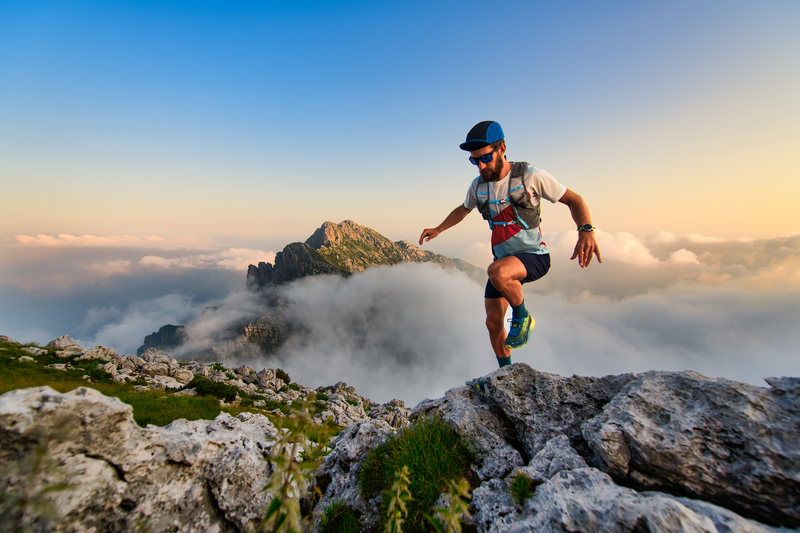
94% of researchers rate our articles as excellent or good
Learn more about the work of our research integrity team to safeguard the quality of each article we publish.
Find out more
ORIGINAL RESEARCH article
Front. Oncol. , 20 November 2024
Sec. Cancer Epidemiology and Prevention
Volume 14 - 2024 | https://doi.org/10.3389/fonc.2024.1419845
Objectives: The purpose of this study was to construct a nomogram to identify patients at high risk of gastric precancerous lesions (GPLs). This identification will facilitate early diagnosis and treatment and ultimately reduce the incidence and mortality of gastric cancer.
Methods: In this single-center retrospective cohort study, 563 participants were divided into a gastric precancerous lesion (GPL) group (n=322) and a non-atrophic gastritis (NAG) group (n=241) based on gastroscopy and pathology results. Laboratory data and demographic data were collected. A derivation cohort (n=395) was used to identify the factors associated with GPLs to develop a predictive model. Then, internal validation was performed (n=168). We used the area under the receiver operating characteristic curve (AUC) to determine the discriminative ability of the predictive model; we constructed a calibration plot to evaluate the accuracy of the predictive model; and we performed decision curve analysis (DCA) to assess the clinical practicability predictive model.
Results: Four –predictors (i.e., age, body mass index, smoking status, and –triglycerides) were included in the predictive model. The AUC values of this predictive model were 0.715 (95% CI: 0.665-0.765) and 0.717 (95% CI: 0.640-0.795) in the derivation and internal validation cohorts, respectively. These values indicated that the predictive model had good discrimination ability. The calibration plots and DCA suggested that the predictive model had good accuracy and clinical net benefit. The Hosmer–Lemeshow test results in the derivation and validation cohorts for this predictive model were 0.774 and 0.468, respectively.
Conclusion: The nomogram constructed herein demonstrated good performance in terms of predicting the risk of GPLs. This nomogram can be beneficial for the early detection of patients at high risk of GPLs, thus facilitating early treatment and ultimately reducing the incidence and mortality of gastric cancer.
Gastric cancer (GC) is a major global health concern because of its poor prognosis and high mortality. It ranks as the fifth most common malignancy in the world and the third leading cause of cancer-related death (1). The age-standardized incidence rates (ASIRs) and age-standardized mortality rates (ASMRs) of GC in the total population, different regions, and countries in Asia showed decreasing trends from 1990 to 2019 (2). In China, there were an estimated 4,824,700 new cancer cases in 2022, 358,700 of which were GC; furthermore, GC remains the fifth most common cancer and the third leading cause of cancer-related deaths in China, despite decreasing trends in the ASIR and ASMR (3). By 2050, it is estimated that there will be 279,707 cases of GC in China, including 122,796 cases of cardiac cancer and 156,911 cases of noncardiac cancer (4). Therefore, it is crucial to develop methods to reduce the global incidence and mortality rates of GC (5, 6).
GC is a multiannual, multistage, and multifactorial disease, and the development of this malignancy has been shown to involve a multistep process from normal gastric mucosa to chronic non-atrophic and atrophic gastritis, intestinal metaplasia, and epithelial dysplasia, and ultimately progressing to GC (7). Various factors can influence the development and progression of GC, including sociodemographic disparities (e.g., age and sex), dietary habits (e.g., high-fat diet and salt intake), bacterial infection (particularly Helicobacter pylori), environmental factors (e.g., geographical differences), inherited genes, and gastric precancerous lesions (GPLs) (2, 7–12). Research on anti-gastrointestinal cancer drugs in China is developing rapidly, including studies on PD-1 inhibitors, chimeric antigen receptor (CAR) T-cell therapy, antibody-coupled drugs and bispecific antibodies (13). The most promising approach for preventing GC is to target precancerous lesions (14). Gastric atrophy, intestinal metaplasia, pseudopyloric gland metaplasia, and dysplasia are classified as GPLs during the pathological progression of GC (15–17). Numerous studies have shown that patients with GPLs are at considerable risk of GC (9, 18, 19). A 20-year follow-up study was conducted on patients with GPLs and revealed that incomplete intestinal metaplasia is a significant risk factors for GC. Moreover, anti-H. Pylori therapy was been shown to exert a long-term positive effect on preventing histological progression in a population at high risk of GC (17). Anti-H. pylori therapy after endoscopic resection for gastric dysplasia (GPL) has been proven to decrease the risk of developing GC in the long term (20). Wang et al. demonstrated that targeting GPLs (specifically, intestinal metaplasia and epithelial dysplasia) can be an effective intervention for preventing the occurrence of GC (21). The above research clearly revealed that GPLs play a crucial role in the development of GC, and timely identification and early intervention of GPLs are crucial in preventing the development of this malignancy. However, the precise mechanism by which GPLs influence GCs has yet to be fully elucidated, and certain factors associated with GPLs have not yet been identified.
There are numerous predictive models that aim to identify factors associated with GC (22–24). On the basis of the identified associated factors, some studies have developed nomograms with high accuracy for predicting the recurrence of early gastric cancer after ESD (25). These models can include a wide range of factors, such as infection (26–28), metabolism (27, 29), genes and proteins (29–31), and pathological and CT images (28, 32). Gene Ontology (GO) analysis revealed that N1-methyladenosinem1A downstream genes are associated with cell proliferation and clinicopathological parameters in gastrointestinal cancers (33). Previous studies have shown that JMJD3 overexpression is correlated with genetic aberrations and DNA methylation, which are associated with shortened overall survival in patients with GC (34). Despite emerging efforts in the development of anti-gastrointestinal cancer drugs, efforts focused on GC remain insufficient (35). More exploration is needed in the treatment of GC. In recent years, there has been a steady increase in the use of predictive models for GPLs. However, different models emphasize different aspects (36–38). For example, Changzheng Ma et al. developed a machine learning model based on tongue images to screen patients with GPLs, aiming to identify and integrate valuable characteristics of noninvasive medical images related to GPLs (37). Qianyu Zhu et al. conducted a study to identify autoantibodies associated with GPLs via serological proteome analysis, nanoliter−liquid chromatography, and quadrupole time-of-flight tandem mass spectrometry to analyze the potential detection value of GPLs via an enzyme-linked immunosorbent assay (38). A nomogram can assign scores based on predictor values, calculate total scores, and estimate outcome risks in ways that traditional statistical methods cannot. As a common data visualization tool, a prediction model can visually show the relationships among multiple variables and provide us with more comprehensive data analysis results. A prediction model for GPLs could provide valuable guidance to clinicians in identifying high-risk GPL patients and planning more intensive follow-up strategies. Additionally, a prediction model could be used for health guidance, decision-making support, and lifestyle changes for the general population.
In our study, we aimed to construct a predictive model for GPLs on the basis of clinical data to facilitate early interventions for GPLs. The objectives of this study were as follows (1): to construct a nomogram that can accurately predict the probability of GPLs, and (2) to prevent the progression of GPLs to GCs by timely identification and early intervention of individual-associated factors, thereby reducing the overall probability of GC occurrence.
This single-center retrospective cohort study was conducted at the Department of Gastroenterology, The First Affiliated Hospital of Anhui Medical University (North District), from January 2019 to June 2022. To establish a stable and reliable predictive model for GPLs, our study focused on three aspects: (1) identifying the statistically significant risk factors associated with GPLs in the derivation cohort and conducting internal validation in the validation cohort; (2) evaluating the discrimination ability (ROC curve), accuracy (calibration plots), clinical practicability (DCA), and Hosmer–Lemeshow test results in both the derivation and validation cohorts; and (3) conducting a random operation using the original data (numerical variables) and proving that the predictive model also has good consistency among numerical variables. We use the 10 events per variable (EPV) method to obtain a rough estimate of the sample size needed (39). The study was conducted in accordance with the Transparent Reporting of a Multivariable Prediction Model for Individual Prognosis or Diagnosis (TRIPOD) Statement (40).
Each enrolled participant underwent gastroscopy and pathology. The clinical data were collected from three different systems: the Hospital Information System (Donghua System), the Image Storage and Communication System (PACS), and the Hospital Electronic Medical Record (HIS). The following data were collected (1). Demographic data included age, sex, body mass index (BMI, kg/m2), history of diabetes, history of hypertension, smoking habit, and alcohol use. (2) Fasting biochemical tests and other blood tests were performed to examine C-reactive protein (CRP, mg/l), triglycerides (TG, mmol/l), low-density lipoprotein-cholesterol (LDL-C, mmol/l), uric acid (UA, µmol/l), carcinoembryonic antigen (CEA, ng/ml), and carbohydrate antigen199 (CA199, U/ml). The laboratory tests were conducted within 7 days prior to gastroscopy, and the reference value ranges are shown in Table 1. (3) Gastroscopy and pathological examination were performed. Gastroscopy was conducted by a skilled gastroenterologist. The procedure began with an examination of the stomach via white light endoscopy. If any abnormal areas were detected, further investigation and sampling were performed via pigment endoscopy and magnifying endoscopy. Biopsy samples were then sent to the pathology department for standard processing, where a pathologist (junior physician) made a diagnosis. Finally, another pathologist (attending physician or higher) reviewed and aligned the diagnostic opinion to ensure accuracy. (4) Classification data were collected. The pathological findings revealed gastric atrophy, intestinal metaplasia, pseudopyloric metaplasia, and dysplasia, all of which were categorized into the GPL group, and individuals with non-atrophic gastritis were classified into the NAG group. (5) H. pylori was detected via methylene blue staining.
Categorical variables are presented as frequencies and percentages. In the Supplementary Materials, numerical variables and measurement data are expressed as the means ± standard deviations (SDs). In the univariate analysis, the chi-square test was used to compare the differences between the GPL group and the NAG group for the categorical variables and counting data. Independent samples t tests or Mann−Whitney U tests were performed to compare quantitative data, as shown in the Supplementary Materials. To select predictive factors for the model, we analyzed each variable via univariate analysis and selected variables with p < 0.2 to be entered into the multivariate binary logistic regression model. Age, BMI, smoking status, and hyperlipidemic status were included in the statistical model (p < 0.2), and based on a review of previous literature considering the impact of HP on GPL, HP was also included in the statistical analysis. Finally, statistically significant variables (p < 0.05) were selected as predictive factors for the establishment of the nomogram. The derivation cohort and internal validation cohort were randomly selected at a 7:3 ratio from the total sample. The internal validation of the GPL model was performed via the bootstrap technique (with 1000 resampling bootstraps). To further verify the reliability of the predictive model, we conducted a random operation using the original data (numerical variables) and demonstrated that the model also showed good consistency among the numerical variables. We calculated the AUC to assess the discriminative ability, constructed a calibration plot to evaluate the accuracy of the predictive model, and utilized DCA to assess the clinical practicability. The evaluation of the GPL model’s performance was conducted on both the derivation and validation cohorts. All the statistical analyses were performed via R software version 4.1.2, and p<0.05 (two-tailed) was considered statistically significant.
A total of 563 participants, comprising 335 males (59.5%) and 228 females (40.5%), were included in this study. A total of 395 participants were included in the derivation cohort, and the validation cohort included 168 participants recruited from January 2019 to June 2022. Both the derivation cohort and the validation cohort consisted of patients with NAGs (167 and 74, respectively) and GPLs (228 and 94, respectively). No significant differences in clinical baseline demographic characteristics were observed between the derivation cohort and the validation cohort, as shown in Table 1.
In the derivation cohort, 6 clinical indicators were statistically significant in the univariate analysis: age (45-64 years: p=0.045; 65+ years: p=0.009), BMI (overweight and obese: all, p<0.001), history of diabetes (p=0.026), smoking (p=0.005), and TG (p<0.001) (Table 2). These indicators were then entered into a multivariate logistic regression model. Additionally, the p value for the HP comparison between the GPL group and the NAG group in the derivation cohort was 0.257, which was close to 0.20. Because HP is a crucial factor in clinical disease, it was also included in the multivariate logistic regression model.
Table 2. Univariate and multivariate logistic regression analysis of risk factors for GPL based on the derivation cohort.
Multivariate logistic regression analysis revealed that age, BMI, and TG were independent predictors of GPL risk. Adding the smoking variable to the regression model improved the overall fit of the model, resulting in an increased NRI value of 0.130. Finally, four valuable risk factors (age, BMI, smoking, and TG) were selected as predictors to establish the predictive model (Table 2), and the Hosmer–Lemeshow test result in the derivation cohort for the predictive model was favorable (p=0.774).
A nomogram was constructed based on the multivariate logistic regression model (Figure 1). To provide more options for clinical use, we listed the formula for the predictive model here: logit(P) =0.749*age(45-64 years old)(or 1.063*age (65 + years old)) + 0.718*BMI(overweight)(or 2.865*BMI (obese)) + 0.495*smoking + 0.923*TC - 1.253; P = 1/[1+e−(0.749*age(45-64 years old)(or 1.063*age(65+ years old)) + 0.718*BMI (overweight)(or 2.865*BMI (obese)) + 0.495*smoking + 0.923*TC - 1.253)].
Figure 1. Nomogram model for the risk prediction of GPL. Age, BMI, smoking and TG. Each categorical variable was scored on a scale of 0–100, and the total score for predicting GPL was obtained by summing up the scores corresponding to each categorical variable. Different total score corresponded to different probability of categorical.
ROC curve analysis revealed that the AUC of this predictive model in the derivation cohort was 0.715 (95% CI: 0.665-0.765). The maximum YDI was 0.649, and the corresponding cutoff value was 0.564, demonstrating good discrimination of the predictive model (Figure 2A).
Figure 2. ROC curves, calibration plots, DCA, and DeLong's test of the prediction model in the derivation cohort and validation cohort. (A, E), (B, F), (C, G) represent the ROC curve, calibration curve, and DCA in the derivation and validation cohort, respectively. (D) represent DeLong's test for ROC curves between the derivation and validation cohorts; Calibration plots showed ideal (100% agreement) curves, the apparent (actual) and bias-corrected (adjusted) (with 1000 resampling bootstrap). The y-axis represents the actual probability of the GPL model, and the x-axis represents the predicted probability of the GPL model. Clinical decision curve: the y-axis represents the net benefit of GPL, while the x-axis represents threshold probability of GPL. The green line stands for the net benefit for the predict-none-patients as GPL, the red line stands for the net benefit for the predict-all-patients as GPL, and the blue line represents net benefit for the GPL model.
The calibration curve showed good concordance between the actual observations and the nomogram-predicted probabilities in the derivation cohort (Figure 2B). The decision curve of this predictive model revealed that this nomogram was effective in clinical practice (Figure 2C).
The bias-corrected AUC derived from internal validation via the bootstrap method was 0.717 (95% CI: 0.640–0.795). The maximum YDI was 0.723, and the corresponding cutoff value was 0.564, similar to the AUC (0.715, 95% CI: 0.665-0.765) calculated in the derivation cohort (Figures 2A, E). The Hosmer–Lemeshow test results in the validation cohort for the predictive model were good (p=0.468). The calibration plot demonstrated good consistency between the actual and nomogram predictions in the internal validation cohort (Figure 2F). The DCA outperformed both extreme lines across a wide range of threshold probabilities, which also demonstrated the good performance of the nomogram in clinical practice in internal validation (Figure 2G). DeLong’s test for ROC curves between the derivation and validation cohorts revealed no significant difference (p = 0.960) (Figure 2D).
To further verify the reliability of the predictive model, we conducted a random operation using the original data (numerical variables) and proved that the model also has good consistency among the numerical variables. In the new derivation and validation, ROC curve analysis revealed that the AUCs of this predictive model were 0.711 (95% CI: 0.661-0.762) and 0.701 (95% CI: 0.622-0.780), respectively. The Hosmer–Lemeshow test results for the new derivation and validation were good (p=0.111, p=0.220). For more details, please refer to the Supplementary Materials.
The calibration plots revealed ideal (100% agreement) curves and apparent (actual) and bias-corrected (adjusted) (1000 resamples) curves. The y-axis represents the actual probability of the GPL model, and the x-axis represents the predicted probability of the GPL model. Clinical decision curve: the y-axis represents the net benefit of the GPL, whereas the x-axis represents the threshold probability of the GPL. The green line represents the net benefit for the predict-no-patients as GPL, the red line represents the net benefit for the predict-all-patients as GPL, and the blue line represents the net benefit for the GPL model.
Numerous studies have confirmed the close relationship between GPLs and GC from various perspectives (41, 42), and patients with GPLs are at considerable risk of GC (9, 18, 19). Gastric atrophy, intestinal metaplasia, pseudopyloric gland metaplasia, and dysplasia are generally recognized as GPLs, and targeting GPLs can be an effective intervention to prevent the occurrence of GC (20, 21). Therefore, developing a simple and convenient predictive model for GPLs, rather than relying on invasive gastroscopy procedures, can aid in early intervention for managing GPLs and effectively reduce the incidence of GC. In this study, we developed and validated a nomogram to preliminarily predict the risk of GPLs. The predictive model includes four predictors: age, BMI, smoking status, and TG.
Age is a significant risk factor for the development of both cancerous and precancerous conditions (14, 37, 43–46). Hai-Fan Xiao et al. conducted a study on risk factors for upper GPLs in non-high-incidence areas and reported that age is the most significant contributor to the risk of developing GPLs (47). A follow-up study of 16,764 French patients who underwent upper endoscopy with gastric biopsies revealed that the severity of precancerous lesions tends to increase with age (48). According to a study conducted by Romańczyk M et al., individuals who are 40 years or older are more likely to be diagnosed with precancerous conditions than patients younger than 40. Furthermore, the risk of dysplasia begins to increase at age 55, whereas the risk of cancer increases at age 60 (43). The prevalence rate of chronic atrophic gastritis is relatively high in China and tends to increase with age (49). A large population-based study in China (27,094 participants) revealed that age was an essential sociodemographic risk factor for GC and GPL and that age was associated with all GPLs, with relative risks ranging from 1.01 to 1.13 (12). In the present study, the age of the GPL group was greater than that of the NAG group in both the derivation and validation cohorts. Among the 322 GPL patients in our study, 180 (55.9%) were between 45 and 64 years old, and 117 (36.3%) were over 65 years old. These proportions align with the findings of the previous study, indicating the need for increased focus on GPL screening for individuals over 45 years old.
Among the various habits that play a role in GPL development, the impact of smoking has been considered (50). Smoking is known to be associated with a greater risk of recurrence and death from GC (51, 52), and this association also seems to be evident in GPLs (16, 45). Smoking can increase gastric secretion and has been linked to increased levels of plasma pepsinogen I, which is a marker for GPLs (53). Our findings revealed that smokers are more likely to have GPLs (OR=1.640), which is consistent with the findings of previous studies (16, 53). Studies have shown that smoking is strongly linked to a greater risk of developing GPLs in China, particularly among Han Chinese individuals. The risk was significantly greater for individuals who smoked fewer than 10 cigarettes per day (OR = 5.24), between 11 and 20 cigarettes per day (OR = 8.19), and those who smoked 21 or more cigarettes per day (OR = 7.07) (16). Early research conducted in rural China has also demonstrated a clear link between smoking and the development of GPLs. Moreover, this association becomes more pronounced with greater daily consumption and a longer duration of smoking (14). A microsimulation model suggested that the relative decline in cancer incidence was accelerated by 7% due to lower rates of smoking initiation and higher rates of smoking cessation observed after the 1960s (54). Currently, implementing tobacco control measures among individuals with GPLs is widely recognized as crucial (16), and these measures play a significant role in preventing the onset and progression of GPLs.
Many studies have confirmed that TG is a risk factor for GPLs, and this predictor is significantly more common in overweight and obese people (55). Overweight and obese individuals often have higher levels of TG (56, 57), which is also known to be a risk factor for GPL and GC and is associated with alterations in the gut microbiota (55). Li Tian et al. demonstrated that obesity promotes diethylnitrosamine-induced precancerous lesions by inducing M1 macrophage polarization and angiogenesis in mice, which may involve obesity and inflammation (58). Our study revealed that the risk of GPLs was 2.020 times greater in overweight individuals and 17.551 times greater in obese individuals than in thin or normal individuals. Additionally, individuals with high TG levels have a 2.516-fold greater risk of GPLs than do individuals with normal TG levels. Combined with our previous findings, we speculate that overweight and obese people have higher TG levels, which may be closely related to their diet.
Many studies have reported strong correlations between TG, BMI, and dietary factors (59), and these findings are also supported by studies on GC, GPL, and dietary factors (14, 44, 52, 59). Seiya Arita M.S. et al. reported that a high-fat diet could cause severe microbiota disorders in the stomachs of mice. Changes in the microbiome are accompanied by increased gastric leptin, leading to intestinal metaplasia. This process involves the gastric leptin signaling pathway regulating the microflora of the gastric mucosa (55), and some domestic studies also support the above results (14, 47). Other dietary studies have suggested that consuming fruits and vegetables may effectively reduce the risk of GC, and adhering to a healthy plant-based diet was also found to be negatively correlated with the occurrence of GPLs. Higher consumption of healthy plant-based foods, such as vegetables, fruits, whole grains, and algae, is associated with increased intake of vitamins, dietary fiber, and phytochemicals. These nutrients contribute to the prevention of gastric mucosal lesions by providing anti-inflammatory and antioxidant benefits (59). Therefore, prevention of GPLs can potentially be achieved through dietary intervention. This involves increasing the consumption of fresh fruits and vegetables while limiting an unhealthy plant-based diet. Additionally, making lifestyle changes such as engaging in more regular exercise can also help reduce the risk of developing GPLs (16, 47, 52). In future screening strategies, populations that are overweight, obese, or on a high-fat diet should be identified as important groups for screening GPLs. Health guidance should be provided to individuals who have a higher recommended frequency of gastrointestinal examinations than that of the general population.
Studies have shown that H. pylori infection causes chronic active gastritis, which can progress to atrophic gastritis, intestinal metaplasia, and GPL (low-grade and high-grade dysplasia), ultimately resulting in the development of GC (60–62). H. pylori infection is prevalent and is a significant contributor to the occurrence of GC (63). A population-based cohort study involving 69,722 patients with gastric dysplasia revealed that H. pylori treatment was associated with a reduced risk of developing GC. In particular, this treatment has a significant protective effect against the development of late-onset GC (20). Many studies have also confirmed the relationship between H. pylori and GPLs (64–66). H. pylori cytotoxin-associated gene A status is crucial for detecting GPLs (65). However, the predictive model of this study did not reveal any relationship between H. pylori and GPLs, which may be attributed to the following. 1) In our study, the subjects were divided into two groups on the basis of pathological findings: the NAG group and the GPL group. During clinical examinations, many individuals do not undergo pathological examinations due to normal findings under gastroscopy. This resulted in a greater number of individuals in the GPL group than in the NAG group, surpassing the figures reported in other studies (48). 2) Smoking has been suggested to possibly decrease the risk of H. pylori infection. This theory is based on the assumption that smoking could increase acid production and pepsin secretion, thereby providing some level of protection for the gastric mucosa against H. pylori infection (16). Notably, this study did not find any evidence of H. pylori infection, which could be explained by this assumption.
In our study, we performed internal validation to assess the performance of the prediction model in terms of discrimination, calibration, and clinical applicability. The results showed that the model performed effectively. Moreover, this prediction model only includes four easily obtainable predictors in clinical practice, making it highly manageable. The aim of constructing this prediction model is to identify individuals who are at risk of developing GPLs and encourage them to undergo early gastroscopy for timely detection and intervention.
The limitations in this study should be considered when interpreting the results. First, this was a single-center retrospective study, and the data collected may have potential bias related to changes in sample size and structure, thus explaining the lack of positive findings for certain important indicators, such as H. pylori. Second, because this study was retrospective, it was not possible to include all relevant measures mentioned in the references. As a result, some clinically significant measures may have been overlooked. Therefore, future research should focus on revising the prediction model. Third, owing to sample size limitations, we were unable to classify patients with GPLs in greater detail, which hindered our ability to identify high-risk patients more accurately. Finally, conducting a multicenter, randomized controlled and large-sample validation study based on the model is crucial and may contribute to uncovering new findings. This helps improve the accuracy of the model and its applicability.
This study developed a preliminary nomogram that is reliable and accurate with respect to predicting the risk of GPLs. The model can help with the early detection of patients at high risk for GPLs and reduce the incidence and mortality of gastric cancer by actively intervening in patients with GPLs. In the future, it will be crucial to include more GPL-related factors in the statistical analysis to improve the model. Furthermore, the model should be externally validated in larger sample sizes from multiple centers to further enhance its effectiveness.
The original contributions presented in the study are included in the article/Supplementary Material. Further inquiries can be directed to the corresponding authors.
The studies involving humans were approved by The Ethics Committee of The First Affiliated Hospital of Anhui Medical University North District. The studies were conducted in accordance with the local legislation and institutional requirements. The ethics committee/institutional review board waived the requirement of written informed consent for participation from the participants or the participants’ legal guardians/next of kin because this study was a retrospective cohort study, it was not possible to obtain informed consent from patients. To ensure the confidentiality of patient data collected during the investigation, clinical data were handled anonymously.
CS: Conceptualization, Formal analysis, Investigation, Validation, and Writing – original draft. RT: Data curation, Methodology, Software, Formal analysis, Visualization, and Writing – review & editing. WW: Methodology and Writing – review & editing. ZD: Formal analysis and Resources. JT: Methodology. XY: Software and Methodology. GX: Software and Investigation. HL and XC: Study design, Supervision, Project administration, Investigation, and Funding acquisition.
The author(s) declare that no financial support was received for the research, authorship, and/or publication of this article.
The authors declare that the research was conducted in the absence of any commercial or financial relationships that could be construed as a potential conflict of interest.
All claims expressed in this article are solely those of the authors and do not necessarily represent those of their affiliated organizations, or those of the publisher, the editors and the reviewers. Any product that may be evaluated in this article, or claim that may be made by its manufacturer, is not guaranteed or endorsed by the publisher.
The Supplementary Material for this article can be found online at: https://www.frontiersin.org/articles/10.3389/fonc.2024.1419845/full#supplementary-material
1. Siegel RL, Miller KD, Wagle NS, Jemal A. Cancer statistics, 2023. CA Cancer J Clin. (2023) 73:17–48. doi: 10.3322/caac.21763
2. Zhang Y, Li P, Han J, Liu Y, Liu J, Li M, et al. Trends in gastric cancer incidence and mortality in Asia and association analysis with human development index, 1990-2019. Cancer Epidemiol. (2024) 88:102517. doi: 10.1016/j.canep.2023.102517
3. Han B, Zheng R, Zeng H, Wang S, Sun K, Chen R, et al. Cancer incidence and mortality in China, 2022. J Natl Cancer Cent. (2024) 4(1):47–53. doi: 10.1016/j.jncc.2024.01.006
4. He F, Wang S, Zheng R, Gu J, Zeng H, Sun K, et al. Trends of gastric cancer burdens attributable to risk factors in China from 2000 to 2050. Lancet Reg Health West Pac. (2024) 44:101003. doi: 10.1016/j.lanwpc.2023.101003
5. Huang B, Ding F, Liu J, Li Y. Government drivers of gastric cancer prevention: The identification of risk areas and macro factors in Gansu, China. Prev Med Rep. (2023) 36:102450. doi: 10.1016/j.pmedr.2023.102450
6. Sung H, Ferlay J, Siegel RL, Laversanne M, Soerjomataram I, Jemal A, et al. Global cancer statistics 2020: GLOBOCAN estimates of incidence and mortality worldwide for 36 cancers in 185 countries. CA Cancer J Clin. (2021) 71:209–49. doi: 10.3322/caac.21660
7. Yakirevich E, Resnick MB. Pathology of gastric cancer and its precursor lesions. Gastroenterol Clin North Am. (2013) 42:261–84. doi: 10.1016/j.gtc.2013.01.004
8. Zhou C, Bisseling TM, van der Post RS, Boleij A. The influence of Helicobacter pylori, proton pump inhibitor, and obesity on the gastric microbiome in relation to gastric cancer development. Comput Struct Biotechnol J. (2023) 23:186–98. doi: 10.1016/j.csbj.2023.11.053
9. de Vries AC, van Grieken NC, Looman CW, Casparie MK, de Vries E, Meijer GA, et al. Gastric cancer risk in patients with premalignant gastric lesions: a nationwide cohort study in the Netherlands. Gastroenterology. (2008) 134:945–52. doi: 10.1053/j.gastro.2008.01.071
10. Zhao B, Wu W, Liang L, Cai X, Chen Y, Tang W. Prediction model of clinical prognosis and immunotherapy efficacy of gastric cancer based on level of expression of cuproptosis-related genes. Heliyon. (2023) 9:e19035. doi: 10.1016/j.heliyon.2023.e19035
11. Fan H, Li X, Li ZW, Zheng NR, Cao LH, Liu ZC, et al. Urine proteomic signatures predicting the progression from premalignancy to Malignant gastric cancer. EBioMedicine. (2022) 86:104340. doi: 10.1016/j.ebiom.2022.104340
12. Sun D, Lei L, Xia C, Li H, Cao M, He S, et al. Sociodemographic disparities in gastric cancer and the gastric precancerous cascade: A population-based study. Lancet Reg Health West Pac. (2022) 23:100437. doi: 10.1016/j.lanwpc.2022.100437
13. Ren C, Xu RH. The drug treatment research of gastrointestinal cancer in China. Eur J Surg Oncol. (2020) 46:e3–6. doi: 10.1016/j.ejso.2020.06.004
14. Liu J, Sun LP, Gong YH, Yuan Y. Risk factors of precancerous gastric lesions in a population at high risk of gastric cancer. Chin J Cancer Res. (2010) 22:267–73. doi: 10.1007/s11670-010-0267-5
15. Cao Y, Wang D, Mo G, Peng Y, Li Z. Gastric precancerous lesions:occurrence, development factors, and treatment. Front Oncol. (2023) 13:1226652. doi: 10.3389/fonc.2023.1226652
16. Wang W, Qiao L, Dong W, Ren J, Chang X, Zhan S, et al. Differences in the association between modifiable lifestyle factors and gastric precancerous lesions among Mongolians and han chinese. Front Oncol. (2022) 12:798829. doi: 10.3389/fonc.2022.798829
17. Piazuelo MB, Bravo LE, Mera RM, Camargo MC, Bravo JC, Delgado AG, et al. The Colombian chemoprevention trial: 20-year follow-up of a cohort of patients with gastric precancerous lesions. Gastroenterology. (2021) 160:1106–1117.e3. doi: 10.1053/j.gastro.2020.11.017
18. Wang J, Zhang B, Wang Y, Zhou C, Vonsky MS, Mitrofanova LB, et al. CrossU-Net: Dual-modality cross-attention U-Net for segmentation of precancerous lesions in gastric cancer. Comput Med Imaging Graph. (2024) 112:102339. doi: 10.1016/j.compmedimag.2024.102339
19. You WC, Zhao L, Chang YS, Blot WJ, Fraumeni JF Jr. Progression of precancerous gastric lesions. Lancet. (1995) 345:866–7. doi: 10.1016/s0140-6736(95)93006-x
20. Yoo HW, Hong SJ, Kim SH. Helicobacter pylori treatment and gastric cancer risk after endoscopic resection of dysplasia: A nationwide cohort study. Gastroenterology. (2024) 166:313–322.e3. doi: 10.1053/j.gastro.2023.10.013
21. Wang Z, Li P, Li C, Guo Y, Shao Y, Yan Q, et al. Study on the mechanism of codonopsis pilosula polysaccharide inhibiting gastric cancer precancerous lesions by regulating wnt/β-catenin signaling pathway. Pharmacol Res - Modern Chin Med. (2024) 10:100391. doi: 10.1016/j.prmcm.2024.100391
22. Park KB, Jun KH, Song KY, Chin H, Lee HH. Development of a staging system and survival prediction model for advanced gastric cancer patients without adjuvant treatment after curative gastrectomy: A retrospective multicenter cohort study. Int J Surg. (2022) 101:106629. doi: 10.1016/j.ijsu.2022.106629
23. Dong Z, Zhang Y, Geng H, Ni B, Xia X, Zhu C, et al. Development and validation of two nomograms for predicting overall survival and cancer-specific survival in gastric cancer patients with liver metastases: A retrospective cohort study from SEER database. Transl Oncol. (2022) 24:101480. doi: 10.1016/j.tranon.2022.101480
24. Qin S, Wang X, Li S, Wu M, Wan X. Personalizing age of gastric cancer screening based on comorbidity in China: Model estimates of benefits, affordability and cost-effectiveness optimization. Prev Med. (2024) 179:107851. doi: 10.1016/j.ypmed.2024.107851
25. Li S, Nahar A, Zhang Q, Xing J, Li P, Zhang S, et al. Risk factors and a nomogram for predicting local recurrence in adult patients with early gastric cancer after endoscopic submucosal dissection. Dig Liver Dis. (2024) 56(11):1921–9. doi: 10.1016/j.dld.2024.04.004
26. Muti HS, Heij LR, Keller G, Kohlruss M, Langer R, Dislich B, et al. Development and validation of deep learning classifiers to detect Epstein-Barr virus and microsatellite instability status in gastric cancer: a retrospective multicenter cohort study. Lancet Digit Health. (2021) 3:e654–64. doi: 10.1016/S2589-7500(21)00133-3
27. Chen QY, Que SJ, Chen JY, Qing-Zhong, Liu ZY, Wang JB, et al. Development and validation of metabolic scoring to individually predict prognosis and monitor recurrence early in gastric cancer: A large-sample analysis. Eur J Surg Oncol. (2022) 48:2149–58. doi: 10.1016/j.ejso.2022.06.019
28. Zhao H, Gao J, Bai B, Wang R, Yu J, Lu H, et al. Development and external validation of a non-invasive imaging biomarker to estimate the microsatellite instability status of gastric cancer and its prognostic value: The combination of clinical and quantitative CT-imaging features. Eur J Radiol. (2023) 162:110719. doi: 10.1016/j.ejrad.2023.110719
29. Wang SY, Wang YX, Shen A, Jian R, An N, Yuan SQ. Construction and validation of a prognostic prediction model for gastric cancer using a series of genes related to lactate metabolism. Heliyon. (2023) 9:e16157. doi: 10.1016/j.heliyon.2023.e16157
30. Zhang H, Li M, Kaboli P, Ji H, Du F, Wu X, et al. Identification of cluster of differentiation molecule-associated microRNAs as potential therapeutic targets for gastrointestinal cancer immunotherapy. Int J Biol Markers. (2021) 36:22–32. doi: 10.1177/17246008211005473
31. Su T, Wang T, Zhang NS, Shen Y, Li WW, Xing HX, et al. Long non-coding RNAs in gastrointestinal cancers: Implications for protein phosphorylation. Biochem Pharmacol. (2022) 197:114907. doi: 10.1016/j.bcp.2022.114907
32. Wang Z, Wang Q, Wu J, Ma M, Pei Z, Sun Y, et al. An ensemble belief rule base model for pathologic complete response prediction in gastric cancer. Expert Syst Appl. (2023) 233:120976. doi: 10.1016/j.eswa.2023.120976
33. Zhao Y, Zhao Q, Kaboli PJ, Shen J, Li M, Wu X, et al. m1A regulated genes modulate PI3K/AKT/mTOR and erbB pathways in gastrointestinal cancer. Transl Oncol. (2019) 12:1323–33. doi: 10.1016/j.tranon.2019.06.007
34. Xu ZY, Xia YB, Xiao ZG, Jia YL, Li L, Jin Y, et al. Comprehensive profiling of JMJD3 in gastric cancer and its influence on patient survival. Sci Rep. (2019) 9:868. doi: 10.1038/s41598-018-37340-w
35. Shen L. Anticancer drug R&D of gastrointestinal cancer in China: Current landscape and challenges. Innovation (Camb). (2022) 3:100249. doi: 10.1016/j.xinn.2022.100249
36. Zhang Y, Wang Y, Zhang B, Li Q. A hyperspectral dataset of precancerous lesions in gastric cancer and benchmarks for pathological diagnosis. J Biophotonics. (2022) 15:e202200163. doi: 10.1002/jbio.202200163
37. Ma C, Zhang P, Du S, Li Y, Li S. Construction of tongue image-based machine learning model for screening patients with gastric precancerous lesions. J Pers Med. (2023) 13:271. doi: 10.3390/jpm13020271
38. Zhu Q, He P, Zheng C, Chen Z, Qi S, Zhou D, et al. Identification and evaluation of novel serum autoantibody biomarkers for early diagnosis of gastric cancer and precancerous lesion. J Cancer Res Clin Oncol. (2023) 149:8369–78. doi: 10.1007/s00432-023-04732-z
39. Vittinghoff E, McCulloch CE. Relaxing the rule of ten events per variable in logistic and Cox regression. Am J Epidemiol. (2007) 165:710–8. doi: 10.1093/aje/kwk052
40. Collins GS, Reitsma JB, Altman DG, Moons KG. Transparent reporting of a multivariable prediction model for individual prognosis or diagnosis (TRIPOD): the TRIPOD statement. BMJ. (2015) 350:g7594. doi: 10.1136/bmj.g7594
41. Li X, Zheng NR, Wang LH, Li ZW, Liu ZC, Fan H, et al. Proteomic profiling identifies signatures associated with progression of precancerous gastric lesions and risk of early gastric cancer. EBioMedicine. (2021) 74:103714. doi: 10.1016/j.ebiom.2021.103714
42. Flores-Luna L, Bravo MM, Kasamatsu E, Lazcano Ponce EC, Martinez T, Torres J, et al. Risk factors for gastric precancerous and cancers lesions in Latin American counties with difference gastric cancer risk. Cancer Epidemiol. (2020) 64:101630. doi: 10.1016/j.canep.2019.101630
43. Romańczyk M, Ostrowski B, Barański K, Romańczyk T, Błaszczyńska M, Budzyń K, et al. Potential benefits of one-time gastroscopy in search for precancerous conditions. Pol Arch Intern Med. (2023) 133:16401. doi: 10.20452/pamw.16401
44. Zhang LY, Zhang J, Li D, Liu Y, Zhang DL, Liu CF, et al. Bile reflux is an independent risk factor for precancerous gastric lesions and gastric cancer: An observational cross-sectional study. J Dig Dis. (2021) 22:282–90. doi: 10.1111/1751-2980.12986
45. Huang Y, Li H, Long X, Liang X, Lu H. Lessons learned from upper gastrointestinal endoscopy in asymptomatic Chinese. Helicobacter. (2021) 26:e12803. doi: 10.1111/hel.12803
46. Yu Y, Fang C, Peng C, Shen S, Xu G, Sun Q, et al. Risk factors for gastric intraepithelial neoplasia in Chinese adults: a case-control study. Cancer Manag Res. (2018) 10:2605–13. doi: 10.2147/CMAR.S166472
47. Xiao HF, Yan SP, Li JG, Shi ZH, Zou YH, Xu KK, et al. Development and external validation of a nomogram to predict the risk of Upper gastrointestinal precancerous lesions in a non-high-incidence area. Cancer Med. (2020) 9:8722–32. doi: 10.1002/cam4.3462
48. Chapelle N, Péron M, Mosnier JF, Quénéhervé L, Coron E, Bourget A, et al. Prevalence, characteristics and endoscopic management of gastric premalignant lesions in France. Dig Dis. (2020) 38:286–92. doi: 10.1159/000503748
49. Wang P, Li P, Chen Y, Li L, Lu Y, Zhou W, et al. Chinese integrated guideline on the management of gastric precancerous conditions and lesions. Chin Med. (2022) 17:138. doi: 10.1186/s13020-022-00677-6
50. Peleteiro B, Lunet N, Figueiredo C, Carneiro F, David L, Barros H. Smoking, Helicobacter pylori virulence, and type of intestinal metaplasia in Portuguese males. Cancer Epidemiol Biomarkers Prev. (2007) 16:322–6. doi: 10.1158/1055-9965.EPI-06-0885
51. Smyth EC, Capanu M, Janjigian YY, Kelsen DK, Coit D, Strong VE, et al. Tobacco use is associated with increased recurrence and death from gastric cancer. Ann Surg Oncol. (2012) 19:2088–94. doi: 10.1245/s10434-012-2230-9
52. Machlowska J, Baj J, Sitarz M, Maciejewski R, Sitarz R. Gastric cancer: epidemiology, risk factors, classification, genomic characteristics and treatment strategies. Int J Mol Sci. (2020) 21:4012. doi: 10.3390/ijms21114012
53. Razuka-Ebela D, Polaka I, Daugule I, Parshutin S, Santare D, Ebela I, et al. Factors associated with false negative results in serum pepsinogen testing for precancerous gastric lesions in a european population in the GISTAR study. Diagnostics (Basel). (2022) 12:1166. doi: 10.3390/diagnostics12051166
54. Yeh JM, Hur C, Schrag D, Kuntz KM, Ezzati M, Stout N, et al. Contribution of H. pylori and smoking trends to US incidence of intestinal-type noncardia gastric adenocarcinoma: a microsimulation model. PloS Med. (2013) 10:e1001451. doi: 10.1371/journal.pmed.1001451
55. Arita S, Inagaki-Ohara K. High-fat-diet-induced modulations of leptin signaling and gastric microbiota drive precancerous lesions in the stomach. Nutrition. (2019) 67-68:110556. doi: 10.1016/j.nut.2019.110556
56. Mikkelsen M, Wilsgaard T, Grimsgaard S, Hopstock LA, Hansson P. Associations between postprandial triglyceride concentrations and sex, age, and body mass index: cross-sectional analyses from the Tromsø study 2015-2016. Front Nutr. (2023) 10:1158383. doi: 10.3389/fnut.2023.1158383
57. Xing J, Guan X, Zhang Q, Chen S, Wu S, Sun X. Triglycerides mediate body mass index and nonalcoholic fatty liver disease: A population-based study. Obes Facts. (2021) 14:190–6. doi: 10.1159/000514848
58. Tian L, Zuoqin D, Jiaqi W, Xiaomeng J, Xin D, Yan Y, et al. Obesity phenotype induced by high-fat diet promotes diethylnitrosamine (DEN)-induced precancerous lesions by M1 macrophage polarization in mice liver. J Nutr Biochem. (2024) 125:109566. doi: 10.1016/j.jnutbio.2023.109566
59. Cheng W, Lin X, Wang T, Zhang X, Xu F, Wang L, et al. Healthy plant-based diet might be inversely associated with gastric precancerous lesions: new evidence from a case-control study based on dietary pattern and fecal metabolic profiling. Int J Food Sci Nutr. (2024) 75:102–18. doi: 10.1080/09637486.2023.2279916
60. Correa P. Human gastric carcinogenesis: a multistep and multifactorial process–First American Cancer Society Award Lecture on Cancer Epidemiology and Prevention. Cancer Res. (1992) 52:6735–40. doi: 10.12259/j.issn.2095-610X.S20230218
61. Ratana-Amornpin S, Pornthisarn B, Chonprasertsuk S, Siramolpiwat S, Bhanthumkomol P, Nunanan P, et al. Asge stomach and small intestine ii prevalence of gastric cancer and gastric precancerous lesions related to infection: A real-world evidence-access from tertiary care center study (pgc-hp trial). Gastrointest Endosc. (2023) 97:Ab1230–Ab. doi: 10.1016/j.gie.2023.04.1860
62. Sayed IM, Sahan AZ, Venkova T, Chakraborty A, Mukhopadhyay D, Bimczok D, et al. Helicobacter pylori infection downregulates the DNA glycosylase NEIL2, resulting in increased genome damage and inflammation in gastric epithelial cells. J Biol Chem. (2020) 295:11082–98. doi: 10.1074/jbc.RA119.009981
63. Yang L, Kartsonaki C, Yao P, de Martel C, Plummer M, Chapman D, et al. The relative and attributable risks of cardia and non-cardia gastric cancer associated with Helicobacter pylori infection in China: a case-cohort study. Lancet Public Health. (2021) 6:e888–96. doi: 10.1016/S2468-2667(21)00164-X
64. Garay J, Piazuelo MB, Majumdar S, Li L, Trillo-Tinoco J, Del Valle L, et al. The homing receptor CD44 is involved in the progression of precancerous gastric lesions in patients infected with Helicobacter pylori and in development of mucous metaplasia in mice. Cancer Lett. (2016) 371:90–8. doi: 10.1016/j.canlet.2015.10.037
65. Yakut M, Örmeci N, Erdal H, Keskin O, Karayel Z, Tutkak H, et al. The association between precancerous gastric lesions and serum pepsinogens, serum gastrin, vascular endothelial growth factor, serum interleukin-1 Beta, serum toll-like receptor-4 levels and Helicobacter pylori Cag A status. Clin Res Hepatol Gastroenterol. (2013) 37:302–11. doi: 10.1016/j.clinre.2012.09.013
Keywords: age, body mass index, smoking, triglyceride, associated factors, prediction model, gastric precancerous lesions
Citation: Shi C, Tao R, Wang W, Tang J, Dou Z, Yuan X, Xu G, Liu H and Chen X (2024) Development and validation of a nomogram for obesity and related factors to detect gastric precancerous lesions in the Chinese population: a retrospective cohort study. Front. Oncol. 14:1419845. doi: 10.3389/fonc.2024.1419845
Received: 04 May 2024; Accepted: 30 October 2024;
Published: 20 November 2024.
Edited by:
Alireza Sadjadi, Tehran University of Medical Sciences, IranReviewed by:
Tao Yi, Hong Kong Baptist University, Hong Kong SAR, ChinaCopyright © 2024 Shi, Tao, Wang, Tang, Dou, Yuan, Xu, Liu and Chen. This is an open-access article distributed under the terms of the Creative Commons Attribution License (CC BY). The use, distribution or reproduction in other forums is permitted, provided the original author(s) and the copyright owner(s) are credited and that the original publication in this journal is cited, in accordance with accepted academic practice. No use, distribution or reproduction is permitted which does not comply with these terms.
*Correspondence: Huanzhong Liu, aHVhbnpob25nbGl1QDEyNi5jb20=; Xi Chen, YXlmeWNoZW54aUAxNjMuY29t
Disclaimer: All claims expressed in this article are solely those of the authors and do not necessarily represent those of their affiliated organizations, or those of the publisher, the editors and the reviewers. Any product that may be evaluated in this article or claim that may be made by its manufacturer is not guaranteed or endorsed by the publisher.
Research integrity at Frontiers
Learn more about the work of our research integrity team to safeguard the quality of each article we publish.