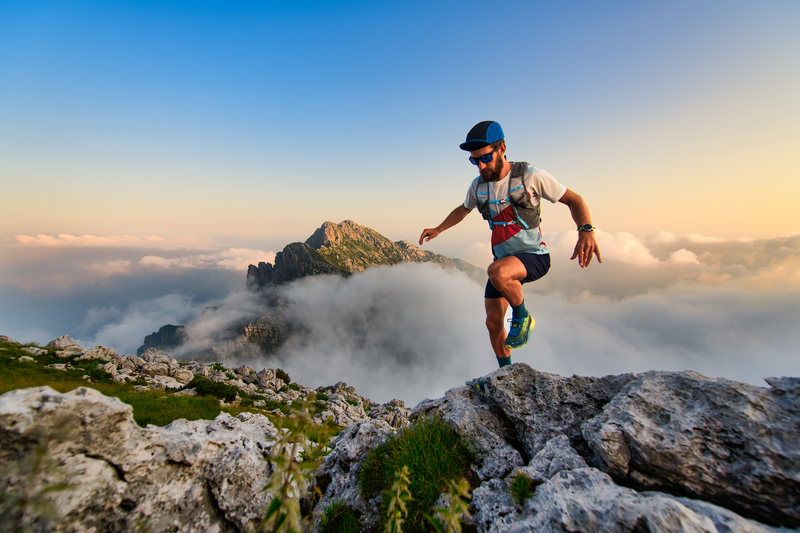
94% of researchers rate our articles as excellent or good
Learn more about the work of our research integrity team to safeguard the quality of each article we publish.
Find out more
SYSTEMATIC REVIEW article
Front. Oncol. , 31 July 2024
Sec. Thoracic Oncology
Volume 14 - 2024 | https://doi.org/10.3389/fonc.2024.1428018
This article is part of the Research Topic Crosstalk between Lung Cancer and Fibrosis: Pathogenesis and Therapeutic Strategies View all articles
Background: Lung cancer (LC) is one of the most common malignant tumors in the world and the leading cause of cancer-related deaths, which seriously threatens human life and health as well as brings a heavy burden to the society. In recent years, the tumor microenvironment (TME) has become an emerging research field and hotspot affecting tumor pathogenesis and therapeutic approaches. However, to date, there has been no bibliometric analysis of lung cancer and the tumor microenvironment from 2014 to 2023.This study aims to comprehensively summarize the current situation and development trends in the field from a bibliometric perspective.
Methods: The publications about lung cancer and the tumor microenvironment from 2014 to 2023 were extracted from the Web of Science Core Collection (WoSCC). The Microsoft Excel, Origin, R-bibliometrix, CiteSpace, and VOSviewer software are comprehensively used to scientifically analyze the data.
Results: Totally, 763 publications were identified in this study. A rapid increase in the number of publications was observed after 2018. More than 400 organizations published these publications in 36 countries or regions. China and the United States have significant influence in this field. Zhou, CC and Frontiers in Immunology are the most productive authors and journals respectively. Besides, the most frequently cited references were those on lung cancer pathogenesis, clinical trials, and treatment modalities. It suggests that novel lung cancer treatment models mainly based on the TME components, such as cancer-associated fibroblasts (CAFs) may lead to future research trends.
Conclusions: The field of lung cancer and the tumor microenvironment research is still in the beginning stages. Gene expression, molecular pathways, therapeutic modalities, and novel detection technologies in this field have been widely studied by researchers. This is the first bibliometric study to comprehensively summarize the research trend and development regarding lung cancer and tumor microenvironment over the last decade. The result of our research provides the updated perspective for scholars to understand the key information and cutting-edge hotspots in this field, as well as to identify future research directions.
Lung cancer (LC) is a global health concern and one of the leading causes of cancer-related mortality. According to the global cancer statistics report published by the International Agency for Research on Cancer (IARC), incidence and mortality rates of lung cancer remain high, accounting for 18% of global cancer deaths in 2020 (1–3).
Surgery, radiotherapy, and chemotherapy have been the standard of care for lung cancer treatment in recent years. However, the clinical use of targeted therapies and immunotherapy has been increasing. The focus has shifted to detecting driver genes associated with tumor development, such as EGFR, KRAS, and MET, and identifying the signaling pathways of cell growth or apoptosis regulated by these genes. Targeting treatment to these genes has significantly improved the intermediate survival of lung cancer patients. Immunotherapy is now the standard first-line treatment for patients with advanced or metastatic mutation-negative driver genes in NSCLC. Unfortunately, tumor recurrence often leads to resistance to the initially effective drug (4).
With the emerging heated concept of tumor microenvironment (TME), increasing evidence suggests that TME promotes cancer progression and may mediate therapeutic resistance. Lung cancer-related therapies and studies are gradually expanding from focusing solely on the tumor cells themselves to the broader field of tumor microenvironment research. The development of cancer is strongly correlated with the physiological status of the tumor microenvironment, which can regulate tumor cells multiplication and bolster resistance to therapy. The TME is a hierarchically structured ecosystem that contains a variety of cell types ranging from tumor-associated macrophages (TAMs), immune cells, and cancer-associated fibroblasts (CAFs), as well as blood vessels, nerve fibers, extracellular matrix, and related noncellular components (5–7). In particular, immune cells play important roles in TME, which includes the promotion of tumor growth, and play a key role in host immune surveillance and elimination of neoplastic cancer cells (8). The cellular composition and functional status of the TME change depending on the tumor category, intrinsic characteristics of the cancer cells, tumor stage, and the characteristics of the individual patient. The effects of these cells can be mutual concerning the tumor and play a key role in host immunosurveillance and elimination of neoplastic cancer cells (9). Collectively, their interactions regulate regional immune effects and ultimately influence lung cancer outcomes, thus the cells in the TME and their secreted molecules are now considered to be critical in the pathogenesis of cancer for which they serve as potential targets for novel therapeutic cancer interventions.
TAM is also believed to be a key factor driving the TME to promote lung cancer development. It can be directly involved in tumor invasion, migration, epithelial-to-mesenchymal transition (EMT), and angiogenesis by secreting the chemokine CCL18. Ultimately, this leads to cancer progression and activates the NF-κB pathway in CAFs, inducing stemness and drug resistance in tumor cells (10, 11). The study by Xiang indicated that the increase in Tregs and dendritic cells (DCs) in the may also to acquired resistance after targeted therapy and immunotherapy (12). Based on scRNA-seq analysis and in vitro experiments, Aiko showed that high levels of IL-1β in TME may cooperate with IFN-γ to induce up-regulated expression of PD-L1 in tumor cells through activation of MAPK signaling, which in turn leads to resistance to tumor immunosuppression (13).
Besides, the TME can be subdivided into six specific categories: hypoxic ecological niche, immune microenvironment, metabolic microenvironment, acidic ecological niche, innervation ecological niche, and mechanical microenvironment (6). There is also bi-directional communication between microenvironments, so that targeting one specific microenvironment may result in a series of changes in other specific microenvironments and relevant pathways. As more and more studies have demonstrated the involvement of TME components in immune evasion and drug resistance against tumor cells (14–16). TAM is dynamic and subject to change due to pathogenic factors. Cigarette smoking is the most significant risk factor for lung cancer incidence and mortality (17, 18). Using a mouse cellular model, Bianchi discovered that exhaled tobacco smoke could induce the polarization of M2 phenotypic macrophages through various mechanisms. This ultimately hinders the anti-inflammatory effects of TAM in the TME of smokers with lung cancer, leading to the development of an immunosuppressive microenvironment (1). The study of lung cancer is shifting from a cancer-centered paradigm to one that considers the tumor microenvironment (TME) as a whole. It will be meaningful to monitor the dynamic pattern of development between changes in the TME and lung cancer.
Bibliometrics is an emerging method of literature analysis, which is a cross-discipline integrating mathematics, statistics, and bibliography. It is now widely applied by researchers, institutions, and countries in multiple disciplines and fields to build up all knowledge carriers into visual knowledge networks from quantitative and qualitative perspectives. Then through analyzing the big data intelligently the trend and current status of a particular research area can be derived, which will help to guide the policy decisions (19). Additionally, with bibliometric analysis, researchers can quickly, accurately, and comprehensively obtain detailed information, including the intellectual network, research topic evolution, potential development prospects, authors, collaborations, keywords, journals, countries, research institutes, references, and other details of the relevant research areas. Eventually, tools like CiteSpace, VoSviewer, R package, etc. can be used to visualize the results. Comparing it with the traditional literature review, the analysis based on bibliometrics provides a more comprehensive perspective of research trends with more objective data (20).
At present, there is no published bibliometric analysis available that covers lung cancer related to TME. Therefore, the research status as well as the research frontiers and hotspots in this field are still unclear. To provide a reference for further research and application, this paper recognizes and collects relevant literature data from databases. Literature analysis software has been used to study the annual number of publications, countries, publishing organizations, journals, authors, keywords, and references for the last 10 years at the intersection of TME and Lung Cancer, which will describe the progress, hotspots, and emerging trends of research in the field.
The data source we used was the Web of Science Core Collection (http://wcs.webofknowledge.com), which includes the Science Citation Index Expanded (SCIE) and the Social Science Citation Index (SSCI). A comprehensive database search was completed by both authors on January 7, 2024. The specific search formula was as follows: (TI = (“tumor microenvironment”) OR AK = (“tumor microenvironment”) OR TI = (“TME”) OR AK = (“TME”)) AND (TS = (“Pulmonary Neoplasm*”) OR TS = (“Lung Cancer*”) OR TS = (“Pulmonary Cancer*”)). After the preliminary search, two authors (XTY and HZL) independently reviewed and screened the searched publications based on the following inclusion criteria (1): the publication timespan was set from 1 January 2014 to 31 December 2023 (2); only English-language publications were included (3); the publication type was limited to articles or reviews; and (4) the publication was related to a study of both lung cancer and the tumor microenvironment. In order to ensure the representativeness of the selected publications, the search results underwent a title and abstract-based filtration process, which excluded irrelevant publications. The screening criteria were shown in Figure 1. A total of 763 publications were included in the final analysis, which were eventually exported in “plain text” format with “full records and references”.
Three scientometric software tools and two statistical mapping software were used in this study. Citespace (version 6.1.6, https://citespace.podia.com) is a Java-based information visualization and analysis software developed by Professor Chen, who specialized in Computer Science and Intelligence at Drexel University (21). After transforming the data using the software, we defined the analysis period as 2014–2023, the time interval as 1 year, the g-index as k = 25, and the node types as “author,” “institution,” and “keyword” for the co-occurrence network analysis. Each node represents a type of project. Plus, the size and color of the node circles indicate the number or frequency of publications and the year in which these projects appeared. The links between nodes reflect the collaboration between different projects. In addition, VOSviewer (version 1.6.19, https://www.vosviewer.com) (22) is a software tool designed by van Eck and Waltman, mainly used to construct and visualize metering networks. Visualization was achieved by analyzing the co-cited authors and co-cited publications through data importation. The bibliometrix package (version 4.2.2, http://www.bibliometrix.org) (23) in R can also play a role in analyzing and visualizing scientific literature. In our study, we primarily utilize a tool to count, analyze, and visualize various aspects such as national geographies, journal trends, top citations, and authors’ publications per year. Meanwhile, the number of publications per year and their respective countries are exported. Finally, the trends are predicted and visualized using Microsoft Excel 2019 and Origin 2021 software.
The number of annual publications is an indicator of the developmental trend of scientific knowledge in a specific field. A total of 763 publications were selected to meet the inclusion criteria. This selection process is illustrated in Figure 1. The publications consisted of 174 reviews (22.8%) and 589 articles (77.2%), of which 4 non-English publications were excluded, and 143 publications were excluded due to inconsistent publications type. According to the analysis of publication numbers, the annual trend can be divided into two phases. From 2014 to 2018, the number of publications fluctuated between 9 and 32. However, the number of publications has shown an upward trend since 2019. It is shown that the number in 2022 surged to its highest level (186, 24.38%). The average annual number of publications was 76.3. Besides, the annual growth rate was 17.7%. A growth trend model was constructed using Microsoft Excel 2019 with the following equation: Y = 2.6326x2-7.4977x +16.183 (R² = 0.9368), where X represents the cumulative publication year (starting from 2014) and Y represents the annual publication (Figure 2A).
Figure 2 (A) Number of annual research publications and growth trends. (B) The visualization of country. (C) The geographical distribution.
A total of 36 countries/regions have published relevant studies. The details of the top 10 most productive countries, along with their H-indexes in the field of oncology in 2022, are presented in Figure 2B. Upon reviewing the number of articles published by countries in this field in descending order, it is evident that China and the United States were the countries with the highest number of publications. In particular, the Chinese region ranked first, accounting for the greatest number of 402 articles (52.69%), followed by the United States with 101 (13.24%) and Japan with 44 (5.77%). The H-Index is a measure of a scholar’s or country’s level of scientific research. It is often used to assess the corresponding scholar’s or country’s influence and contributions to the academic field (24). By analyzing the H-index in the field of oncology, we found that the United States has the highest overall scientific impact on oncology research (score = 848), followed by the United Kingdom, Germany, and France. Although China is ranked first in the number of publications, its H-index is relatively low (score = 327), indicating a need to enhance both the quality and the academic impact of its research. As shown in the world map in Figure 2C, the overall distribution of publications within the country-region is clearly illustrated. The darker colors indicate a higher number of publications in those regions. It also illustrates the collaboration between countries and regions, such as China and the United States, China and Australia, and the United States and Brazil, etc. The single-country and multinational cooperative publications for the top 10 countries are presented in Supplementary Table 1. It can be observed that China (25) ranked first with the highest number of mutual co-publishing articles with other countries, followed by the United States (26) and Germany (9). However, considering the variation in the total number of publications per country, we utilize statistical analysis to compare them in terms of percentages. Consequently, the rate of cooperative papers published by Australia (70%) was much higher than that of France (54.5%), India (53.8%), and Germany (34.6%). Additionally, Supplementary Table 2 presents the total citation data per country, with China ranking first (5,103), followed by the United States (3,284), and Japan (735). The average number of citations per paper for articles reflects the quality and influence of publications in each country. In sum, Australia ranks first in average citations with 94.8, while the United Kingdom comes in second with 93.
More than 400 institutions engaged in research regarding xxx between 2014 and 2023. The top 10 most prolific institutes are shown in Supplementary Table 3. Meanwhile, we establish an institutional collaboration network by using the CiteSpace software (Figure 3A). Finally, it generates a total of 297 nodes and 477 edges, the numbers of which represent extensive cooperation between established institutions. The top 5 prolific institutions are Tongji University with 24 publications, Nanjing Medical University (21), Peking Union Medical College of the Chinese Academy of Medical Sciences (17), Sichuan University (16), and Sun Yat-sen University (16). What’s more, 20 institutions in total have published more than 10 publicaitons, with the majority of these research institutions located in China.
Figure 3 (A) The visualization of institution. (B) The visualization of author. (C) Cumulative publication trend of the top journals. (D) The dual-map overlay of journals.
Publications related to the tumor microenvironment and lung cancer have been published in 262 journals. The relative contents of the top 10 journals were listed in Supplementary Table 4, including information such as country, academic district, impact factor, H-index, and total citations. Frontiers in Immunology published the highest number of papers (n = 57, 7.47%), followed by Cancers (n = 44, 5.77%), Journal for Immunotherapy of Cancer (n = 37, 4.85%), and Frontiers in Oncology (n = 31, 4.06%). There are 11 journals with more than 10 publications. Impact factor (IF) and academic reputation are important criteria for evaluating research outcomes and academic excellence. The journal with the highest impact factor in 2022 is the Journal of Thoracic Oncology (IF = 20.4), followed by the Journal for Immunotherapy of Cancer (IF = 10.9) and Frontiers in Immunology (IF = 7.3). Although the majority of journals are primarily located in Europe, there are also a few in Asia, the Americas, and Oceania. The H-index of a journal usually refers to the number of journal publications that have been cited at least H times by other publications. It is also an indicator of academic quality and influence. In general, a journal with a higher H-index would obtain greater impact. The journal with the largest H-index and the largest total citations is the Journal for Immunotherapy of Cancer (H-index = 14, total citations = 877), while the journal with the second largest number of publications is Frontiers in Immunology (H-index = 13), Cancers (H-index = 11), and Frontiers in Oncology (H-index = 10). In addition to these journals, the top 3 cited journals also include the Journal of Thoracic Oncology (total citations = 732) and Frontiers in Immunology (total citations = 628). Visualization of the cumulative publications from the top 10 journals is shown in Figure 3C via the bibliometric package in R. A noticeable increasing trend in the publications of many journals can be observed. It is worth noting that Frontiers in Immunology and Cancers have demonstrated a particularly notable increase from 2020 to 2023, with growth exceeding that of other journals during the same period. The dual-mapped overlay of scholarly journals illustrates the relationship between citing and cited journals (Figure 3D). Labels indicate the subject areas of the journals, and colored lines represent different citation paths, with the width of the paths proportional to the z-score levels (27). The two main pathways were (1) molecular, biology, and immunology - molecular, biology, genetics (z = 5.8265, f = 5754) (2); medicine, medical, clinical - molecular, biology, genetics (z = 3.0848, f = 3173).
The top 10 most productive authors with the highest number of publications in this field are presented in Supplementary Table 3. Zhou, Caicun from Tongji University Affiliated Shanghai Pulmonary Hospital, and Savai, Rajkumar from the Department of Lung Development and Remodeling were the two most prolific authors, with 10 and 8 publications, respectively. Moreover, most of them are from China, which suggests that China is still in the leading position in this field. According to the author cooperation interrelationships in this field drawn by citespace (Figure 3B), a total of 382 nodes and 794 edges, indicate that authors with a higher number of papers typically collaborate with regular co-authors and teams.
Co-cited author analysis is a method used to assess the influence and contributions of authors within the academic community based on how frequently they have been cited in scholarly literature. This type of analysis can help researchers gain insights into academic trends, research hotspots, and academic authorities in a particular field.
The network structure of co-cited authors was analyzed using VOSviewer software, as depicted in Figure 4A. The analysis revealed a total of 26,096 co-cited authors, with 51 authors cited more than 40 times. It can be observed in Supplementary Table 5 lists the top 10 cited authors, their affiliations, and the H-index. Martin Reck from the LungenClinic Grosshansdorf had the largest total number of citations (n = 178), followed by Herbst, Roy S. from Yale University (n = 172) and Rebecca L. Siegel from the American Cancer Society (n = 161). Most of these co-cited authors are from Europe or North America. Furthermore, the author with the highest H-index among the top 10 is Alberto Mantovani (187), who has made a significant impact on scholarship in this field. Following Mantovani is Ahmedin Jemal (139) in second place, and then Douglas Hanahan (109).
Figure 4 (A) The co-cited authors analysis. (B) The visualization of keyword. (C) Timeline view for keywords. (D) The three-field plot of lung cancer and the tumor microenvironment.
The keyword co-occurrence analysis involves extracting and analyzing the keywords in the publication. Through the above process, it can help us identify the main topics, core contents, and key points so that the information can be well understood and utilized (26). The timeline view map is typically used to illustrate the evolution of specific research subjects or keywords over time, which enables researchers to observe research hotspots, citation relationships, and trends related to a particular topic or field across distinct timeframes. Furthermore, it facilitates an enhanced understanding of research dynamics and field evolution. The visualization of the relationship between keywords (Figure 4B) is achieved by constructing a visual network using the Citespace software, which consists of 401 nodes and 2766 edges. The size of the node is proportional to the frequency of occurrence of the associated keywords within the field. The larger the node, the greater the frequency of occurrence. Contour color reflects the year of occurrence, with darker colors indicating earlier years, while centrality indicates key nodes within a network. The top 20 keywords and their centrality are shown in Supplementary Table 6. Only the keyword “breast cancer” has a centrality greater than 0.1, while the rest have centrality values below 0.1. This indicates that there are few key nodes in the network. Keywords with higher frequencies include “tumor microenvironment,” “lung cancer,” “expression,” “cancer,” “non-small cell cancer,” and so on. As demonstrated in Figure 4C, the evolution of the tumor microenvironment and lung cancer in this field is delineated through the log-likelihood ratio (LLR) algorithm, the modularity value (Q-value), and the mean silhouette (S-value), which serve as crucial metrics for evaluating the outcomes of graph plotting. The Q-value of the graph, which reached 0.3555 (>0.3), was rationalized into loosely coupled clusters, and the homogeneity within the clusters was credible. The S-value of 0.6853 indicates that the clustering configuration is reasonable and is divided into nine clusters. These clusters are based on the keywords “macrophage,” “EGFR mutation,” “tumor progression,” “drug resistance,” “immune infiltration,” “metabolism,” “lung cancer,” “single-cell RNA sequencing,” and “machine learning.” Additionally, the association between the top 10 countries, institutions, and shared keywords was analyzed using R-bibliometrix (Figure 4D).
A total of 763 publications have been cited 13,663 times, with an average of 17.91 citations per paper. The top 10 most frequently cited publications are presented in Supplementary Table 7 (28–37). The article by Koyama S et al. (32), published in Cancer Research in 2016 and titled “STK11/LKB1 Deficiency Promotes Neutrophil Recruitment and Proinflammatory Cytokine Production to Suppress T-cell Activity in the Lung Tumor Microenvironment,” ranked first in this field with a total of 374 citations. Three reviews were included in the top 10 most cited publications. The most cited article was published by Koyama S et al. (32), which received an average of 41.56 citations per year, followed by Bremnes Rm et al. (29), whose article was cited 37.11 times per year.
In the field, there are 36,868 co-citations available, out of which 72 references had more than 20 co-citations. As demonstrated in Figure 5A, the relational network graph, comprising more than 35 co-citations selected through the VOSviewer software, has a total of 322 edges and a total link strength of 1882. Besides, the top 10 co-cited references are listed in Supplementary Table 8 (25, 38–46). The reference “Global Cancer Statistics” by Ahmedin Jemal in CA: A Cancer Journal for Clinicians had the greatest number of citations (25), while “Hallmarks of Cancer: The Next Generation” (40) and “Cancer Statistics, 2021” (45) follow closely. As can be seen in the table, the top ten co-cited references were mainly published between 2011 and 2021. With two articles published in the New England Journal, two in CA: A Cancer Journal for Clinicians, and the remaining articles published in various journals.
Figure 5 (A)The co-cited references analysis. (B) The top twenty-five references with the strongest citation bursts.
An outbreak of co-citation refers to literature that has been cited together by a wide range of researchers within a specific period. Based on a co-citation literature analysis of 763 documents imported into Citespace, the blue timeline in the figure illustrates the strongest citation bursts. These bursts are defined as the periods between co-cited references by different researchers. The red segments on the timeline represent the time intervals between bursts and indicate the start and end years of the bursts (47). The top 25 references with the strongest citation bursts are displayed in Figure 5B, as identified by the Citespace software. These bursts occurred as early as 2015 and as late as 2023. The most significant citation bursts were published in 2015 by Borghaei et al. (39) in the New England Journal, with a burst intensity of 12 and an outbreak period from 2016 to 2020. Overall, the citation burst intensity of these 25 references ranged from 12 to 3.68. The majority of the strongest citation bursts were published in the New England Journal of Medicine (7).
As far as incomplete statistics are concerned, this paper is the first bibliometric article in this field. The study involves a statistical analysis of the hotspots and trends related to lung cancer and the tumor microenvironment over the past decade using bibliometrics. This analysis utilizes software tools like Citespace, VOSviewer, and the R programming language. As illustrated in Figure 2A, the research in this field demonstrates a persistent upward trend, with a non-significant growth rate observed between 2014 and 2018. However, the number of publications has exhibited a notable acceleration since 2019, reaching levels comparable to those observed in 2022 and 2023. The number of annual publications has surpassed 100 for the first time in 2021, suggesting that this field is gradually attracting widespread attention from researchers, and the prospect of development is promising. The number of annual publications in the field is expected to reach approximately 650 by 2030, as predicted in the fitted model. By analyzing national and regional publications, it is evident that China and the United States are the primary research hubs in this field. This trend is closely linked to the concentration of research technology and top-tier talent in both countries. In addition, another important factor contributing to the annual increase in the number of articles published in China is likely associated with the large population base in China and the continuously rising incidence and mortality rate of lung cancer (48), a topic that receives widespread attention and support for research. The data presented in Supplementary Table 1 indicate a relatively low proportion of collaborative research among countries in this field, with the majority of such research mainly being conducted in Europe and Oceania. Moreover, the average citation ranking of articles suggests that academic research conducted through international collaborations is more likely to attract greater attention and have a higher academic impact. While the East Asia region shows a significant publication volume, the level of inter-country cooperation is comparatively low. Therefore, it is evident that cooperation across countries will contribute to improving resource efficiency and academic development in this field in the future.
Research institutes refer to organizations or units specialized in scientific research, technological development, and innovation. This typically includes universities, research institutes, laboratories, and similar entities. These institutions can reflect the background and sources of research findings, as well as directly affect the quality and credibility of academic research. This study finds that the primary research institutions in this field are situated in China, which is closely associated with the large number of publications in the Chinese region. Meanwhile, Tongji University in China, which ranked first, has been at the forefront of lung cancer research, strongly correlated with the institution’s investment and adequate resources in research. As shown in Supplementary Table 4, most journals published in this field are predominantly located in the Q1 and Q2 quartiles of the Journal Citation Reports (JCR), indicating that they are highly valued by researchers worldwide. Nevertheless, the impact factor of the journals is relatively low, suggesting that scholarship in this area of research still needs improvement.
In terms of authors, Prof. Caicun Zhou from Tongji University in China is the most productive author. He has a long-term involvement in research on the molecular mechanisms and clinical effectiveness of drugs in lung cancer. In particular, Prof. Zhou has made significant contributions in the following areas: mechanisms of lung cancer drugs, immunotherapy for lung cancer, and the establishment of a model for predicting the risk of recurrence of lung cancer (37, 49–51). The following most prolific author is Professor Rajkumar Savai from the Department of Lung Development and Remodeling. He specializes in researching the association between lung cancer and the tumor microenvironment through tumor signaling pathways, macrophages, immunotherapy, etc. (52–55).
The most cited article in this field is a paper published in 2016 by Koyama S et al. regarding the effect of STK11/LKB1 deletion on the immune microenvironment in a KRAS-driven mouse model of NSCLC. The study found that immune escape is mediated by the suppression of myeloid cells and aberrant cytokine production in LKB1-deficient tumors (32). Next is a review of tumor-infiltrating lymphocytes (TILs) and non-small cell lung cancer by Bremnes Rm and colleagues in 2016 (29), as well as a review of the tumor microenvironment and metastatic mechanisms in lung cancer by Wood Sl et al. in 2014 (35).
Keywords and cluster analysis are significant tools for identifying the hotspots and frontiers of research. As a result, we summarize the analysis to provide insight into the interconnection between lung cancer and the tumor microenvironment, suggesting several potential directions for future research.
The first topic to be considered is the relationship between gene expression and signaling pathways in lung cancer in the context of the tumor microenvironment. From the clustering in Figure 4C, it can be concluded that macrophages represent a significant category within the field of study. These cells commonly originate from bone marrow hematopoietic stem cells and subsequently differentiate into circulating monocytes in the peripheral blood. Then they migrate to various tissues and organs within the body, including the skeletal system, lungs, and liver. In these locations, they eventually transform into macrophages with specificity (56, 57). Specifically, macrophages can be divided into two subtypes depending on their activation status, function, and secreted cytokines. One subtype, M1-type macrophages, is activated by LPS, IFN-γ, or TNF and secretes high levels of pro-inflammatory cytokines. These cytokines play a role in the cellular immune response facilitated by type I helper T cells. The other subtype, M2-type macrophages, is polarized by Th2 cytokines. They induce immunosuppression, participate in pro-carcinogenic functions, and promote tumor growth and metastasis (58–60). Liu et al. (61) demonstrated that glucose metabolic pathways are intricately linked with polarization state shifts in tumor-associated macrophages. M1 macrophages upregulate glycolysis and the pentose phosphate pathway to trigger inflammation, while M2 macrophages rely more on the tricarboxylic acid cycle and mitochondrial metabolism to suppress anti-tumor immunity and promote tumor metastasis. In addition, tumor-associated fibroblasts (CAFs) were identified as a potential trend and direction for future research in this field, based on the outbreak of cited literature. Cancer-associated fibroblasts (CAFs) influence the creation of extracellular matrix (ECM) structures and metabolism in the TME. They play a crucial role in regulating tumor immunity and resistance to chemotherapeutic agents (62). Qiao et al. (63) found that in KRAS-mutant lung adenocarcinoma, STK11/LKB1 mutation by constructing a mouse model of lung cancer. Adhesion plaque kinase (FAK) inhibitors inhibited the activation of CAFs and further promoted the infiltration of CD8 T cells, DC cells, and M1-type macrophages into the tumors, thus remodeling the tumor microenvironment. Samart et al. (64) concluded that Musashi-2 (MSI2) has a potential impact on CAFs in regulating the invasive and metastatic spread of NSCLC cells by analyzing genomics and proteomics data. Additionally, a new complex regulatory axis involving MSI2/IL-6 was identified, indicating an interaction between NSCLC-derived CAFs and NSCLC cells through paracrine signaling. Cords et al. (65) found differences in the spatial distribution of distinct CAF phenotypes in TME and identified specific CAF phenotypes that were associated with good versus poor patient prognosis. Tumor metabolism essentially refers to the abnormal metabolic features of tumor cells that contribute to the proliferation and progression of tumors. This involves glucose uptake and utilization, as well as nucleotide synthesis. While metabolism-targeted therapies are widely discussed nowadays (66, 67). In line with the research focus of this field, lung cancer offers a promising path for exploring tumor metabolism and tumor microenvironment in precision medicine. Tumor metabolism has a significant impact on the tumor microenvironment, thus affecting the proliferation of lung cancer cells. Moreover, this research area offers insights that can inform the treatment of lung cancer (68). Liu et al. (69) predicted the prognosis of lung adenocarcinoma (LUAD) and the efficacy of various immunotherapies by constructing a model of glutamine metabolism. Furthermore, a research has demonstrated that deoxypodophyllotoxin (DPT) inhibits glycolysis by preventing the overexpression of HIF-1α, which in turn suppresses cell proliferation in NSCLC (70).
Secondly, we will be examining the links and interactions between lung cancer treatment and the tumor microenvironment. The analysis of keywords and clusters has revealed that scholars have devoted considerable attention to the treatment of lung cancer research, including studies on tumor progression and drug resistance. Firstly, the conventional treatment options include surgical intervention, radiation therapy, and chemotherapy. Surgery is considered the most effective clinical treatment for lung cancer (71). Yet, surgery is often limited to patients with stage I and II operable NSCLC and a preferred local treatment modality is recommended (8, 72). On the other hand, radiotherapy applies to all stages of lung cancer, affecting the functions of the immune system in various ways. With advances in imaging, radiotherapy is now faster and more precise in the treatment of lung cancer (73). Chemotherapy has a high frequency of keyword occurrences in this field, and platinum-based adjuvant chemotherapy is commonly used in patients diagnosed with stage II and stage III NSCLC (74). A combination of two cytotoxic drugs is recommended as first-line treatment for advanced metastatic NSCLC (8). However, the chemotherapy drugs can cause central and peripheral neurotoxicity, cardiotoxicity, gastrointestinal toxicity, and hematologic toxicity in humans (75, 76). From the perspective of immune mechanisms, chemotherapeutic agents can enhance the immune response by inducing immunogenic cell death, potentiating T-cell activation, and increasing the activity of tumor-killing immune cells (77). Whereas, the tumor microenvironment also contributes to an increase in resistance to chemotherapy drugs (78). With ongoing research on tumor molecules, targeted therapy is becoming a crucial treatment for non-small cell lung cancer. EGFR, KRAS, and ALK are the primary susceptibility genes for common NSCLC driver mutations (79, 80). Among these, EGFR mutation is a crucial factor for cluster analysis in this field. Targeted therapies exert their effects primarily on tumor cells by blocking specific signaling pathways. In addition, targeted therapies have a direct effect on tumor cells mainly by blocking specific signaling pathways, which the immune microenvironment interacts with to affect targeted drug sensitivity (81).
The tumor microenvironment also influences targeted drug resistance. EGFR mutations may decrease the number of CD8+ cells, elevate the Treg population, and activate the STAT-3 intracellular pathway, leading to immune escape and increased resistance to targeted drugs (82). Moreover, vascular endothelial growth factor (VEGF)-targeted drugs can affect the tumor microenvironment in NSCLC by inhibiting immune escape, normalizing tumor vasculature, modulating T-cell numbers, and increasing tumor immune cells (83). The field of immunotherapy has seen significant progress in the last decade, introducing new therapies that have become a standard treatment for patients with stage III or IV NSCLC. The tumor microenvironment plays a significant role in determining both the sensitivity and resistance to immune drugs (84). Immune checkpoint inhibitors (ICIs) represent another significant cluster in this field, encompassing a range of molecules, including PD-1, PD-L1, and CTLA-4, which exert anti-tumor effects by regulating the interaction of Treg cells with antigen-presenting cells or tumor cells (85). The antitumor efficacy of PD-1/PD-L1 blockers has been shown to correlate with an increased presence of CD8+ tumor-infiltrating lymphocytes and the overexpression of chemokines and cytokines in the tumor microenvironment (86). The efficacy of immune checkpoint inhibitors is linked to the activation of effector immune cells, such as tumor-infiltrating lymphocytes, dendritic cells, and others. Conversely, resistance is mainly associated with the infiltration of immune cells, including regulatory Treg cells, myeloid-derived suppressor cells (MDSCs), and tumor-associated macrophages, as well as the recruitment of chemokines and high expression of vascular endothelial growth factor (VEGF) (87). Compared with NSCLC, SCLC has no significant effect on immunotherapy. In addition to the low expression of PD-L1 in tumor cells, various factors such as low expression or deletion of MHC I and MHC II proteins in the tumor microenvironment, and inhibition of the proliferation of CD4+ cells can also contribute to immune escape (88).
Thirdly, the discussion will focus on the impact of new technologies and methods on the direction of research within this field. Single-cell RNA sequencing (scRNA-seq), one of the crucial research techniques in the field, is a novel approach that allows for the examination of the transcriptome of individual cells within a sequenced sample. This method facilitates the analysis of cell types and heterogeneity in gene expression (89). Hu et al. (90) demonstrated, using single-cell techniques and in vivo experiments, that tumor-associated macrophages (TAMs) promote IL-6 expression through the formation of an IL6-STAT3-C/EBPβ-IL6 positive feedback loop. This loop, in turn, induces the epithelial-to-mesenchymal transition (EMT) pathway as a mechanism to enhance migration, invasion, and metastasis in lung cancer. Han (91) found that Osimertinib, in combination with anti-angiogenic agents, increased the number of CD8 T cells and proliferation of T cells compared with a single agent by analyzing tumor tissue using ScRNA-seq. Mao (92) demonstrated that the expression of the CDC25C gene affects the invasion and migration of lung cancer cells through the study and analysis of scRNA-seq data, suggesting it may play a crucial role in the EMT pathway. Machine learning is also a significant research area in this field. It primarily focuses on the general concept of various models and strategies. Currently, machine learning methods are experiencing a gradual increase in the field of medical research (93). Cury et al. (94) employed machine learning modeling to predict the impact of the pectoralis major muscle region on NSCLC.
This study is subject to certain limitations. Firstly, the research data were exclusively retrieved from the SSCI and SCI-E databases of WoSCC, which may lead to incomplete data and related results. Secondly, the data we selected were exclusively published in English. This exclusion of books, conference papers, and other types of publications may have resulted in the omission of some articles. Thirdly, although this study employed rigorous screening criteria and a comprehensive double search and review process, the search formula may not fully encompass all relevant research findings in this field, potentially leading to the omission of crucial research contributions. Fourthly, since the software is not analyzed in the same manner, errors may exist in some of the results.
In this research, bibliometric methods were used to visualize articles on lung cancer and the tumor microenvironment published between 2014 and 2023. This approach enabled researchers to gain into the current status, frontiers, and hotspots in this field. The findings indicate that the number of publications in this field is generally increasing. The majority of these publications are authored by researchers in China and the United States. Additionally, research on the correlation between the tumor microenvironment and lung cancer molecular signaling pathways and therapy is gaining increasing attention. These fields are expected to be significant focal points for future research on lung cancer and tumor microenvironment.
The original contributions presented in the study are included in the article/Supplementary Material. Further inquiries can be directed to the corresponding author.
ZH: Data curation, Formal Analysis, Investigation, Methodology, Project administration, Supervision, Validation, Visualization, Writing – original draft, Writing – review & editing. TX: Formal Analysis, Investigation, Software, Supervision, Validation, Visualization, Writing – original draft, Writing – review & editing. WX: Funding acquisition, Project administration, Resources, Supervision, Validation, Writing – original draft, Writing – review & editing. ZC: Data curation, Investigation, Software, Validation, Visualization, Writing – review & editing. ZW: Investigation, Methodology, Software, Validation, Visualization, Writing – review & editing. LY: Investigation, Methodology, Software, Validation, Visualization, Writing – review & editing.
The author(s) declare financial support was received for the research, authorship, and/or publication of this article. This work was supported by the Sanming Project of Medicine in Shenzhen (grant number SZZYSM202311001).
The authors declare that the research was conducted in the absence of any commercial or financial relationships that could be construed as a potential conflict of interest.
All claims expressed in this article are solely those of the authors and do not necessarily represent those of their affiliated organizations, or those of the publisher, the editors and the reviewers. Any product that may be evaluated in this article, or claim that may be made by its manufacturer, is not guaranteed or endorsed by the publisher.
The Supplementary Material for this article can be found online at: https://www.frontiersin.org/articles/10.3389/fonc.2024.1428018/full#supplementary-material
1. Schabath MB, Cote ML. Cancer progress and priorities: lung cancer. Cancer Epidemiol Biomarkers Prev. (2019) 28:1563–79. doi: 10.1158/1055-9965.EPI-19-0221
2. Siegel RL, Miller KD, Wagle NS, Jemal A. Cancer statistics, 2023. CA Cancer J Clin. (2023) 73:17–48. doi: 10.3322/caac.21763
3. Sung H, Ferlay J, Siegel RL, Laversanne M, Soerjomataram I, Jemal A, et al. Global cancer statistics 2020: GLOBOCAN estimates of incidence and mortality worldwide for 36 cancers in 185 countries. CA Cancer J Clin. (2021) 71:209–49. doi: 10.3322/caac.21660
4. Muthusamy B, Berktas M, Li J, Thomas DS, Sun P, Taylor A, et al. EGFR mutation testing, treatment and survival in stage I-III non-small-cell lung cancer: CancerLinQ Discovery database retrospective analysis. Future Oncol. (2024), 1–14. doi: 10.1080/14796694.2024.2347826
5. Dai J, Su Y, Zhong S, Cong L, Liu B, Yang J, et al. Exosomes: key players in cancer and potential therapeutic strategy. Signal Transduct Target Ther. (2020) 5:145. doi: 10.1038/s41392-020-00261-0
6. Jin MZ, Jin WL. The updated landscape of tumor microenvironment and drug repurposing. Signal Transduct Target Ther. (2020) 5:166. doi: 10.1038/s41392-020-00280-x
7. Zulfiqar B, Farooq A, Kanwal S, Asghar K. Immunotherapy and targeted therapy for lung cancer: Current status and future perspectives. Front Pharmacol. (2022) 13:1035171. doi: 10.3389/fphar.2022.1035171
8. Lemjabbar-Alaoui H, Hassan OU, Yang YW, Buchanan P. Lung cancer: Biology and treatment options. Biochim Biophys Acta. (2015) 1856:189–210. doi: 10.1016/j.bbcan.2015.08.002
9. de Visser KE, Joyce JA. The evolving tumor microenvironment: From cancer initiation to metastatic outgrowth. Cancer Cell. (2023) 41:374–403. doi: 10.1016/j.ccell.2023.02.016
10. Korbecki J, Olbromski M, Dzięgiel P. CCL18 in the progression of cancer. Int J Mol Sci. (2020) 21(21):7955. doi: 10.3390/ijms21217955
11. Zeng W, Xiong L, Wu W, Li S, Liu J, Yang L, et al. CCL18 signaling from tumor-associated macrophages activates fibroblasts to adopt a chemoresistance-inducing phenotype. Oncogene. (2023) 42:224–37. doi: 10.1038/s41388-022-02540-2
12. Xiang Y, Liu X, Wang Y, Zheng D, Meng Q, Jiang L, et al. Mechanisms of resistance to targeted therapy and immunotherapy in non-small cell lung cancer: promising strategies to overcoming challenges. Front Immunol. (2024) 15:1366260. doi: 10.3389/fimmu.2024.1366260
13. Hirayama A, Tanaka K, Tsutsumi H, Nakanishi T, Yamashita S, Mizusaki S, et al. Regulation of PD-L1 expression in non-small cell lung cancer by interleukin-1β. Front Immunol. (2023) 14:1192861. doi: 10.3389/fimmu.2023.1192861
14. Kao KC, Vilbois S, Tsai CH, Ho PC. Metabolic communication in the tumour-immune microenvironment. Nat Cell Biol. (2022) 24:1574–83. doi: 10.1038/s41556-022-01002-x
15. Lim AR, Rathmell WK, Rathmell JC. The tumor microenvironment as a metabolic barrier to effector T cells and immunotherapy. Elife. (2020) 9:e55185. doi: 10.7554/eLife.55185
16. Zhou Y, Cheng L, Liu L, Li X. NK cells are never alone: crosstalk and communication in tumour microenvironments. Mol Cancer. (2023) 22:34. doi: 10.1186/s12943-023-01737-7
17. Barta JA, Powell CA, Wisnivesky JP. Global epidemiology of lung cancer. Ann Glob Health. (2019) 85(1):8. doi: 10.5334/aogh.2419
18. Clément-Duchêne C, Vignaud JM, Stoufflet A, Bertrand O, Gislard A, Thiberville L, et al. Characteristics of never smoker lung cancer including environmental and occupational risk factors. Lung Cancer. (2010) 67:144–50. doi: 10.1016/j.lungcan.2009.04.005
19. Thompson DF, Walker CK. A descriptive and historical review of bibliometrics with applications to medical sciences. Pharmacotherapy. (2015) 35:551–9. doi: 10.1002/phar.1586
20. Abouzid M, Karaźniewicz-Łada M, Abdelazeem B, Brašić JR. Research trends of vitamin D metabolism gene polymorphisms based on a bibliometric investigation. Genes (Basel). (2023) 14(1):215. doi: 10.3390/genes14010215
21. Chen C. Science mapping: A systematic review of the literature. J Data Inf Sci. (2017) 2:1–40. doi: 10.1515/jdis-2017-0006
22. van Eck NJ, Waltman L. Software survey: VOSviewer, a computer program for bibliometric mapping. Scientometrics. (2010) 84:523–38. doi: 10.1007/s11192-009-0146-3
23. Massimo A, Corrado C. bibliometrix: An R-tool for comprehensive science mapping analysis. J Informetrics. (2017) 11:959–75. doi: 10.1016/j.joi.2017.08.007
24. Xia DM, Wang XR, Zhou PY, Ou TL, Su L, Xu SG. Research progress of heat stroke during 1989-2019: a bibliometric analysis. Mil Med Res. (2021) 8:5. doi: 10.1186/s40779-021-00300-z
25. Jemal A, Bray F, Center MM, Ferlay J, Ward E, Forman D. Global cancer statistics. CA Cancer J Clin. (2011) 61:69–90. doi: 10.3322/caac.v61:2
26. Zhao J, Li M. Worldwide trends in prediabetes from 1985 to 2022: A bibliometric analysis using bibliometrix R-tool. Front Public Health. (2023) 11:1072521. doi: 10.3389/fpubh.2023.1072521
27. Xu Q, Zhou Y, Zhang H, Li H, Qin H, Wang H. Bibliometric analysis of hotspots and frontiers of immunotherapy in pancreatic cancer. Healthcare (Basel). (2023) 11(3):304. doi: 10.3390/healthcare11030304
28. Badalamenti G, Fanale D, Incorvaia L, Barraco N, Listì A, Maragliano R, et al. Role of tumor-infiltrating lymphocytes in patients with solid tumors: Can a drop dig a stone? Cell Immunol. (2019) 343:103753. doi: 10.1016/j.cellimm.2018.01.013
29. Bremnes RM, Busund LT, Kilvær TL, Andersen S, Richardsen E, Paulsen EE, et al. The role of tumor-infiltrating lymphocytes in development, progression, and prognosis of non-small cell lung cancer. J Thorac Oncol. (2016) 11:789–800. doi: 10.1016/j.jtho.2016.01.015
30. Caetano MS, Zhang H, Cumpian AM, Gong L, Unver N, Ostrin EJ, et al. IL6 blockade reprograms the lung tumor microenvironment to limit the development and progression of K-ras-mutant lung cancer. Cancer Res. (2016) 76:3189–99. doi: 10.1158/0008-5472.CAN-15-2840
31. Faget J, Groeneveld S, Boivin G, Sankar M, Zangger N, Garcia M, et al. Neutrophils and snail orchestrate the establishment of a pro-tumor microenvironment in lung cancer. Cell Rep. (2017) 21:3190–204. doi: 10.1016/j.celrep.2017.11.052
32. Koyama S, Akbay EA, Li YY, Aref AR, Skoulidis F, Herter-Sprie GS, et al. STK11/LKB1 deficiency promotes neutrophil recruitment and proinflammatory cytokine production to suppress T-cell activity in the lung tumor microenvironment. Cancer Res. (2016) 76:999–1008. doi: 10.1158/0008-5472.CAN-15-1439
33. Lou Y, Diao L, Cuentas ER, Denning WL, Chen L, Fan YH, et al. Epithelial-mesenchymal transition is associated with a distinct tumor microenvironment including elevation of inflammatory signals and multiple immune checkpoints in lung adenocarcinoma. Clin Cancer Res. (2016) 22:3630–42. doi: 10.1158/1078-0432.CCR-15-1434
34. Steggerda SM, Bennett MK, Chen J, Emberley E, Huang T, Janes JR, et al. Inhibition of arginase by CB-1158 blocks myeloid cell-mediated immune suppression in the tumor microenvironment. J Immunother Cancer. (2017) 5:101. doi: 10.1186/s40425-017-0308-4
35. Wood SL, Pernemalm M, Crosbie PA, Whetton AD. The role of the tumor-microenvironment in lung cancer-metastasis and its relationship to potential therapeutic targets. Cancer Treat Rev. (2014) 40:558–66. doi: 10.1016/j.ctrv.2013.10.001
36. Zhang X, Zeng Y, Qu Q, Zhu J, Liu Z, Ning W, et al. PD-L1 induced by IFN-γ from tumor-associated macrophages via the JAK/STAT3 and PI3K/AKT signaling pathways promoted progression of lung cancer. Int J Clin Oncol. (2017) 22:1026–33. doi: 10.1007/s10147-017-1161-7
37. Zhao S, Ren S, Jiang T, Zhu B, Li X, Zhao C, et al. Low-dose apatinib optimizes tumor microenvironment and potentiates antitumor effect of PD-1/PD-L1 blockade in lung cancer. Cancer Immunol Res. (2019) 7:630–43. doi: 10.1158/2326-6066.CIR-17-0640
38. Altorki NK, Markowitz GJ, Gao D, Port JL, Saxena A, Stiles B, et al. The lung microenvironment: an important regulator of tumour growth and metastasis. Nat Rev Cancer. (2019) 19:9–31. doi: 10.1038/s41568-018-0081-9
39. Borghaei H, Paz-Ares L, Horn L, Spigel DR, Steins M, Ready NE, et al. Nivolumab versus docetaxel in advanced nonsquamous non-small-cell lung cancer. N Engl J Med. (2015) 373:1627–39. doi: 10.1056/NEJMoa1507643
40. Hanahan D, Weinberg RA. Hallmarks of cancer: the next generation. Cell. (2011) 144:646–74. doi: 10.1016/j.cell.2011.02.013
41. Herbst RS, Morgensztern D, Boshoff C. The biology and management of non-small cell lung cancer. Nature. (2018) 553:446–54. doi: 10.1038/nature25183
42. Newman AM, Liu CL, Green MR, Gentles AJ, Feng W, Xu Y, et al. Robust enumeration of cell subsets from tissue expression profiles. Nat Methods. (2015) 12:453–7. doi: 10.1038/nmeth.3337
43. Reck M, Rodríguez-Abreu D, Robinson AG, Hui R, Csőszi T, Fülöp A, et al. Pembrolizumab versus chemotherapy for PD-L1-positive non-small-cell lung cancer. N Engl J Med. (2016) 375:1823–33. doi: 10.1056/NEJMoa1606774
44. Rittmeyer A, Barlesi F, Waterkamp D, Park K, Ciardiello F, von Pawel J, et al. Atezolizumab versus docetaxel in patients with previously treated non-small-cell lung cancer (OAK): a phase 3, open-label, multicentre randomised controlled trial. Lancet. (2017) 389:255–65. doi: 10.1016/S0140-6736(16)32517-X
45. Siegel RL, Miller KD, Fuchs HE, Jemal A. Cancer statistics, 2021. CA Cancer J Clin. (2021) 71:7–33. doi: 10.3322/caac.21654
46. Yoshihara K, Shahmoradgoli M, Martínez E, Vegesna R, Kim H, Torres-Garcia W, et al. Inferring tumour purity and stromal and immune cell admixture from expression data. Nat Commun. (2013) 4:2612. doi: 10.1038/ncomms3612
47. Huang X, Fan X, Ying J, Chen S. Emerging trends and research foci in gastrointestinal microbiome. J Transl Med. (2019) 17:67. doi: 10.1186/s12967-019-1810-x
48. Long J, Zhai M, Jiang Q, Li J, Xu C, Chen D. The incidence and mortality of lung cancer in China: a trend analysis and comparison with G20 based on the Global Burden of Disease Study 2019. Front Oncol. (2023) 13:1177482. doi: 10.3389/fonc.2023.1177482
49. Chen P, Zhang L, Zhang W, Sun C, Wu C, He Y, et al. Galectin-9-based immune risk score model helps to predict relapse in stage I-III small cell lung cancer. J Immunother Cancer. (2020) 8(2):e001391. doi: 10.1136/jitc-2020-001391
50. Jiang M, Wu C, Zhang L, Sun C, Wang H, Xu Y, et al. FOXP3-based immune risk model for recurrence prediction in small-cell lung cancer at stages I-III. J Immunother Cancer. (2021) 9(5):e002339. doi: 10.1136/jitc-2021-002339
51. Qiao M, Jiang T, Liu X, Mao S, Zhou F, Li X, et al. Immune checkpoint inhibitors in EGFR-mutated NSCLC: dusk or dawn? J Thorac Oncol. (2021) 16:1267–88. doi: 10.1016/j.jtho.2021.04.003
52. Karger A, Mansouri S, Leisegang MS, Weigert A, Günther S, Kuenne C, et al. ADPGK-AS1 long noncoding RNA switches macrophage metabolic and phenotypic state to promote lung cancer growth. EMBO J. (2023) 42:e111620. doi: 10.15252/embj.2022111620
53. Karger A, Nandigama R, Stenzinger A, Grimminger F, Pullamsetti SS, Seeger W, et al. Hidden treasures: macrophage long non-coding RNAs in lung cancer progression. Cancers (Basel). (2021) 13(16):4127. doi: 10.3390/cancers13164127
54. Marwitz S, Turkowski K, Nitschkowski D, Weigert A, Brandenburg J, Reiling N, et al. The multi-modal effect of the anti-fibrotic drug pirfenidone on NSCLC. Front Oncol. (2019) 9:1550. doi: 10.3389/fonc.2019.01550
55. Sarode P, Schaefer MB, Grimminger F, Seeger W, Savai R. Macrophage and tumor cell cross-talk is fundamental for lung tumor progression: we need to talk. Front Oncol. (2020) 10:324. doi: 10.3389/fonc.2020.00324
56. Mass E, Ballesteros I, Farlik M, Halbritter F, Günther P, Crozet L, et al. Specification of tissue-resident macrophages during organogenesis. Science. (2016) 353(6304):aaf4238. doi: 10.1126/science.aaf4238
57. Song Y, Hu J, Ma C, Liu H, Li Z, Yang Y. Macrophage-derived exosomes as advanced therapeutics for inflammation: current progress and future perspectives. Int J Nanomedicine. (2024) 19:1597–627. doi: 10.2147/IJN.S449388
58. Boutilier AJ, Elsawa SF. Macrophage polarization states in the tumor microenvironment. Int J Mol Sci. (2021) 22(13):6995. doi: 10.3390/ijms22136995
59. Li C, Xu X, Wei S, Jiang P, Xue L, Wang J. Tumor-associated macrophages: potential therapeutic strategies and future prospects in cancer. J Immunother Cancer. (2021) 9(1):e001341. doi: 10.1136/jitc-2020-001341
60. Orecchioni M, Ghosheh Y, Pramod AB, Ley K. Macrophage Polarization: Different Gene Signatures in M1(LPS+) vs. Classically and M2(LPS-) vs. Alternatively Activated Macrophages. Front Immunol. (2019) 10:1084. doi: 10.3389/fimmu.2019.01084
61. Liu J, Cao X. Glucose metabolism of TAMs in tumor chemoresistance and metastasis. Trends Cell Biol. (2023) 33:967–78. doi: 10.1016/j.tcb.2023.03.008
62. Kalluri R. The biology and function of fibroblasts in cancer. Nat Rev Cancer. (2016) 16:582–98. doi: 10.1038/nrc.2016.73
63. Qiao M, Zhou F, Liu X, Jiang T, Wang H, Li X, et al. Targeting focal adhesion kinase boosts immune response in KRAS/LKB1 co-mutated lung adenocarcinoma via remodeling the tumor microenvironment. Exp Hematol Oncol. (2024) 13:11. doi: 10.1186/s40164-023-00471-6
64. Samart P, Heenatigala Palliyage G, Issaragrisil S, Luanpitpong S, Rojanasakul Y. Musashi-2 in cancer-associated fibroblasts promotes non-small cell lung cancer metastasis through paracrine IL-6-driven epithelial-mesenchymal transition. Cell Biosci. (2023) 13:205. doi: 10.1186/s13578-023-01158-5
65. Cords L, Engler S, Haberecker M, Rüschoff JH, Moch H, de Souza N, et al. Cancer-associated fibroblast phenotypes are associated with patient outcome in non-small cell lung cancer. Cancer Cell. (2024) 42:396–412.e5. doi: 10.1016/j.ccell.2023.12.021
66. Martínez-Reyes I, Chandel NS. Cancer metabolism: looking forward. Nat Rev Cancer. (2021) 21:669–80. doi: 10.1038/s41568-021-00378-6
67. Stine ZE, Schug ZT, Salvino JM, Dang CV. Targeting cancer metabolism in the era of precision oncology. Nat Rev Drug Discovery. (2022) 21:141–62. doi: 10.1038/s41573-021-00339-6
68. Fahrmann JF, Vykoukal JV, Ostrin EJ. Amino acid oncometabolism and immunomodulation of the tumor microenvironment in lung cancer. Front Oncol. (2020) 10:276. doi: 10.3389/fonc.2020.00276
69. Liu J, Shen H, Gu W, Zheng H, Wang Y, Ma G, et al. Prediction of prognosis, immunogenicity and efficacy of immunotherapy based on glutamine metabolism in lung adenocarcinoma. Front Immunol. (2022) 13:960738. doi: 10.3389/fimmu.2022.960738
70. Yang Y, Liu L, Sun J, Wang S, Yang Z, Li H, et al. Deoxypodophyllotoxin inhibits non-small cell lung cancer cell growth by reducing HIF-1α-mediated glycolysis. Front Oncol. (2021) 11:629543. doi: 10.3389/fonc.2021.629543
71. da Cunha Santos G, Shepherd FA, Tsao MS. EGFR mutations and lung cancer. Annu Rev Pathol. (2011) 6:49–69. doi: 10.1146/annurev-pathol-011110-130206
72. Hirsch FR, Scagliotti GV, Mulshine JL, Kwon R, Curran WJ Jr., Wu YL, et al. Lung cancer: current therapies and new targeted treatments. Lancet. (2017) 389:299–311. doi: 10.1016/S0140-6736(16)30958-8
73. Vinod SK, Hau E. Radiotherapy treatment for lung cancer: Current status and future directions. Respirology. (2020) 25 Suppl 2:61–71. doi: 10.1111/resp.13870
74. Nagasaka M, Gadgeel SM. Role of chemotherapy and targeted therapy in early-stage non-small cell lung cancer. Expert Rev Anticancer Ther. (2018) 18:63–70. doi: 10.1080/14737140.2018.1409624
75. Feliu J, Heredia-Soto V, Gironés R, Jiménez-Munarriz B, Saldaña J, Guillén-Ponce C, et al. Management of the toxicity of chemotherapy and targeted therapies in elderly cancer patients. Clin Transl Oncol. (2020) 22:457–67. doi: 10.1007/s12094-019-02167-y
76. Livshits Z, Rao RB, Smith SW. An approach to chemotherapy-associated toxicity. Emerg Med Clin North Am. (2014) 32:167–203. doi: 10.1016/j.emc.2013.09.002
77. Xue Y, Gao S, Gou J, Yin T, He H, Wang Y, et al. Platinum-based chemotherapy in combination with PD-1/PD-L1 inhibitors: preclinical and clinical studies and mechanism of action. Expert Opin Drug Delivery. (2021) 18:187–203. doi: 10.1080/17425247.2021.1825376
78. Herzog BH, Devarakonda S, Govindan R. Overcoming chemotherapy resistance in SCLC. J Thorac Oncol. (2021) 16:2002–15. doi: 10.1016/j.jtho.2021.07.018
79. Leiter A, Veluswamy RR, Wisnivesky JP. The global burden of lung cancer: current status and future trends. Nat Rev Clin Oncol. (2023) 20:624–39. doi: 10.1038/s41571-023-00798-3
80. Nooreldeen R, Bach H. Current and future development in lung cancer diagnosis. Int J Mol Sci. (2021) 22(16):8661. doi: 10.3390/ijms22168661
81. Jia Y, Li X, Jiang T, Zhao S, Zhao C, Zhang L, et al. EGFR-targeted therapy alters the tumor microenvironment in EGFR-driven lung tumors: Implications for combination therapies. Int J Cancer. (2019) 145:1432–44. doi: 10.1002/ijc.32191
82. Madeddu C, Donisi C, Liscia N, Lai E, Scartozzi M, Macciò A. EGFR-mutated non-small cell lung cancer and resistance to immunotherapy: role of the tumor microenvironment. Int J Mol Sci. (2022) 23(12):6489. doi: 10.3390/ijms23126489
83. Zhao Y, Guo S, Deng J, Shen J, Du F, Wu X, et al. VEGF/VEGFR-targeted therapy and immunotherapy in non-small cell lung cancer: targeting the tumor microenvironment. Int J Biol Sci. (2022) 18:3845–58. doi: 10.7150/ijbs.70958
84. Dantoing E, Piton N, Salaün M, Thiberville L, Guisier F. Anti-PD1/PD-L1 immunotherapy for non-small cell lung cancer with actionable oncogenic driver mutations. Int J Mol Sci. (2021) 22(12):6288. doi: 10.3390/ijms22126288
85. Castellanos EH, Horn L. Immunotherapy in lung cancer. Cancer Treat Res. (2016) 170:203–23. doi: 10.1007/978-3-319-40389-2_10
86. Wu X, Gu Z, Chen Y, Chen B, Chen W, Weng L, et al. Application of PD-1 blockade in cancer immunotherapy. Comput Struct Biotechnol J. (2019) 17:661–74. doi: 10.1016/j.csbj.2019.03.006
87. Horvath L, Thienpont B, Zhao L, Wolf D, Pircher A. Overcoming immunotherapy resistance in non-small cell lung cancer (NSCLC) - novel approaches and future outlook. Mol Cancer. (2020) 19:141. doi: 10.1186/s12943-020-01260-z
88. Meijer JJ, Leonetti A, Airò G, Tiseo M, Rolfo C, Giovannetti E, et al. Small cell lung cancer: Novel treatments beyond immunotherapy. Semin Cancer Biol. (2022) 86:376–85. doi: 10.1016/j.semcancer.2022.05.004
89. Zhang J, Liu X, Huang Z, Wu C, Zhang F, Han A, et al. T cell-related prognostic risk model and tumor immune environment modulation in lung adenocarcinoma based on single-cell and bulk RNA sequencing. Comput Biol Med. (2023) 152:106460. doi: 10.1016/j.compbiomed.2022.106460
90. Hu Z, Sui Q, Jin X, Shan G, Huang Y, Yi Y, et al. IL6-STAT3-C/EBPβ-IL6 positive feedback loop in tumor-associated macrophages promotes the EMT and metastasis of lung adenocarcinoma. J Exp Clin Cancer Res. (2024) 43:63. doi: 10.1186/s13046-024-02989-x
91. Han R, Guo H, Shi J, Zhao S, Jia Y, Liu X, et al. Osimertinib in combination with anti-angiogenesis therapy presents a promising option for osimertinib-resistant non-small cell lung cancer. BMC Med. (2024) 22:174. doi: 10.1186/s12916-024-03389-w
92. Mao S, Wang Y, Chao N, Zeng L, Zhang L. Integrated analysis of single-cell RNA-seq and bulk RNA-seq reveals immune suppression subtypes and establishes a novel signature for determining the prognosis in lung adenocarcinoma. Cell Oncol (Dordr). (2024). doi: 10.1007/s13402-024-00948-4
93. Cooray U, Watt RG, Tsakos G, Heilmann A, Hariyama M, Yamamoto T, et al. Importance of socioeconomic factors in predicting tooth loss among older adults in Japan: Evidence from a machine learning analysis. Soc Sci Med. (2021) 291:114486. doi: 10.1016/j.socscimed.2021.114486
Keywords: Lung cancer, the tumor microenvironment (TME), bibliometric, visualized analysis, trend
Citation: Huang Z, Xie T, Xie W, Chen Z, Wen Z and Yang L (2024) Research trends in lung cancer and the tumor microenvironment: a bibliometric analysis of studies published from 2014 to 2023. Front. Oncol. 14:1428018. doi: 10.3389/fonc.2024.1428018
Received: 05 May 2024; Accepted: 16 July 2024;
Published: 31 July 2024.
Edited by:
Dong Hu, Anhui University of Science and Technology, ChinaReviewed by:
Shen Chen, Peking University, ChinaCopyright © 2024 Huang, Xie, Xie, Chen, Wen and Yang. This is an open-access article distributed under the terms of the Creative Commons Attribution License (CC BY). The use, distribution or reproduction in other forums is permitted, provided the original author(s) and the copyright owner(s) are credited and that the original publication in this journal is cited, in accordance with accepted academic practice. No use, distribution or reproduction is permitted which does not comply with these terms.
*Correspondence: Wei Xie, eGlldzA3MDNAMTYzLmNvbQ==
†These authors have contributed equally to this work
Disclaimer: All claims expressed in this article are solely those of the authors and do not necessarily represent those of their affiliated organizations, or those of the publisher, the editors and the reviewers. Any product that may be evaluated in this article or claim that may be made by its manufacturer is not guaranteed or endorsed by the publisher.
Research integrity at Frontiers
Learn more about the work of our research integrity team to safeguard the quality of each article we publish.