curation, Methodology, Writing – review & editing. SC: Conceptualization, Data curation, Methodology, Writing – review & editing. UM: Supervision, Validation, Visualization, Writing – review & editing. SN: Supervision, Validation, Visualization, Writing – review & editing. EB: Supervision, Validation, Visualization, Writing – review & editing. SP: Conceptualization, Data curation, Methodology, Supervision, Validation, Visualization, Writing – original draft, Writing – review & editing. MM: Conceptualization, Data curation, Supervision, Validation, Visualization, Writing – original draft, Writing – review & editing.
Funding
The author(s) declare financial support was received for the research, authorship, and/or publication of this article. This project was sponsored and financially supported by Roche S.p.A. The funder was not involved in the study design, collection, analysis, interpretation of data, the writing of this article, or the decision to submit it for publication.
Acknowledgments
We acknowledge the participating centers and the relevant healthcare personnel involved in this research: Policlinico Universitario A.Gemelli (Roma), Azienda Ospedaliera San Giovanni Addolorata (Roma), Policlinico Umberto I (Roma); Azienda Ospedaliera Ospedali Riuniti Villa Sofia Cervello (Palermo); Ospedale San Vincenzo Taormina (Taormina); Azienda Ospedaliero Universitaria Policlinico “G.Rodolico - San Marco” (Catania); Azienda Ospedaliera Universitaria Integrata Verona (Verona); Ospedale P. Pederzoli (Peschiera); Ospedale San Bortolo di Vicenza (Vicenza); Ospedale Santa Chiara di Trento (Trento); Agenzia Ospedale Università (Padova); Azienda Ospedaliero Universitaria San Giovanni di Dio Ruggi d’Aragona (Palermo). LB and MM are supported by Piano Nazionale di Ripresa e Resilienza (PNRR) projects HEAL Italia and INNOVA. MM is supported by Research Projects of National Relevance (PRIN) TELUAD. We acknowledge Ethan Sokol, Smruthy Sivakumar, and Thomas Wieland from FMI for the suggestions and text revision. We also acknowledge AdRes Health Economics & Outcomes Research, that provided statistical analyses, and SEEd Medical Publishers, that provided publishing support and journal styling services, both funded by Roche SpA.
Conflict of interest
MaS declares travel fees from Roche and Sanofi unrelated to the current work. LB declares travel fees from Eli Lilly, Sanofi and Takeda, Advisory Board role from AMGEN, Roche and Takeda, Speaker Honoraria from Amgen and BMS, Research funding from BMS, unrelated to the current work. HS declares advisory board role for BMS, MSD, Roche, Pfizer, Takeda, AstraZeneca, Merck unrelated to the current work. MO declares travel accommodation from Eli Lilly and Advisory Board role/Speaker Honoraria from Astra Zeneca, BMS and MSD, outside the submitted work. ES and SC are employed at Roche. LP is the co-owner and an employee of AdRes, which has received project funding from Roche; outside the submitted work, received payment or honoraria for lectures, presentations, speakers bureaus, manuscript writing or educational event from Fresenius Kabi, Janssen Cilag, SINPE; AdRes received grants or contracts from Janssen Cilag, Roche, Novartis, Sanofi, Astellas, Diasorin, Nestlè, Shionogi, Boehringer Ingelheim, GSK, and others, outside the submitted work. CP is employee of Adres. The funder had no role in the data collection and no access to patient level data. UM has received personal fees as consultant and/or speaker bureau from Boehringer Ingelheim, Roche, MSD, Amgen, Thermo Fisher Scientific, Eli Lilly, Diaceutics, GSK, Merck and AstraZeneca, Janssen, Diatech, Novartis and Hedera unrelated to the current work. SN reports personal fees as speaker bureau or advisor from Eli Lilly, MSD, Roche, BMS, Takeda, Pfizer, Astra Zeneca and Boehringer Ingelheim, unrelated to the current work. EB received speakers’ and travel fees from MSD, Astra-Zeneca, Celgene, Pfizer, Helsinn, Eli-Lilly, BMS, Novartis, and Roche, institutional research grants from Astra-Zeneca and Roche. SP received honoraria or speakers’ fees from Astra-Zeneca, Eli-Lilly, BMS, MSD, Takeda, Amgen, Novartis, and Roche, unrelated to the current work.
The remaining authors declare that the research was conducted in the absence of any commercial or financial relationships that could be construed as a potential conflict of interest.
The author(s) declared that they were an editorial board member of Frontiers, at the time of submission. This had no impact on the peer review process and the final decision.
Publisher’s note
All claims expressed in this article are solely those of the authors and do not necessarily represent those of their affiliated organizations, or those of the publisher, the editors and the reviewers. Any product that may be evaluated in this article, or claim that may be made by its manufacturer, is not guaranteed or endorsed by the publisher.
Supplementary material
The Supplementary Material for this article can be found online at: https://www.frontiersin.org/articles/10.3389/fonc.2024.1436588/full#supplementary-material
Glossary
cfDNA: circulating free DNA
CGP: comprehensive genomic profiling
CNAs: copy number alterations
CS: conditional survival
ctDNA: circulating tumor DNA
EGFR: epidermal growth factor receptor
ESMO: European Society for Medical Oncology
FFPE: formalin-fixed paraffin-embedded
F1CDx: FoundationOne®CDx
F1L: FoundationOne®Liquid
F1LCDx: FoundationOne®Liquid CDx
FISH: fluorescence in situ hybridization
GCN: gene copy number
hOS: overall survival from histological diagnosis
ICF: informed consent form
IHC: immunohistochemistry
IMMINENT: IMpact of broad genoMic profIling oN advancEd NSCLC outcome
IQR: interquartile range
ISH: in situ hybridization
KM: Kaplan-Meier
LUAD: adenocarcinoma
mOS: overall survival from metastatic diagnosis
MSI: microsatellite instability
MS: microsatellite status
NGS: next generation sequencing
NSCLC: non-small-cell lung cancer
PD-L1: programmed death-ligand 1
RT-PCR: reverse transcriptase-polymerase chain reaction
SCC: squamous cell carcinoma
SCLC: small-cell lung cancer
SNV: single nucleotide variations
TCGA: The Cancer Genome Atlas
TMB: tumor mutational burden
References
1. World Health Organization. Cancer (2022). Available online at: https://www.who.int/news-room/fact-sheets/detail/cancer (Accessed April 19, 2023).
2. AIOM, AIRTUM, Fondazione AIOM, et al. I numeri del cancro in Italia 2022 (2022). Available online at: https://www.aiom.it/wp-content/uploads/2022/12/2022_AIOM_NDC-web.pdf (Accessed April 18, 2023).
3. Siegel RL, Miller KD, Wagle NS, Jemal A. Cancer statistics, 2023. CA: A Cancer J Clin. (2023) 73:17–48. doi: 10.3322/caac.21763
4. Friedlaender A, Banna G, Malapelle U, Pisapia P, Addeo A. Next generation sequencing and genetic alterations in squamous cell lung carcinoma: where are we today? Front Oncol. (2019) 9:166. doi: 10.3389/fonc.2019.00166
5. AIOM. Linee Guida Neoplasie del Polmone (2021). Available online at: https://www.iss.it/documents/20126/8403839/LG%20149_Polmone_agg2021 (Accessed May 9, 2023).
6. Hendriks LE, Kerr KM, Menis J, Mok TS, Nestle U, Passaro A, et al. Oncogene-addicted metastatic non-small-cell lung cancer: ESMO Clinical Practice Guideline for diagnosis, treatment and follow-up. Ann Oncol. (2023) 34(4):339–57. doi: 10.1016/j.annonc.2022.12.009
7. Mosele F, Remon J, Mateo J, Westphalen CB, Barlesi F, Lolkema MP, et al. Recommendations for the use of next-generation sequencing (NGS) for patients with metastatic cancers: a report from the ESMO Precision Medicine Working Group. Ann Oncol. (2020) 31:1491–505. doi: 10.1016/j.annonc.2020.07.014
8. Presley CJ, Tang D, Soulos PR, Chiang AC, Longtine JA, Adelson KB, et al. Association of broad-based genomic sequencing with survival among patients with advanced non-small cell lung cancer in the community oncology setting. JAMA. (2018) 320:469–77. doi: 10.1001/jama.2018.9824
9. Mateo J, Chakravarty D, Dienstmann R, Jezdic S, Gonzalez-Perez A, Lopez-Bigas N, et al. A framework to rank genomic alterations as targets for cancer precision medicine: the ESMO Scale for Clinical Actionability of molecular Targets (ESCAT). Ann Oncol. (2018) 29:1895–902. doi: 10.1093/annonc/mdy263
10. Rolfo C, Mack P, Scagliotti GV, Aggarwal C, Arcila ME, Barlesi F, et al. Liquid biopsy for advanced NSCLC: A consensus statement from the international association for the study of lung cancer. J Thorac Oncol. (2021) 16:1647–62. doi: 10.1016/j.jtho.2021.06.017
11. Foundation Medicine Inc. FoundationOne® CDx. Technical information. (2019). Available at: https://info.foundationmedicine.com/hubfs/FMI%20Labels/FoundationOne_CDx_Label_Technical_Info.pdf.
12. Foundation Medicine Inc. FoundationOne® Liquid CDx. Technical Information. Available at: https://www.foundationmedicine.com/test/foundationone-liquid-cdx.
13. Frampton GM, Fichtenholtz A, Otto GA, Wang K, Downing SR, He J, et al. Development and validation of a clinical cancer genomic profiling test based on massively parallel DNA sequencing. Nat Biotechnol. (2013) 31:1023–31. doi: 10.1038/nbt.2696
14. Milbury CA, Creeden J, Yip WK, Smith DL, Pattani V, Maxwell K, et al. Clinical and analytical validation of FoundationOne®CDx, a comprehensive genomic profiling assay for solid tumors. PloS One. (2022) 17:e0264138. doi: 10.1371/journal.pone.0264138
15. Chalmers ZR, Connelly CF, Fabrizio D, Gay L, Ali SM, Ennis R, et al. Analysis of 100,000 human cancer genomes reveals the landscape of tumor mutational burden. Genome Med. (2017) 9:34. doi: 10.1186/s13073-017-0424-2
16. Clark TA, Chung JH, Kennedy M, Hughes JD, Chennagiri N, Lieber DS, et al. Analytical validation of a hybrid capture-based next-generation sequencing clinical assay for genomic profiling of cell-free circulating tumor DNA. J Mol Diagn. (2018) 20:686–702. doi: 10.1016/j.jmoldx.2018.05.004
17. Woodhouse R, Li M, Hughes J, Delfosse D, Skoletsky J, Ma P, et al. Clinical and analytical validation of FoundationOne Liquid CDx, a novel 324-Gene cfDNA-based comprehensive genomic profiling assay for cancers of solid tumor origin. PloS One. (2020) 15:e0237802. doi: 10.1371/journal.pone.0237802
18. Normanno N, De Luca A, Abate RE, Morabito A, Milella M, Tabbò F, et al. Current practice of genomic profiling of patients with advanced solid tumours in Italy: the Italian Register of Actionable Mutations (RATIONAL) study. Eur J Cancer. (2023) 187:174–84. doi: 10.1016/j.ejca.2023.03.027
19. Zhang C, Kim RY, McGrath CM, Andronov M, Haas AR, Ma KC, et al. The performance of an extended next generation sequencing panel using endobronchial ultrasound-guided fine needle aspiration samples in non-squamous non-small cell lung cancer: A pragmatic study. Clin Lung Cancer. (2023) 24:e105–12. doi: 10.1016/j.cllc.2022.11.010
20. Arcila ME, Yang SR, Momeni A, Mata DA, Salazar P, Chan R, et al. Ultrarapid EGFR mutation screening followed by comprehensive next-generation sequencing: A feasible, informative approach for lung carcinoma cytology specimens with a high success rate. JTO Clin Res Rep. (2020) 1:100077. doi: 10.1016/j.jtocrr.2020.100077
21. Drilon A, Clark JW, Weiss J, Ou SI, Camidge DR, Solomon BJ, et al. Antitumor activity of crizotinib in lung cancers harboring a MET exon 14 alteration. Nat Med. (2020) 26:47–51. doi: 10.1038/s41591-019-0716-8
22. Camidge DR, Otterson GA, Clark JW, Ignatius Ou SH, Weiss J, Ades S, et al. Crizotinib in patients with MET-amplified NSCLC. J Thorac Oncol. (2021) 16:1017–29. doi: 10.1016/j.jtho.2021.02.010
23. Hofman P. Next-generation sequencing with liquid biopsies from treatment-naïve non-small cell lung carcinoma patients. Cancers. (2021) 13:2049. doi: 10.3390/cancers13092049
24. Mehrad M, Roy S, Bittar HT, Dacic S. Next-generation sequencing approach to non-small cell lung carcinoma yields more actionable alterations. Arch Pathol Lab Med. (2018) 142:353–7. doi: 10.5858/arpa.2017-0046-OA
25. Özdemir B, Charrier M, Gerard CL, Wicky A, Caikovski M, Cuendet M, et al. 7P Comparison of the clinical utility of two different size next generation sequencing (NGS) gene panels for solid tumours. Ann Oncol. (2020) 31:S1219. doi: 10.1016/j.annonc.2020.08.2166
26. Caputo V, De Falco V, Ventriglia A, Famiglietti V, Martinelli E, Morgillo F, et al. Comprehensive genome profiling by next generation sequencing of circulating tumor DNA in solid tumors: a single academic institution experience. Ther Adv Med Oncol. (2022) 14:17588359221096878. doi: 10.1177/17588359221096878
27. Rizvi H, Sanchez-Vega F, La K, Chatila W, Jonsson P, Halpenny D, et al. Molecular determinants of response to anti-programmed cell death (PD)-1 and anti-programmed death-ligand 1 (PD-L1) blockade in patients with non-small-cell lung cancer profiled with targeted next-generation sequencing. J Clin Oncol. (2018) 36:633–41. doi: 10.1200/JCO.2017.75.3384
28. Wang X, Ricciuti B, Nguyen T, Li X, Rabin MS, Awad MM, et al. Association between smoking history and tumor mutation burden in advanced non-small cell lung cancer. Cancer Res. (2021) 81:2566–73. doi: 10.1158/0008-5472.CAN-20-3991
29. Husain H, Pavlick DC, Fendler BJ, Madison RW, Decker B, Gjoerup O, et al. Tumor fraction correlates with detection of actionable variants across > 23,000 circulating tumor DNA samples. JCO Precis Oncol. (2022) 6:e2200261. doi: 10.1200/PO.22.00261
30. Luchini C, Bibeau F, Ligtenberg MJL, Singh N, Nottegar A, Bosse T, et al. ESMO recommendations on microsatellite instability testing for immunotherapy in cancer, and its relationship with PD-1/PD-L1 expression and tumour mutational burden: a systematic review-based approach. Ann Oncol. (2019) 30:1232–43. doi: 10.1093/annonc/mdz116
31. Li N, Wan Z, Lu D, Chen R, Ye X. Long-term benefit of immunotherapy in a patient with squamous lung cancer exhibiting mismatch repair deficient/high microsatellite instability/high tumor mutational burden: A case report and literature review. Front Immunol. (2022) 13:1088683. doi: 10.3389/fimmu.2022.1088683
32. Skoulidis F, Heymach JV. Co-occurring genomic alterations in non-small-cell lung cancer biology and therapy. Nat Rev Cancer. (2019) 19:495–509. doi: 10.1038/s41568-019-0179-8
33. Halvorsen AR, Silwal-Pandit L, Meza-Zepeda LA, Vodak D, Vu P, Sagerup C, et al. TP53 mutation spectrum in smokers and never smoking lung cancer patients. Front Genet. (2016) 7:85. doi: 10.3389/fgene.2016.00085
34. Toyooka S, Tsuda T, Gazdar AF. The TP53 gene, tobacco exposure, and lung cancer. Hum Mutat. (2003) 21:229–39. doi: 10.1002/(ISSN)1098-1004
35. Ferrara MG, Belluomini L, Smimmo A, Sposito M, Avancini A, Giannarelli D, et al. Meta-analysis of the prognostic impact of TP53 co-mutations in EGFR-mutant advanced non-small-cell lung cancer treated with tyrosine kinase inhibitors. Crit Rev Oncol Hematol. (2023) 184:103929. doi: 10.1016/j.critrevonc.2023.103929
36. Bria E, Pilotto S, Amato E, Fassan M, Novello S, Peretti U, et al. Molecular heterogeneity assessment by next-generation sequencing and response to gefitinib of EGFR mutant advanced lung adenocarcinoma. Oncotarget. (2015) 6:12783–95. doi: 10.18632/oncotarget.v6i14
37. Offin M, Chan JM, Tenet M, Rizvi HA, Shen R, Riely GJ, et al. Concurrent RB1 and TP53 Alterations Define a Subset of EGFR-Mutant Lung Cancers at risk for Histologic Transformation and Inferior Clinical Outcomes. J Thorac Oncol. (2019) 14:1784–93. doi: 10.1016/j.jtho.2019.06.002
38. Belluomini L, Caliò A, Giovannetti R, Motton M, Mazzarotto R, Micheletto C, et al. Molecular predictors of EGFR-mutant NSCLC transformation into LCNEC after frontline osimertinib: digging under the surface. ESMO Open. (2021) 6:100028. doi: 10.1016/j.esmoop.2020.100028
39. Del Re M, Rofi E, Restante G, Crucitta S, Arrigoni E, Fogli S, et al. Implications of KRAS mutations in acquired resistance to treatment in NSCLC. Oncotarget. (2018) 9:6630–43. doi: 10.18632/oncotarget.v9i5
40. Kaufman JM, Amann JM, Park K, Arasada RR, Li H, Shyr Y, et al. LKB1 Loss induces characteristic patterns of gene expression in human tumors associated with NRF2 activation and attenuation of PI3K-AKT. J Thorac Oncol. (2014) 9:794–804. doi: 10.1097/JTO.0000000000000173
41. Ricciuti B, Arbour KC, Lin JJ, Vajdi A, Vokes N, Hong L, et al. Diminished efficacy of programmed death-(Ligand)1 inhibition in STK11- and KEAP1-mutant lung adenocarcinoma is affected by KRAS mutation status. J Thorac Oncol. (2022) 17:399–410. doi: 10.1016/j.jtho.2021.10.013
42. Jänne PA, Riely GJ, Gadgeel SM, Heist RS, Ou SI, Pacheco JM, et al. Adagrasib in non–small-cell lung cancer harboring a KRASG12C mutation. N Engl J Med. (2022) 387:120–31. doi: 10.1056/NEJMoa2204619
43. cBioPortal for Cancer Genomics. Available online at: https://www.cbioportal.org/ (Accessed November 8, 2023).
44. Pécuchet N, Zonta E, Didelot A, Combe P, Thibault C, Gibault L, et al. Base-position error rate analysis of next-generation sequencing applied to circulating tumor DNA in non-small cell lung cancer: A prospective study. PloS Med. (2016) 13:e1002199. doi: 10.1371/journal.pmed.1002199
45. Franchi M, Cortinovis D, Corrao G. Treatment patterns, clinical outcomes and healthcare costs of advanced non-small cell lung cancer: A real-world evaluation in Italy. Cancers (Basel). (2021) 13:3809. doi: 10.3390/cancers13153809
46. Aggarwal C, Marmarelis ME, Hwang WT, Scholes DG, McWilliams TL, Singh AP, et al. Association between availability of molecular genotyping results and overall survival in patients with advanced nonsquamous non-small-cell lung cancer. JCO Precis Oncol. (2023) 7:e2300191. doi: 10.1200/PO.23.00191
47. Yorio J, Lofgren KT, Lee JK, Tolba K, Oxnard GR, Schrock AB, et al. Association of timely comprehensive genomic profiling with precision oncology treatment use and patient outcomes in advanced non-small-cell lung cancer. JCO Precis Oncol. (2024) 8:e2300292. doi: 10.1200/PO.23.00292
48. Basse C, Carton M, Milder M, Beaucaire Danel S, Daniel C, Du Rusquec P, et al. 54P Overall survival in patients with metastatic lung cancer from 2000 to 2020: Implementation of innovative strategies in a real-world setting. Ann Oncol. (2022) 33:S57. doi: 10.1016/j.annonc.2022.02.063
49. Nesline MK, Subbiah V, Previs RA, Strickland KC, Ko H, DePietro P, et al. The impact of prior single-gene testing on comprehensive genomic profiling results for patients with non-small cell lung cancer. Oncol Ther. (2024) 12(2):329–43. doi: 10.1007/s40487-024-00270-x
50. Rolfo CD, Madison RW, Pasquina LW, Brown DW, Huang Y, Hughes JD, et al. Measurement of ctDNA tumor fraction identifies informative negative liquid biopsy results and informs value of tissue confirmation. Clin Cancer Res. (2024) 30:2452–60. doi: 10.1158/1078-0432.CCR-23-3321
51. Aldea M, Tagliamento M, Bayle A, Vasseur D, Vergé V, Marinello A, et al. Liquid biopsies for circulating tumor DNA detection may reveal occult hematologic Malignancies in patients with solid tumors. JCO Precis Oncol. (2023) 7:e2200583. doi: 10.1200/PO.22.00583
Keywords: next generation sequencing, non-small cell lung cancer, precision medicine, liquid biopsy, target therapy
Citation: Sposito M, Belluomini L, Nocini R, Insolda J, Scaglione IM, Menis J, Simbolo M, Lugini A, Buzzacchino F, Verderame F, Spinnato F, Aprile G, Calvetti L, Occhipinti M, Marinelli D, Veccia A, Lombardo F, Soto Parra HJ, Ferraù F, Savastano C, Porta C, Pradelli L, Sicari E, Castellani S, Malapelle U, Novello S, Bria E, Pilotto S and Milella M (2024) Tissue- and liquid-biopsy based NGS profiling in advanced non-small-cell lung cancer in a real-world setting: the IMMINENT study. Front. Oncol. 14:1436588. doi: 10.3389/fonc.2024.1436588
Received: 22 May 2024; Accepted: 24 June 2024;
Published: 09 July 2024.
Edited by:
Lizza E. L. Hendriks, Maastricht University Medical Centre, NetherlandsCopyright © 2024 Sposito, Belluomini, Nocini, Insolda, Scaglione, Menis, Simbolo, Lugini, Buzzacchino, Verderame, Spinnato, Aprile, Calvetti, Occhipinti, Marinelli, Veccia, Lombardo, Soto Parra, Ferraù, Savastano, Porta, Pradelli, Sicari, Castellani, Malapelle, Novello, Bria, Pilotto and Milella. This is an open-access article distributed under the terms of the Creative Commons Attribution License (CC BY). The use, distribution or reproduction in other forums is permitted, provided the original author(s) and the copyright owner(s) are credited and that the original publication in this journal is cited, in accordance with accepted academic practice. No use, distribution or reproduction is permitted which does not comply with these terms.
*Correspondence: Sara Pilotto, c2FyYS5waWxvdHRvQHVuaXZyLml0; Michele Milella, bWljaGVsZS5taWxlbGxhQHVuaXZyLml0
†These authors share first authorship
‡These authors share last authorship