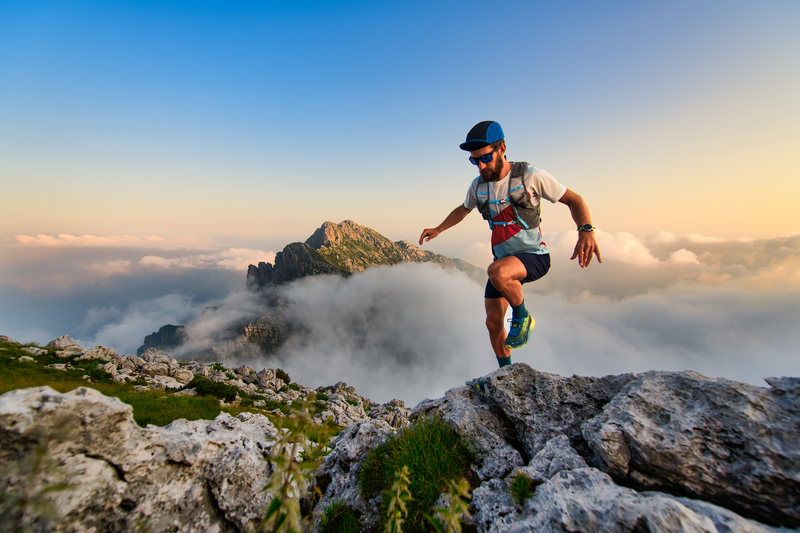
95% of researchers rate our articles as excellent or good
Learn more about the work of our research integrity team to safeguard the quality of each article we publish.
Find out more
REVIEW article
Front. Oncol. , 09 December 2024
Sec. Thoracic Oncology
Volume 14 - 2024 | https://doi.org/10.3389/fonc.2024.1422623
Non-small cell lung cancer (NSCLC) is a major subtype of lung cancer and poses a serious threat to human health. Due to the advances in lung cancer screening, more and more clinical T1 NSCLC defined as a tumor with a maximum diameter of 3cm surrounded by lung tissue or visceral pleura have been detected and have achieved favorable treatment outcomes, greatly improving the prognosis of NSCLC patients. However, the preoperative lymph node staging and intraoperative lymph node dissection patterns of operable clinical T1 NSCLC are still subject to much disagreement, as well as the heterogeneity between primary tumors and metastatic lymph nodes poses a challenge in designing effective treatment strategies. This article comprehensively describes the clinical risk factors of clinical T1 NSCLC lymph node metastasis, and its invasive and non-invasive prediction, focusing on the genetic heterogeneity between the primary tumor and the metastatic lymph nodes, which is significant for a thoroughly understanding of the biological behavior of early-stage NSCLC.
Non-small cell lung cancer (NSCLC) is the most diagnosed subtype of lung cancer worldwide and poses a growing threat to human health (1). Despite the breakthroughs in precision molecular targeted therapies and immunotherapy have dramatically improved the treatment landscape for patients with advanced lung cancer (2, 3), significantly reducing mortality in lung cancer patients is attributed to the low-dose computed tomography (LDCT)-based lung cancer screening in high-risk populations, which has resulted in an increasing number of early-stage lung cancers being detected especially clinical T1 NSCLC (tumor size ≤ 3 cm) (4). Furthermore, early cancer detection creates time window to minimize lymph node metastasis(LNM) (5). LNM is the most common metastatic route and the most critical factor affecting the prognosis of NSCLC. However, there is still no consensus on the lymph node detection and dissection strategy for clinical T1 NSCLC (6, 7). In addition, accurate identification of lymph nodes and prediction of tumor spread risk, as well as tumor mutational heterogeneity, are critical for guiding optimal staging and personalized treatment of NSCLC patients (8).
LNM in NSCLC patients is strongly associated with age, smoking status, tumor size, histology and differentiation, carcinoembryonic antigen level, and vascular invasion (+) and pleural involvement (+) (9–11). The pattern of regional LNM in clinical T1 peripheral NSCLC is significantly influenced by tumor size (12). Compared to primary tumor size, a large solid portion is a more critical predictor of LNM in patients with clinical T1 partially solid lung adenocarcinoma (13, 14). Moreover, patients with micropapillary or solid components are associated with LNM, while the gross glass opacity (GGO) components or microscopic invasive adenocarcinomas ≤2.0 cm or invasive mucinous adenocarcinomas are less prone to LNM (15–17). Besides, centrally located T1 tumors also predict a higher risk of pathological upstaging (18, 19) Figure 1.
Lymph node dissection (LND) is categorized into systemic/lobe-specific mediastinal lymph node dissection (m-LND) and hilar lymph node dissection (h-LND) only according to its extent (20). Selective LND is feasible in patients with clinical T1N0 NSCLC with predominantly GGO tumors, and pure GGO subproportion even do not need LND given the approximate 0% rate of LNM (21, 22). Interestingly, some studies show there is no significant correlation between LNM rate and tumor size for patients in the ≤2 cm mixed ground glass nodule (mGGN) group, and lobe-specific m-LND was suggested for patients with a solid component ≤2 cm pure solid nodule (PSN) (23–25). Furthermore, different primary tumor lung lobe locations with different propensities for mediastinal lymph node metastasis are observed in clinical T1N0 peripheral NSCLC just as for tumors in the upper lobe (≤3 cm), lower m-LND is not required, whereas for tumors in the lower lobe (≤2 cm), upper m-LND is not necessary (26, 27). However, occult nodal disease in patients with small (≤2 cm) nodes of clinical N0 NSCLC often occurs at the peripheral N1 station (11-13), therefore h-LND is essential for accurate staging in patients with clinical N0 NSCLC (28). Yet the controversy remains, a multicentric evaluation study reported that pN1 status in cN0 patients with central NSCLC tumors was observed in up to 27% of cases (29). This is inconsistent with newer studies reporting occult N1 rates of only between 2-3% (30, 31). Differently, some demonstrate that routine dissection of the aortopulmonary zone and inferior mediastinal nodes is sufficient to ensure staging accuracy, and more LND does not improve survival but may increase the risk of postoperative complications (32). Clinical parameters such as lung membrane invasion, vascular invasion, and carcinoembryonic antigen (CEA) were applied to stratify the risk of LNM, which in turn led to different LNDs (33). Others, such as intratumoral lymphovascular infiltration, are risk factors for LNM in patients with NSCLC, and adjuvant therapy should be considered for such patients (34).
Due to tumor trans-airspace spread, sublobar resection is associated with regionally occult lymph node metastasis and further stratifying patients with stage IA lung adenocarcinoma on the risk of recurrence according to the extent of resection (wedge resection > segmental resection > lobectomy) (35). Additionally, video-assisted thoracoscopic surgery and robotic lobectomy have a lower rate of pathologic LN upstaging after lobectomy compared with conventional open-thoracic surgery (36). Surgeons should thoroughly evaluate hilar and mediastinal nodal metastases and select a reasonable LND.
Radiologists’ assessment of lymph node status based on preoperative CT lacks high accuracy for patients with early-stage lung cancer and is inefficient. Combining CT radiological features with clinical histopathological models of the primary tumor and lymph nodes shows great potential in predicting lymph node metastasis in resectable NSCLC. More importantly, the pre-surgical CT-based radiomics model performed better than the clinical model in predicting LNM in stage IA NSCLC patients, and can be used for non-invasive quantitative prediction of mediastinal LNM in lung adenocarcinoma (37, 38). Using inner margin ratio (IMR) and outer margin ratio (OMR) thresholds are capable of predicting N1 metastases in patients with clinical T1 NSCLC staged on imaging (39), and electron density (ED) derived from dual-energy CT (DECT) is useful in the diagnosis of LNM in NSCLC (40). Some textural features from CT are associated with the degree of malignancy of mediastinal lymph nodes (41). Models of radiomic features extracted from gross tumor volume (GTV), peritumor volume (PTV), and CT histogram analysis of tumors could be used for preoperative prediction of LNM in T1 peripheral lung adenocarcinoma. Besides, CT-based radiological consensus clustering is able to identify associations between radiological features and clinicopathological and genomic features and prognosis (42–45).
Deep machine learning of radiologists’ CT readings and their clinical information can be used to guide clinical management of high-risk populations following screening CT (46). Swin Transformer-based deep learning features in predicting LNM outperforms radiomics features and clinical semantic models in extracting common multilevel features from high-resolution 3D CT images, where the Feature Dynamic Transfer (FDT) module facilitates the ability to recognize LNM (47, 48). Additionally, a cross-modal 3D neural network deep learning approach based on CT images and prior clinical knowledge performed significantly better than the radiomics approach and radiologists, improving the diagnostic accuracy of predicting LNM in clinical stage T1 lung adenocarcinoma (49, 50).
PET/CT is more accurate and sensitive than CT for mediastinal staging (51). Lymph nodes in NSCLC patients are usually evaluated using [18F]FDG-PET/CT, and DTP PET/CT using a semiquantitative technique has demonstrated good diagnostic performance in detecting mediastinal LNM in NSCLC patients (52, 53). Metastatic lymph nodes showed higher FDG uptake than false-positive lymph nodes (54). Lung invasive adenocarcinomas with micropapillary or solid components had higher SUVmax, MTV, TLG, and were associated with LNM. SUVmax had high specificity in predicting mediastinal LNM in 10.1-30.0 mm solid NSCLC. Assessment of SUVmax on a 5-point scale using the Deauville score is helpful in predicting LNM in early-stage lung adenocarcinoma. Lymph nodes SUVmax is also associated with the presence of tumor-promoting stromal cells in LNM (55–58). TLGsur showed strong predictive performance in predicting occult lymph node metastasis in clinically node-negative (cN0) lung adenocarcinoma (59). However, FDG PET/CT has a limited role in the preoperative detection of lymph nodes or distant metastases in patients with sub-solid NSCLC with solid portions 3 cm or smaller in size (60).
A machine learning model based on [18F]FDG-PET/CT routinely available variables improves the accuracy of mediastinal LN staging compared to established visual assessment criteria; a support vector machine (SVM) model is able to predict metastatic lymph nodes, and a machine-learning-based model-free algorithm for generating probabilistic maps based on a number of spatial and temporal features of 18F-FDG uptake can improve the specificity of distinguishing adenocarcinoma and its identification of metastatic lymph nodes (61–63). Machine learning models that incorporate clinical information into quantitative variables of 18F-FDG PET/CT can improve the diagnostic accuracy of LNM. The Tumor and Lymph PET/CT Clinical Model (TLPC) model can noninvasively predict LNM in NSCLC, which can help clinicians develop more rational treatment strategies (64, 65).
Circulating tumor DNA (ctDNA) has emerged as a non-invasive biomarker for dynamic tumor monitoring and is a non-invasive means of assessing intra-tumor heterogeneity (66). For instance, high Variant allele frequency (VAF) levels in preoperative ctDNA may predict LNM in resectable NSCLC (67). Compared with conventional radiography, ctDNA analysis can detect the smallest residual lesions of resectable NSCLC, thus facilitating early intervention (68). Utilizing ctDNA-based liquid biopsies with help to gain insight into the process of metastatic spread (69). A ctDNA-based preoperative noninvasive prediction model for LNM in patients with resectable NSCLC has satisfactory discrimination and calibration.
One-step nucleic acid amplification (OSNA) is a rapid intraoperative molecular testing technique to quantitatively assess tumor burden in resected lymph nodes of lung cancer patients by quantitatively measuring keratin 19 (CK19) mRNA, which provides high diagnostic accuracy and speed for detection of LNM, and can be applied to intraoperative decision-making for personalized lung cancer surgery (70, 71). Moreover, folate receptor-positive circulating tumor cells have a predictive value for the preoperative diagnosis of LNM (72). More and more measures are developed, as a zebrafish tumor xenograft zebrafish tumor xenograft model based on implantation of Patient-derived xenograft tissue fragments has high sensitivity for predicting LNM (73).
In NSCLC patients at risk for LNM, invasive mediastinal lymph node staging prior to curative resection is associated with significantly improved survival (74). EBUS-TBNA has been established as a first-line staging option for patients with lung cancer (75). Especially in patients with central clinically staged T1N0M0 NSCLC, EBUS-TBNA provide an extraordinary diagnostic accuracy for mediastinal staging (76). Therefor, guidelines recommend invasive mediastinal staging for patients with centrally locate NSCLC, however, the selection of candidates for invasive mediastinal staging for patients with clinical T1N0M0 lung cancer based solely on the location of the central tumor is controversial (77). Some studies demonstrate that subsequent invasive staging may not be necessary for those with peripheral T1 tumors with a prominent ground-glass component after a negative PET-CT (18).
Additionally, micrometastases such as occult lymph node metastasis(OLM) should be selected for mediastinoscopy or EBUS-TBNA (78). Accurate pN0 diagnosis depends on the number of LNs examined, which in turn quantifies the risk of OLM in patients with pN0 NSCLC, and a risk stratification model categorizes EBUS-TBNA-negative lymph nodes into different risk groups (79, 80). Metastatic hilar or mediastinal lymph nodes can be effectively obtained by EBUS-TBNA or the convex probe EBUS (CP-EBUS), and further EGFR, KRAS evaluation is not inferior to conventional lung cancer tissue samples (81, 82).
Unlike EBUS-TBNA bronchoscopy which is used primarily via the airway, endoscopic ultrasound with bronchoscopy-guided fine-needle aspiration (EUS-B-FNA) is used transesophagically for evaluation of lesions that cannot be accessed via the airway are gradually attracting the attention of oncologists. EUS-B-FNA improves the diagnostic yield of EBUS bronchoscopy for intrathoracic lesions (83). EUS-B-FNA is also a safe and accurate method for the diagnosis of paraesophageal lung lesions (84). Furthermore, the combination of EBUS and EUS can significantly improve the sensitivity of detection of mediastinal nodal metastases, thereby reducing the need for surgical staging (85). However, given the low incidence of occult mediastinal metastases and the poor sensitivity of endoscopy in this population, strategies for invasive mediastinal staging need to be adapted accordingly (86).
In addition to clinical and/or pathological diagnosis, comparison of driver mutation profiles of primary lung cancer tumors and their LNM can further differentiate between primary and metastatic tumors. Intratumor heterogeneity is the presence of multiple genetically distinct populations within a primary tumor, providing the basis for tumor metastasis (87). There are differences in the mutation profiles of key genes such as EGFR between primary lesions and metastatic lymph nodes in NSCLC (88, 89). Numerous studies have shown that lymph node metastatic status is associated with mutations in NSCLC driver genes (90). Different genotypes of NSCLC have different propensities for LNM, cases with fusion mutations have a higher risk and burden of LNM than other genotypes, and EGFR mutations are associated with N2 jump metastasis (N2 lymph node metastasis in the absence of N1) in lung adenocarcinoma (91–94). Increasingly, novel genes such as SMARCA1, SMARCA4 and SMAD4 alterations in lung adenocarcinoma are independently associated with LNM in lung adenocarcinoma (95, 96). The EGFR mutation status of metastatic lymph nodes also serves as a predictor of response to EGFR-TKI therapy in patients with recurrent NSCLC after surgical resection (97). Consequently, differences in gene mutation status between the primary tumor and the corresponding LNM should be taken into account when formulating a tyrosine kinase inhibitor-targeted treatment regimen (98).
In contrast, some researchers illustrate that given the minimal functional driver gene heterogeneity in primary-metastasis, a single biopsy of the primary tumor is sufficient to capture the majority of functionally important mutations in metastases (99, 100). Contradictory findings may be related to the means of detection, with ALK results detected by FISH showing more frequent inconsistencies between primary tumors and matched metastases compared to IHC, which may be due to the technique and the quality of the samples (101). Therefore, laboratory quality control of samples and the corresponding technical standards for testing should be continuously optimized.
More and more clinical T1N0 NSCLC are detected and effectively intervened with good prognosis, and the existence of heterogeneity between NSCLC primary tumor and metastatic lymph node lesions has been increasingly revealed with the application of high-throughput sequencing technology. However, the LNM pattern and the specific mechanism of early-stage lung cancer still need further research. Given early-stage lung cancer patients with high risk of LNM should be subjected to more intensive surveillance strategies after radical surgical treatment, developing the risk-predicting mold combining clinical and tumor genomic features which are capable of identifying patients at risk of pathological LNM is warranted. Especially, new approaches such as the use of validated predictive models that combine radiomics and ctDNA for noninvasive prediction will help to better select patients, still needs to be confirmed by prospective studies. Consequently, optimal preoperative lymph node assessment and prediction, as well as the extent of intraoperative lymph node dissection need to be confirmed by large-scale clinical randomized controlled trials.
LZ: Conceptualization, Data curation, Formal analysis, Investigation, Methodology, Project administration, Validation, Visualization, Writing – original draft, Writing – review & editing. FZ: Conceptualization, Investigation, Resources, Software, Supervision, Validation, Visualization, Writing – original draft, Writing – review & editing. GL: Investigation, Supervision, Validation, Writing – review & editing. XX: Data curation, Methodology, Project administration, Writing – review & editing. HL: Methodology, Project administration, Supervision, Validation, Writing – review & editing. YZ: Investigation, Methodology, Software, Supervision, Validation, Writing – review & editing.
The author(s) declare that no financial support was received for the research, authorship, and/or publication of this article.
The authors declare that the research was conducted in the absence of any commercial or financial relationships that could be construed as a potential conflict of interest.
All claims expressed in this article are solely those of the authors and do not necessarily represent those of their affiliated organizations, or those of the publisher, the editors and the reviewers. Any product that may be evaluated in this article, or claim that may be made by its manufacturer, is not guaranteed or endorsed by the publisher.
[18F]FDG-PET/CT, 18F-fluorodeoxyglucose positron emission tomography/computed tomography; SUVmax, maximum standardized uptake value; TLG, total lesion glycolysis; MTV, metabolic tumor volume; EBUS, endobronchial ultrasonography; EUS, esophageal ultrasonography; EBUS-TBNA, endobronchial ultrasound-guided transbronchial needle aspiration; EUS-B-FNA, EUS with bronchoscope-guided fine-needle aspiration; CP-EBUS, convex probe endobronchial ultrasound-guided transbronchial needle aspiration; EGFR, epidermal growth factor receptor; EGFR-TKI, epidermal growth factor receptor-tyrosine kinase inhibitor; KRAS, kirsten rat sarcoma; ALK, anaplastic lymphoma kinase; FISH, fluorescence in situ hybridization; IHC, Immunohistochemistry.
1. Zhang Y, Vaccarella S, Morgan E, Li M, Etxeberria J, Chokunonga E, et al. Global variations in lung cancer incidence by histological subtype in 2020: a population-based study. Lancet Oncol. (2023) 24:1206–18. doi: 10.1016/S1470-2045(23)00444-8
2. Li Z, Feiyue Z, Gaofeng L, Haifeng L. Lung cancer and oncolytic virotherapy–enemy’s enemy. Transl Oncol. (2023) 27:101563. doi: 10.1016/j.tranon.2022.101563
3. Li Y, Chen T, Nie TY, Han J, He Y, Tang X, et al. Hyperprogressive disease in non-small cell lung cancer after PD-1/PD-L1 inhibitors immunotherapy: underlying killer. Front Immunol. (2023) 14:1200875. doi: 10.3389/fimmu.2023.1200875
4. Oudkerk M, Liu S, Heuvelmans MA, Walter JE, Field JK. Lung cancer LDCT screening and mortality reduction - evidence, pitfalls and future perspectives. Nat Rev Clin Oncol. (2021) 18:135–51. doi: 10.1038/s41571-020-00432-6
5. Tang WF, Wu M, Bao H, Xu Y, Lin JS, Liang Y, et al. Timing and origins of local and distant metastases in lung cancer. J Thorac Oncol. (2021) 16:1136–48. doi: 10.1016/j.jtho.2021.02.023
6. Ray MA, Smeltzer MP, Faris NR, Osarogiagbon RU. Survival after mediastinal node dissection, systematic sampling, or neither for early stage NSCLC. J Thorac Oncol. (2020) 15:1670–81. doi: 10.1016/j.jtho.2020.06.009
7. Darling GE, Allen MS, Decker PA, Ballman K, Malthaner RA, Inculet RI, et al. Randomized trial of mediastinal lymph node sampling versus complete lymphadenectomy during pulmonary resection in the patient with N0 or N1 (less than hilar) non-small cell carcinoma: results of the American College of Surgery Oncology Group Z0030 Trial. J Thorac Cardiovasc Surg. (2011) 141:662–70. doi: 10.1016/j.jtcvs.2010.11.008
8. Asamura H, Chansky K, Crowley J, Goldstraw P, Rusch VW, Vansteenkiste JF, et al. The international association for the study of lung cancer lung cancer staging project: proposals for the revision of the N descriptors in the forthcoming 8th edition of the TNM classification for lung cancer. J Thorac oncology: Off Publ Int Assoc Study Lung Cancer. (2015) 10:1675–84. doi: 10.1097/JTO.0000000000000678
9. Shan L, Zhang L, Zhu X, Wang Z, Fang S, Lin J, et al. Chinese never smokers with adenocarcinoma of the lung are younger and have fewer lymph node metastases than smokers. Respir Res. (2022) 23:293. doi: 10.1186/s12931-022-02199-z
10. Chen B, Xia W, Wang Z, Zhao H, Li X, Liu L, et al. Risk analyses of N2 lymph-node metastases in patients with T1 non-small cell lung cancer: a multi-center real-world observational study in China. J Cancer Res Clin Oncol. (2019) 145:2771–7. doi: 10.1007/s00432-019-03006-x
11. Liang RB, Yang J, Zeng TS, Long H, Fu JH, Zhang LJ, et al. Incidence and distribution of lobe-specific mediastinal lymph node metastasis in non-small cell lung cancer: data from 4511 resected cases. Ann Surg Oncol. (2018) 25:3300–7. doi: 10.1245/s10434-018-6394-9
12. Deng HY, Zhou J, Wang RL, Jiang R, Qiu XM, Zhu DX, et al. Surgical choice for clinical stage IA non-small cell lung cancer: view from regional lymph node metastasis. Ann Thorac Surg. (2020) 109:1079–85. doi: 10.1016/j.athoracsur.2019.10.056
13. Lee SY, Jeon JH, Jung W, Chae M, Hwang WJ, Hwang Y, et al. Predictive factors for lymph node metastasis in clinical stage I part-solid lung adenocarcinoma. Ann Thorac Surg. (2021) 111:456–62. doi: 10.1016/j.athoracsur.2020.05.083
14. Li W, Zhou F, Wan Z, Li M, Zhang Y, Bao X, et al. Clinicopathologic features and lymph node metastatic characteristics in patients with adenocarcinoma manifesting as part-solid nodule exceeding 3cm in diameter. Lung Cancer. (2019) 136:37–44. doi: 10.1016/j.lungcan.2019.07.029
15. Zhang Y, Fu F, Wen Z, Deng L, Wang S, Li Y, et al. Segment location and ground glass opacity ratio reliably predict node-negative status in lung cancer. Ann Thorac Surg. (2020) 109:1061–8. doi: 10.1016/j.athoracsur.2019.10.072
16. Pani E, Kennedy G, Zheng X, Ukert B, Jarrar D, Gaughan C, et al. Factors associated with nodal metastasis in 2-centimeter or less non-small cell lung cancer. J Thorac Cardiovasc Surg. (2020) 159:1088–1096.e1. doi: 10.1016/j.jtcvs.2019.07.089
17. Wang L, Jiang W, Zhan C, Shi Y, Zhang Y, Lin Z, et al. Lymph node metastasis in clinical stage IA peripheral lung cancer. Lung Cancer (Amsterdam Netherlands). (2015) 90:41–6. doi: 10.1016/j.lungcan.2015.07.003
18. Gao SJ, Kim AW, Puchalski JT, Bramley K, Detterbeck FC, Boffa DJ, et al. Indications for invasive mediastinal staging in patients with early non-small cell lung cancer staged with PET-CT. Lung Cancer. (2017) 109:36–41. doi: 10.1016/j.lungcan.2017.04.018
19. Lee PC, Port JL, Korst RJ, Liss Y, Meherally DN, Altorki NK. Risk factors for occult mediastinal metastases in clinical stage I non-small cell lung cancer. Ann Thorac Surg. (2007) 84:177–81. doi: 10.1016/j.athoracsur.2007.03.081
20. Hattori A, Matsunaga T, Takamochi K, Oh S, Suzuki K. Significance of lymphadenectomy in part-solid lung adenocarcinoma: propensity score matched analysis. Ann Thorac Surg. (2018) 106:989–97. doi: 10.1016/j.athoracsur.2018.04.069
21. Lin YH, Chen CK, Hsieh CC, Hsu WH, Wu YC, Hung JJ, et al. Lymphadenectomy is unnecessary for pure ground-glass opacity pulmonary nodules. J Clin Med. (2020) 9(3):672. doi: 10.3390/jcm9030672
22. Haruki T, Aokage K, Miyoshi T, Hishida T, Ishii G, Yoshida J, et al. Mediastinal nodal involvement in patients with clinical stage I non-small-cell lung cancer: possibility of rational lymph node dissection. J Thorac Oncol. (2015) 10:930–6. doi: 10.1097/JTO.0000000000000546
23. Ye T, Deng L, Wang S, Xiang J, Zhang Y, Hu H, et al. Lung adenocarcinomas manifesting as radiological part-solid nodules define a special clinical subtype. J Thorac Oncol. (2019) 14:617–27. doi: 10.1016/j.jtho.2018.12.030
24. Wo Y, Li H, Chen Z, Peng Y, Zhang Y, Ye T, et al. Lobe-Specific Lymph Node Dissection May be Feasible for Clinical N0 Solid-Predominant Part-Solid Lung Adenocarcinoma With Solid Component Diameter ≤ 2 cm. Clin Lung Cancer. (2023) 24:437–44. doi: 10.1016/j.cllc.2023.03.004
25. Choi S, Yoon DW, Shin S, Kim HK, Choi YS, Kim J, et al. Importance of lymph node evaluation in ≤2-cm pure-solid non-small cell lung cancer. Ann Thorac Surg. (2024) 117(3):586–93. doi: 10.1016/j.athoracsur.2022.11.040
26. Yang MZ, Hou X, Liang RB, Lai RC, Yang J, Li S, et al. The incidence and distribution of mediastinal lymph node metastasis and its impact on survival in patients with non-small-cell lung cancers 3 cm or less: data from 2292 cases. Eur J Cardiothorac Surg. (2019) 56:159–66. doi: 10.1093/ejcts/ezy479
27. Deng HY, Zhou J, Wang RL, Jiang R, Zhu DX, Tang XJ, et al. Lobe-specific lymph node dissection for clinical early-stage (cIA) peripheral non-small cell lung cancer patients: what and how? Ann Surg Oncol. (2020) 27:472–80. doi: 10.1245/s10434-019-07926-3
28. Robinson EM, Ilonen IK, Tan KS, Plodkowski AJ, Bott M, Bains MS, et al. Prevalence of occult peribronchial N1 nodal metastasis in peripheral clinical N0 small (≤2 cm) non-small cell lung cancer. Ann Thorac Surg. (2020) 109:270–6. doi: 10.1016/j.athoracsur.2019.07.037
29. Decaluwé H, Petersen RH, Brunelli A, Pompili C, Seguin-Givelet A, Gust L, et al. Multicentric evaluation of the impact of central tumour location when comparing rates of N1 upstaging in patients undergoing video-assisted and open surgery for clinical Stage I non-small-cell lung cancer†. Eur J Cardiothorac Surg. (2018) 53:359–65. doi: 10.1093/ejcts/ezx338
30. Kukhon FR, Lan X, Helgeson SA, Arunthari V, Fernandez-Bussy S, Patel NM. Occult lymph node metastasis in radiologic stage I non-small cell lung cancer: The role of endobronchial ultrasound. Clin Respir J. (2021) 15:676–82. doi: 10.1111/crj.13344
31. Vial MR, Khan KA, O’Connell O, Peng SA, Gomez DR, Chang JY, et al. Endobronchial ultrasound-guided transbronchial needle aspiration in the nodal staging of stereotactic ablative body radiotherapy patients. Ann Thorac Surg. (2017) 103:1600–5. doi: 10.1016/j.athoracsur.2016.09.106
32. Wo Y, Li H, Zhang Y, Peng Y, Wu Z, Liu P, et al. The impact of station 4L lymph node dissection on short-term and long-term outcomes in non-small cell lung cancer. Lung Cancer. (2022) 170:141–7. doi: 10.1016/j.lungcan.2022.06.018
33. Zhao F, Zhen FX, Zhou Y, Huang CJ, Yu Y, Li J, et al. Clinicopathologic predictors of metastasis of different regional lymph nodes in patients intraoperatively diagnosed with stage-I non-small cell lung cancer. BMC Cancer. (2019) 19:444. doi: 10.1186/s12885-019-5632-2
34. Moulla Y, Gradistanac T, Wittekind C, Eichfeld U, Gockel I, Dietrich A. Predictive risk factors for lymph node metastasis in patients with resected non-small cell lung cancer: a case control study. J cardiothoracic Surg. (2019) 14:11. doi: 10.1186/s13019-019-0831-0
35. Vaghjiani RG, Takahashi Y, Eguchi T, Lu S, Kameda K, Tano Z, et al. Tumor spread through air spaces is a predictor of occult lymph node metastasis in clinical stage IA lung adenocarcinoma. J Thorac Oncol. (2020) 15:792–802. doi: 10.1016/j.jtho.2020.01.008
36. Kneuertz PJ, Cheufou DH, D’Souza DM, Mardanzai K, Abdel-Rasoul M, Theegarten D, et al. Propensity-score adjusted comparison of pathologic nodal upstaging by robotic, video-assisted thoracoscopic, and open lobectomy for non-small cell lung cancer. J Thorac Cardiovasc Surg. (2019) 158:1457–1466.e2. doi: 10.1016/j.jtcvs.2019.06.113
37. Cong M, Feng H, Ren JL, Xu Q, Cong L, Hou Z, et al. Development of a predictive radiomics model for lymph node metastases in pre-surgical CT-based stage IA non-small cell lung cancer. Lung Cancer. (2020) 139:73–9. doi: 10.1016/j.lungcan.2019.11.003
38. Zhong Y, Yuan M, Zhang T, Zhang YD, Li H, Yu TF. Radiomics approach to prediction of occult mediastinal lymph node metastasis of lung adenocarcinoma. AJR Am J Roentgenol. (2018) 211:109–13. doi: 10.2214/AJR.17.19074
39. Sanz-Santos J, Martínez-Palau M, Jaen À, Rami-Porta R, Barreiro B, Call S, et al. Geometrical Measurement of Central Tumor Location in cT1N0M0 NSCLC Predicts N1 but Not N2 Upstaging. Ann Thorac Surg. (2021) 111:1190–7. doi: 10.1016/j.athoracsur.2020.06.040
40. Nagano H, Takumi K, Nakajo M, Fukukura Y, Kumagae Y, Jinguji M, et al. Dual-energy CT-derived electron density for diagnosing metastatic mediastinal lymph nodes in non-small cell lung cancer: comparison with conventional CT and FDG PET/CT findings. AJR Am J Roentgenol. (2022) 218:66–74. doi: 10.2214/AJR.21.26208
41. Meyer HJ, Schnarkowski B, Pappisch J, Kerkhoff T, Wirtz H, Höhn AK, et al. CT texture analysis and node-RADS CT score of mediastinal lymph nodes - diagnostic performance in lung cancer patients. Cancer Imaging. (2022) 22:75. doi: 10.1186/s40644-022-00506-x
42. Shimada Y, Kudo Y, Furumoto H, Imai K, Maehara S, Tanaka T, et al. Computed tomography histogram approach to predict lymph node metastasis in patients with clinical stage IA lung cancer. Ann Thorac Surg. (2019) 108:1021–8. doi: 10.1016/j.athoracsur.2019.04.082
43. Gu Y, She Y, Xie D, Dai C, Ren Y, Fan Z, et al. A texture analysis-based prediction model for lymph node metastasis in stage IA lung adenocarcinoma. Ann Thorac Surg. (2018) 106:214–20. doi: 10.1016/j.athoracsur.2018.02.026
44. Wang X, Zhao X, Li Q, Xia W, Peng Z, Zhang R, et al. Can peritumoral radiomics increase the efficiency of the prediction for lymph node metastasis in clinical stage T1 lung adenocarcinoma on CT? Eur Radiol. (2019) 29:6049–58. doi: 10.1007/s00330-019-06084-0
45. Perez-Johnston R, Araujo-Filho JA, Connolly JG, Caso R, Whiting K, Tan KS, et al. CT-based radiogenomic analysis of clinical stage I lung adenocarcinoma with histopathologic features and oncologic outcomes. Radiology. (2022) 303:664–72. doi: 10.1148/radiol.211582
46. Huang P, Lin CT, Li Y, Tammemagi MC, Brock MV, Atkar-Khattra S, et al. Prediction of lung cancer risk at follow-up screening with low-dose CT: a training and validation study of a deep learning method. Lancet Digit Health. (2019) 1:e353–62. doi: 10.1016/S2589-7500(19)30159-1
47. Zhang Y, Yuan N, Zhang Z, Du J, Wang T, Liu B, et al. Unsupervised domain selective graph convolutional network for preoperative prediction of lymph node metastasis in gastric cancer. Med Image Anal. (2022) 79:102467. doi: 10.1016/j.media.2022.102467
48. Ma X, Xia L, Chen J, Wan W, Zhou W. Development and validation of a deep learning signature for predicting lymph node metastasis in lung adenocarcinoma: comparison with radiomics signature and clinical-semantic model. Eur Radiol. (2023) 33:1949–62. doi: 10.1007/s00330-022-09153-z
49. Zhao X, Wang X, Xia W, Zhang R, Jian J, Zhang J, et al. 3D multi-scale, multi-task, and multi-label deep learning for prediction of lymph node metastasis in T1 lung adenocarcinoma patients’ CT images. Comput Med Imaging Graph. (2021) 93:101987. doi: 10.1016/j.compmedimag.2021.101987
50. Zhao X, Wang X, Xia W, Li Q, Zhou L, Li Q, et al. A cross-modal 3D deep learning for accurate lymph node metastasis prediction in clinical stage T1 lung adenocarcinoma. Lung Cancer. (2020) 145:10–7. doi: 10.1016/j.lungcan.2020.04.014
51. Gould MK, Kuschner WG, Rydzak CE, Maclean CC, Demas AN, Shigemitsu H, et al. Test performance of positron emission tomography and computed tomography for mediastinal staging in patients with non-small-cell lung cancer: a meta-analysis. Ann Intern Med. (2003) 139:879–92. doi: 10.7326/0003-4819-139-11-200311180-00013
52. Shinya T, Rai K, Okumura Y, Fujiwara K, Matsuo K, Yonei T, et al. Dual-time-point F-18 FDG PET/CT for evaluation of intrathoracic lymph nodes in patients with non-small cell lung cancer. Clin Nucl Med. (2009) 34:216–21. doi: 10.1097/RLU.0b013e31819a1f3d
53. Lee SW, Kim SJ. Is delayed image of 18F-FDG PET/CT necessary for mediastinal lymph node staging in non-small cell lung cancer patients? Clin Nucl Med. (2022) 47:414–21. doi: 10.1097/RLU.0000000000004110
54. Endoh H, Yamamoto R, Ichikawa A, Shiozawa S, Nishizawa N, Satoh Y, et al. Clinicopathologic significance of false-positive lymph node status on FDG-PET in lung cancer. Clin Lung Cancer. (2021) 22:218–24. doi: 10.1016/j.cllc.2020.05.002
55. Kagimoto A, Tsutani Y, Izaki Y, Handa Y, Mimae T, Miyata Y, et al. Prediction of lymph node metastasis using semiquantitative evaluation of PET for lung adenocarcinoma. Ann Thorac Surg. (2020) 110:1036–42. doi: 10.1016/j.athoracsur.2020.03.032
56. Chang C, Sun X, Zhao W, Wang R, Qian X, Lei B, et al. Minor components of micropapillary and solid subtypes in lung invasive adenocarcinoma (≤ 3 cm): PET/CT findings and correlations with lymph node metastasis. Radiol Med. (2020) 125:257–64. doi: 10.1007/s11547-019-01112-x
57. Zhu Y, Cai Q, Wang Y, You N, Yip R, Lee DS, et al. Pre-surgical assessment of mediastinal lymph node metastases in patients having ≥ 30 mm non-small-cell lung cancers. Lung Cancer. (2021) 161:189–96. doi: 10.1016/j.lungcan.2021.09.013
58. Nomura K, Nakai T, Nishina Y, Sakamoto N, Miyoshi T, Tane K, et al. 18F-fluorodeoxyglucose uptake in PET is associated with the tumor microenvironment in metastatic lymph nodes and prognosis in N2 lung adenocarcinoma. Cancer Sci. (2022) 113:1488–96. doi: 10.1111/cas.v113.4
59. Ouyang ML, Tang K, Xu MM, Lin J, Li TC, Zheng XW. Prediction of occult lymph node metastasis using tumor-to-blood standardized uptake ratio and metabolic parameters in clinical N0 lung adenocarcinoma. Clin Nucl Med. (2018) 43:715–20. doi: 10.1097/RLU.0000000000002229
60. Suh YJ, Park CM, Han K, Jeon SK, Kim H, Hwang EJ, et al. Utility of FDG PET/CT for preoperative staging of non-small cell lung cancers manifesting as subsolid nodules with a solid portion of 3 cm or smaller. AJR Am J Roentgenol. (2020) 214:514–23. doi: 10.2214/AJR.19.21811
61. Bianchetti G, Taralli S, Vaccaro M, Indovina L, Mattoli MV, Capotosti A, et al. Automated detection and classification of tumor histotypes on dynamic PET imaging data through machine-learning driven voxel classification. Comput Biol Med. (2022) 145:105423. doi: 10.1016/j.compbiomed.2022.105423
62. Yin G, Song Y, Li X, Zhu L, Su Q, Dai D, et al. Prediction of mediastinal lymph node metastasis based on (18)F-FDG PET/CT imaging using support vector machine in non-small cell lung cancer. Eur Radiol. (2021) 31:3983–92. doi: 10.1007/s00330-020-07466-5
63. Rogasch JMM, Michaels L, Baumgärtner GL, Frost N, Rückert JC, Neudecker J, et al. A machine learning tool to improve prediction of mediastinal lymph node metastases in non-small cell lung cancer using routinely obtainable [(18)F]FDG-PET/CT parameters. Eur J Nucl Med Mol Imaging. (2023) 50:2140–51. doi: 10.1007/s00259-023-06145-z
64. Yoo J, Cheon M, Park YJ, Hyun SH, Zo JI, Um SW, et al. Machine learning-based diagnostic method of pre-therapeutic (18)F-FDG PET/CT for evaluating mediastinal lymph nodes in non-small cell lung cancer. Eur Radiol. (2021) 31:4184–94. doi: 10.1007/s00330-020-07523-z
65. Wang M, Liu L, Dai Q, Jin M, Huang G. Developing a primary tumor and lymph node 18F-FDG PET/CT-clinical (TLPC) model to predict lymph node metastasis of resectable T2-4 NSCLC. J Cancer Res Clin Oncol. (2023) 149:247–61. doi: 10.1007/s00432-022-04545-6
66. Mao X, Zhang Z, Zheng X, Xie F, Duan F, Jiang L, et al. Capture-based targeted ultradeep sequencing in paired tissue and plasma samples demonstrates differential subclonal ctDNA-releasing capability in advanced lung cancer. J Thorac Oncol. (2017) 12:663–72. doi: 10.1016/j.jtho.2016.11.2235
67. Zhang R, Zhang X, Huang Z, Wang F, Lin Y, Wen Y, et al. Development and validation of a preoperative noninvasive predictive model based on circular tumor DNA for lymph node metastasis in resectable non-small cell lung cancer. Transl Lung Cancer Res. (2020) 9:722–30. doi: 10.21037/tlcr-20-593
68. Li N, Wang BX, Li J, Shao Y, Li MT, Li JJ, et al. Perioperative circulating tumor DNA as a potential prognostic marker for operable stage I to IIIA non-small cell lung cancer. Cancer. (2022) 128:708–18. doi: 10.1002/cncr.v128.4
69. Abbosh C, Frankell AM, Harrison T, Kisistok J, Garnett A, Johnson L, et al. Tracking early lung cancer metastatic dissemination in TRACERx using ctDNA. Nature. (2023) 616:553–62. doi: 10.1038/s41586-023-05776-4
70. Zhou M, Wang X, Jiang L, Chen X, Bao X, Chen X. The diagnostic value of one step nucleic acid amplification (OSNA) in differentiating lymph node metastasis of tumors: A systematic review and meta-analysis. Int J Surg. (2018) 56:49–56. doi: 10.1016/j.ijsu.2018.05.010
71. Escalante Pérez M, Hermida Romero MT, Otero Alén B, Álvarez Martínez M, Fernández Prado R, de la Torre Bravos M, et al. Detection of lymph node metastasis in lung cancer patients using a one-step nucleic acid amplification assay: a single-centre prospective study. J Transl Med. (2019) 17:233. doi: 10.1186/s12967-019-1974-4
72. Li Z, Xu K, Xu L, Dai J, Jin K, Zhu Y, et al. Predictive value of folate receptor-positive circulating tumor cells for the preoperative diagnosis of lymph node metastasis in patients with lung adenocarcinoma. Small Methods. (2021) 5:e2100152. doi: 10.1002/smtd.202100152
73. Ali Z, Vildevall M, Rodriguez GV, Tandiono D, Vamvakaris I, Evangelou G, et al. Zebrafish patient-derived xenograft models predict lymph node involvement and treatment outcome in non-small cell lung cancer. J Exp Clin Cancer Res. (2022) 41:58. doi: 10.1186/s13046-022-02280-x
74. Osarogiagbon RU, Lee YS, Faris NR, Ray MA, Ojeabulu PO, Smeltzer MP. Invasive mediastinal staging for resected non-small cell lung cancer in a population-based cohort. J Thorac Cardiovasc Surg. (2019) 158:1220–1229.e2. doi: 10.1016/j.jtcvs.2019.04.068
75. Visser MPJ, van Grimbergen I, Hölters J, Barendregt WB, Vermeer LC, Vreuls W, et al. Performance insights of endobronchial ultrasonography (EBUS) and mediastinoscopy for mediastinal lymph node staging in lung cancer. Lung Cancer. (2021) 156:122–8. doi: 10.1016/j.lungcan.2021.04.003
76. Serra Mitjà P, García-Cabo B, Garcia-Olivé I, Radua J, Rami-Porta R, Esteban L, et al. EBUS-TBNA for mediastinal staging of centrally located T1N0M0 non-small cell lung cancer clinically staged with PET/CT. Respirology. (2024) 29(2):158–65. doi: 10.1111/resp.14613
77. Kim H, Choi H, Lee KH, Cho S, Park CM, Kim YT, et al. Definitions of central tumors in radiologically node-negative, early-stage lung cancer for preoperative mediastinal lymph node staging: A dual-institution, multireader study. Chest. (2022) 161:1393–406. doi: 10.1016/j.chest.2021.11.005
78. Kaseda K, Asakura K, Kazama A, Ozawa Y. Risk factors for predicting occult lymph node metastasis in patients with clinical stage I non-small cell lung cancer staged by integrated fluorodeoxyglucose positron emission tomography/computed tomography. World J Surg. (2016) 40:2976–83. doi: 10.1007/s00268-016-3652-5
79. Tan KS, Hsu M, Adusumilli PS. Pathologic node-negative lung cancer: Adequacy of lymph node yield and a tool to assess the risk of occult nodal disease. Lung Cancer. (2022) 174:60–6. doi: 10.1016/j.lungcan.2022.10.004
80. Evison M, Morris J, Martin J, Shah R, Barber PV, Booton R, et al. Nodal staging in lung cancer: a risk stratification model for lymph nodes classified as negative by EBUS-TBNA. J Thorac Oncol. (2015) 10:126–33. doi: 10.1097/JTO.0000000000000348
81. Nakajima T, Yasufuku K, Nakagawara A, Kimura H, Yoshino I. Multigene mutation analysis of metastatic lymph nodes in non-small cell lung cancer diagnosed by endobronchial ultrasound-guided transbronchial needle aspiration. Chest. (2011) 140:1319–24. doi: 10.1378/chest.10-3186
82. Folch E, Yamaguchi N, VanderLaan PA, Kocher ON, Boucher DH, Goldstein MA, et al. Adequacy of lymph node transbronchial needle aspirates using convex probe endobronchial ultrasound for multiple tumor genotyping techniques in non-small-cell lung cancer. J Thorac Oncol. (2013) 8:1438–44. doi: 10.1097/JTO.0b013e3182a471a9
83. Torii A, Oki M, Yamada A, Kogure Y, Kitagawa C, Saka H. EUS-B-FNA enhances the diagnostic yield of EBUS bronchoscope for intrathoracic lesions. Lung. (2022) 200:643–8. doi: 10.1007/s00408-022-00563-w
84. Mondoni M, Gasparini S, Varone F, Trisolini R, Mancino L, Rossi G, et al. Accuracy and predictors of success of EUS-B-FNA in the diagnosis of pulmonary Malignant lesions: A prospective multicenter italian study. Respiration. (2022) 101:775–83. doi: 10.1159/000524398
85. Korevaar DA, Crombag LM, Cohen JF, Spijker R, Bossuyt PM, Annema JT. Added value of combined endobronchial and oesophageal endosonography for mediastinal nodal staging in lung cancer: a systematic review and meta-analysis. Lancet Respir Med. (2016) 4:960–8. doi: 10.1016/S2213-2600(16)30317-4
86. Shin SH, Jeong BH, Jhun BW, Yoo H, Lee K, Kim H, et al. The utility of endosonography for mediastinal staging of non-small cell lung cancer in patients with radiological N0 disease. Lung Cancer. (2020) 139:151–6. doi: 10.1016/j.lungcan.2019.11.021
87. Rogiers A, Lobon I, Spain L, Turajlic S. The genetic evolution of metastasis. Cancer Res. (2022) 82:1849–57. doi: 10.1158/0008-5472.CAN-21-3863
88. Park S, Holmes-Tisch AJ, Cho EY, Shim YM, Kim J, Kim HS, et al. Discordance of molecular biomarkers associated with epidermal growth factor receptor pathway between primary tumors and lymph node metastasis in non-small cell lung cancer. J Thorac oncology: Off Publ Int Assoc Study Lung Cancer. (2009) 4:809–15. doi: 10.1097/JTO.0b013e3181a94af4
89. Chen Y, Mao B, Peng X, Zhou Y, Xia K, Guo H, et al. A comparative study of genetic profiles of key oncogenesis-related genes between primary lesions and matched lymph nodes metastasis in lung cancer. J Cancer. (2019) 10:1642–50. doi: 10.7150/jca.28266
90. Bailey MH, Tokheim C, Porta-Pardo E, Sengupta S, Bertrand D, Weerasinghe A, et al. Comprehensive characterization of cancer driver genes and mutations. Cell. (2018) 174:1034–5. doi: 10.1016/j.cell.2018.07.034
91. Liu Z, Liang H, Lin J, Cai X, Pan Z, Liu J, et al. The incidence of lymph node metastasis in patients with different oncogenic driver mutations among T1 non-small-cell lung cancer. Lung Cancer. (2019) 134:218–24. doi: 10.1016/j.lungcan.2019.06.026
92. Castellanos E, Feld E, Horn L. Driven by mutations: the predictive value of mutation subtype in EGFR-mutated non-small cell lung cancer. J Thorac Oncol. (2017) 12:612–23. doi: 10.1016/j.jtho.2016.12.014
93. Oxnard GR, Binder A, Jänne PA. New targetable oncogenes in non-small-cell lung cancer. J Clin Oncol. (2013) 31:1097–104. doi: 10.1200/JCO.2012.42.9829
94. Guerrera F, Renaud S, Tabbó F, Voegeli AC, Filosso PL, Legrain M, et al. Epidermal growth factor receptor mutations are linked to skip N2 lymph node metastasis in resected non-small-cell lung cancer adenocarcinomas. Eur J cardio-thoracic Surg. (2017) 51:680–8. doi: 10.1093/ejcts/ezw362
95. Wu Y, Ni H, Yang D, Niu Y, Chen K, Xu J, et al. Driver and novel genes correlated with metastasis of non-small cell lung cancer: A comprehensive analysis. Pathol Res Pract. (2021) 224:153551. doi: 10.1016/j.prp.2021.153551
96. Caso R, Connolly JG, Zhou J, Tan KS, Choi JJ, Jones GD, et al. Preoperative clinical and tumor genomic features associated with pathologic lymph node metastasis in clinical stage I and II lung adenocarcinoma. NPJ Precis Oncol. (2021) 5:70. doi: 10.1038/s41698-021-00210-2
97. Shimizu K, Yukawa T, Hirami Y, Okita R, Saisho S, Maeda A, et al. Heterogeneity of the EGFR mutation status between the primary tumor and metastatic lymph node and the sensitivity to EGFR tyrosine kinase inhibitor in non-small cell lung cancer. Targeted Oncol. (2013) 8:237–42. doi: 10.1007/s11523-012-0241-x
98. Schmid K, Oehl N, Wrba F, Pirker R, Pirker C, Filipits M. EGFR/KRAS/BRAF mutations in primary lung adenocarcinomas and corresponding locoregional lymph node metastases. Clin Cancer Res. (2009) 15:4554–60. doi: 10.1158/1078-0432.CCR-09-0089
99. Reiter JG, Makohon-Moore AP, Gerold JM, Heyde A, Attiyeh MA, Kohutek ZA, et al. Minimal functional driver gene heterogeneity among untreated metastases. Science. (2018) 361:1033–7. doi: 10.1126/science.aat7171
100. Lorber T, Andor N, Dietsche T, Perrina V, Juskevicius D, Pereira K, et al. Exploring the spatiotemporal genetic heterogeneity in metastatic lung adenocarcinoma using a nuclei flow-sorting approach. J Pathol. (2019) 247:199–213. doi: 10.1002/path.2019.247.issue-2
Keywords: clinical T1 non-small cell lung cancer, lymph node metastasis, lymph node dissection, noninvasive prediction, heterogeneity
Citation: Zhang L, Zhang F, Li G, Xiang X, Liang H and Zhang Y (2024) Predicting lymph node metastasis of clinical T1 non-small cell lung cancer: a brief review of possible methodologies and controversies. Front. Oncol. 14:1422623. doi: 10.3389/fonc.2024.1422623
Received: 24 April 2024; Accepted: 25 November 2024;
Published: 09 December 2024.
Edited by:
David Fielding, Royal Brisbane and Women’s Hospital, AustraliaReviewed by:
Song Xu, Tianjin Medical University General Hospital, ChinaCopyright © 2024 Zhang, Zhang, Li, Xiang, Liang and Zhang. This is an open-access article distributed under the terms of the Creative Commons Attribution License (CC BY). The use, distribution or reproduction in other forums is permitted, provided the original author(s) and the copyright owner(s) are credited and that the original publication in this journal is cited, in accordance with accepted academic practice. No use, distribution or reproduction is permitted which does not comply with these terms.
*Correspondence: Li Zhang, emhhbmdsaTY3QGttbXUuZWR1LmNu; Haifeng Liang, bGhmMDY3MUAxMjYuY29t; Yan Zhang, WlkwNjIwQHZpcC4xNjMuY29t
†These authors have contributed equally to this work and share first authorship
Disclaimer: All claims expressed in this article are solely those of the authors and do not necessarily represent those of their affiliated organizations, or those of the publisher, the editors and the reviewers. Any product that may be evaluated in this article or claim that may be made by its manufacturer is not guaranteed or endorsed by the publisher.
Research integrity at Frontiers
Learn more about the work of our research integrity team to safeguard the quality of each article we publish.